Abstract
Advanced analytics offers new means by which to increase efficiency. However, real-world applications of advanced analytics in manufacturing are scarce. One reason is that the management task of selecting advanced analytics technologies (AATs) for application areas in manufacturing is not well understood. In practice, choosing AATs is difficult because a myriad of potential techniques (e.g. diagnostic, predictive, and prescriptive) are suitable for different areas in the value chain (e.g. planning, scheduling, or quality assurance). It is thus challenging for managers to identify AATs that yield economic benefit. We propose a multi-criteria decision model that managers can use to select efficient AATs tailored to company-specific needs. Based on a data envelopment analysis, our model evaluates the efficiency of each AAT with respect to cost drivers and performance across common application areas in manufacturing. The effectiveness of our decision model is demonstrated by applying it to two manufacturing companies. For each company, a customized portfolio of efficient AATs is derived for a sample of use cases. Thereby, we aid management decision-making concerning the efficient allocation of corporate resources. Our decision model not only facilitates optimal financial allocation for operations in the short-term but also guides long-term strategic investments in AATs.
Acknowledgements
The authors would like to thank Geberit Produktions AG in Jona and ABB Turbo Systems AG in Baden for their valuable input on this topic, which greatly assisted the research.
Notes
1 In this paper, AATs subsume all algorithms from the wider realm of artificial intelligence and machine learning that aid data-driven decision-making.
2 In theory, process control represents a natural application for (safe) reinforcement learning techniques (Sutton and Barto Citation2018); however, research in this field is still in its infancy and, instead, problem-specific algorithms are usually developed.
3 For instance, in order to be considered BCC-efficient, the AAT (18.) reinforcement learning must decrease its inputs radially by 0.988 and additionally increase its output for production planning by 0.508 units and for quality driver identification by 1.14 units.
Additional information
Notes on contributors
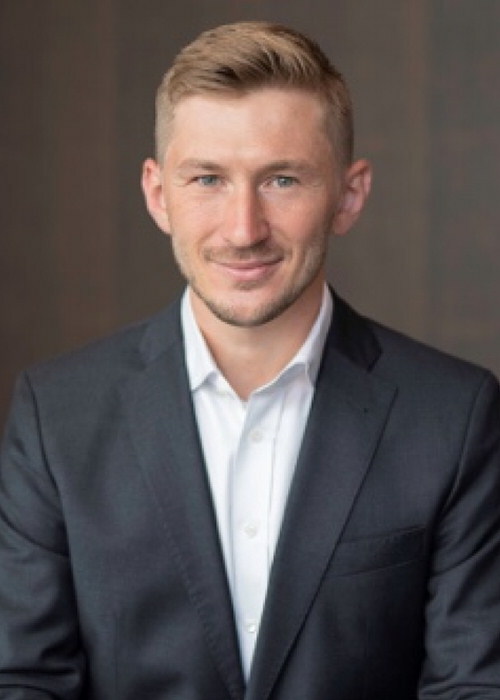
Rafael Lorenz
Rafael Lorenz holds a Ph.D. from ETH Zurich where he graduated from the Chair of Production and Operations Management (POM). His doctoral thesis focused on the effective use of digital technologies to enhance lean production systems. His research was published in leading journals, including the International Journal of Operations & Production Management and the International Journal of Production Research.
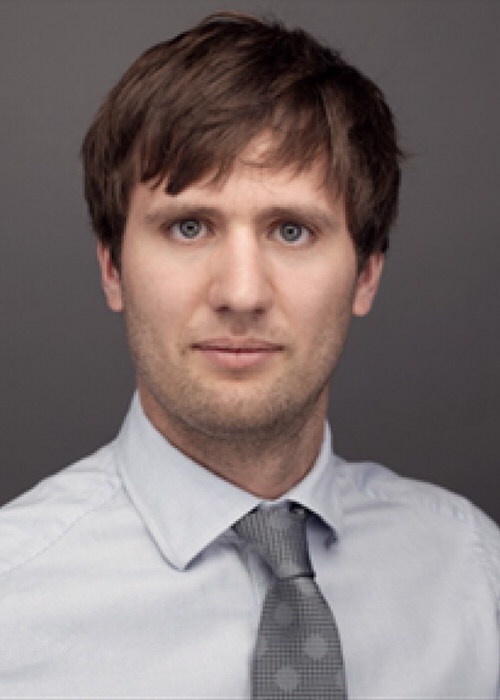
Mathias Kraus
Mathias Kraus holds the Assistant Professorship for Data Analytics at the Institute of Information Systems at FAU Erlangen-Nürnberg. Before that, he worked as a research assistant at ETH Zurich and University of Freiburg. The main objective of his research is to develop innovative methodology from the fields of statistics, machine learning, and big data to contribute to further research developments in data-driven decision support across various tasks.
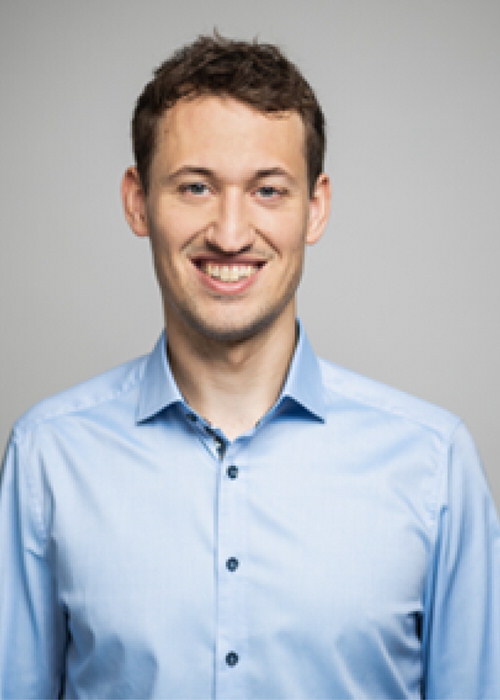
Hergen Wolf
Hergen Wolf studied Industrial Engineering at Technical University Dresden. For his master’s thesis, he joined the Chair of Management Information Systems (MIS) at ETH Zurich. His research focussed on applications of advanced analytics techniques in manufacturing and was published at the APMS. After his studies, Hergen joined Sunfire, a German clean-tech company that develops industrial electrolyzers for the production of renewable hydrogen. As Director Product Management, he is today responsible for Sunfire’s strategic product and service development.
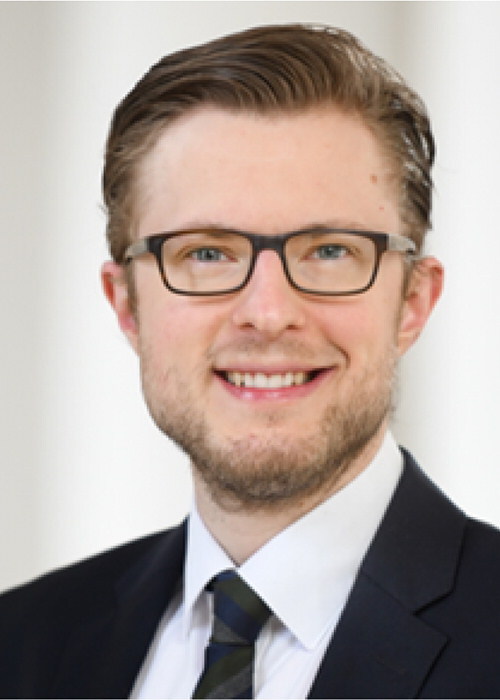
Stefan Feuerriegel
Stefan Feuerriegel is a full professor at LMU Munich School of Management at LMU Munich, where he heads the Institute of AI in Management. Previously, he was an assistant professor at ETH Zurich. In his research, Stefan develops, implements, and evaluates Artificial Intelligence technologies that improve management decision-making.
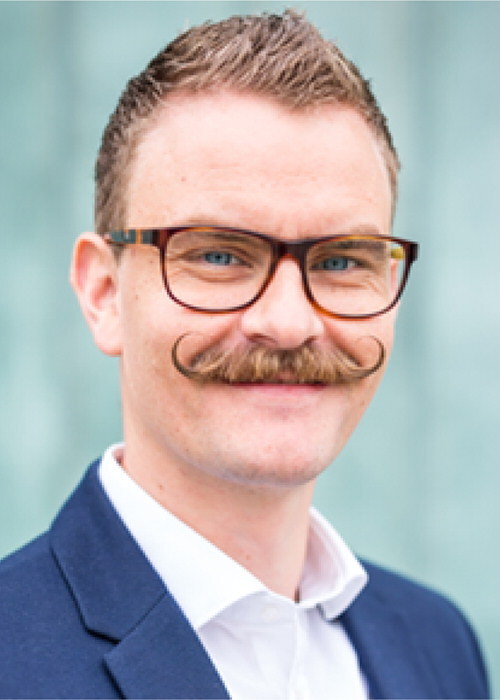
Torbjørn H. Netland
Torbjørn H. Netland is the Head of Chair of Production and Operations Management (www.pom.ethz.ch) at ETH Zurich, Switzerland, and Co-founder of EthonAI. He is a Member of the World Economic Forum’s Global Future Council on Advanced Manufacturing and Value Chains and a Fellow of the European Academy for Industrial Management. His research on manufacturing excellence appears in leading scientific journals, such as Management Science, Journal of Operations Management, Production and Operations Management, MIT Sloan Management Review, International Journal of Operations & Production Management, and Production Planning and Control. He is co-author of the textbook Introduction to Manufacturing: An Industrial Engineering and Management Perspective (Routledge 2022). He is also the recipient of two Shingo Research Awards and numerous teaching awards.