ABSTRACT
Background
Predicting severe preeclampsia with need for intensive care is challenging. To better predict high-risk pregnancies to prevent adverse outcomes such as eclampsia is still an unmet need worldwide. In this study we aimed to develop a prediction model for severe outcomes using routine biomarkers and clinical characteristics.
Methods
We used machine learning models based on data from an intensive care cohort with severe preeclampsia (n=41) and a cohort of preeclampsia controls (n=40) with the objective to find patterns for severe disease not detectable with traditional logistic regression models.
Results
The best model was generated by including the laboratory parameters aspartate aminotransferase (ASAT), uric acid and body mass index (BMI) with a cross-validation accuracy of 0.88 and an area under the curve (AUC) of 0.91. Our model was internally validated on a test-set where the accuracy was lower, 0.82, with an AUC of 0.85.
Conclusion
The clinical routine blood parameters ASAT and uric acid as well as BMI, were the parameters most indicative of severe disease. Aspartate aminotransferase reflects liver involvement, uric acid might be involved in several steps of the pathophysiologic process of preeclampsia, and obesity is a well-known risk factor for development of both severe and non-severe preeclampsia likely involving inflammatory pathways..
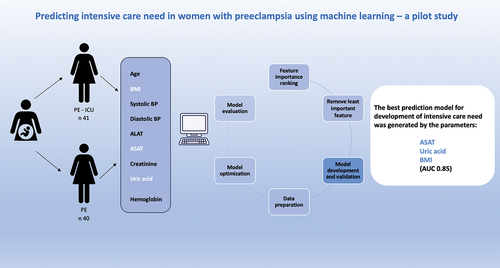
Acknowledgments
This project was supported by the Gorthon Foundation, Helsingborg Hospital, Swedish ALF (Agreement concerning research and education of doctors), Swedish SUS Foundations and the Swedish Research Council (VR).
Disclosure statement
No potential conflict of interest was reported by the author(s).
CRediT authorship contribution statement
Conceptualization: C.E., S.R.H.
Methodology: O.B., C.E., S.R.H.
Software: O.B.
Formal analysis: O.B., C.E.
Investigation: C.E., O.B.
Resources: C.E., O.B., S.R.H.
Data curation: O.B., C.E.
Writing – original draft preparation: C.E., O.B.
Writing – review and editing: all authors.
Visualization: C.E., L.E., S.R.H.
Supervision: S.R.H., L.E.
Project administration: C.E, S.R.H.
Highlights
A prediction model for estimating the risk of intensive care need in preeclampsia.
Machine learning found correlations not shown by traditional logistic regression .
The best parameters for predicting intensive care need were ASAT, uric acid and BMI.
Data availability statement
The datasets generated during and/or analyzed during the current study are available from the corresponding author on reasonable request.
Supplementary material
Supplemental data for this article can be accessed online at https://doi.org/10.1080/10641955.2024.2312165