Figures & data
Figure 4. Bad conditions of the hives and packaging containers; hives on the ground are subject to rot and termite attack.
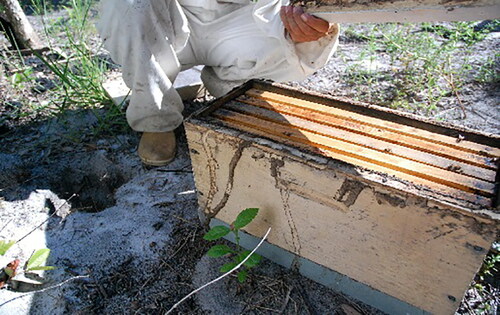
Figure 7. Example of a warehouse for bee pollen production in Brazil. This is one of the largest in Latin America, located in the state of Bahia, northeast Brazil. The main source of pollen is the coconut palm, achieving high standards of excellence and quality of the product: (a) Dehydration room 40 °C; (b) distribution trays; (c) aeration of the dehydrated product room.
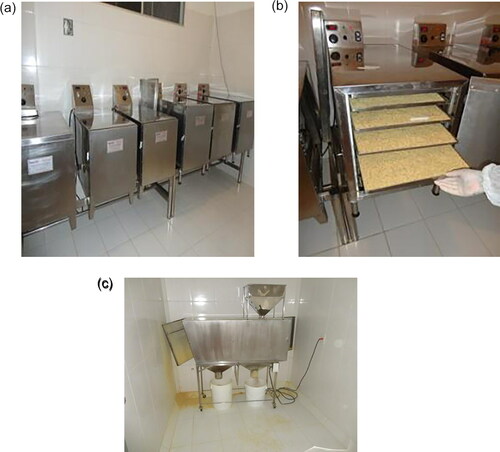
Figure 8. The proposed standard method for detecting unknown pollen loads. The processing chain finishes with an authentication output.
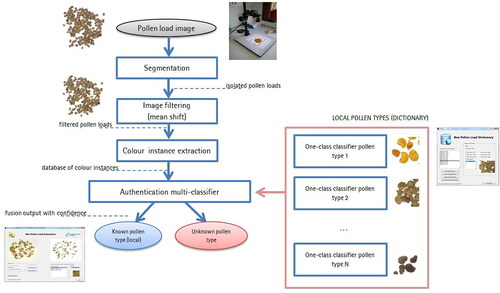
Figure 9. The computer vision system needs inexpensive hardware such as a camera and a stand to control illumination.
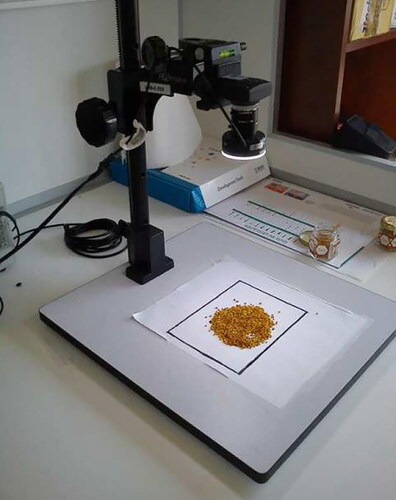
Figure 10. Different images of pollen load samples which were taken with our vision system. Left and central images belong to known pollen types (Rubus, Cistus ladanifer, Quercus ilex, and Echium, respectively). Right images are non-local samples and must be rejected by the system.
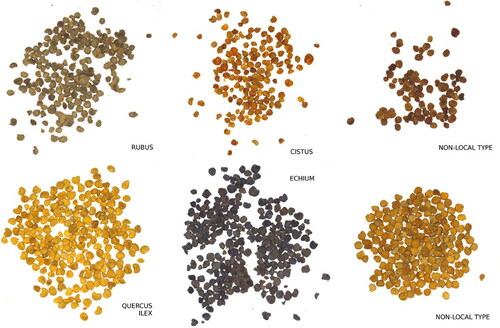
Figure 11. Original image and resulting images after applying the mean shift algorithm (varying spatial bandwidth to 7, 15, and 30, respectively).
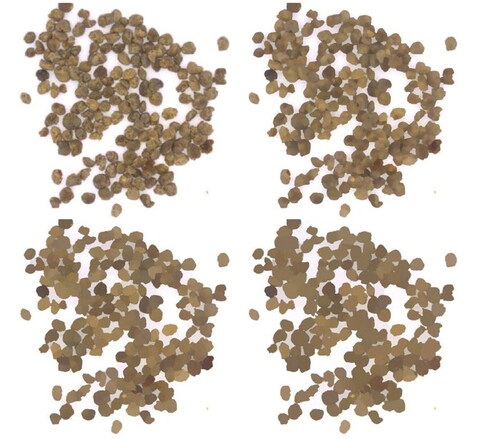
Table 1. Description of the three independent datasets used to train, test the classifiers, and validate the one-class multi-classifier.
Table 2. Evaluation measures obtained by the one-class kNN classifiers for each of the four pollen types.
Table 3. Evaluation measures of the final multi-classifier.
Table 4. Confusion matrix of the multi-classification system formed by the four one-class kNN classifiers (one for each pollen type).
Figure 13. Screenshot of the prototype pollen dictionary. The ambiguity discovery algorithm checks if there is an existing pollen type having identical features compared to the new sample.
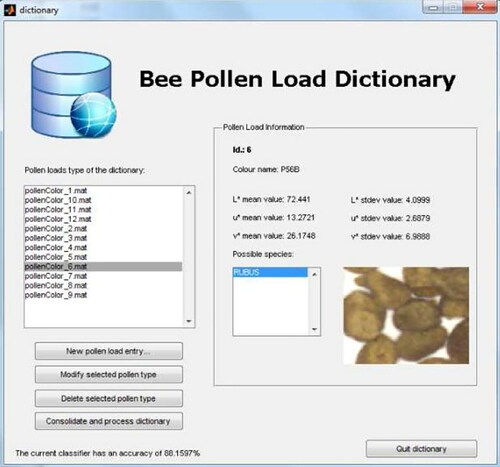
Figure 14. (a and b). Two screenshots of the prototype software detecting a local pollen type in the pollen load images.
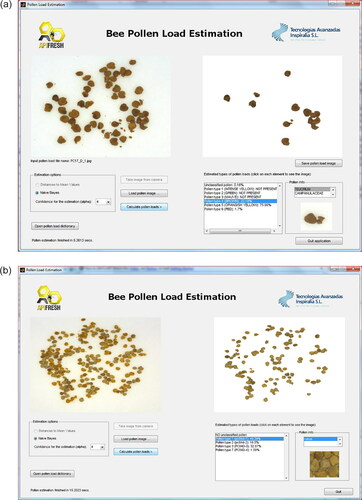
Table 5. Descriptor for quantitative character – principle of completeness.
Table 6. Structure of open and closed intervals in descriptor grades.
Figure 23. Visual representation of the line for the limit points xmin = 1.81; xmax = 4.26 and the coordinate “x4” to the point “y4.”
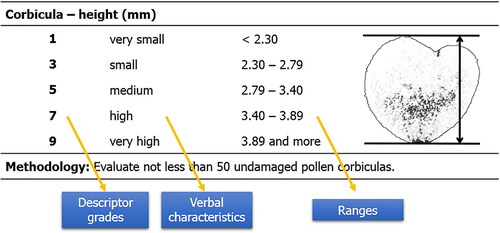
Table 7. Calculated value of variable “x.”
Table 8. Determination of limit points in ranges for five descriptor levels.
Table 9. Ranges of 5 descriptor levels for height.
Table 10. Descriptor for qualitative character – structure of grades.
Table 11. Descriptor for qualitative character – structure of grades in the case of ordinal scale.
Table 12. Structure of alternative character.
Table 13. Complete descriptor with methodology and pictures.
Table 14. Descriptor for characteristic – corbicula – height (mm).
Table 15. Descriptor for characteristic – corbicula – width (mm).
Table 16. Descriptor for characteristic – corbicula – Feret maximum (mm).
Table 17. Descriptor for characteristic – corbicula – Feret minimum (mm).
Table 18. Descriptor for characteristic – corbicula – shape index (width/height).
Table 19. Descriptor for characteristic – corbicula – symmetry (Feret min/Feret max).
Table 20. Descriptor for characteristic – corbicula – area (mm2).
Table 21. Descriptor for characteristic – corbicula – weight (mg).
Table 22. Descriptor for characteristic – corbicula – texture.
Table 23. Descriptor for characteristic – corbicula – shape.
Table 24. Descriptor for characteristic – corbicula – heart shape.
Table 25. Descriptor for characteristic – corbicula – cutout depth.
Table 26. Evaluation of corbicular color (Nôžková, Brindza et al., Citation2010).
Table 27. Descriptor for characteristic – corbicula -color.
Figure 26. Diagrams showing the structure of a pollen grain in optical section (Sawyer, Citation1981).
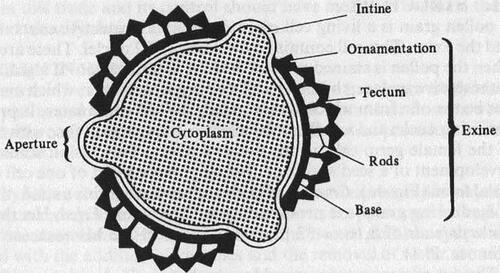
Figure 27. Diagrams showing details of pollen grain walls (Sawyer, Citation1981).
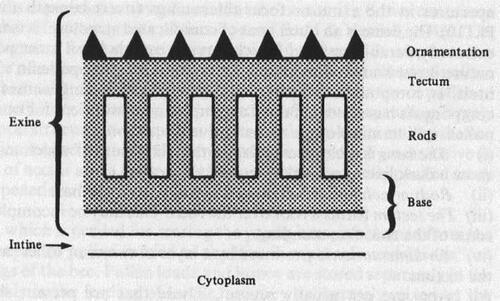
Figure 28. Diagrams illustrating the morphological regions of a pollen grain and pollen grain apertures: (a) equatorial view; (b) polar view (Sawyer, Citation1981).
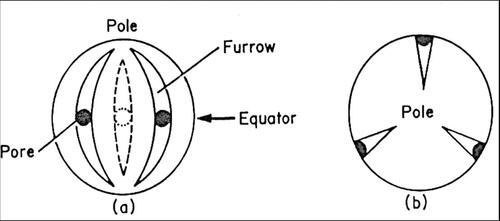
Table 28. Diagnostic pollen grain features used for identification (Vorwohl, Citation1990).
Figure 29. Photos to illustrate the variety of colors of pollen loads of different species of plants: (a) gray – Vicia faba; (b) lemon yellow – Brassica napus; (c) dark gray – Papaver rhoeas; (d) blue – Phacelia tanacetifolia; (e) mixed yellow pollen – mixed pollen from fruit trees (Prunus, Pyrus, Malus) and bright orange – Taraxacum officinale.
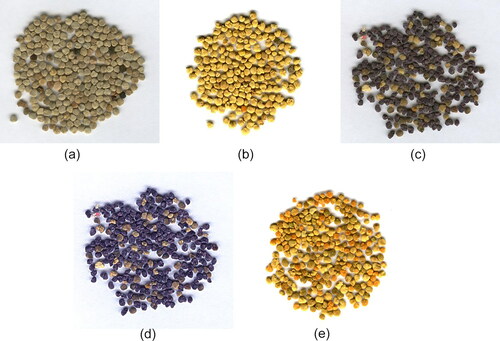
Figure 30. Typical (normal) pollen grains (scanning electron microscopy): (a) Aesculus hippocastanum; and (b) Vicia faba.
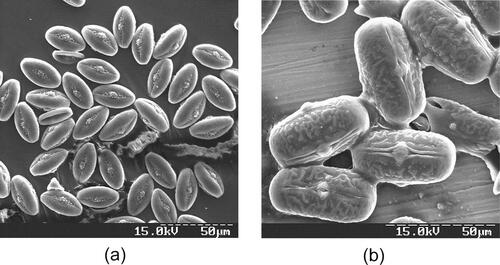
Figure 31. Pollen grains with changed morphological structures (scanning electron microscopy): (a) Phacelia tanacetifolia; (b) Taraxacum officinale; and (c) Scilla bifolia.
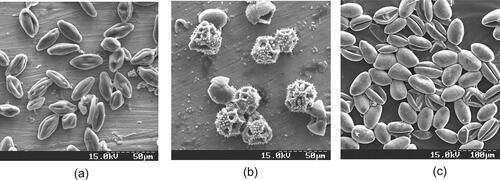
Figure 32. Separation of bee pollen by color pellets from a sample that include different floral origin pollens.
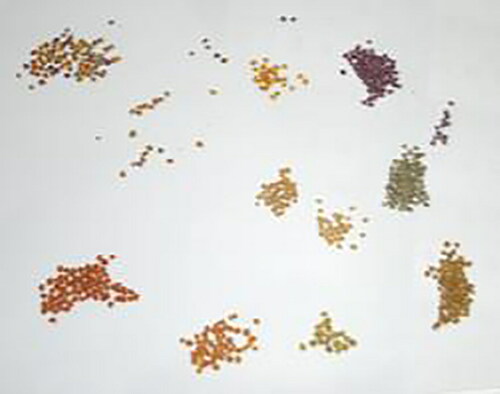
Figure 33. Basic flavonoid structure in order to show a better understanding of the explanation of the UV spectra related to the position of the substitution in the molecule (From Campos & Markham, Citation2007. Reproduced with permission).
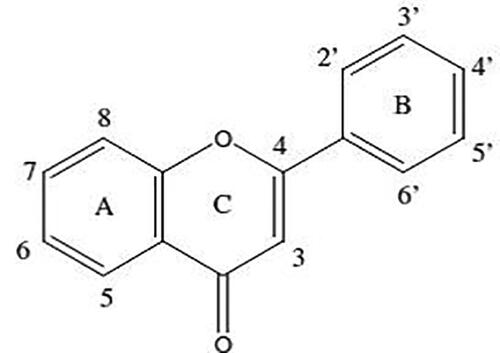
Figure 34. Examples of the more common UV- spectra found in bee pollens: (a) apigenin; (b) apigenin-7-O-derivative; (c) luteolin; (d) kaempferol-3-O-derivative; (e) quercitin-3-O-derivative; (f) isorhamnetin-3-O-derivative; (g) chalcone.
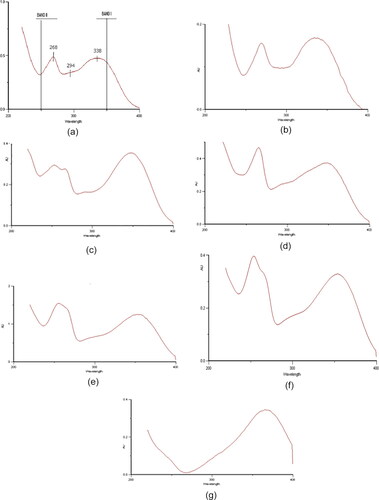
Figure 35. Fingerprint of Eucalyptus globulus obtained with a pollen pellet (100% pure) and a mixture of bee pollen with about 60% of Eucalyptus globulus pollen pellets.
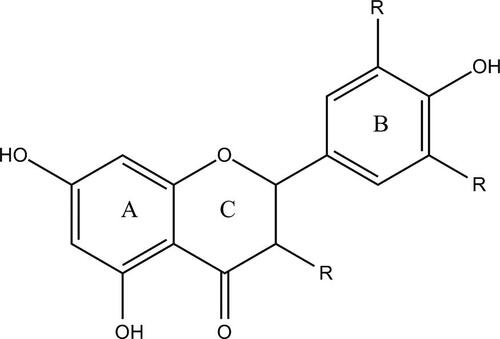
Table 29. Amplitude of the Wavelengths in Band I of flavones and flavonols UV spectra.
Table 30. Wavelengths of Band I in UV spectra of flavonols with a different pattern of oxygenation in B-ring.
Table 31. Wavelengths of Band II in UV spectra of flavones with oxygenation only in A-ring.
Table 32. Model of chemical structures of subclasses or representative molecule of subclasses of non-flavonoids phenolic compounds.
Table 33. Model of chemical structures of flavonoids.
Table 34. Some publications on LC and LC/MS analysis of polyphenolic compounds from bee pollen samples.
Table 37. International data on the protein content (% D.M) of bee pollen.
Table 35. Mass spectral data of compounds detected in extracts of bee pollen.
Table 36. Primer Sequences with indexes SA501 – SB712 (adapted from Kozich et al., Citation2013).
Figure 38. Base peak chromatograms of (a) cherry wine and (b) Cabernet Sauvignon-Central Serbia in positive ion mode. (Pantelić et al., Citation2014).
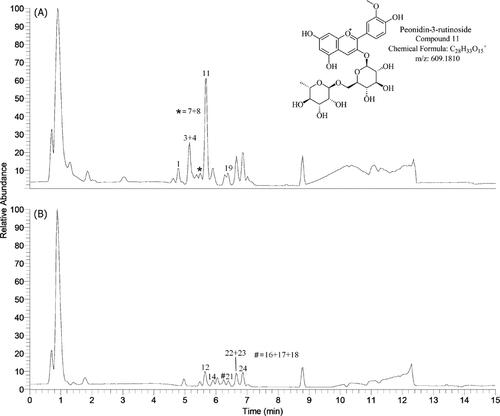
Figure 39. Extracted ion chromatograms and MS/MS spectra of cyanidin-3-rutinoside (Pantelić et al., Citation2014).
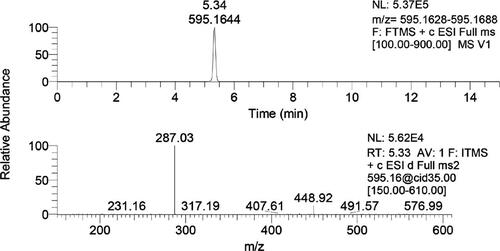
Table 39. Protein content (%) using different distillation times.
Table 40. Protein content (%) analyzing different quantities of pollen.
Table 38. Determination of the protein content (%) using different volumes of the H3BO3 solution.
Table 43. Total lipid content (%) in pollen samples, by using different elution times (3 h, 5 h, 7 h).
Figure 40. (a) Extracted ion chromatograms of phenolic glyceride derivatives with their retention times and accurate masses; (b and c) mass spectra of two phenolic glyceride derivatives with the same accurate masses, m/z 413 (Ristivojević et al. 2014).
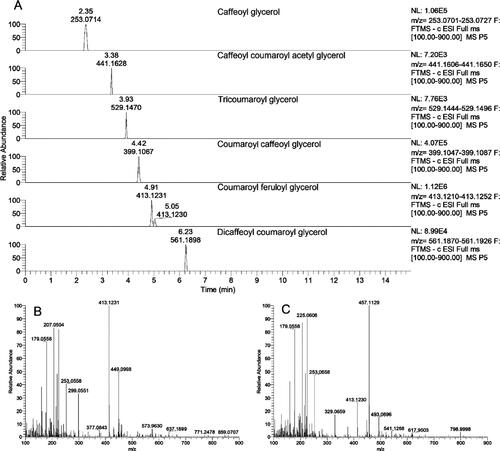
Figure 41. Planning scheme for samples and the corresponding index-combinations. Roman numbers indicate PCR plate numbers, bold Arabian numbers on 96 well plates indicate normalization plate number.
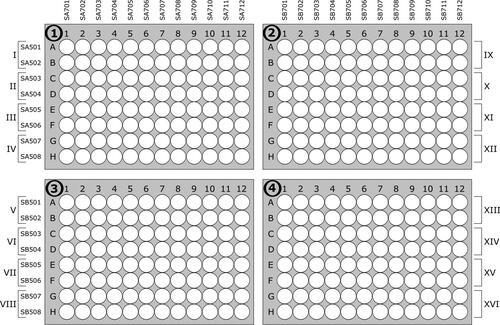
Table 41. International data on the lipid content (% DM) of bee pollen.
Table 42. Total lipid content (%) by using different amount of pollen for the analysis.
Table 44. Total lipid content (%) in pollen samples, by using different solvents (acetone, petroleum ether, hexane).
Figure 42. Detailed workflow (schematic), suitable for laboratories with limited access to equipment for automated pipetting. Bold numbers indicated step number of Design 2 in Section 8.1.3.2.
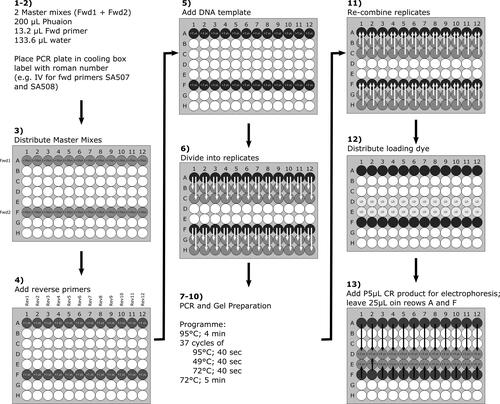
Figure 44. Protein content (% D.M) of mixed pollen samples harvested during the beekeeping season of 2011.
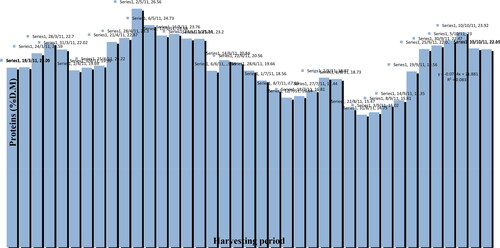
Figure 46. Lipid content (% D.M) of mixed pollen samples harvested during the beekeeping season of 2013.
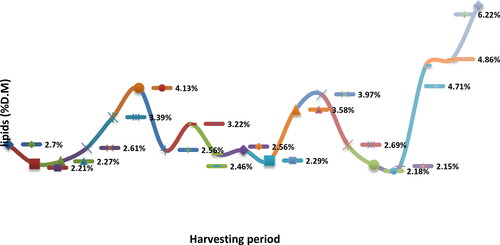
Figure 47. (a) Structure of PAs: Necinic acids are connected to the necine base by ester bond in position 1 and/or 7. Necinic acids can be connected to form a macrocyclic ester; (b) Example of PA free base and N-oxide macrocyclic esters (Hartmann & Witte, Citation1995).
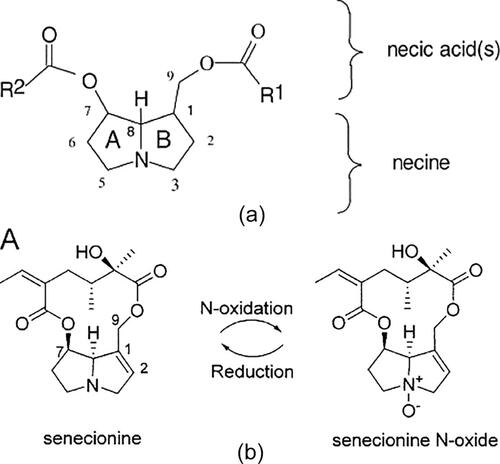
Table 45. Literature data on the carbohydrate content of bee pollen calculated as: 100 – (g fat + g protein + g ash + g water) (g/100 g DM).
Table 46. Literature data on the content of sugars in bee pollen (g/100 g DM).
Table 47. Methods used to evaluate the vitamin content of bee pollen.
Table 48. Calibration curves obtained for echimidine, lycopsamine and intermedine.
Table 51. PAs which have not been previously reported from E. vulgare and E. cannabinum.
Table 50. MS/MS fragment ions for PA-N-oxides from E. vulgare or E. cannabinum.
Figure 52. Extracted ion chromatograms of PAs identified in plant pollen of E. vulgare (A) and E. cannabinum (B). Peak numbers correspond to the PAs described in (1: echimidine-N-oxide; 2: vulgarine-N-oxide; 3: acetylechimidine-N-oxide; 4: acetylvulgarine-N-oxide; 5: echivulgarine-N-oxide; 6: lycopsamine; 7: intermedine; 8-9: lycopsamine-N-oxide or intermedine-N-oxide).
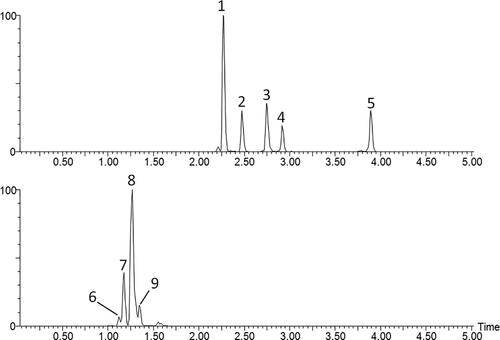
Table 52. Frequency of each plant genus as predominant pollen, secondary pollen, important minor pollen and minor pollen in the 134 pollen samples mixture analyzed.
Table 49. Retention and mass characteristics of known Echium-type and Eupatorium-type PAs (free bases/-N-oxides and isomers).
Table 53. Statistics of the 134 sample of bee pollen analyzed.
Table 54. Results for calibration and cross-validation.
Table 55. Condition variations of the DPPH* assay on bee pollen according to different authors.
Table 56. Advantages and disadvantages of some techniques and methods used for anti-microbial analysis.
Table 57. Summary of some antimicrobial effects of bee pollen.
Figure 57. Microtiter plate (96 wells), showing the color change indicating the reduction of TTC by microorganisms.
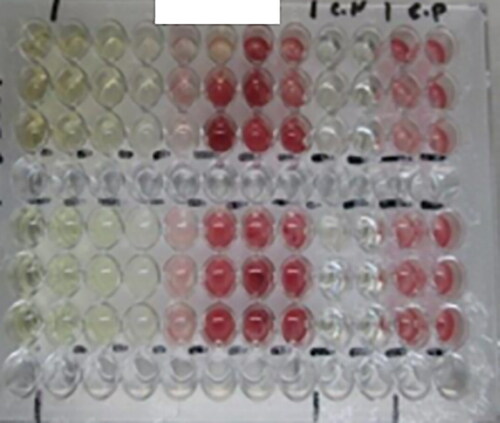