Figures & data
Figure 1. Comparision of multimodal framework (b) and the existing unimodality approaches (a), a notable distinction lies in their treatment of labels. In the case of (a), labels are typically encoded into numerical values or one-hot vectors. However, multimodal framework in (b) leverages the inherent semantic information within the label text itself. The goal of the multimodal framework is to bring video representations corresponding to the labels closer together, driven by the underlying semantics of the label text.
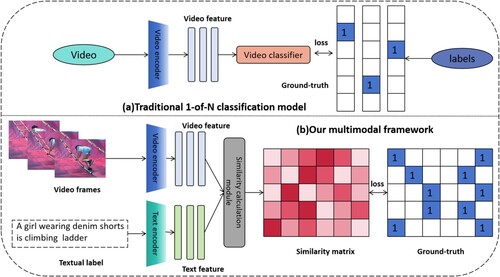
Figure 2. Our approach involves joint training of a video encoder and a text encoder to estimate the correct pairings for a set of training examples that consist of video frames and corresponding texts. During the testing process, the zero shot/few shot classifier calculates similarity scores of video frames and descriptions of the target actions' classes.
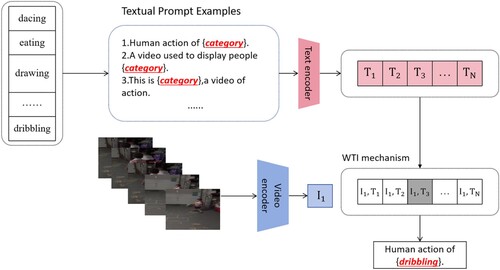
Figure 3. Overview of proposed Vision-Language adaptation. Two single-modal encoders initialise parameters from pre-trained CLIP-based model. A cross-modal linear probing(CMLP) which refers to WTI is designed to calculate similarity among visual and textual tokens. CMLP performs hierarchical cross-modality matching at both semantic-level and feature-level, and chooses key words and relevant frames instead of using average pooling. Kullback–Leibler divergence is defined as the text-video contrastive loss function used to optimise the parameters.
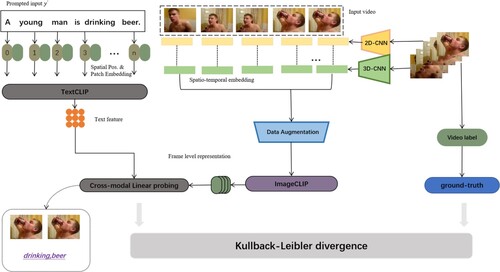
Figure 4. Overview of the proposed disentangled representation learning design for video action recognition. The left part shows the Weighted Token-wise Interaction(WTI) block. The right part shows the details of the network for learning weight, which is composed of typical ResNet architectures.
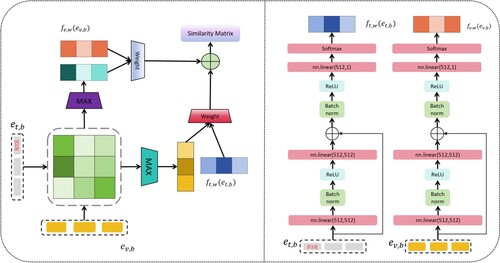
Table 1. Quantitative results on Kinetics-400,UCF-101 and HMDB-51 datasets.
Figure 5. Zero-shot/few-shot results on Kinetics-400 (left), HMDB-51 (middle) and UCF-101 (right). Our approach leads in delivering superior performance in these challenging, data-scarce conditions. It has the capability to achieve zero-shot recognition across all 3 datasets. A capability that STM lacks under the same condition. Additionally, our method excels at few-shot classification.
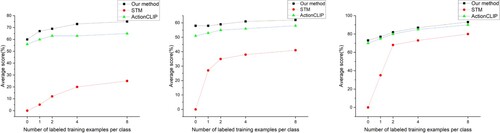
Table 2. Ablation of the ‘pre-train’ step.
Table 3. We adopt the HandEngineered templates selected by Tip-Adapter and CoOp codebase, unless otherwise stated.
Table 4. Influence of backbones and input frames.
Table 5. GFLOPs and inference speed comparison.