Figures & data
Table 1. Maternal characteristics.
Figure 1. The receiver operating characteristic curve for the interaction of the parameters (cervical length in the first trimester and second trimester hCG level) was statistically significant (area under the curve of 0.681, p = .006).
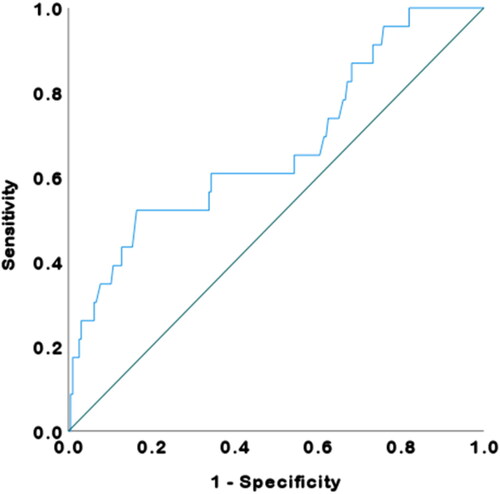
Table 2. Multivariate logistic regression analysis.
Table 3. Neonatal characteristics.
Figure 2. Visualization of the data: each point corresponds to a woman in Group 1 or 2 and is characterized by features a and b, where feature a is the cervical length at the first anatomical scan and feature b is the second trimester hCG level. Clear separation between Groups 1 + 2 and 3 is observed. A Linear Support Vector Machine (LSVM) classification using the MATLAB Classification Learner software was used. The LSVM is a classical machine learning approach widely used in supervised learning to find a line with the largest margin that separates different class data points. Since the available data sets were limited in size, validation of the model was performed using 5-fold cross-validation.
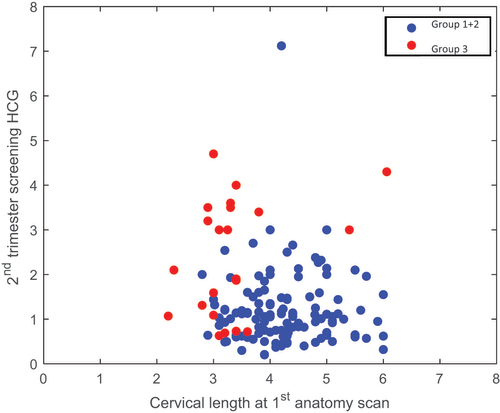
Data availability statement
Data is available upon request.