Figures & data
Table 1 Examples for Candidate Effect Modifiers Identified from the Literature
Figure 1 A simulation study to illustrate the concept of confounding by indication and effect measure modification.
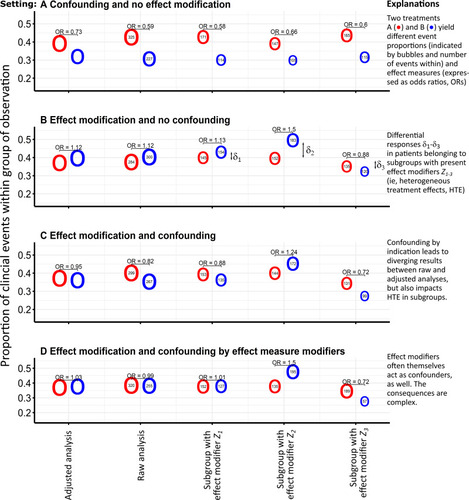
Figure 2 Prediction of individual treatment effects and recommendations from individualized treatment rules.
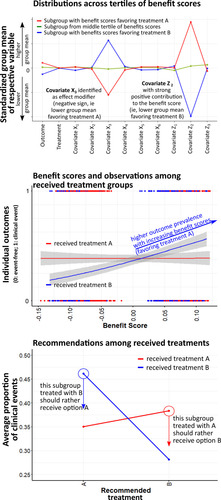