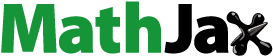
Abstract
Objective
Many patients have medically unexplained physical symptoms (MUPS); some of them attribute their health complaints to dental amalgam fillings. The aim of this study was to assess the validity and responsiveness of General Health Complaints index (GHC-index) for measuring the symptom load in MUPS patients compared to the widely used symptom outcome measure, Giessen Subjective Complaints List (GBB-24).
Methods
Three outcome measures – GHC-index, GBB-24, and Munich Amalgam Scale (MAS) – were administered at baseline and 12 months after removal of all dental amalgam restorations. The validity and responsiveness of these symptom measures were tested against external anchors: bodily distress syndrome (BDS), SF-36 vitality, and visual analogue scale (VAS). We tested both convergent and known group validities. We also examined the predictive validity and responsiveness to changes for each instrument.
Results
All the main outcome measures showed evidence of convergent and known group validities. The GHC-index, GBB-24 and MAS were all able to detect the anticipated differences in BDS and Energy. But the GBB-24 was more efficient in discriminating the BDS compared with the GHC-index (relative efficiency: RE = 0.69; 95% CI: 0.41–0.96) and MAS (RE = 0.59; 95% CI: 0.32–0.86). Each main outcome variable revealed good predictive validity for vitality (standardized coefficient: b ≈ 0.71 and R2 ≈ 0.50). Moderate to high sensitivity to change over time was demonstrated, with GHC-index performing better.
Conclusion
The GHC-index is a valid and responsive instrument for assessing symptom load in MUPS patients attributing their health complaints to amalgam fillings and undergoing amalgam removal.
Introduction
Patients with medically unexplained physical symptoms (MUPS) suffer from persistent health complaints that cannot be sufficiently explained by observable physical pathology despite intensive diagnostic efforts [Citation1,Citation2]. Studies suggest between 3% and 50% of primary care patients present with MUPS [Citation3–6]. Such variations in the prevalence of MUPS could be due to differences in the diagnostic criteria [Citation3]. Evidence suggests that MUPS exists on a continuum of severity, ranging from patients with transient, mild symptoms to those with multiple, debilitating unexplained symptoms [Citation7,Citation8], constituting a major burden with considerable societal costs of direct healthcare or lost productivity. In a Dutch study, the sum of direct healthcare and productivity-related costs were estimated at €6,816 per patient per year [Citation9]. The costs attributable to MUPS due to lost productivity alone is over £5 billion per annum to the UK economy [Citation10], and €7645 per patient per 6-month in Germany [Citation11].
The assessment of the burden of MUPS is important in clinical settings and in the general population for identifying individuals at risk as well as for evaluating treatment effects. Thus, well validated measurement tools are needed. The choice of functional measure for use as a primary outcome in studies of MUPS patients is challenging due to few suitable instruments. The choice of instrument depends on the symptoms and outcomes of interest and the psychometric properties of the instruments [Citation12]. Although a number of outcome measures have been developed to measure the patient’s own perception of symptoms and functional activities, they varied regarding usability and burden to participants as well as relevance to a variety of populations [Citation13].
Some patients with MUPS attribute their health complaints to dental amalgam restorations. In this patient group, there is some evidence of symptom relief after removal of amalgam [Citation14,Citation15]. Among MUPS symptoms, neurological symptoms such as fatigue and dizziness are the most reported complaints attributed to dental amalgam [Citation16]. Pain in muscles and joints, and headache as well as gastrointestinal symptoms are also commonly reported [Citation17]. A General Health Complaints index (GHC-index), which includes common general health complaints in patients referred to the Norwegian Dental Biomaterials Adverse Reactions Unit, has widely been used in Norway [Citation14,Citation15,Citation18,Citation19]. The GHC-index was intended to capture these major symptoms, but its validity and responsiveness have so far not been formally investigated.
Thus, the aim of this study was to assess the validity and responsiveness of GHC-index in MUPS patients who attributed their health complaints to amalgam restorations in relation to a widely used outcome measure for physical complaints of different causes – the 24-item Giessen Subjective Complaints List (GBB-24) [Citation20]. To test the consistency of our results, a comparison will also be made with an instrument previously used in a German intervention study of patients with amalgam-attributed health complaints [Citation21], which we refer hereafter as the Munich Amalgam scale (MAS).
Methods
Study design and data
The analysis was based on a longitudinal prospective cohort study in Norway on MUPS patients who had all amalgam fillings removed. The study was designed using a non-equivalent comparison-group design with pre- and post-test, where three groups were recruited separately. The main target group consisted of patients with MUPS, which they attributed to dental amalgam restorations and who wished to have their amalgam fillings removed (Amalgam cohort; n = 32). The second group included patients with MUPS recruited from general practice without symptom attribution to amalgam fillings (MUPS cohort; n = 28). The last group was participants who identified themselves as healthy (Healthy cohort; n = 19). This analysis is based on the Amalgam cohort. Initially, 49 participants were assessed for inclusion in the Amalgam cohort, of which 12 subjects did not fulfil the eligibility criteria and 5 did not complete the amalgam removal. Thus, a total of 32 participants were available for the follow-up analysis. Detailed recruitment procedures and eligibility criteria were reported elsewhere [Citation14, Citation22].
The research is registered at ClinicalTrials.gov. Identifier: NCT01682278. Date for registration: 10 September 2012, https://clinicaltrials.gov/ct2/show/NCT01682278.
Variable measures
Main outcome measures
Data for three health complaint measures were collected at baseline, and 12 months after removal of amalgam fillings:
General health complaints index (GHC-index)
The GHC-index consists of 12 items: musculoskeletal complaints, gastrointestinal complaints, cardiovascular complaints, skin problems, complaints related to eyes/sight, complaints related to ears/hearing/nose/throat, tiredness, dizziness, headaches, memory problems, difficulty concentrating, and anxiety/depression. For each item, symptom intensity is assessed on a numeric rating scale from 0 (no symptoms) to 10 (worst imaginable symptoms). The sum score for the 12 items ranges from 0 to 120 [19], where lower scores indicate less health complaints. Negative change scores represent improvement.
Health complaints according to the GBB-24
The GBB-24 consists of 24 different health complaints, each rated on a five point severity scale: 0 (not at all), 1 (slightly), 2 (somewhat), 3 (considerably) and 4 (very much) [Citation20]. The complaints are grouped and summarized into four subscales, each with six complaints: Cardiovascular complaints, gastrointestinal complaints, musculoskeletal complaints, and exhaustion. In this analysis, the scores of the 24 single complaints were summed up in a total score (‘complaints load’) ranging from 0 to 96 where 0 is no complaints at all while 96 represent all listed complaints at highest severity. Like the GHC-index, negative change scores in GBB-24 represent improvement.
Munich amalgam scale (MAS)
MAS is a symptom list with 50 items, each with four intensity levels ranging from 0 (not present) to 3 (strong intensity) [Citation21]. The total theoretical summary score is ranging from 0 (no symptom) to 150 (all symptoms of strong intensity).
Anchors
For purposes of examining the validity of GHC-index in MUPS patients attributing their health complaints to amalgam fillings, we used the following variables as external anchors: Bodily Distress Syndrome (BDS) checklist, the Short Form 36-questionnaire (SF-36) Vitality subscale, the Visual Analogue Scale of the EQ-5D instrument (VAS) and the Cantril Ladder of Life Scale (CL) as a measure of life satisfaction. These anchors are selected based on the assumption that they have some relationship with the main outcome measures.
BDS checklist
We applied the BDS checklist, which measures similar daily bothersome physical symptoms such as MUPS, as the main external anchor against which the main outcome variables were compared. The BDS checklist starts with the question ‘have you been bothered by…’ followed by a list of 25 symptom items measured on a 5-point Likert scale from 0 (‘not at all’) to 4 (‘a lot’) [Citation23]. We calculated the sum score by adding the single item scores from the 25 items (ranging from 0 to 100). A recent study validated the BDS checklist total sum score as a measure of symptom burden and illness severity, establishing the usefulness of the BDS checklist in both clinical practice and epidemiological research [Citation24]. We also used the BDS as a binary indicator variable (no BDS versus moderate to severe BDS). We denoted the continuous total sum score of BDS as BDSC to distinguish it from binary BDS.
SF-36 vitality subscale and energy item
One of the most frequent symptoms reported by MUPS patients is fatigue [Citation19]. To capture this, we used the Vitality scale of the SF-36 instrument [Citation25] as an external anchor against which we tested the validity of the main outcome measures. The Vitality scale assesses energy and fatigue to capture differences in quality of life, and is based on four questions: How much of the time during the past 4 weeks (i) did you have a lot of energy? (ii) have you felt full of life? (iii) did you feel worn out? and iv) did you feel tired? Each question has a five-point scale ranging from none of the time to all of the time. The total summary score ranges from 0 to 100, with lower score indicating less vitality. In general, Vitality is hypothesized to be highly associated with the main outcome variables since they measure similar clinical phenomena (fatigue and tiredness). To test the discriminative ability of each outcome variable, we also considered the first question, Energy, as a categorical variable at follow-up.
VAS
To check the consistency of our results, we also used VAS as an external anchor against which the main outcome variables were compared. VAS records the respondent’s self-rated health on a vertical scale, where the end points are labelled 0 (‘the worst imaginable health’) and 100 (‘the best imaginable health’). The respondents were asked to choose on any point of the VAS scale that best represents their health. The VAS scores were summarized and analyzed as continuous data.
CL life satisfaction
The CL is a self-reported measure of life satisfaction in response to the question: Please imagine a ladder with steps numbered from zero at the bottom to ten at the top. Suppose we say that the top of the ladder represents the best possible life for you and the bottom of the ladder represents the worst possible life for you. On which step of the ladder do you feel you personally stand at the present time? CL is treated as a continuous variable. In the present study we used a scale from 1 (worst possible life) to 10 (best possible life).
Validation analysis
A measurement tool is said to be valid if it measures what it intends to measure. However, it is difficult to ascertain that a measure is valid in the absence of a gold standard measure against which we compare [Citation12], and, thus, validation is a process of hypothesis testing to increase confidence that a measurement scale has the properties that would be expected if it was valid. Validation tests are variously classified; we here present tests of convergent, known group and predictive validity, as well as responsiveness over time and of reliability test.
Internal consistency and convergent validity
Internal consistency was tested using Cronbach’s alpha (α). It is a statistic commonly used to assess whether instruments that have been constructed measures what they intend to measure [Citation26]. This statistic was estimated for each of the main outcome measures at both baseline and follow-up. The commonly used cut-off points for inferring adequate internal consistency was: α > 0.70 [Citation26,Citation27].
Convergent validity assesses the strength of the relationship between measures. To determine the degree to which the symptom measures are related to other measures of similar construct, convergent validity was examined by comparing them to the scores reported on the BDSC, Vitality, VAS, and CL at follow-up using Spearman’s correlation coefficients (rho, ρ). We expected strong correlations between the BDSC and the symptom measures, as well as the Vitality. Correlation analysis can indicate the degree to which instruments are measuring related factors. Absolute correlation strength is classified as weak (<0.3), moderate (0.3 to <0.5), and strong (>0.5) [Citation28].
Known-group validity
Known-group validity assesses the extent to which instrument scores differ across groups that are expected to differ and was used to examine the discriminative validity of each of the symptom measures. The BDS and the SF-36 item Energy were used as external anchors. Subjects with poorer health status were hypothesized to have lower scores on the main outcome measures. The Kruskal–Wallis test and relative efficiency (RE) were used to explore the known-group validity of different symptom measures. The RE statistic could be defined as the ratio of either chi-squared () statistics or squared t statistics, and can be used to evaluate the sensitivity of different main outcome measures to known group differences [Citation29]. Here, RE is defined as the ratio of
where GBB-24 was used as a reference in the denominator. Thus, a RE value less than 1 implies that the GBB-24 is more able to discriminate between meaningfully different groups (e.g. level of Energy or BDS), and the inverse is true for an RE value of greater than 1.
Predictive validity
Predictive validity was tested by the ability of GHC-index to predict changes in the symptom or health predicted by other instruments (GBB-24 and MAS). We applied binary logistic regression models to evaluate the ‘predictive validity’ of each symptom measure as predictor of unfavourable outcomes at follow-up: (a) low self-rated health; and (b) moderate/severe BDS type. Let Yi denote the binary independent variable (e.g. 1 for ‘low self-rated health’ and 0 for ‘high self-rated health’), and Xi is one of the main outcome measures. The model is given by:
where
denote the maximum likelihood of the success probabilities, β0 and β1 are constant parameters to be estimated, and
is the error term.
The increased odds of having an unfavourable outcome for a one SD change in each of the symptom measures (standardized coefficient of X) were calculated to facilitate the comparison of the predictive effects of each instrument (GHC-index, GBB-24 and MAS) measured in different scales. The standardization of X alone produces the relative importance of X. We also reported the coefficient of discrimination (D) for logistic regression [Citation30], which is closely related to the classical coefficient of determination (R2) in linear regression. It is given by the difference between the mean predicted probabilities for successes () and failures (
), and hence, used as a standard measure of explanatory power [Citation28]. That is,
Furthermore, we applied ordinary least square linear regression models to determine the ability of each measure to predict vitality as well as the bodily distress syndrome: Here,
is a continuous response variable (measured by Vitality or BDSC), and all others are as defined before. In addition to the standardized β coefficients, the amount of total variance explained (R2) in Vitality or BDSC was used to compare the predictive validity across main outcome measures.
Responsiveness
Responsiveness is defined as the degree to which a measure detects meaningful change. Meaningful change can be determined using either distribution-based methods (statistical distributions of change and associated reliability) or anchor-based methods (external criterion of change reflecting a patient or clinician’s perspective [Citation31]. In the present study, we calculated the following metrics to measure the responsiveness of the main outcome variables: mean change score (MCS), effect size (ES), standard response mean (SRM), standard error of measurement (SEM) and minimal detectable change (MDC).
Effect size and standard response mean
To provide a metric of responsiveness independent of direction, we computed absolute ES for each outcome measure: ES = abs((M2 − M1))/S1, where M2 is the mean score at follow-up, M1 is the mean score at baseline, and S1 is the standard deviation (SD) of the baseline. The SRM is also a measure of effect size index used to gauge the responsiveness of scales to clinical change. The SRM is computed in a similar way as the ES but using the standard deviation of the mean change in the denominator. The thresholds for interpreting ES values are: small (0.20), medium (0.50), and large (0.80) [Citation28]. The same thresholds applied for interpreting SRM.
Standard error of measurement
The SEM is the variation in measured symptom attributed to the unreliability of outcome measures, where a change smaller than the value of SEM would likely be due to measurement error instead of a true observed change [Citation32]. The SEM is a theoretically fixed test characteristic of any measure and not sensitive to the number of participants in a study [Citation33]. It is calculated as: SEM = S1(1 − α), where α is the reliability coefficient. In this analysis, the value for the reliability coefficient was estimated by the internal consistency reliability, usually referred to as Cronbach’s alpha (α), as suggested in the literature [Citation34,Citation35]. For the derivation of SEM, the value of α at follow-up period was used.
There is no standard threshold value for SEM to indicate an individual’s score change as the smallest meaningful change, though ±1 SEM (equivalent to 63% confidence interval) is a frequently used threshold [Citation36]. However, a more conservative criterion of ±1.645 SEM could be considered as the safest threshold for identifying statistically detectable individual score change, which is equivalent to 90% confidence interval for SEM [Citation36,Citation37]. We used this conservative criterion (±1.645*SEM). Thus, SEM provides a measure of variability and is primarily used to compute the minimally detectable change (MDC) described below.
Minimal detectable change
The MDC is the minimum amount of change in a patient’s score that ensures the change is not the result of measurement error [Citation37]. It is calculated in terms of confidence of prediction, and hence, MDC scores with 90% confidence (MDC90) were calculated as: SEM*Z90*√2, where z is the z-value for the 90% confidence level [Citation38]. The multiplier of √2 is to account for the additional uncertainty introduced by using different scores from measurements at 2 time points – baseline and follow-up. The MDC90 corresponds to the smallest amount of change that falls outside of measurement error. The percentage of participants who demonstrated a change ≥ the MDC90 from baseline to follow-up was calculated for each measure.
Results
Reliability and convergent validity
Internal consistency and convergent validity of the main outcome measures are reported in . Cronbach’s alpha for internal consistency exceeded 0.80 for all main outcome variables, indicating excellent internal consistency. There was evidence of strong convergent validity (ρ ≈ 0.50 and above) for most combination of main outcome variables (GBB-24, GHC-index, MAS) and anchor variables (BDSC, VAS, Vitality and CL) at both baseline and follow-up. Exceptions were for baseline observations between VAS and MAS and between Vitality and MAS, where moderate convergent validity was found. At follow-up, the highest correlation was observed between GBB-24 and BDSC (ρ = 0.94), followed by the correlation between GHC-index and BDSC (ρ = 0.83).
Table 1. Cronbach’s alpha for reliability test and correlation coefficients for convergent validity.
Known group validity
Known group validity is reported in , using Chi-squared statistics and RE values. All outcome measures showed evidence for known-group validity in detecting significant (p < .001) differences between different status of bodily distress syndrome and Energy, being used as the known group variables. Compared to GBB-24, the GHC-index and MAS were less efficient in discriminating BDS, with the RE being significantly less than 1. However, there was no significant difference across the outcome variables in discriminating patient ratings of their energy.
Table 2. Known group validity: Kruskal–Wallis statistics and relative efficiency of GHC-index and MAS against GBB-24.
Predictive validity
Predictive validity is presented in . In the upper panel A, the logistic regression models show the odds for an unfavourable outcome (low self-reported health, moderate to severe BDS) for every 1 standard deviation (SD) increase in each of the main outcome measures (GBB-24, GHC-index, MAS). All three main outcome measures showed high predictive validity for low self-rated health at follow-up, with GHC-index performing best: a 1 SD increase in GHC-index leads to a 1.464 increase in the log-odds of having low self-rated health. A similar pattern was observed when using the coefficient of discrimination. Similarly, high predictive validity for moderate to severe BDS was observed across the main outcome measures, particularly for GBB-24 as demonstrated by high coefficient of discrimination (0.746) and greater standardized coefficient. For instance, a 1 SD increase in GBB-24 resulted, on average, in almost 6.8 increase in the log-odds of having bodily distress syndrome. The corresponding values for 1 SD increase in GHC-index and MAS were 2.803 and 1.741, respectively.
Table 3. Predictive validity of GBB-24, GHC-index and MAS: logistic and ordinary least square regressions.
In the lower panel B of , predictive validity of Vitality and BDSC in ordinary least square regression models is presented. The three main outcome variables were equally good predictors of Vitality, with similar standardized coefficients (≈ 0.71) and coefficient of determination (R2 ≈ 0.50). The predictive validity for BDSC was also comparable across measures, with GBB-24 performing better. For instance, GBB-24 was the best predictor, explaining the highest percentage of the variability in BDSC checklist (R2 = 0.887), followed by the GHC-index and MAS (R2 = 0.696 and 0.666, respectively).
Responsiveness
Responsiveness, independent of direction, is presented in . Mean differences in the pre- and post-treatment scores were significantly different for all three outcome measures (p < .001, paired t-tests), with GHC-index showing the highest mean score changes. Moderate to large absolute SMR were observed. For the GBB-24 and MAS, moderate SRMs were observed (0.66 and 0.67, respectively). For the GHC-index, we observed large SRM (0.81). All outcome measures revealed moderate ES, with GHC-index performing best. The percentages of participants with meaningful changes in either direction (a change ≥ MDC90) for each outcome measure varied between 43.8% (for GBB-24) and 56.3% (for GHC-index), with the GHC-index performing slightly better than both MAS and GBB-24.
Table 4. Responsiveness of the symptom load measures: GHC-index, GBB-24 and MAS.
Discussion
This analysis contributes to the knowledge of the psychometric properties of questionnaires used to measure symptom load in MUPS patients. This is important for monitoring of symptom change in similar studies and other interventions on MUPS patients. The purpose of this study was, therefore, to determine the validity and responsiveness of GHC-index as compared with two other instruments – GBB-24 and MAS – in patients with MUPS attributed to dental amalgam restorations undergoing amalgam removal.
In our analyses, the GHC-index was an economical, reliable, and valid symptom-specific instrument for the assessment of MUPS in in patients who attribute their MUPS to amalgam restorations. Cronbach’s alpha for GHC-index at both baseline and follow-up was very high (α ≥ 0.80), indicating an excellent internal consistency of the instrument. Similar results were also obtained for the comparators (GBB-24 and MAS).
To our knowledge, this is the first analysis of the convergent validity of GHC-index. In our study, the correlations of the GHC-index with different anchors were all significant, with Spearman rank order correlations greater than 0.50 both at baseline and follow-up. All outcome measures showed strong correlation with the four anchors, particularly with BDS that cover similar domains (ρ > 0.80), indicating that the instruments are measuring related aspects of the same underlying construct. Furthermore, our results confirmed the ability of the GHC-index to discriminate between different severity levels of BDS and Energy in MUPS patients with amalgam attribution, and so do the GBB-24 and MAS. All outcome measures are similar in discriminating the levels of Energy, and hence, there is no statistical difference in their discriminative efficiency of Energy in the present patient group. However, the GBB-24 was more efficient than both GHC-index and MAS in discriminating the BDS severity levels. This is not surprising because GBB-24 measures similar symptom loads with BDS as compared to other instruments. In general, each symptom instrument significantly discriminated between known groups (e.g. by the levels of Energy or no BDS vs moderate to severe BDS).
Our results from linear and logistic regression on predictive validity of these instruments supported this finding. For instance, the predictive ability of GBB-24 for BDS was 74.6% using logistic regression and 88.7% for linear regression. The respective values for GHC-index were 38.0% and 69.6%. All symptom measures performed quite similarly in predicting vitality. In the prediction of self-reported health, the highest coefficient of discrimination is associated with the GHC-index, indicating greater predictive validity by this instrument.
Other measures of responsiveness produced consistent results, with all main outcome measures showing good responsiveness, with the GHC-index performing slightly better. Large SRM was observed for GHC-index, indicating stronger responsiveness compared to other measures. Similarly, the percentages of participants demonstrating a change ≥ the MDC90 was the largest for the GHC-index (56.3%), followed by MAS (46.9%). This again shows the usefulness of specific questionnaires aimed at the actual patient group.
Strengths of the study were extensive screening procedures and high-quality treatment protocols for amalgam removal following generally accepted guidelines [Citation14]. Furthermore, the clinical screening and examination performed by dentists and additional information from general practitioners limited the probability that the presence of health complaints could be explained by other diseases. Finally, we addressed both validity and responsiveness with multiple approaches and several alternative anchors that enable us to confirm the consistency of our results.
Some limitations of this study must be considered. Due to the small sample size, variability in parameter estimates were relatively wide. Nonetheless, the presence of statistically significant results indicate that the study provided good evidence about the reliability and usefulness of the instruments applied. The patients in the amalgam cohort had to send an application to the study office to be included in the study and their inclusion in the study was subject to several selection criteria, including the desire to have their amalgam restorations removed [Citation14]. Thus, the findings of this analysis may not be generalizable to MUPS patients without amalgam restorations nor to patients who do not attribute their health complaints to dental amalgam.
In conclusion, the analyses indicate that GHC-index had acceptable construct validity and internal consistency reliability when used with patients with health complaints attributed to amalgam restorations. In this respect, all outcome measures have good discriminative power. The mean change score as diagnostic test and other alternative measures of responsiveness suggest that the GHC-index is responsive to change. The comparison with a validated instrument – GBB-24 – support our conclusion. However, firm conclusions cannot be made until our findings have been confirmed in other studies using additional indicators with larger sample size.
Ethics approval
The trial was approved by the local research ethics committee (REK2012/331) and registered at ClinicalTrials.gov (https://clinicaltrials.gov/ct2/show/NCT01682278).
Consent to participate
Written informed consent was obtained from all participants in this study.
Acknowledgement
The study was funded by Norwegian Ministry of Health and Care Services via the Norwegian Directorate of Health. The funder has no role in the design of the study, statistical analyses, or interpretation of the results.
Disclosure of interest
The authors report no conflict interests.
Data availability statement
The datasets generated and analyzed during the current study are not publicly available due to privacy concern, as relatively few patients participated in the study with implications for potential identification through personal characteristics.
Additional information
Funding
References
- Konnopka A, Schaefert R, Heinrich S, et al. Economics of medically unexplained symptoms: a systematic review of the literature. Psychother Psychosom. 2012;81(5):265–275.
- Jadhakhan F, Lindner OC, Blakemore A, et al. Prevalence of medically unexplained symptoms in adults who are high users of health care services: a systematic review and meta-analysis protocol. BMJ Open. 2019;9(7):e027922.
- Burton C. Beyond somatisation: a review of the understanding and treatment of medically unexplained physical symptoms (MUPS). Br J Gen Pract. 2003;53(488):231–239.
- Kroenke K. Efficacy of treatment for somatoform disorders: a review of randomized controlled trials. Psychosom Med. 2007;69(9):881–888.
- Rasmussen EB. Making and managing medical anomalies: exploring the classification of 'medically unexplained symptoms'. Soc Stud Sci. 2020;50(6):901–931.
- Aamland A, Malterud K, Werner EL. Patients with persistent medically unexplained physical symptoms: a descriptive study from Norwegian general practice. BMC Fam Pract. 2014;15(1):107.
- Brown RJ. Introduction to the special issue on medically unexplained symptoms: background and future directions. Clin Psychol Rev. 2007;27(7):769–780.
- Edwards TM, Stern A, Clarke DD, et al. The treatment of patients with medically unexplained symptoms in primary care: a review of the literature. Ment Health Fam Med. 2010;7(4):209–221.
- Zonneveld LNL, Sprangers MAG, Kooiman CG, et al. Patients with unexplained physical symptoms have poorer quality of life and higher costs than other patient groups: a cross-sectional study on burden. BMC Health Serv Res. 2013;13(1):520.
- Bermingham SL, Cohen A, Hague J, et al. The cost of somatisation among the working-age population in England for the year. Ment Health Fam Med. 2010;7(2):71–84.
- Konnopka A, Kaufmann C, König H-H, et al. Association of costs with somatic symptom severity in patients with medically unexplained symptoms. J Psychosom Res. 2013;75(4):370–375.
- Richardson J, Iezzi A, Khan MA, et al. Validity and reliability of the assessment of quality of life (AQoL)-8D multi-attribute utility instrument. Patient. 2014;7(1):85–96.
- Zijlema WL, BioSHaRE, Stolk RP, Löwe B, et al. How to assess common somatic symptoms in large-scale studies: a systematic review of questionnaires. J Psychosom Res. 2013;74(6):459–468.
- Björkman L, Musial F, Alraek T, et al. Removal of dental amalgam restorations in patients with health complaints attributed to amalgam: a prospective cohort study. J Oral Rehabil. 2020;47(11):1422–1434.
- Sjursen TT, Lygre GB, Dalen K, et al. Changes in health complaints after removal of amalgam fillings. J Oral Rehabil. 2011;38(11):835–848.
- Vamnes JS, Lygre GB, Grönningsaeter AG, et al. Four years of clinical experience with an adverse reaction unit for dental biomaterials. Community Dent Oral Epidemiol. 2004;32(2):150–157.
- Melchart D, Wuhr E, Weidenhammer W, et al. A multicenter survey of amalgam fillings and subjective complaints in non-selected patients in the dental practice. Eur J Oral Sci. 1998;106(3):770–777.
- Lygre GB, Gjerdet NR, Björkman L. A follow-up study of patients with subjective symptoms related to dental materials. Commun Dent Oral Epidemiol. 2005;33(3):227–234.
- Lygre GB, Sjursen TT, Svahn J, et al. Characterization of health complaints before and after removal of amalgam fillings-3-year follow-up. Acta Odontol Scand. 2013;71(3–4):560–569.
- Brähler E, Schumacher J, Brähler C. Erste gesamtdeutsche normierung der kurzform des gießener beschwerdebogens GBB-24. [First standardization of the short version of the Giessen subjective complaints list GBB-24 in re-unified Germany]. PPmP: Psychotherapie Psychosomatik Medizinische Psychologie. 2000;50(1):14–21.
- Melchart D, Vogt S, Kohler W, et al. Treatment of health complaints attributed to amalgam. J Dent Res. 2008;87(4):349–353.
- Lamu AN, Björkman L, Hamre HJ, et al. Validity and responsiveness of EQ-5D-5L and SF-6D in patients with health complaints attributed to their amalgam fillings: a prospective cohort study of patients undergoing amalgam removal. Health Qual Life Outcomes. 2021;19(1):125.
- Budtz-Lilly A, Fink P, Ørnbøl E, et al. A new questionnaire to identify bodily distress in primary care: the 'BDS checklist'. J Psychosom Res. 2015;78(6):536–545.
- Petersen MW, Rosendal M, Ørnbøl E, et al. The BDS checklist as measure of illness severity: a cross-sectional cohort study in the Danish general population, primary care and specialised setting. BMJ Open. 2020;10(12):e042880.
- Brazier JE, Harper R, Jones NM, et al. Validating the SF-36 health survey questionnaire: new outcome measure for primary care. BMJ. 1992;305(6846):160–164.
- Taber KS. The use of Cronbach’s alpha when developing and reporting research instruments in science education. Res Sci Educ. 2018;48(6):1273–1296.
- Cortina JM. What is coefficient alpha? An examination of theory and applications. J Appl Psychol. 1993;78(1):98–104.
- Cohen J. A power primer. Psychol Bull. 1992;112(1):155–159.
- Hays RD, Anderson R, Revicki D. Psychometric considerations in evaluating health-related quality of life measures. Qual Life Res. 1993;2(6):441–449.
- Tjur T. Coefficients of determination in logistic regression models—a new proposal: the coefficient of discrimination. Am Stat. 2009;63(4):366–372.
- de Vet HC, Terwee CB, Ostelo RW, et al. Minimal changes in health status questionnaires: distinction between minimally detectable change and minimally important change. Health Qual Life Outcomes. 2006;4:54–54.
- Copay AG, Subach BR, Glassman SD, et al. Understanding the minimum clinically important difference: a review of concepts and methods. Spine J. 2007;7(5):541–546.
- Nunnally JC, Nunnaly JC. Psychometric theory. New York, NY: McGraw-Hill; 1978.
- Wyrwich KW. Minimal important difference thresholds and the standard error of measurement: is there a connection? J Biopharm Stat. 2004;14(1):97–110.
- Wyrwich KW, Nienaber NA, Tierney WM, et al. Linking clinical relevance and statistical significance in evaluating intra-individual changes in health-related quality of life. Med Care. 1999;37(5):469–478.
- Brunner HI, Higgins GC, Klein-Gitelman MS, et al. Minimal clinically important differences of disease activity indices in childhood-onset systemic lupus erythematosus. Arthritis Care Res. 2010;62(7):950–959.
- de Vet HC, Terwee CB. The minimal detectable change should not replace the minimal important difference. J Clin Epidemiol. 2010;63(7):804–805.
- Haley SM, Fragala-Pinkham MA. Interpreting change scores of tests and measures used in physical therapy. Phys Ther. 2006;86(5):735–743.