ABSTRACT
In many settings, fleets of assets must perform series of missions with in-between finite breaks. For such fleets, a widely used maintenance strategy is the fleet selective maintenance (FSM). Under resource constraints, the FSM problem selects an optimal subset of feasible maintenance actions to be performed on a subset of components to minimise the maintenance cost while ensuring high system reliability during the upcoming mission. The majority of extant FSMP models are focussed on traditional physics-based reliability models. With recent advances in Machine Learning (ML) and Deep Learning (DL) algorithms, data-driven methods have shown accuracy in predicting remaining useful life (RUL). This paper proposes a predictive FSM strategy for fleets of complex and large multicomponent systems. It relies on a concurrent ML/DL and optimisation approach where a clustering algorithm is used to determine the health states of components and a probabilistic RUL prognostics model is used for component reliability assessment. An optimisation model is developed to solve the predictive FSM problem to ensure high reliability of all systems within the fleet. An efficient two-phase solution approach is developed to solve this complex optimisation problem. Numerical experiments show the validity of the approach and highlight the improved maintenance plans achieved.
Disclosure statement
No potential conflict of interest was reported by the author(s).
Data availability statement
The authors confirm that the data supporting the findings of this study are available within the article.
Additional information
Funding
Notes on contributors
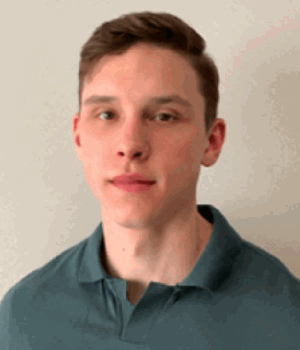
R. O'Neil
Ryan O'Neil is currently pursuing a Ph.D. degree from the Department of Industrial Engineering at Dalhousie University, Halifax, Nova Scotia, Canada. He holds a Master of Applied Science degree and a bachelor's degree in Industrial Engineering from Dalhousie University. His current research interests include machine learning, large-scale and robust optimisation of intelligent maintenance operations.

A. Khatab
Abdelhakim Khatab Ph.D., is Professor at Université de Lorraine (France). After graduating from the High Normal School (ENS-Mohammedia, Morocco), He received a DEA (Msc degree) and a PhD in Industrial automation engineering from National Institute of Applied Science (INSA) of Lyon (France). Since 2018, Dr. Khatab is adjunct Professor at the Department of Industrial Engineering-Dalhousie University (Canada). He is member of IFAC TC 5.2 where he is chair of a working group on Smart, Reliable and Sustainable Manufacturing-Distribution Systems. He also served as a scientific expert member of the Natural Sciences and Engineering Research Council of Canada (NSERC). Dr. Khatab research interests include reliability theory, optimisation and decision support for system's production and intelligent maintenance management, design and optimisation of reverse supply chains, sustainability, and remanufacturing.
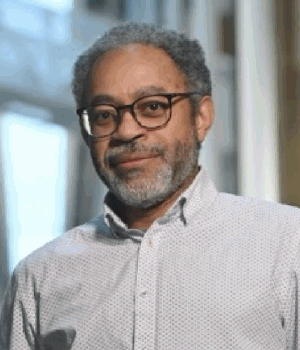
C. Diallo
Claver Diallo Ph.D., P.Eng., is Professor in the Department of Industrial Engineering at Dalhousie University in Halifax, Nova Scotia. He has taught at Dalhousie University since October 2007. He holds a Ph.D. and a Master of Applied Science degree in Industrial Engineering, and a bachelor's degree in mechanical engineering from Laval University, Quebec, Canada. He is a member of the Institute of Industrial and Systems Engineering (IISE), the Canadian Operational Research Society (CORS) and Engineers Nova Scotia (ENS). He is a member of IFAC TC 5.2. His current research is focussed on reliability engineering & predictive maintenance, production and distribution systems design within the Industry 4.0/5.0 context which includes hyperconnected logistics networks, smart production planning and control, and sustainable supply chain management.