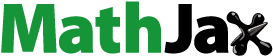
Abstract
Accurate range prediction requires good knowledge of the prevailing wind conditions and how they affect the energy consumption of the ego vehicle. A few different simplified vehicle air drag models that explicitly include the effect from crosswinds are presented and compared through some objective criteria. The models are developed from the normal air drag equation where the effect from wind is implicit and therefore often forgotten or neglected. The purpose is to find a low-complexity model complementing CFD models and wind tunnel tests, that can be used for range estimation and predictive energy management algorithms. To simplify online estimation, a requirement is that the air drag models only contain a few tuning parameters. The models are validated against CFD calculations for a few vehicle combinations and the best models show good accuracy for air attack angles up to at least 60 degrees. It is shown that the parameters of the simplified models can loosely be connected to some basic geometrical attributes of a vehicle combination so it should be possible to give at least a rough estimate of the parameters of a simplified model based on these geometrical attributes. This is useful for making a first estimate of the aerodynamic properties of a vehicle combination after major changes in the exterior, e.g. when adding a trailer. It also highlights that the size and the shape of the vehicle side may be mainly responsible for the high longitudinal air drag sensitivity to crosswinds for large vehicle combinations.
1. Introduction
Range anxiety is regarded as one of the real obstructions to a broad public acceptance of battery electric road vehicles [Citation1]. For many commercial transport missions, there are also requirements for delivery on time. The combination of limited range and time requirements is especially bothersome since an attempt to increase the vehicle speed in order to increase the probability of fulfilling the time requirement may decrease the range and the probability of reaching the end destination or a charging spot and vice versa. Range prediction is about estimating available energy stored onboard the vehicle and predicting future vehicle energy consumption. There is a vast amount of research around range estimation (e.g. [Citation2–4]).
It is well known that the environment is impacting vehicle energy consumption a lot. Road surface type (e.g. asphalt, concrete, dirt), roughness and conditions (e.g. dry, humid, wet) interact with the ambient conditions (e.g. temperature, precipitation) and the tires to form rolling resistance, [Citation5,Citation6] and weather factors like ambient temperature, wind, and ambient pressure have an effect on air drag [Citation7]. Crosswinds may also cause energy losses from lateral forces in the form of cornering resistance [Citation8]. Furthermore, [Citation9] discusses the aerodynamic properties of wings and how they can be used to harvest wind energy. It relates the power generation to the total aerodynamic force of the wing, the wind speed, and the angle between the aerodynamic force and the wind and it mentions that wind can generate up to 2 MW from a single kite system. With that said, wind is a powerful force, and it needs to be taken into account when doing vehicle range estimation.
It is well established that the longitudinal (axial) air drag is proportional to the square of the airspeed, implying that a 10% increase in airspeed is actually increasing the air drag by 21%. The large body of a heavy-duty vehicle combination makes it sensitive not only to wind in longitudinal direction (headwind/tailwind) but also to wind in lateral direction (crosswind). This is also true for all types of vehicles from passenger cars to trains, [Citation10,Citation11], airplanes, and ships, [Citation12], as well as offshore rigs, [Citation13,Citation14], but to different extent.
Computational Fluid Dynamics (CFD) calculations and wind tunnel tests, see e.g. [Citation15–18], can be used to show how huge the effect is from headwind and crosswind. Figure is illustrating the relative air drag of a tractor and semitrailer combination in different wind conditions using CFD simulations. The simulations show the influence of wind speeds relative to the case of no wind when driving at 85 km/h (23.6 m/s). As can be seen in Figure (a), the crosswind is at least as important as the headwind. Applying this knowledge to a range estimation application, the relative effect from crosswinds turns out to be very important. For a truck going back and forth on the same road, assuming that the wind is in the same direction as the road, a large proportion of the increase in air drag induced energy consumption that comes from the headwind effect in one direction is canceled out by the tailwind in the other direction. However, this is not true for the crosswind component. It is more or less equally bad in both directions, and the combination of both headwind and crosswind is by far the worst.
Figure 1. CFD simulations, [Citation19], of air drag dependency on headwind and crosswind for a truck with semitrailer combination. The CFD tool used is called Simcenter STAR-CCM+, [Citation19] and the calculations are done using a method called RANS (Reynolds Averaged Navier Stokes) with a standard model for the turbulent flow [Citation20]. (a) Relative air drag as function of headwind and crosswind speed and (b) Relative air drag as function of wind speed. The wind comes in at
from the front (positive velocity) or back (negative velocity).
![Figure 1. CFD simulations, [Citation19], of air drag dependency on headwind and crosswind for a truck with semitrailer combination. The CFD tool used is called Simcenter STAR-CCM+, [Citation19] and the calculations are done using a method called RANS (Reynolds Averaged Navier Stokes) with a standard k−ϵ model for the turbulent flow [Citation20]. (a) Relative air drag as function of headwind and crosswind speed and (b) Relative air drag as function of wind speed. The wind comes in at 45∘ from the front (positive velocity) or back (negative velocity).](/cms/asset/45808906-6ba8-4bc6-a049-b4d78b3f41e0/nvsd_a_2213786_f0001_oc.jpg)
Figure (b) shows how the air drag is changing with wind speed if the wind comes in at from the front (positive velocity) or back (negative velocity). When driving at 85 km/h (23.6 m/s) a wind speed of 6 m/s almost doubles the air drag, while a wind speed of 15 m/s increases the air drag by a factor of four. Worth noting is that the air drag dependency from the wind coming from 45 degrees tail/side (negative wind speed in Figure (b)) is quite low. A conclusion is that while the combination of crosswinds and headwinds is affecting energy consumption a lot, the combination of crosswinds and tailwinds is not. In the latter case, the impaired aerodynamic properties due to crosswinds are canceled out by the reduction in longitudinal airspeed. Altogether, since the wind contributes to the propulsion energy loss, in some conditions a lot, it is beneficial from a vehicle range estimation perspective to have an air drag model that is capable of taking wind information into account.
The focus of this paper is on how the wind is affecting aerodynamic resistance, or air drag, as air drag contributes a large share of the total propulsion energy loss of a vehicle combination. Sarrafan et al. [Citation21] is pointing out that the air drag often is the largest energy loss of an electrified vehicle and that the effects of air drag from crosswinds cannot be neglected. The platooning effect is well known and [Citation22] has done a comprehensive literature study of the research in this field. Worth mentioning is that according to [Citation23,Citation24], platooning effect remains in crosswind conditions even though somewhat reduced. Air drag is also affected by the surrounding traffic. McAuliffe and Ahmadi-Baloutaki [Citation25] and McAuliffe et al. [Citation26] have investigated the influence of close-proximity traffic and calls the resulting reduction in air drag background platooning since the effect resembles the effect of running vehicles closely after each other, i.e. in a platoon. The effect from traffic is complex though and is not yet fully understood.
Even though CFD calculations and wind tunnel tests can give quite accurate results, due to the shadowing effect, i.e. the change in air drag on a component that is behind (in the shadow of) another component, that is pointed out by both [Citation14,Citation27], air drag of any combination of geometrical shapes is not the same as the sum of the air drag of the individual components alone. Hence, when the exterior of a vehicle changes, for example when adding a trailer, changing a trailer, changing the setting of an air deflector, or adding headlights, it is not enough to know the aerodynamic properties of the added or removed parts, the air drag of the whole combination after the change needs to be taken into account. The exterior of a heavy-duty vehicle can change often and many times and the option of redoing CFD calculations or wind tunnel tests every time this happens is expensive, time-consuming, and not always feasible. This gives a strong motivation for estimating the air drag properties online instead, as done in [Citation28,Citation29]. However, the effect on the air drag coefficient from crosswinds is discussed [Citation28] but it is not dealt with in the actual estimation in any of the mentioned works, probably because it is very difficult to estimate an air drag coefficient online for every possible air attack angle since this would require a massive amount of data to properly excite the system. This motivates the development of a simple air drag model which only requires determination of a few parameters.
Using a simplified air drag model including crosswind effects together with accurate predictions of the wind speed and wind direction at the road level has the potential to improve the accuracy of air drag energy consumption predictions substantially. This is essential not only for doing accurate vehicle range prediction but also for investigating how the vehicle speed in different road segments affects the overall energy consumption which is fundamental for advanced predictive energy-efficient cruise controllers and route planning functions. Moreover, a simplified model can be very useful for understanding how the crosswind sensitivity of a vehicle combination changes when the geometry of the combination changes, for example when adding or removing a trailer. This understanding could be used for improving range estimation models for vehicles that are used to transport different kinds of trailers as well as improve route and charge planning for vehicle combinations when information on crosswind speeds on the possible route options are available and also serve as an initial guess for the aerodynamic parameters using an on-line estimation algorithm. As seen in Figure , crosswind induced air drag energy consumption is something that cannot be neglected and is interacting with the longitudinal airspeed induced air drag.
The aim of this paper is to derive and evaluate different models that can include both head- and crosswinds. The models should be simple with only a few parameters and the intended use of the models is model-based control and estimator design, with a particular interest in range estimation or energy management. It is important to point out, that such simplified models by no means replace CFD models or wind tunnel tests. It is a complement to achieve a more accurate understanding of the air drag in different wind conditions when detailed CFD model information and wind tunnel test results are missing, for example after the change of trailer or loading of a timber truck.
The paper is organised in the following way. In the Modeling section it is shown how crosswinds are implicitly affecting the results from calculating air drag force using the standard equation. From this, a number of different simplified models including explicit effects from crosswinds are proposed. In the Results section, the performance of the proposed models for three different heavy-duty vehicle combinations is evaluated through a few objective criteria and using CFD calculations as a comparison. The results are followed by a discussion on what model to use, why and when, and plausible causes for the strong influence of crosswinds on air drag, and at the end, the paper is summarised with some concluding remarks.
2. Modeling
Air drag is a complex force counteracting the vehicle's motion. The state-of-the-art air drag model used in a vast amount of research papers related to aerodynamics is:
(1)
(1) where
is the air drag force acting on the vehicle in the longitudinal direction, ρ the air density,
the air drag coefficient,
the frontal projection area of the vehicle, and
the relative airspeed. In many papers, e.g. [Citation30–32], this equation is used as it is with constant values on air density, air drag coefficient, frontal area, and the relative airspeed equal to the vehicle speed.
Before extending the model to also include crosswinds we need to make some definitions related to wind and airspeed. The wind speed is divided into a headwind speed component, , acting opposed to the vehicle's motion direction and a crosswind component,
, perpendicular to the vehicle motion direction, see Figure .
The wind direction is determined as the angle between the headwind and the crosswind components:
(2)
(2) The relative longitudinal airspeed,
, then becomes the sum of
and the relative air speed due to the vehicle speed,
, i.e.:
(3)
(3) and the relative lateral airspeed becomes the crosswind component,
. The total relative airspeed
can be determined from the longitudinal and lateral air speed components as:
(4)
(4) and the angle between them is commonly denoted as the so-called air attack angle:
(5)
(5) CFD calculation and wind tunnel tests can be used to get detailed information on the air drag of a specific vehicle for different values of θ. Figure (a) shows the heavy-duty vehicle combinations used as examples throughout this paper, i.e. a rigid truck, a tractor with a semitrailer, and a timber truck with a trailer. Figure (b) shows how
, i.e. the shape factor lumped together with the projection area, relative to
for the tractor-semitrailer combination at θ = 0 changes with θ and the corresponding area projections, while as Table gives some geometrical data for the three vehicle combinations. The
values are based on CFD calculations, [Citation19,Citation20], of the air drags with respect to θ. From Figure (b) it can easily be seen that both the air drag coefficient and the projection area vary with θ, confirming that to consider the effect of crosswinds, the independent variable of the air attack angle needs to be introduced in the aerodynamic drag function.
As mentioned earlier, the idea of the paper is to derive models which can capture crosswinds in a better way compared to the state-of-the-art model, Equation (Equation1(1)
(1) ). The model must therefore be dependent on θ, so the model structure will be modified to:
(6)
(6) where
is the air drag coefficient as a function of θ and lumped together with the projection area.Footnote1
Another thing to clarify with Equation (Equation6(6)
(6) ) is that it only gives the air drag, i.e. the aerodynamic force component in the longitudinal direction of the vehicle. If being interested in the total force or perhaps any of the other force components, the lateral component (the side force) and the z-component (the lift force) can be computed by replacing
in Equation (Equation6
(6)
(6) ) with
, the aerodynamic side force coefficient and
, the lift coefficient, respectively.
Figure 3. Examples of heavy-duty vehicle combinations and how the air drag is changing with θ. The data comes from CFD calculations for the corresponding vehicle combinations. (a) Heavy duty combinations used as examples and (b) Relative and projection areas of example vehicles.
is normalised with respect to
in pure headwind, i.e. θ = 0 for tractor with semitrailer.

Table 1. Geometrical vehicle data.
The function in Equation (Equation6
(6)
(6) ), is the function to be modeled and the method used is a data-driven approach with CFD data to represent the true physical system. It should be noted that the function
includes the dependency on θ for both the air drag coefficient,
, and the projection area,
, lumped together. Different model structures are proposed using physical insights, and the model parameters are determined using regression.
2.1. Model structures
Vehicle air drag consists of two different parts, pressure drag and friction drag. Pressure drag is the force acting perpendicular to the vehicle's surface, as air impact the area of the vehicle it builds up an air pressure and consequently a force acting on the surface area. Furthermore, as air slides across the surface of the vehicle, this sliding motion generates friction forces that act parallel to the vehicle's surface. This is denoted as friction drag. Without the influence of crosswinds, most vehicles can be approximated as symmetric in the relative air direction which means that pressure drag is mainly dependent on the frontal area size while friction drag is mainly dependent on the size of the side area. However, when adding the influence from crosswinds, it gets more complex since the vehicle will no longer be symmetric in air direction and due to this asymmetry, pressure drag may also arise on the side area and friction drag on the frontal area. The intention of this section is to reason around how the pressure drag, friction drag and total air drag for a commercial heavy-duty vehicle combination is changing with air attack angle. The reasoning is then formalised into some simplified model structures including crosswind effects.
Force is the product of pressure and area. Neglecting friction drag, and separating the air drag coefficient in Equation (Equation6
(6)
(6) ) from the area projection
, suggests that the pressure build-up on the vehicle's surface is proportional to the square of the airspeed,
. When crosswinds are added, the air attack angle starts to grow and so does the area projection in air direction and pressure will be built up on the side area as well. Since the vehicle body will no longer be symmetric with respect to the air attack angle the resulting aerodynamic force will no longer act only in the air direction but will also have a perpendicular component. Assuming that the average pressure delta in the vehicle motion direction is proportional to the square of the longitudinal air speed component,
gives
(7)
(7) where
is a constant to be determined from measurements or CFD calculations. Equation (Equation7
(7)
(7) ) is denoted Model 1. If the area projection is not known but measures of the frontal and side areas can be obtained, it can be estimated by approximating the geometry of the truck as a cuboid according to
(8)
(8) where
is the frontal area and
is the side area. Figure shows the accuracy of the cuboid approximation of the three vehicle combinations. A plausible reason for the relatively large deviation between the true area projection of the rigid timber truck with trailer and the cuboid formula is the large air gaps between the different sections of this vehicle combination. For small values of θ, these spaces will not be visible and
will be closer to the area of a cuboid where these air gaps are part of the side area. Hence,
grows faster than the cuboid approximation based on the side area. When θ becomes large, the air gaps start to be visible, and the deviation between the true projection area and the cuboid model area shrinks.
It is likely that crosswinds also increase pressurisation of less aerodynamic shaped details like wheelhouses, gaps between tractor and trailer, and so on, and therefore increase the pressure drag. Friction drag may also be affected by crosswinds since they are 'pushing' the airflow along the windward side of the vehicle combination towards the side area. This can motivate a model that is somewhat more affected by crosswinds. A simple assumption would be that the air drag is increasing linearly with the product of the longitudinal and lateral air speeds, i.e. , which gives
(9)
(9) where
and
are constants. This model is denoted Model 2. An alternative model is formed by assuming that the air drag simply increases linearly with the product of the side area and θ. This gives
(10)
(10) where
and
are constants. This model is denoted Model 3. It may also be complemented with a term dealing with asymmetries of the vehicle combination's side area projection. Due to this asymmetry, both pressure drag and friction drag may arise from direct crosswinds. Assuming that the sum of these drag components is proportional to the product of the side area,
and the square of the crosswind speed,
this component can be added to Model 3 to form
(11)
(11) where
,
and
are constants. This model is denoted Model 4.
A direct crosswind component can also be added to Model 2. Since the model now have separate terms for effects from longitudinal airspeed, lateral airspeed and interaction between them, the dependency of the area projection, may be split and spread out so that there is a frontal area dependency on longitudinal airspeed, a side area dependency on lateral airspeed and a dependency on the square root of the product of frontal area and side area on the interaction term. This can be summarised as
(12)
(12) where
,
and
are constants. This model is denoted Model 5 and has some nice intuitive properties. By expanding
into the vehicle speed
and the wind speed components
and
respectively, the model can be rewritten as:
(13)
(13) It divides the air drag into three components. The first component is only affected by the longitudinal airspeed, the second, the interaction effect from both lateral and longitudinal airspeed, and the last component is only affected by the lateral airspeed. An interpretation of the first component is the nominal air resistance. This is exactly the air resistance that is normally stated which assumes that there is no crosswind.
The second component can be interpreted as the crosswind sensitivity air resistance. It relates to how sensitive the combination is to airspeed in driving direction and crosswind. The investigated vehicle combinations with large side areas have higher crosswind sensitivity than vehicle combinations with smaller side areas, but this factor is also likely to be affected by how irregular the surface of the side area is. For example, the Rigid timber truck with a trailer only has a slightly larger side area than the Tractor with a semitrailer combination but still, the crosswind sensitivity air resistance is much higher. This is likely to be partly due to the irregular side surface of the timber and partly due to larger gaps between different units of the combination.
Finally, the third component is the vehicle's direct crosswind air resistance. This term holds a direct effect on the road resistance from crosswinds. For the investigated truck combinations, the direct crosswind air resistance is negative and quite small in magnitude suggesting that it often can be neglected and only have a significant effect if driving slowly in hard crosswinds.
A common crosswind model found in the literature is the model proposed by Baker [Citation33] and later used by Walczak [Citation34]. In their work they present a model of on the form:
(14)
(14) where
and
are constants to be tuned for good model fit. To benchmark, the proposed models, Model 1–Model 5, the model represented by Equation (Equation14
(14)
(14) ) is introduced as Model 6. All models are summarised in Table .
Table 2. Model overview.
2.2. Parameter estimation
Given the model structures, presented in Section 2.1, and CFD data, model parameters can be fitted to match the CFD data as well as possible. The fit is measured by its residual, e, i.e. the difference between the CFD data and the model output:
(15)
(15) where
is the i:th CFD data point and
is the model output, given θ and the model parameters
. The model parameters are found by minimising a weighted sum of squared errors, E:
(16)
(16) where N is the number of data points, and
is the weighting factor.
As all model structures proposed are linear in the parameters, there exists an analytical solution to the least-squares problem, making it also tractable for use in real vehicle applications.
3. Results
This section presents the results from the parameter estimation of the models. To be able to objectively compare the six different models presented in this paper, a few criteria are used:
RMS–Root Mean Squared Error of CFD data and model output:
i.e.
for all data points.
Weighted RMS
–Weighted Root Mean Squared Error of CFD data and model output:
The scaling factors allows for fitting the data points better to a certain range of θ. The scaling factors used are:
The reason for having scaling factors that are declining with θ is that the total vehicle energy consumption when θ is large in real-world driving cases is assumed to be relatively small. Kawamata et al. [Citation16] states that up to 50% of the total energy consumption of cars due to air drag in the U.S. occurs when θ is between
and
. Even though θ is likely to be somewhat larger for trucks due to lower average speed, it is safe to say that for most applications, the majority of the air drag energy consumption occurs when
and that the total vehicle energy consumption from air drag when
is very low compared to air drag consumption when θ is smaller.
Model range (MR
)–the maximum allowed deviation, T, between model output and CFD output. The threshold value T represents the maximum allowed absolute difference between model output and CFD output. The valid model range is determined in increasing θ and defines the largest θ for which it is possible to find model parameters that keep the maximum absolute model error smaller than the threshold T.
NTP–The number of tuning parameters of the model.
NGP–The number of geometrical parameters of the simplified model, i.e. parameters that can be directly connected to geometrical attributes like frontal area and side area.
Figure shows the results of the values given from CFD calculations for the three example vehicle combinations. The CFD tool used is called Simcenter STAR-CCM+, [Citation19] and the calculations are done using a method called RANS (Reynolds Averaged Navier Stokes) with a standard
model for the turbulent flow [Citation20]. As can be seen in the figure, all models are performing well. Note that the
values of the timber truck have been downscaled by a factor of two in order to give it a similar magnitude as the other vehicle combinations and that the plotted
are all values relative
for the tractor with semitrailer combination.
Figure 5. approximations as function of θ for different types of vehicle combinations. (a) Model 1. (b) Model 2. (c) Model 3. (d) Model 4. (e) Model 5 and (f) Model 6.

Table summarises the different objective criteria for each of the simplified models presented. To the results, also a comparison with the state-of-the-art model, Equation (Equation1(1)
(1) ), is appended, as Model 0.
Table 3. Model performance comparison.
An observation is that while Model 1 gives fairly accurate results for a wide range of θ, it also gives relatively large errors for small values of θ. A conclusion of this is that the sensitivity to longitudinal airspeed is not the same thing as the sensitivity to crosswinds.
To connect model accuracy to a range estimation application, an error in ,
, scales to air drag energy consumption prediction error per distance,
, for a vehicle running at constant speed
, in no wind according to:
(17)
(17) For a vehicle driving at 85 km/h (23.6 m/s), in no wind condition and
, this mean that a model error of
corresponds to a
J/m, or equally, 0.09 kWh/km. In a full day of driving, e.g. 600 km, this error grows to more than 50 kWh.
4. Discussion
A model with a good fit to data is useful since it is capable of accurately quantifying the air drag. The wider the model range, the more different wind and vehicle speed conditions can be handled, and if the model contains few tuning parameters, it is likely to be more suitable for online estimation. If the model parameters can be roughly estimated from geometrical attributes, the change in aerodynamic properties can be predicted for changes in the vehicle exterior which is useful for, for example, when doing the route and charge planning for a mission that includes exterior change like a pic-up of a trailer. However, using geometrical attributes as model parameters may also introduce uncertainties and errors in the model unless detailed information on the geometrical attributes exists. Hence, few geometrical parameters may also be considered positive. The models are compared through objective measures but will also be discussed separately in more subjective terms.
The results summarised in Table show that it is difficult to find a model that is best in all aspects. First of all, the trend is that the model fit and valid model range are improving with the number of tuning parameters. This is consistent with estimation theory in general, a larger number of tuning parameters makes it possible to tailor the details of the model more carefully, i.e. a higher degree of model fit can be achieved. Having too many parameters can cause problems with overfitting, i.e. fitting the model to noise, measurement/CFD modeling errors, and other disturbances that were not intended to be modeled, and it makes it more difficult to estimate the parameters online.
Which of the simplified models to use depends on the application and on what information is available. Model accuracy is for sure of interest for a range estimation algorithm. The calculation of energy consumption prediction error, , in the results chapter reveals that this error, and hence the range estimation error, is linear in model error size,
. It is very difficult to put a real value on what is a sufficiently precise prediction since it depends on the situation. However, in some situations, e.g. when the range is very close to the actual remaining driving distance, accuracy is of high importance. This would suggest that Model 4 would be the best choice. However, note that the stated error measures in Table show the model error for the best possible choice of model parameters. There is no point in choosing a three-parameter model unless the model parameters can be found with good accuracy. The choice of model to use should instead be governed by the model error including model parameter errors which are likely to increase with the number of tuning parameters. Our recommendation is to use Model 4 or Model 5 for well-known applications where CFD or wind tunnel test data exists. Model 1 could be used where no such data exists and online estimation cannot be done while Model 2 or Model 3 may be used when online estimation can be used. Model 0 should only be used when either wind information is missing or when
for
is the only information we have on the air drag properties of our vehicle and online estimation cannot be performed.
A learning from this investigation is that may be seen as mainly dependent on two factors, the size of the object which can be related to the area projection,
, and the shape of the object. Using this knowledge, it is possible to get an idea of how an exterior change affects
. If the change is just a scaling of either the complete vehicle combination or only the side area, the effect on
should be reflected in the change in
, i.e. it should be possible to do a fairly good prediction of how
will be after the change only with knowledge of how
is changed. Changes that are either targeting improved crosswind sensitivity [Citation17] or targeting improved longitudinal airspeed sensitivity like changing the setting of a roof air deflector [Citation18], often leave
more or less unchanged. For these types of changes, the change in
will instead appear in the shape factor
. Exact instructions on how to set the shape factor after such a change is left for future research. However, some hints can be found in previous work by others for determining the effect of the change of gap size between tractor and trailer [Citation27], and for determining the effect of rounding edges on the tractor and air deflector setting [Citation18]. In general, the approach of using research data as a base for setting shape parameters is recommended to be used only as a starting point when no better information is available. If using simplified models, like the ones presented in this paper, combined with more exact information of the projection area depending on air attack angle, online estimation can be used to improve the accuracy of the shape parameters. Combined with precise predictions of road wind conditions accurate determination of air drag losses depending on vehicle speed profile over any road segment could be enabled which is an important part of an accurate range prediction algorithm.
The simplified models in this paper are developed to provide a mean to include crosswind in air drag calculations. It will by no means replace CFD models and wind tunnel tests which will provide a deeper understanding of what really creates air drag and other aerodynamic forces. As an example, [Citation17], show through CFD simulations not only how the dimensions of a trailer boat tail affect the air drag forces in crosswinds but also why. Vortexes created at the rear of the vehicle and in the gap between the tractor and the trailer change with crosswinds and boat tail dimensions. These kinds of things are certainly not something that could be studied through simplified models. With that said, there is room for models and tests of all levels of detail. What is the best choice is often driven by the application, i.e. the planned usage of the result and the available information.
5. Conclusion
The combination of limited range, limited charging opportunities, and long charging times of battery electric vehicles demands accurate range prediction. The effect from both headwind and crosswind has a huge influence on the air drag of heavy-duty vehicle combinations and can therefore not be neglected. Since the exterior and hence the aerodynamic properties of a large vehicle combination can change drastically with little possibility of retrieving good information about the air drag after the change, online estimation may be the only viable option. To support this process, simplified models of including the effects of crosswinds were developed and compared through objective measures.
A key parameter for deciding on what model to use is how many model parameters it should have. If only one tuning parameter is used, a model consisting of a constant multiplied with the area projection in air attack angle performs surprisingly well. This area projection gives a measure of the air mass that is affected by the vehicle and seems to be more or less proportional to this measure. However, in order to have a range estimation algorithm capable of delivering accurate results in low vehicle speeds and moderate to high crosswind conditions, model accuracy in a wider range of air attack angle is of importance. This demands a model with more degrees of freedom that separates headwind sensitivity from crosswind sensitivity. Either a linear interaction effect from a lateral wind component on the longitudinal air component can be added or simply use a model of
that is linear in θ. These two alternatives can be complemented with a direct effect on
from the lateral air component. In the former case this results in a three parameters model that is quadratic in the longitudinal airspeed component, linear in the product of the longitudinal air and lateral air components, and quadratic in the lateral air component. In conclusion, a model with three parameters seems to be a good compromise between model simplicity, size of the valid model range, and model accuracy for range estimation applications taking wind conditions into account.
Disclosure statement
No potential conflict of interest was reported by the author(s).
Additional information
Funding
Notes
1 It should be noted that air density is far from constant. It has a strong dependency on ambient temperature, a moderate dependency on air pressure, and a minor dependency on air humidity. It is hence important to clarify under what weather conditions the air drag will be calculated, as discussed in [Citation35].
References
- Dong J, Wu X, Liu C, et al. The impact of reliable range estimation on battery electric vehicle feasibility. Int J Sustain Transp. 2020;14(11):833–842.
- Hong J, Park S, Chang N. Accurate remaining range estimation for electric vehicles. Macao, China. Proceedings of 21st Asia and South Pacific Design Automation Conference (ASP-DAC) 2016. p. 781–786.
- Oliva JA, Weihrauch C, Bertram T. A model-based approach for predicting the remaining driving range in electric vehicles. New Orleans, LA, US. Annual Conference of the PHM Society. Vol. 5, no 1. 2013.
- Grubwinkler S, Brunner T, Lienkamp M. Range prediction for EVs via crowd-sourcing. Coimbra, Portugal. IEEE Vehicle Power and Propulsion Conference (VPPC). 2014. p. 1–6.
- Srirangam S, Anupam K, Kasbergen C, et al. Study of influence of operating parameters on braking friction and rolling resistance. J Transp Res Board. 2015;2525(1):79–90.
- Carlson A, Vieira T. The effect of water and snow on the road surface on rolling resistance. Statens väg-och transportforskningsinstitut (VTI) report 971A. Available from: https://www.vti.se/en/publications; 2021.
- Sovran G. The effect of ambient wind on a road vehicle's aerodynamic work requirement and fuel consumption. SAE Transactions. 1984;93(Section 2):840222–840402. p. 449-472.
- Beckers CJJ, Besselink IJM, Nijmeijer H. Assessing the impact of cornering losses on the energy consumption of electric city buses. Transp Res Part D Transp Environ. 2020;86:Article ID 102360.
- Diehl M. Airborne wind energy: basic concepts and physical foundations. In: Airborne wind energy. Berlin, Heidelberg: Springer; 2013. p. 3–22.
- Quazi A, Crouch T, Bell J, et al. A field study on the aerodynamics of freight trains. J Wind Eng Ind Aerodyn. 2021; 209. 104463.
- Shao XM, Wan J, Chen DW, et al. Aerodynamic modeling and stability analysis of a high-speed train under strong rain and crosswind conditions. J Zhejiang Univ Sci A. 2011;12(12):964–970.
- Trivyza E, Boulougouris E. Aerodynamic study of superstructures of megayachts. Glasgow, UK. Proceedings of the 9th International Workshop on Ship and Marine Hydrodynamics. 2015.
- Popescu M, Simbotin A. 1998. Theoretic and experimental studies for determination of wind loads on offshore platform structures. Bucharest, Romania
- Liu G, Sun Y, Zhong B, et al. Analysis of wind load effect on key components in a jack-up offshore platform. Appl Ocean Res. 2020;101.102263.
- Guilmineau E, Chikhaoui O, Deng G, et al. Cross wind effects on a simplified car model by a DES approach. Comput Fluids. 2013;78:29–40.
- Kawamata H, Kuroda S, Tanaka S, et al. Improvement of practical electric consumption by drag reducing under cross wind. SAE Technical Paper, No. 2016-01-1626; 2016.
- Hassaan M, Badlani D, Nazarinia M. On the effect of boat-tails on a simplified heavy vehicle geometry under crosswinds. J Wind Eng Ind Aerodyn. 2018;183:172–186.
- Gilhaus A. The influence of cab shape on air drag of trucks. J Wind Eng Ind Aerodyn. 1981;9(1-2):77–87.
- Simcenter [Internet]. Siemens; [cited 2022 Oct 18]. Available from: https://www.plm.automation.siemens.com/global/en/products/simcenter/STAR-CCM.html.
- Giancarlo A. Reynolds-averaged Navier–Stokes equations for turbulence modeling. Appl Mech Rev. 2009;62(4).040802.
- Sarrafan K, Sutanto D, Muttaqi KM, et al. Accurate range estimation for an electric vehicle including changing environmental conditions and traction system efficiency. IET Electr Syst Transp. 2016;7(2):117–124.
- Zhang L, Chen F, Ma X, et al. Fuel economy in truck platooning: a literature overview and directions for future research. J Adv Transp. 2020. 2604012.
- Marcu B, Browand F. Aerodynamic forces experienced by a 3-vehicle platoon in a crosswind. SAE technical paper No. 1999-01-1324; 1999.
- Trnell J, Sebben S, Elofsson P. Experimental investigation of a two-truck platoon considering inter-vehicle distance, lateral offset and yaw. J Wind Eng Indust Aerodyn. 2021;213:Article ID 104596.
- McAuliffe B, Ahmadi-Baloutaki M. An investigation of the influence of close-proximity traffic on the aerodynamic drag experienced by tractor-trailer combinations. SAE Int J Adv Curr Pract Mobil. 2019;1(3):1251–1264.
- McAuliffe B, Raeesi A, Lammert M, et al. Impact of mixed traffic on the energy savings of a truck platoon: preprint. Golden (CO): National Renewable Energy Lab.(NREL); 2020.
- Allan JW. Aerodynamic drag and pressure measurements on a simplified tractor-trailer model. J Wind Eng Ind Aerodyn. 1981;9(1-2):125–136.
- Andersson R. Online estimation of rolling resistance and air drag for heavy duty vehicles [Master of Science Thesis]. Industrial Engineering and Management Machine Design, KTH, Sweden; 2012.
- El Gaouti Y, Colin G, Thiam B, et al. Online vehicle aerodynamic drag observer with Kalman filters. IFAC-PapersOnLine. 2021;54(2):51–56.
- Marzbanrad J, Tahbaz-zadeh Moghaddam I. Self-tuning control algorithm design for vehicle adaptive cruise control system through real-time estimation of vehicle parameters and road grade. Veh Syst Dyn. 2016;54(9):1291–1316.
- Fotouhi A, Shateri N, Shona Laila D, et al. Electric vehicle energy consumption estimation for a fleet management system. Int J Sustain Transp. 2020;15(1):40–54.
- Delgado OF, Clark NN, Thompson GJ. Heavy duty truck fuel consumption prediction based on driving cycle properties. Int J Sustain Transp. 2012;6(6):338–361.
- Baker CJ. A simplified analysis of various types of wind-induced road vehicle accidents. J Wind Eng Ind Aerodyn. 1986;22(1):69–85.
- Walczak S. Analysis of vehicle dynamics under sadden cross wind. Krakow, Poland, IOP Conference Series: Materials Science and Engineering..2016;148(1):012030.
- Jones FE. The air density equation and the transfer of the mass unit. J Res Natl Bur Stand. 1978;83(5):419.