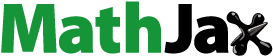
Abstract
The contribution of solar PV to the electricity generation in Kenya was approximately 3.6% as of June 2021. The challenges for low utilising were associated with limited information on the potential opportunities and economic benefits. The objective of this study was to conduct a techno-economic performance of PV systems in Kenya. The methodology involved a case analysis of four grid-tied PV systems installed at Strathmore University. The meteorological data were collected from the weather station, located within 300 metres from the power plant. The electrical parameters were captured by the SolarEdge monitoring system. The performance of the systems was assessed based on two scenarios. Scenario one was on the same fixed tilt angle but different orientation while second scenario consisted of same orientations but different tilt angle. The results indicated a performance ratio and levelized cost of energy (LCOE) of 68% and US$0.17 respectively. The model developed indicates that an increase in relative humidity decreases power output of PV system. The results provide clear evidence of the technical and economic viability of PV technology in tropical savanna climatic conditions and contributes to better understanding of the status and prospects for PV systems uptake in Kenya.
Abbreviations
A | = | Amperes |
AC | = | Alternating current |
CIS | = | Copper indium selenium |
CO | = | initial capital investment |
Ct | = | Discounted annual cash flows |
CUF | = | Capacity utilisation factor |
DC | = | Direct current |
Diff. | = | Difference |
FF | = | Fill factor |
FiT | = | Feed-in tariff |
IEC | = | International Electrotechnical commission |
It | = | Annual cash inflows |
kWh | = | Kilowatt-hour |
LCA | = | Life cycle assessment |
LCOE | = | Levelised cost of energy |
LID | = | Light-induced degradation |
M | = | measured value |
MAD | = | mean average deviation |
MAE | = | mean absolute error |
MAPE | = | mean average percentage error |
RMSE | = | root mean square error |
M-Si | = | monocrystalline |
MW | = | Megawatt |
MWh | = | Megawatt hour |
NPV | = | Net present value |
O&M | = | Operation and maintenance |
P | = | Predicted value |
P | = | Power |
PR | = | Performance ratio |
P-Si | = | Polycrystalline |
Pu | = | per unit cost of electrical energy in Kenya |
PV | = | Photovoltaic |
R | = | Discounted rate |
STC | = | Standard test conditions |
TLCC | = | Total life-cycle cost |
UMP | = | University of Malaysia, Pahang |
US | = | United State of America |
V | = | Volt |
W | = | Watt |
Subscripted Symbols
CO2 | = | Carbon dioxide |
GO | = | reference irradiance |
HT | = | In-plane radiation |
IMPP | = | Maximum current PowerPoint |
LT | = | total energy loss |
MWp | = | Megawatt power |
VMP | = | Maximum voltage point |
VOC | = | Open circuit voltage |
YF | = | Final yield |
YR | = | reference yield |
Greek Symbols
η | = | Efficiency |
1. Introduction
As part of activities toward attainment of sustainable peace and prosperity for people and the planet, and thereby improve the condition and standard of living of the global population, all United Nations Member states adopted 17 Sustainable Development Goals (SDGs) in 2015 termed ‘The 2030 Agenda for Sustainable Development’. At the heart of these 17 SDGs is Goal 7, which aims to ensure access to affordable, reliable, sustainable, and modern energy for all by 2030. Attainment of this goal could have a direct impact on the achievement of some other SDGs, such as SDG 3 (Good health and well-being), SDG 8 (Economic growth), SDG 11 (Sustainable cities and communities), and SDG 13 (Climate Action). Due to their being environmentally friendly, the development of renewable energy sources, which can be defined as energy resources that are ever available in a continuous supply, could play a significant role in the achievement of SDG 7. Furthermore, being ‘local’ energy resources, the development of renewable energy-based energy systems could play a crucial role in improving national and regional energy security.
One of the renewable energy technologies that have attracted more interest globally is solar photovoltaic (PV) technology. Solar PV technology can be used to generate electricity directly without additional mechanical and generating components. Furthermore, solar PV technologies are scalable and allow users to install various size solar PV modules according to their needs and financial ability. Solar PV technologies have other advantages compared to other sources of renewable energy, such as short implementation period, availability, less visual and noise impacts if installed on building roofs, it reduces energy-food conflict issues, and transmission and distribution losses.
Despite these advantages and the availability of solar energy resources, the uptake of solar PV as the source of electrical energy in Africa is still low compared to other sources such as hydropower and thermal. According to (IEA Citation2020), in the year 2020 only of the electrical energy produced in Africa was from solar PV systems. One of the major challenges of low uptake of solar PV in Kenya as a source of electrical energy is attributed to a lack of information on solar technology potential to generate electricity, potential opportunities (e.g. energy consumption savings), its performance under local climatic conditions, and the economic benefits (National Energy Policy, Kenya Citation2018). The other issue for low utilisation of solar PV energy in Kenya is associated with a lack of sufficient data which can inform the economic viability and sustainability of different PV technologies (National Energy Policy, Kenya Citation2018). Therefore, a comprehensive study of solar PV performance under the Kenyan climate conditions is essential to avail necessary data on performance and economic benefits. Two approaches, which are computer simulations and real-system tests, were used to study the performance of solar PV installations.
1.1. Literature review
This section is divided into four sections namely; Comparison based on measured and simulated data on performance of PV modules, effect of weather and environmental conditions on performances of PV modules, tilt and orientation angles on performances of PV modules, and technical and economics performances PV module system.
1.2. Comparison of solar PV performance based on measured and simulated data
Studies been conducted around the globe on PV modules based on measured verses simulated data such us (Goel and Sharma Citation2021; KhareSaxena, Saxena, and Sudhakar Citation2021; Shiva and Sudhakar Citation2015; Thotakura et al. Citation2020; Vidal et al. Citation2020). The performance of solar PV systems using both measured and simulated data yield different results as discussed in the following literature. Thotakura et al. (Citation2020) reported that, when compared with real-life performance, the software models underestimated the energy injected into the grid as follows: 5.33%, Photovoltaic Geographical Information System (PVGIS), 12.33%, PVWatts Calculator (PVwatts), and 30.64%, Photovoltaic System (PVSyst) in tropical wet and dry climates of India. This could be owed to the sources of the input data and the different methodologies of estimating the weather parameters for the different simulation tools. In a separate study, (Goel and Sharma Citation2021) using PVSyst, and Helioscope software found that annual yield to be 9.2% and 14.75%, respectively higher than the measured value. We conjecture that this is due to different sources of satellite data, (Meteonoum and ISHRAE).
Banda, Nyeinga, and Okello (Citation2019) reported a marginal difference between the measured and simulated performance ratio (PR) of 79.5% and 80% respectively for a grid-connected solar PV power plant in Malawi. In another study, (Ramanan, Kalidasa, and Karthick Citation2019) indicated uncertainty of measured and predicted values of 0.32% and 0.47% for polycrystalline(p-Si) and Copper indium selenium (CIS) systems, respectively. Vidal et al. (Citation2020) indicated a simulated PR value of 85.5% and measured the PR value as 89% with a discrepancy of 4%.
From the foregoing literature, it is clearly evident that simulation results do not always mimic the actual (measured) results. It can either overestimate or underestimate the actual performance. This can lead to wrong decisions on the system design, investment, and benefits associated with the utilisation of the technology. However, the use of the actual data provides a good estimate and helps in the development of reliable models for actual performance, reliability, and economic benefit of the system of the climatic condition under consideration of the region.
1.3. Effect of weather and environmental conditions on solar PV performance
Many studies have investigated the effect of direct environmental variables on solar PV performance and developed models for predicting system performance. Sahouane et al. (Citation2019) reported that the environmental parameter variation has a direct effect on the performance of both the energy conversion efficiency and the system losses. Al-Badi (Citation2020) showed that high solar radiation and ambient temperature combined with low wind speed increase the module temperature and as a result increase the cell temperature loss. Similarly, (Arora, Arora, and Sridhara Citation2022) found that with the rise in ambient temperature the energy generated by solar PV modules decreases. The study also indicated that environmental conditions are the key factors for overall system performance.
Several studies in the open literature have developed models to estimate the performance of solar PV modules in relation to environmental parameters as summarised in Table . The table shows models which have developed using one or two weather parameters to predict an electrical parameter as an output. The table further shows relationships obtained by the model developed. The use of one or two parameters may not indicate good accuracy and validity of the predicted results. Weather parameters can play a significant role in predicting the performance of a solar PV module in a specific region.
Table 1. Model developed from environmental parameters to predict solar PV performance.
Furthermore, the selection of the combination of environmental parameters greatly determines the accuracy of the model results. Ziane et al. (Citation2021) indicated that preprocessing techniques can be a useful tool to identify crucial and important parameters, in order to construct a lightweight prediction model that depends on a lesser number of variables as possible. This saves time of computations and avoid overfitting the model. AlSkaif et al. (Citation2019) using principal component analysis (PCA) showed that relative humidity, visibility, temperature and cloud cover had the highest importance when selecting the PV system output power. Saad et al. (Citation2022) used plane of array irradiance, precipitations, pressure and temperature as the environmental variables on the study of the impact of forecasting horizon and resolution on PV Power Prediction using Artificial Neural Networks. Most of these studies selected certain variables based on their availability.
Some studies such us (Saad et al. Citation2022; Salimi et al. Citation2020; Ziane et al. Citation2021) have previously developed mathematical models on PV modules. However, these studies used one or two weather parameters as input variable and power output as an output variable. These models indicated low value of coefficient of correlation which may lead to significant difference between actual and predicted values. In addition, Gopi et al. (Citation2021) developed models using more than one weather parameters which yields less difference between predicted and actual values. However, those models are complex to use and time consuming (Ziane et al. Citation2021), due to several input parameters, and sometimes unavailability of data. Furthermore, most of the studies from open literature such (Bhattacharya, Chakraborty, and Pal Citation2014; Mensah, Yamoah, and Adaramola Citation2019; Salimi et al. Citation2020), have indicated the effects of solar irradiance, ambient temperature, and wind speed, however, not much has been done on the effects of relative humidity on the performance of PV modules (Gopi et al. Citation2021).
Finally, studies such us (Ayadi et al. Citation2019; Gopi et al. Citation2021) used one-year data to develop models. Those models may underestimate or overestimate the performances of PV modules due to trends and degradation factors. A good model can be a useful tool during design and determination of economic analysis viability of PV module. The novelty of this work is, therefore, to develop accurate analytical model that is relatively less complex and easy to use, using five years data. The developed model combined effects of solar irradiance, ambient temperature, wind speed, and relative humidity on the performance of PV modules. This study used preprocessing technique by developing models comparing different combination of weather parameters such us (power output and solar irradiance, power output verses wind speed and irradiance, power output verses relative humidity etc.), to access the predicting capability of the model by using coefficient of correlation and Mean Percentage Error (MAPE) statistical indicators.
1.4. Tilt and orientation angles on solar PV performance
The performance of the solar PV module is affected by weather and meteorological conditions, efficiencies of the main components of the system, and their responses to environmental conditions as well as installation conditions (tilt angle and orientations) (Oloya, Gutu, and Adaramola Citation2021). Due to the availability of data, this study considers two scenarios, which are (1) systems with the same fixed tilt angle but different orientations and (2) systems with the same orientation but different tilt angles. All the systems considered in this study were set up in the same location.
Seme et al. (Citation2019) indicated that the performance of the photovoltaic system primarily depends on the proper inclination, azimuth angle shading, and snow barriers. The study also indicated that PV systems installed in higher latitudes have lower final yields. Omar and Mahmoud (Citation2018) indicated that fixed PV arrays should be tilted at the latitude of the location to capture the most solar energy. The study also indicated the optimum orientation of a PV module as the geographic true south. The study further used PVSYST software to compute the output power of a 1 kW peak PV system with different tilt angles. The results indicated that the system generated more energy with a tilt angle less than latitude and less energy with a tilt angle more than the latitude angle of the place.
1.5. Technical and economics performances of solar PV module system
Environmental conditions are location specific and different geographical regions have indicated different solar PV module performances due to different weather parameters. Technical analysis plays a critical role in presenting the relevant data for decision-making for further improvement in design, installation, and commissioning, leading to better performance (Chawla and Tikkiwal Citation2021; Martín-Martínez et al. Citation2019). Table shows the results of the studies which have been conducted in Asia, Middle East, America, Europe, West Africa, and South Africa. These studies are regionally unbalanced while the PV module performance is location-specific Despite the usefulness of performance analysis of solar PV installations for stakeholders, to our knowledge, much has not been done (open literature) as far as the study of solar PV module performance analysis in the East Africa region. This study, therefore, addresses the gap on the missing information on PR studies of the climatic conditions of East Africa region. The technical performance indicators which have been considered in the literature include reference yield (YR), final yield (YF), capacity utilisation factor (CUF), efficiency (η), and performance ratio (PR).
Table 2. Summary of selected previous studies on solar PV modules performances.
Studies been conducted around the globe on PV modules technical and economic performance analysis using one year data such as (Alshare et al. Citation2020; Sreenath, Sudhakar, and Af Citation2021; Thotakura et al. Citation2020; Vidal et al. Citation2020). Martín-Martínez et al. (Citation2019) reported that underestimating the complexity of a dual tracking system in the design phase underestimates operation and maintenance (O&M) which translates to underestimating the LCOE of the plant. The study proposes further work on the longer time span in order to complete and extend performance analysis and confirm the trends observed. With more data, new mathematical models of PV could be developed to improve the accuracy of the PV power plants design. Chawla and Tikkiwal (Citation2021) indicated that the PV modules when arranged in variable-tilt will produce a higher yield annually. The study proposes an economic and environmental assessment of the proposed setup. Mensah, Yamoah, and Adaramola (Citation2019) indicated the LCOE, discounted payback period, and simple payback period of 2.5MW solar PV installed in Ghana as years, and
years, respectively.
In addition to the technical performance of solar PV installations, from an economic point of view and to assist in making informed-decision, it is essential to know the financial value of generated electricity by the solar PV installations. This information could be helpful in assessing the economic viability of the installation as well as to compare the cost of energy produced with global data and available local or national retail utility electricity prices or alternative sources of electricity. Hence, several studies have recommended that the economic performance of solar PV installations should be carried out along with technical assessment performance (Chawla and Tikkiwal Citation2021; Seme et al. Citation2019).
1.6. Goal and contribution of this study
From the reviewed literature it can be indicated that;
Studies on performance of PV modules are regional unbalanced while the performances are location specific. This study addresses this gap by establishing the performances tropical savanna of East Africa region which little or no information is available in open literature.
Models developed on power output of PV modules used one or two weather parameters which indicates low value of coefficients of correlation which may lead to significant difference between actual and predicted values. This study addresses this gap by developing models with three weather parameters which indicates high value of coefficients of correlation with insignificant difference between actual and predicted values.
Studies have been conducted using less or one-year data which may underestimate or overestimate the technical performance and cost of maintenance and operation and lead to wrong LCOE. This study utilises five-year data which addresses the actual cost of operation and maintenance of PV module power plant.
Studies have recommended that economic performances should be done together with technical performances.
Therefore, the goal of the study is to examine the technical and economic performance and develop the models of solar PV installations using long-term performance data, which can provide more data for better assessment of PV modules in tropical savanna of East Africa region, as well as investigate inter-annual variability in the performance of the installation. The calculated economic indicators of the installations using the location economic/financial parameters could provide useful information to policymakers and other interested organisations in making the right decisions in the formulation of policies, installation design, and improvement of the products.
2. Materials and methods
2.1. Installation descriptions and location
This study was conducted on four solar PV installations (each has an installed capacity of 20 kW), which are located at Strathmore University, Nairobi, Kenya (Latitude: , Longitude
and Attitude: 1691.8 m). This site of Strathmore University is classified under tropical savanna climate according to the Köppen climatic map. According to data available from Kenya Meteorological Department, Strathmore University receives an average ambient temperature, relative humidity, and rainfall of
and
.
The solar PV systems were installed in June 2014 and comprised 2,400 PV modules with each module having a rated peak power of 250 W, which amounted to 600 kW, and they are arranged into 30 systems. Each system consists of 80 modules, which are arranged into 2 parallel strings, and each string consists of 40 modules connected in series. Each system was connected to the grid through a SMA Sunny Box DC–AC inverter. Each system supplied power to the building it was installed, and the surplus was supplied to the grid. The PV modules were installed on selected building rooftops on buildings that have different orientations relative to the compass south and have different pitch angles, hence some of the arrays are installed at different tilt angles and orientations. Table shows the technical specifications of the modules installed while Table indicates the specifications of the inverter.
Table 3. Solar PV module specification.Table Footnotea
Table 4. Inverter specification.Table Footnotea
Table 5. Design parameters of the economic modeling.
For this study, 4 of the 30 arrays are selected and they are grouped into two (2) scenarios. The first scenario examined the effects of orientation on the performance of two systems’ that are inclined at a tilt angle of 180, first one oriented west to east (see Figure C (4)) and the second oriented south (see Figure A (1,2)). The second scenario examined the effects of different tilt angles on the performance of two installations with the same orientations (oriented toward south), one tilted at an angle of (see Figure A (1,2)) and the second tilted at an angle of
(see Figure B (3)). Figure indicates the schematic diagram of the solar PV power plant.
2.2. Measurement and data collection
The data was acquired through the data acquisition module of the inverter and collected by the SolarEdge monitoring system from June 2014 and is still ongoing. The data collected includes voltages, current, power, and energy of each System and averaged at an interval of 1 h where daily, weekly, monthly, and yearly collected parameters are derived. The results presented in this study consist of the data recorded between January 1, 2015, to December 31, 2019. The parameters of solar irradiance, ambient temperature, and wind speed were collected from the Kenya meteorological department weather station located 300 meters from the Strathmore University solar PV power plant.
2.3. Performance indicators
Conventionally, the performance monitoring of PV modules/systems in real operating conditions is studied as per guidelines of international standard IEC 61724 provided by the International Electrotechnical Commission, which is based on the technical indicators namely final yields, reference yields, capacity utilisation factor, system efficiency, and performance ratio. The economic viability of solar PV installation can be assessed by using a set of financial indicators, namely net present value (NPV), the discounted payback period (DPP), the Levelised cost of energy (LCOE), and internal rate of return (IRR).
2.3.1. Technical performance indicators
Total energy generated (kWh)
System yields
The reference yield (YR) is the ratio of the total in-plane solar radiation (HT) to the array reference irradiance (GO) (usually taken as 1 kW/m2). It is a measure of the theoretical energy available at a specific location over a specified period. It represents the number of peak hours per day or the solar radiation (Sharma and Chandel Citation2013). The reference yield was calculated using Equation (3) as used by (Chawla and Tikkiwal Citation2021). where HT is the in-plane radiation and GO is the reference irradiance.
(3)
(3)
Capacity utilisation factor (CUF)
The capacity utilisation factor is the ratio of AC energy (kWh) generated by the solar PV system over a given period (monthly, year) to the energy output (kWh) that would have been generated if the system were operated at full capacity for the entire period. It indicates the number of hours the system will operate at its fully rated capacity per day. CUF can be used to estimate the power output that the system will generate in a particular climatic condition. It can also be used to estimate the economic viability of a solar PV power plant project. The annual capacity utilisation factor of the solar PV system is calculated using Equation (4) as presented by (Malvoni et al. Citation2020):
(4)
(4) where CUF is the capacity utilisation factor, YF is the final yield, PR is the performance ratio and YR is the reference yield.
System efficiency
This is the inverter AC output power () divided by the Total in-plane solar irradiation
multiplied by total PV array area (
) and is determined using equation (5) as used by (Malvoni et al. Citation2020):
(5)
(5)
Total energy losses
The total energy losses of the PV plant (combining PV losses due to irradiance level, array temperature, quality of the module, wiring losses, mismatch, and inverter losses, etc.) represent the difference between the reference yield
and the final yield
and it is calculated as follows as presented by (Adaramola and Vågnes Citation2015):
(6)
(6)
Performance ratio
It is the ratio of final energy yield () and reference yield (
). It allows the comparison of different PV systems independent of geographical location, tilt angle, orientation, and power plant capacity. It is expressed, mathematically, as showed by (Chawla and Tikkiwal Citation2021) in Equation (7):
(7)
(7)
2.3.2. Economic analysis
The aim of the economic analysis was to calculate the costs and the benefits of investment and quantify through financial indicators the economic viability of the PV systems project in the region. The discounted cash flows generated from the investment have been calculated for a period of 20 years, equal to the period in which the feed-in tariff (FiT) is granted by the Government of Kenya (GoK). The financial indicators used for economic analysis in this study are net present value (NPV), the discounted payback period (DPP), the Levelised cost of energy (LCOE), and internal rate of return (IRR). The Strathmore University solar PV power plant project was fully funded through a soft loan at a rate of 4.1% per annum (Da Silva Citation2017). Table presents data used in carrying out the economic analysis of this study as provided through discussions and availed documents.
Levelised cost of energy (LCOE)
Table 6. Financial indicators of solar PV systems.
The levelised cost of energy is the ratio of the total life-cycle cost of the installation to discounted produced energy over its economic life. It is calculated as follows (Behar, Sbarbaro, and Moran Citation2021):
(8)
(8)
(9)
(9) Where
is energy produced,
is degradation rate, and TLCC is the total life-cycle cost. TLCC represents the costs incurred over the economic life of the solar PV installation, and it is calculated using the initial capital investment and annual operation and maintenance cost
.
Net present value (NPV)
This checks the feasibility of the solar PV power plant project. A positive NPV indicates an economically feasible project while a negative NPV indicates an economically infeasible project. It was calculated as follows using Equation (12) indicated by (Behar, Sbarbaro, and Moran Citation2021):
(10)
(10) where
is initial capital investment,
is the discounted cash flow in the year;
is the time of the cash flow;
, is the lifespan of the project
is the discount rate.
Discounted Cash flow
The discounted annual cash flow was obtained from the difference between the annual inflows and annual outflows using Equation (11) (Tudisca et al. Citation2013) where is the annual cash inflows and
is the operation and maintenance (O&M) cost.
(11)
(11)
The discounted annual inflows cash flow was calculated using Equation (12) (Tudisca et al. Citation2013):
(12)
(12) where
is the electrical energy feed to the grid, which was taken as
;
is the electrical energy consumed by the owner taken as
according to (Munene Citation2019).
is the feed-in tariff rate as taken
and annual increment rate of
(Ministry of Energy, Kenya Citation2012), and
is the unit cost of the electrical energy in Kenya which varied from
to
during this period. The energy consumed by the owner was considered as saving.
Discounted Payback period (DPP)
The DPP is the number of years required so that the cumulative discounted cash flow equals the initial capital investment (CO) as presented by (Oloya, Gutu, and Adaramola Citation2021), in Equation (13).
(13)
(13)
Internal rate of return (IRR)
The IRR is the discount rate at which the discounted benefits are equal to the discounted costs. This is determining when a value of NPV equals zero. An investment is convenient if its IRR is higher than a predetermined reference discount rate. The IRR determined using Equation (14) (Behar, Sbarbaro, and Moran Citation2021).
(14)
(14)
2.3.3. Data analysis
The Microsoft Excel spreadsheet was used during the model development and simulation phase. The model developed used power output as output variable, while wind speed, relative humidity, solar irradiance, and ambient temperature were used as input variables. Previous studies in the open literature and the availability of data informed the decision of the selection of the four weather parameters. The study used correlation of different variables with power output for feature selection. The preprocessing technique of each individual simulations were carried out and using coefficient of correlation, P-value, and significance F the best combination of variables was selected. To assess the performance of the developed Model Root Mean Squared Error (RMSE), Mean Average Deviation (MAD), Mean Absolute Error (MAE), and Mean Percentage Error (MAPE) were used.
3. Results and discussion
The results of technical and economic performances, as well as the model development, are presented in this chapter. The chapter begins with technical analysis, development of the model, and economic analysis.
3.1. Technical performance analysis
3.1.1. Reference yield
Figure presents the average daily annual reference yield (YR) calculated for the installation site for a period of 5 years. The results indicated an average reference yield varying from a maximum of 5.54 kWh/kW-day (in year 2015) to a minimum of 5.09 kWh/kW-day (in year 2019) with an average of 5.28 kWh/kW-day and inter-annual coefficient of variability of 2.20%. Similar average results of kWh/kW-day, and
kWh/kW-day were obtained by (Arora, Arora, and Sridhara Citation2022; Daher et al. Citation2018) in northern India and tropical desert respectively. From Table the reference yield of different solar PV systems installed in different regions varied from
kWh/kW-day to
kWh/kW-day. Martín-Martínez et al. (Citation2019) indicated the reference yield of 8 different solar plants which varied from
kWh/kW-day to
kWh/kW-day. Therefore, the values obtained in this study fall within the values reported in other regions.
3.2. Scenario 1 (orientation effect) on system 1 (south) and 4 (west–east) directions
Figure indicates the energy output (kWh) generated by the two systems for a period of 5 years from 2015 to 2019. System 1 generated an energy output of maximum a in the year 2015, and a minimum of
in the year 2018, indicating an average of
per annum and inter-annual coefficient of variability of 2.06%. While system 4 generated a maximum of
in the year 2015 and a minimum of
in the year 2016 indicating an average value of
per annum and an inter-annual coefficient of variability of 1.50%. The energy output (kWh) of systems 1 and 4 declined at a rate of
per year, and
per year respectively. Similar results were obtained by (Oloya, Gutu, and Adaramola Citation2021) indicated more energy output in years
and
compared to energy output in the year
for a system installed near the equator.
Figure shows that System 1 generated more energy output (kWh) performance than System 4 which was associated with good installation orientation. The high performance in the year 2015 can be associated with modules being newly installed, thus high radiation transmittance due to absence of dust accumulation and other degradation mechanisms. While low performance in 2018 can be associated with high relative humidity experienced during the year. The effect of relative humidity on the power output of solar PV modules has been demonstrated by the model presented in Section 3.4 of this paper. The lowest performance in 2016 can be associated with the Light Induced Degradation (LID) which usually affects the module during its initial period of operation.
Figure shows the final yield (YF) for system 1 and system 4 for a period of 5 years. The results indicate an average yearly final yield of the system 1 which had been installed at a fixed angle of and oriented south indicating a maximum value of 3.80 kWh/kW-day, in the year 2015, and a minimum value of 3.43 kWh/kW-day, in the year 2018 and an annual average value of 3.60 kWh/kW-day and inter-annual coefficient of variability of 2.63%. The final yield of system 4 which was installed at a fixed angle of
and oriented east and west indicated a maximum value of 3.65 kWh/kW-day, in the year 2015, and a minimum value of 3.38 kWh/kW-day, in the year 2016, with an annual average value of 3.46 kW/kW-day and inter-annual coefficient of variability of 1.51%.
Similar average values of 3.78, 3.98 kWh/kW-day and kWh/kW-day were obtained by (Al-Badi Citation2020; Necaibia et al. Citation2018; Yadav and Bajpai Citation2018). From Table the final yield of different solar PV systems installed in different regions varied from
kWh/kW-day to
kWh/kW-day. Martín-Martínez et al. (Citation2019) calculated the final yield of 8 different solar plants which varied from 2.04 kWh/kW-day to
kWh/kW-day.
Figure shows the system efficiency for the two installations for a period of 5 years. The results indicate an average system efficiency of system 1 which had been installed at a fixed angle of and oriented south recorded a minimum of 9.35%, in the year 2018 and a maximum of 10.89% in the year, 2017, with an annual average of 10.09% and inter-annual coefficient of variability of 4.13%. The efficiency of system 4 which had been installed at a fixed angle of
and oriented east and west indicated a minimum of 9.18%, in the year 2018 and a maximum of 10.47% in the year 2017 with an annual average of 9.78% and inter-annual coefficient of variability of 3.05%. From Table the system efficiency of different solar PV systems installed in different regions varied from
to
Martín-Martínez et al. (Citation2019) indicated the efficiency of 8 different solar plants that varied from
to
Therefore, the findings of this study were consistent with the results obtained from other regions of low operating efficiency of modules in real life climatic conditions.
Figure shows the CUF for system 1 and system 4 for a period of 5 years. The results indicate an average CUF of system 1 which had been installed at a fixed angle of and oriented south indicated of a maximum value of as
in the year 2015 and a minimum value of
in the year
and in the year
with an annual average of
and inter-annual coefficient of variability of 3.06%. The CUF of system 4 which had been installed at fixed angle of
and oriented east and west was maximum in the year 2015 at 0.15, and a minimum of 0.14, in the subsequent years with an annual average/mode of 0.14 and inter-annual coefficient of variability of 1.59%. Seme et al. (Citation2019) found that CUF varied from 9.61% to 14.57% with an average of 11.85%. This implies that solar PV can operate for an average of 2.84 h per day in Slovenia which is lower than the average of 3.6 h per day in tropical savanna climatic conditions in Kenya.
From Table the CUF of different solar PV systems installed in different regions varied from to
which are within the range of results reported by previous studies in different locations such as Shiva and Sudhakar (Citation2015), 17.68%; Khare Saxena, Saxena, and Sudhakar (Citation2021), 19.27%; Sreenath, Sudhakar, and Yusop (Citation2022), 16.5% to 18.8%; Sreenath, Sudhakar, and Af (Citation2021), 14.25% to 17.09%; and Saxena, Saxena, and Sudhakar (Citation2021), 19% to 21%. Martín-Martínez et al. (Citation2019) calculated the CUF of 8 different solar plant which varied from
to
Figure shows the PR for the two installations for a period of 5 years. The results indicate an average PR of the system 1 which had been installed at fixed angle of and oriented south recorded a minimum of 64%, in the year 2018 and a maximum of 75%, in the year 2019, with an annual average of 69% and inter-annual coefficient of variability of 3.34%. The PR of system 4 which had been installed at fixed angle of
and oriented east and west was calculated a minimum of 64%, in the year 2018 and a maximum of 70%, in the year 2019, with an annual average of 67% and inter-annual coefficient of variability of 1.94%.
From the information presented in Table , it can be observed that the PR obtained from this study are similar to results reported by studies in different locations of different solar PV systems installed in different regions varied from to
See for examples: Shiva and Sudhakar (Citation2015), 86.12%; Khare Saxena, Saxena, and Sudhakar (Citation2021), 80.72%; Sreenath, Sudhakar, and Yusop (Citation2022), 75%; Sreenath, Sudhakar, and Af (Citation2021), 80%; (Saxena, Saxena, and Sudhakar Citation2021), 70% to 80% and (Martín-Martínez et al. Citation2019),
to
3.3. Scenario 2 tilt angle effect on system 2 (18o) and system 3 (11o)
Figure shows the energy output (kWh) generated by the two systems for a period of 5 years from the year 2015 to the year 2019. System 2 generated energy output (MWh) of maximum in the year 2015 and a minimum of
in the year 2018, recording an annual average value of
and inter-annual coefficient of variability of 2.58%. While system 3 generated a maximum of
in the year 2015 and a minimum of
in the year
with an annual average value of
and inter-annual coefficient of variability of 4.34%. The energy output (kWh) of systems 2 and 3 declined at an annual average rate of
and
respectively.
System 3 indicates better performance than system 4 between the years and
which can be associated with lower tilt angle. The site being near the equator low tilt angle will reduce partial shading of the system hence better performance. However, low tilt angle causes dust accumulation on the lower side of the module with time, hence creating partial shading or hot spots and eventually reduction in energy output as indicated by performance of System 4 in year
and
. Therefore, regular manual cleaning was required. This study results shows that as tilt angle is increased, the performance also increases as indicated by (Babatunde, Abbasoglu, and Senol Citation2018).
Figure shows the average final yield of the system 2 which had been installed at fixed angle of and oriented south, indicated a maximum of 3.80 kWh/kW-day in the year
, a minimum of 3.46 kWh/kW-day in the year
, with an annual average of 3.61 kWh/kW-day and inter-annual coefficient of variability of 2.13%. The final yield of the system 3 which was installed at fixed angle of
and oriented south, was calculated as maximum of 3.86 kWh/kW-day in the year
, and minimum of 3.20 kWh/kW-day in the year
with an annual average of 3.54 kW/kW-day and inter-annual coefficient of variability of 4.55%. (Ashwini, Raj, and Gupta Citation2016) conducted simulation of fixed tilt angles of
,
and
which determined the final yield (kWh/kW-day) as
,
and
, respectively. Hence the highest tilt angle showed the highest performance.
Figure shows the yearly average system efficiency of the system 2 which had been installed at fixed angle of and oriented south was determine as a maximum of 11% in the year
, and a minimum of 9.38% in the year
with an annual average of 10.22% and inter-annual coefficient of variability of 4.01%. The efficiency of system 3 which was installed at fixed angle of
and oriented south was determined as a maximum of 10.91% in the year
and a minimum of 8.43% in the year
with an annual average of 9.78% and inter-annual coefficient of variability of 4.86%.
Figure shows the yearly average CUF of the system 2 which had been installed at a fixed angle of and oriented south was determined as a maximum of 0.16 in the year
a minimum of 0.14 in the year
with an annual average of 0.15 and inter-annual coefficient of variability of 1.89%. The CUF of system 3 which was installed at a fixed angle of
and oriented south was determined as a maximum of 0.16 in the year
and a minimum of 0.13 in the year 2019, with an annual average of 0.15 and inter-annual coefficient of variability of 4.97%.
Figure shows the average PR of system 2 which had been installed at a fixed angle of and oriented south had was determined as maximum of 72% in years
and
and a minimum of 65% in the year
, with an annual average of 69% and inter-annual coefficient of variability of 2.36%. The PR of system 3 which was installed at fixed angle of
and oriented south was determined` as a maximum of 74% in the year
, a minimum of 62% in the year
, with an annual average of 67% and inter-annual coefficient of variability of 3.90%. Ashwini, Raj, and Gupta (Citation2016) conducted simulation of fixed tilt angles of
,
and
and determined the performance ratio (%) as
,
and
, respectively. Chawla and Tikkiwal (Citation2021) simulated solar PV system of fixed and variable tilt angles of Poly-Si in Barmer, Jodhpur, Jaisalmer, and Kalan Ghat in arid climatic conditions of India and established the PR as
and
respectively in a fixed tilt angle and
and
for a variable tilt angle.
The first scenario was concerned with the effects of orientation against performance. System 1 which was installed with a tilt angle of and oriented south was established as an average of energy output, final yield, CUF, PR, and efficiency of
3.6kWh/kW,
While system 4 which was installed with a tilt angle of
and oriented west and east was determined as average of energy output, final yield, CUF, PR and efficiency of
3.46kWh/kW,
System 1 indicated slightly better performance than system 4 due to the fact that it was better oriented in relation to the solar path.
The second scenario was concerned with the effects of tilt angle against performance. The solar module installed with a tilt angle of and oriented south was determined as an average of energy output, final yield, CUF, PR and efficiency of
3.61kWh/kW,
While the solar module installed with a tilt angle of
oriented south was determined as an average of energy output, final yield, CUF, PR, and efficiency of
3.54kWh/kW,
The overall average CUF for all four systems in 5 years was established as 0.15. The CUF of 0.15 implies that the solar PV power plant operated for 3.6 h per day at its fully rated capacity. The country estimates solar insolation of 4–6 kWh/m2/day. The lowest CUF was obtained in the year 2017 in the month of June by systems 1 and 2 which was determined as 0.08. This implies that the system operated for 1.92 h per day at its fully rated capacity. The highest CUF was determined as 0.21 in the month of January by system 1. This implied that the system operated for 5.04 h per day at its fully rated capacity. The overall average PR for the site was established as 69%. The lowest PR was determined in the month of March in the year 2018 where systems 1, 2, 3, and 4 were determined as 44%, 44%, 43%, and 43%, respectively. The highest was determined in the month of December 2019 at 97%. The results indicated that solar PV power plants can generate power output throughout the year in the region.
3.4. Estimation of power output (kW) using environmental parameters
The environmental parameters which include solar irradiance (W/m2), ambient temperature ( wind velocity (m/s), and relative humidity were considered in this study. The use of a power output model to estimate power output can be a useful tool in predicting the performance and economic indicators of the solar PV power plant during the design, installation, and operation stages. The use of weather parameters as input variables in the prediction of the power output model can be useful due to the availability of the data from various weather stations and satellite data. Most of the models developed such as (Ayadi et al. Citation2019; Bhattacharya, Chakraborty, and Pal Citation2014; Mensah, Yamoah, and Adaramola Citation2019; Njok and Ogbulezie Citation2019; Salimi et al. Citation2020) has used a single parameter as input to predict the power output, efficiency, current, and voltage.
To the best of our knowledge as indicated by various models in the open literature, none had considered the use of three parameters as input to the model. This study has developed two models using the three weather parameters as input, with power output as output variable. One of the parameters is held constant depending on the average value of that day, month, or year. This holding of one of the parameters constants, but can be varied depending on the conditions, is useful in estimating power output under given conditions. The first model uses solar irradiance, wind speed and ambient temperature as input variables, while the second model utilises solar irradiance, relative humidity, and wind speed as inputs.
Figure shows the relationship between the monthly average power output (W) of the solar PV power plant against the monthly solar irradiance (W/m2), wind speed (m/s), holding ambient temperature average value of 20.44. Using the information presented in Figure , expression (with coefficient of correlation R2 = 0.97) was developed for the final power output as a function of solar irradiance, wind speed, and ambient temperature as presented in Equation (15). Ayadi et al. (Citation2019) developed a model to indicate the relationship between power output and wind speed with
and power output against temperature with
Figure 14. Power output when ambient temperature is held at an average value of 20.44°C. Y and X axis indicate average monthly values (January to December).

Bhattacharya, Chakraborty, and Pal (Citation2014) developed a model between efficiency and wind speed with and efficiency and ambient temperature with
Salimi et al. (Citation2020) developed a model between power output versus solar radiation and ambient temperature with
(Hammad et al. Citation2018) developed a model between the effects of dust, temperature versus efficiency with
Mensah, Yamoah, and Adaramola (Citation2019) developed a model for energy generated against solar radiation with
Njok and Ogbulezie (Citation2019) developed models on current versus panel temperature with
and effieciency versus ambient temperature with
(15)
(15) where
is the solar irradiance (
),
is the wind speed (
) and
is the ambient temperature (
). The results indicate that as the wind speed and solar irradiance increases the power output increases. It can also be noted that as the solar irradiance increases and the wind speed increases, it translates to an increase of power output. The model indicated the mean average deviation (MAD) of 237.81 between the measured and predicted values. In addition, the mean average percentage error (MAPE) was determined as 4.11%, MAE was established as 21.62, and RMSE was calculated as 286.35. Ziane et al. (Citation2021) indicated the RMSE values that ranged from 104.67 to 325.21 and MAE ranged from 47.39 to 167.84. In addition, Gopi et al. (Citation2021) developed different models and reported percentage variation between measured and predicted values as 3.76%,1.31%,7.61%, 7.29%, 9.76% and 12.56%.
The second consideration was to develop a model using power output (W), solar irradiance (W/m2), relative humidity (%), and wind speed (m/s). Figure shows the variation of power output with solar irradiance, relative humidity while holding wind speed at an average value of 3.17 m/s. The results were obtained from Equation (16) with coefficient of correlation of R2 = 0.91, where is relative humidity. Ayadi et al. (Citation2019) developed a model of power output against relative humidity with
Njok and Ogbulezie (Citation2019) developed two model between current versus relative humidity with
and efficiency versus relative humidity with
Therefore, by the use of more weather parameters, the coefficient obtained from the derived model indicates good accuracy and validity of the predicted results.
(16)
(16)
Figure 15. Power output (W) when wind speed (m/s) is held at an average value of 3.17 m/s. Y and X axis indicate average monthly values (January to December).

The results indicate that as relative humidity decreases, wind speed increases and solar irradiance decreases, the power output increases. The model indicated the mean average deviation (MAD) of 190.33 between the measured and predicted values. In addition, the mean average percentage error (MAPE) was determined as 3.59%, MAE was established as 17.3, and RMSE was calculated as 245.5.
3.5. Economic analysis
The total project cost of kW was installed at
. This amount was used to install
arrays. All the 30-arrays had identical parts such as number and types of modules, inverters, and other components. Therefore, this study assumed that each array cost was the same, hence
each. The project life span was taken as 20 years according to the FiT policy (Ministry of Energy, Kenya Citation2012). The LCOE of the project was established as US$0.1679/kWh taking the loan repayment period as 7 years.
The financial indicators of the project were calculated as NPV of IRR of
DPB of
years and SPP of
years. Table indicates the results of NPV, IRR, DPP and LCOE when the four systems were considered individually. The results indicate that NPV ranged from
to
DPB ranged between
years to
years, IRR ranged from
to
and LCOE ranged from
to
Other studies have determined the LCOE values of
by (Adaramola Citation2015), (Mensah, Yamoah, and Adaramola Citation2019) indicated a LCOE of USA$0.2411 and payback period (DPB) of 14.95 years.
Yazdani and Yaghoubi (Citation2021) indicated a LCOE of and DPB of 5.82 years, Alshare et al. (Citation2020) indicated PBP of
years and IRR of
(Omar and Mahmoud Citation2018) indicated DPB (years) and IRR (%) of the three systems as
years,
years and
years and
and
respectively while Sharma and Chandel (Citation2013) reported DPB of
years; Khare Saxena, Saxena, and Sudhakar (Citation2021), reported a simple payback period of 5.9 years; Saxena, Saxena, and Sudhakar (Citation2021) reported a 5–6 years; and Sreenath, Sudhakar, and Af (Citation2021) reported a 7.9 years and LCOE of US$0.0102. The LCOE values determined in this study, which are between
and
, are relatively lower than values reported by other studies (as indicated above). This may be associated with low cost financing the project at discount rate of 4.1% and relatively energy high energy yield when compared with other studies in different climatic regions. However, the discounted payback period agrees with other studies of
years and
years.
4. Conclusions
The aim of the study was to examine the technical and economic performance and develop the models of solar PV installations using long-term performance data. This has provided more information for better assessment of solar PV installation in the region. The annual average energy output , power output
reference yields
final yields
capacity utilisation factor (CUF), system efficiency (η) and PR(%) were established as
and
, respectively. The economic indicators of the system were established as LCOE of
NPV of
DPP of
years, SPP of
years and IRR of
This study has developed two models to estimate power output using 3 weather parameters as input variables. The results have indicated a high coefficient of determination (R2) obtained from the models developed which translates to good accuracy and validity of the predicted results. This study has established that when relative humidity increases the power output of a solar PV system decreases. Increase in wind speed and solar irradiance will affect the power output of solar PV modules positively. More models need to be developed in order to estimate the power output of solar PV systems which can help in design, installation and operation stages. Furthermore, the study recommends development of methods to maintain optimum weather parameters operation conditions especially ambient temperature and the investigation of the effects of climatic change on the performance of solar PV modules.
The study established that the solar PV system was more useful in terms of energy savings. Hence, the solar PV module using polycrystalline technology can be used as an alternative source of electrical energy and the project can be economically viable in tropical savanna climatic conditions in the region. The results of this study were based on the cost of the year 2013 to the year 2014 during the design, installations, and commissioning of the project. The study recommends further investigation to establish LCOE using the cost reduction trends and effects in the improvement of the efficiency of the solar PV technology. Finally, the study further recommends the use of other methods other than correlation and feature selection to determine the impact of meteorological variables on forecasting the power output PV module systems in tropical savanna climatic conditions.
Acknowledgements
I wish to thank ACE II PTRE, Moi University for funding this research and Strathmore University for accepting this study to use their facility as the case study. In addition, support provided by the project ‘Strengthening education, research and innovation capacity in sustainable energy for economic development’, a collaborative project between Norwegian University of Life Sciences Ås, Norway and Moi University Eldoret Kenya, under the Norwegian Partnership Programme for Global Academic Cooperation (NORPART) and funded by the Norwegian Ministry of Education and Research and the Norwegian Ministry of Foreign Affairs, is acknowledged.
Disclosure statement
No potential conflict of interest was reported by the author(s).
Additional information
Funding
References
- Adaramola, M. S. 2015. “Techno-Economic Analysis of a 2.1 kW Rooftop Photovoltaic-Grid-Tied System Based on Actual Performance.” Energy Conversion and Management 101: 85–93. https://doi.org/10.1016/j.enconman.2015.05.038.
- Adaramola, M. S., and E. E. T. Vågnes. 2015. “Preliminary Assessment of a Small-Scale Rooftop PV-Grid Tied in Norwegian Climatic Conditions.” Energy Conversion and Management 90: 458–465. https://doi.org/10.1016/j.enconman.2014.11.028.
- Al-Badi, A. 2020. “Performance Assessment of 20.4 kW Eco-House Grid-Connected PV Plant in Oman.” International Journal of Sustainable Engineering 13 (3): 230–241. https://doi.org/10.1080/19397038.2019.1658824.
- Alshare, A., B. Tashtoush, S. Altarazi, and H. El-khalil. 2020. “Energy and Economic Analysis of a 5 MW Photovoltaic System in Northern Jordan.” Case Studies in Thermal Engineering 21: 100722. https://doi.org/10.1016/j.csite.2020.100722.
- AlSkaif, T., S. Dev, L. Visser, M. Hossari, and W. van Sark. 2019. “On the Interdependence and Importance of Meteorological Variables for Photovoltaic Output Power Estimation.” 2019 IEEE 46th Photovoltaic Specialists Conference (PVSC), 2117–2120. https://doi.org/10.1109/PVSC40753.2019.8981308.
- Arora, R., R. Arora, and S. N. Sridhara. 2022. “Performance Assessment of 186 kWp Grid Interactive Solar Photovoltaic Plant in Northern India.” International Journal of Ambient Energy 43 (1): 128–141. https://doi.org/10.1080/01430750.2019.1630312.
- Ashwini, K., A. Raj, and M. Gupta. 2016. “Performance Assessment and Orientation Optimization of 100 kWp Grid Connected Solar PV System in Indian Scenario.” 2016 International Conference on Recent Advances and Innovations in Engineering (ICRAIE), 1–7. https://doi.org/10.1109/ICRAIE.2016.7939505.
- Ayadi, F., I. Colak, N. Genc, and H. I. Bulbul. 2019. “Impacts of Wind Speed and Humidity on the Performance of Photovoltaic Module.” 2019 8th International Conference on Renewable Energy Research and Applications (ICRERA), Brasov, Romania, 2019, 229–233, https://doi.org/10.1109/ICRERA47325.2019.8996718.
- Babatunde, A. A., S. Abbasoglu, and M. Senol. 2018. “Analysis of the Impact of Dust, Tilt Angle and Orientation on Performance of PV Plants.” Renewable and Sustainable Energy Reviews 90: 1017–1026. https://doi.org/10.1016/j.rser.2018.03.102.
- Banda, M. H., K. Nyeinga, and D. Okello. 2019. “Performance Evaluation of 830 kWp Grid-Connected Photovoltaic Power Plant at Kamuzu International Airport-Malawi.” Energy for Sustainable Development 51: 50–55. doi:10.1016/j.esd.2019.05.005.
- Behar, O., D. Sbarbaro, and L. Moran. 2021. “Which is the Most Competitive Solar Power Technology for Integration Into the Existing Copper Mining Plants: Photovoltaic (PV), Concentrating Solar Power (CSP), or Hybrid PV-CSP?” Journal of Cleaner Production 287: 125455. https://doi.org/10.1016/j.jclepro.2020.125455.
- Bhattacharya, T., A. K. Chakraborty, and K. Pal. 2014. “Effects of Ambient Temperature and Wind Speed on Performance of Monocrystalline Solar Photovoltaic Module in Tripura, India.” Journal of Solar Energy 2014: 1–5. https://doi.org/10.1155/2014/817078.
- Chawla, S., and V. A. Tikkiwal. 2021. “Performance Evaluation and Degradation Analysis of Different Photovoltaic Technologies Under Arid Conditions.'.” International Journal of Energy Research 45 (1): 786–798. https://doi.org/10.1002/er.5901.
- Daher, D. H., L. Gaillard, M. Amara, and C. Ménézo. 2018. “Impact of Tropical Desert Maritime Climate on the Performance of a PV Grid-Connected Power Plant.” Renewable Energy 125: 729–737. https://doi.org/10.1016/j.renene.2018.03.013.
- Da Silva, I. P. 2017. Towards a Sustainable Strathmore University: 600 kW Grid Connected Solar Energy System [Interview]. Retrieved 15 March 2023. https://strathmore.edu/news/towards-a-sustainable-strathmore-university-600-kw-grid-connected-solar-energy-system/.
- El Hacen Jed, M., R. Ihaddadene, N. Ihaddadene, C. E. Elhadji Sidi, and M. El Bah. 2020. “Performance analysis of 954,809 kWp PV array of Sheikh Zayed solar power plant (Nouakchott, Mauritania).” Renewable Energy Focus 32: 45–54. https://doi.org/10.1016/j.ref.2019.11.002.
- Goel, S., and R. Sharma. 2021. “Analysis of Measured and Simulated Performance of a Grid-Connected PV System in Eastern India.” Environment, Development and Sustainability 23 (1): 451–476. https://doi.org/10.1007/s10668-020-00591-7.
- Gopi, A., K. Sudhakar, N. W. Keng, A. R. Krishnan, and S. S. Priya. 2021. “Performance Modeling of the Weather Impact on a Utility-Scale PV Power Plant in a Tropical Region.” International Journal of Photoenergy 2021: 5551014. https://doi.org/10.1155/2021/5551014.
- Hammad, B., M. Al-Abed, A. Al-Ghandoor, A. Al-Sardeah, and A. Al-Bashir. 2018. “Modeling and Analysis of Dust and Temperature Effects on Photovoltaic Systems’ Performance and Optimal Cleaning Frequency: Jordan Case Study.” Renewable and Sustainable Energy Reviews 82: 2218–2234. https://doi.org/10.1016/j.rser.2017.08.070.
- IEA. 2020. Electricity generation by source, Africa. International Energy Agency. Retrieved 15 March 2023. https://www.iea.org/regions/africa.
- KhareSaxena, A., S. Saxena, and K. Sudhakar. 2021. “Energy Performance and Loss Analysis of 100 kWp Grid-Connected Rooftop Solar Photovoltaic System.” Building Services Engineering Research and Technology 42 (4): 485–500. https://doi.org/10.1177/0143624421994224.
- Malvoni, M., N. M. Kumar, S. S. Chopra, and N. Hatziargyriou. 2020. “Performance and Degradation Assessment of Large-Scale Grid-Connected Solar Photovoltaic Power Plant in Tropical Semi-Arid Environment of India.” Solar Energy 203: 101–113. https://doi.org/10.1016/j.solener.2020.04.011.
- Martín-Martínez, S., M. Cañas-Carretón, A. Honrubia-Escribano, and E. Gómez-Lázaro. 2019. “Performance Evaluation of Large Solar Photovoltaic Power Plants in Spain.” Energy Conversion and Management 183: 515–528. https://doi.org/10.1016/j.enconman.2018.12.116.
- Mensah, L. D., J. O. Yamoah, and M. S. Adaramola. 2019. “Performance Evaluation of a Utility-Scale Grid-Tied Solar Photovoltaic (PV) Installation in Ghana.” Energy for Sustainable Development 48: 82–87. https://doi.org/10.1016/j.esd.2018.11.003.
- Ministry of Energy, Kenya. 2012. Feed-In-Tariffs Policy on Wind, Biomass, Small-Hydro, Geothermal, Biogas and Solar Resource Generated Electricity. Government of Kenya. Retrieved 15 March 2023. https://repository.kippra.or.ke/bitstream/handle/123456789/1016/Feed%20in%20Tariff%20Policy%202012.pdf?sequence=1.
- Munene, L. N. 2019. “Reducing Carbon Emissions: Strathmore University Contributions Towards Sustainable Development in Kenya.” African Journal of Business Ethics 13: 1. https://doi.org/10.15249/13-1-173.
- National Energy Policy, Kenya. 2018. National Energy Policy. Government of Kenya. Retrieved 15 March 2023. https://repository.kippra.or.ke/bitstream/handle/123456789/1947/BL4PdOqKtxFT_National%20Energy%20Policy%20October%20%202018.pdf?sequence=1&isAllowed=y.
- Necaibia, A., A. Bouraiou, A. Ziane, N. Sahouane, S. Hassani, M. Mostefaoui, R. Dabou, and S. Mouhadjer. 2018. “Analytical Assessment of the Outdoor Performance and Efficiency of Grid-Tied Photovoltaic System Under hot dry Climate in the South of Algeria.” Energy Conversion and Management 171: 778–786. https://doi.org/10.1016/j.enconman.2018.06.020.
- Ngure, S. M., A. B. Makokha, E. O. Ataro, and M. S. Adaramola. 2022. “Degradation Analysis of Solar Photovoltaic Module Under Warm Semiarid and Tropical Savanna Climatic Conditions of East Africa.” International Journal of Energy and Environmental Engineering 13 (2): 431–447. https://doi.org/10.1007/s40095-021-00454-5.
- Njok, A. O., and J. C. Ogbulezie. 2019. “The Effect of Relative Humidity and Temperature on Polycrystalline Solar Panels Installed Close to a River.” Physical Science International Journal 20 (4): 1–11. https://doi.org/10.9734/PSIJ/2018/44760.
- Oloya, I. T., T. J. L. Gutu, and M. S. Adaramola. 2021. “Techno-Economic Assessment of 10 MW Centralised Grid-Tied Solar Photovoltaic System in Uganda.” Case Studies in Thermal Engineering 25: 100928. https://doi.org/10.1016/j.csite.2021.100928.
- Omar, M. A., and M. M. Mahmoud. 2018. “Grid Connected PV- Home Systems in Palestine: A Review on Technical Performance, Effects and Economic Feasibility.'.” Renewable and Sustainable Energy Reviews 82: 2490–2497. https://doi.org/10.1016/j.rser.2017.09.008.
- Ramanan, P., M. K. Kalidasa, and A. Karthick. 2019. “Performance Analysis and Energy Metrics of Grid-Connected Photovoltaic Systems.” Energy for Sustainable Development 52: 104–115. https://doi.org/10.1016/j.esd.2019.08.001.
- Saad, B., R. Errattahi, A. E. Hannani, and A. Aqqal. 2022. “The Impact of Forecasting Horizon and Resolution on PV Power Prediction using Artificial Neural Networks.” 11th International Symposium on Signal, Image, Video and Communications (ISIVC), 1–6. https://doi.org/10.1109/ISIVC54825.2022.9800748.
- Sahouane, N., R. Dabou, A. Ziane, A. Neçaibia, A. Bouraiou, A. Rouabhia, and B. Mohammed. 2019. “Energy and Economic Efficiency Performance Assessment of a 28 kWp Photovoltaic Grid-Connected System Under Desertic Weather Conditions in Algerian Sahara.” Renewable Energy 143: 1318–1330. https://doi.org/10.1016/j.renene.2019.05.086.
- Salimi, H., H. A. D. Ashtiani, A. L. Mirabdolah, and R. Fazaeli. 2020. “Experimental Analysis and Modeling of Weather Condition Effects on Photovoltaic Systems’ Performance: Tehran Case Study.” Energy Sources, Part A: Recovery, Utilization, and Environmental Effects, https://doi.org/10.1080/15567036.2020.1765902.
- Saxena, A. K., S. Saxena, and K. Sudhakar. 2021. “Energy, Economic and Environmental Performance Assessment of a Grid-Tied Rooftop System in Different Cities of India Based on 3E Analysis.” Clean Energy 5 (2): 288–301. https://doi.org/10.1093/ce/zkab008.
- Seme, S., K. Sredenšek, B. Štumberger, and M. Hadžiselimović. 2019. “Analysis of the Performance of Photovoltaic Systems in Slovenia.” Solar Energy 180: 550–558. https://doi.org/10.1016/j.solener.2019.01.062.
- Sharma, V., and S. S. Chandel. 2013. “Performance Analysis of a 190 kWp Grid Interactive Solar Photovoltaic Power Plant in India.” Energy 55: 476–485. https://doi.org/10.1016/j.energy.2013.03.075.
- Shiva, K. B., and K. Sudhakar. 2015. “Performance Evaluation of 10 MW Grid Connected Solar Photovoltaic Power Plant in India.” Energy Reports 1: 184–192. https://doi.org/10.1016/j.egyr.2015.10.001.
- Sreenath, S., K. Sudhakar, and Y. Af. 2021. “7E Analysis of a Conceptual Utility-Scale Land-Based Solar Photovoltaic Power Plant.” Energy 219: 119610. https://doi.org/10.1016/j.energy.2020.119610.
- Sreenath, S., K. Sudhakar, and A. F. Yusop. 2022. “Carbon Mitigation Potential of the Airport-Based Solar PV Plants in the Indian Context.” International Journal of Ambient Energy 43 (1): 1311–1319. https://doi.org/10.1080/01430750.2019.1696888.
- Thotakura, S., S. C. Kondamudi, J. F. Xavier, M. Quanjin, G. R. Reddy, P. Ganger, and S. L. Davuluri. 2020. “Operational Performance of Megawatt-Scale Grid Integrated Rooftop Solar PV System in Tropical wet and dry Climates of India.” Case Studies in Thermal Engineering 18: 100602. https://doi.org/10.1016/j.csite.2020.100602.
- Tudisca, S., A. M. Di Trapani, F. Sgroi, R. Testa, and R. Squatrito. 2013. “Economic Analysis of PV Systems on Buildings in Sicilian Farms.” Renewable and Sustainable Energy Reviews 28: 691–701. https://doi.org/10.1016/j.rser.2013.08.035.
- Vidal, H., M. Rivera, P. Wheeler, and N. Vicencio. 2020. “The Analysis Performance of a Grid-Connected 8.2 kWp Photovoltaic System in the Patagonia Region.” Sustainability 12 (21): 9227. https://doi.org/10.3390/su12219227.
- Yadav, S. K., and U. Bajpai. 2018. “Performance Evaluation of a Rooftop Solar Photovoltaic Power Plant in Northern India.” Energy for Sustainable Development 43: 130–138. https://doi.org/10.1016/j.esd.2018.01.006.
- Yazdani, H., and M. Yaghoubi. 2021. “Techno-Economic Study of Photovoltaic Systems Performance in Shiraz, Iran.” Renewable Energy 172: 251–262. https://doi.org/10.1016/j.renene.2021.03.012.
- Ziane, A., A. Necaibia, N. Sahouane, R. Dabou, M. Mostefaoui, A. Bouraiou, S. Khelifi, A. Rouabhia, and M. Blal. 2021. “Photovoltaic Output Power Performance Assessment and Forecasting: Impact of Meteorological Variables.” Solar Energy 220: 745–757. https://doi.org/10.1016/j.solener.2021.04.004.