Abstract
Background
Arterial stiffening is believed to contribute to the worsening of insulin resistance, and factors which are associated with needing pharmacological treatment of gestational diabetes (GDM), such as maternal obesity or advanced age, are associated with impaired cardiovascular adaptation to pregnancy. In this observational study, we aimed to investigate causal relationships between maternal haemodynamics and treatment requirement amongst women with GDM.
Methods
We assessed maternal haemodynamics in women with GDM, comparing those who remained on dietary treatment with those who required pharmacological management. Maternal haemodynamics were assessed using the Arteriograph® (TensioMed Ltd, Budapest, Hungary) and the NICOM® non-invasive bio-reactance method (Cheetah Medical, Portland, Oregon, USA). A graphical causal inference technique was used for statistical analysis.
Results
120 women with GDM were included in the analysis. Maternal booking BMI was identified as having a causative influence on treatment requirement, with each unit increase in BMI increasing the odds of needing metformin and/or insulin therapy by 12% [OR 1.12 (1.02 − 1.22)]. The raw values of maternal heart rate (87.6 ± 11.7 vs. 92.9 ± 11.90 bpm, p = 0.014) and PWV (7.8 ± 1.04 vs. 8.4 ± 1.61 m/s, p = 0.029) were both significantly higher amongst the women requiring pharmacological management, though these relationships did not remain significant in causal logistic regression.
Conclusions
Maternal BMI at booking has a causal, rather than simply associational, relationship on the need for pharmacological treatment of GDM. No significant causal relationships were found between maternal haemodynamics and the need for pharmacological treatment.
PLAIN LANGUAGE SUMMARY
This observational study is the first to examine relationships between maternal haemodynamics and treatment requirement for gestational diabetes (GDM). This is also the first study to demonstrate a causative, rather than simply associational, relationship between maternal body mass index (BMI) and the need for pharmacological treatment of GDM, with each unit increase in BMI increasing the odds of needing metformin and/or insulin therapy by 12%. Maternal heart rate and pulse wave velocity were significantly higher among women with GDM requiring pharmacological management, but this finding did not remain significant in logistic regression analysis, and no causative relationships between maternal hemodynamics and treatment requirement were identified. Our findings highlight the importance of pre- and peri-conception weight control, but do not support a role for measurement of maternal hemodynamics in the prediction of women who are likely to require pharmacological management of GDM.
Introduction
Gestational Diabetes Mellitus (GDM) is one of the most common medical disorders encountered in pregnancy. The incidence is also rising rapidly, driven largely by the increase in maternal obesity (Petry et al. Citation2019). Various factors have been implicated with needing pharmacological therapy for treatment of GDM in addition to dietary intervention, including a history of GDM, higher fasting and post-prandial diagnostic glucose levels, Black and Asian ethnicity, increased body mass index (BMI), and increased maternal age (Eleftheriades et al. Citation2021; Martine-Edith et al. Citation2021; Pertot et al. Citation2011; Sapienza et al. Citation2010; Wang et al. Citation2021).
During normal pregnancy the maternal cardiovascular system undergoes significant changes, characterised by an increase in cardiac output (CO) and decrease in total peripheral resistance (TPR) and central arterial stiffness (AS) (Anness et al. Citation2022; Meah et al. Citation2016). Several studies investigating the cardiovascular changes in pregnancies complicated by GDM have demonstrated cardiovascular maladaptation. Mean arterial pressure (MAP) and brachial (BrAIx) and aortic (AoAIx) augmentation index are higher amongst women who tested positively for GDM compared to women whose screen was negative (Osman et al. Citation2018) and other studies have reported reduced CO (Meccaci et al. 2022), increased MAP (Anzoategui et al. Citation2022) and increased AS (Kintiraki et al. Citation2018; Savvidou et al. Citation2010) amongst women with GDM compared to uncomplicated pregnancies, although these conclusions have not been unanimous (Mansukhani et al. Citation2022).
Factors associated with needing pharmacological management of GDM are associated with impaired cardiovascular adaptation to pregnancy: increasing maternal age is associated with decreased CO and increased TPR and AS, and increasing BMI is associated with increased CO, TPR and AS (Anness et al. Citation2022; Guy et al. Citation2017; Ling et al. Citation2021; Perry et al. Citation2019). In addition, the relationship between insulin resistance and vascular dysfunction is thought to be reciprocal, with endothelial damage and arterial stiffening caused by higher levels of nitric oxide and endothelin-1 contributing to a worsening insulin resistance, and vice versa (Kim et al. Citation2006). Given these associational and biological links, it is possible that maternal haemodynamics may be associated with needing pharmacological management of GDM, however there are currently no studies which have investigated this hypothesis.
Most studies investigating haemodynamics amongst women with GDM have presented women with GDM on all treatments as a single study group. Mansukhani et al. (Citation2022) compared women with GDM on dietary management with those on metformin or insulin therapy reported a higher pulse wave velocity (PWV) in those treated pharmacologically, but only examined women at a single time point in the third trimester and did not examine the women prior to initiation of pharmacological treatment. The study therefore only described the women on different treatments and was not able to comment on whether the need for pharmacological treatment arose from the altered haemodynamics seen in these groups.
In addition, the designs and statistical methods employed by studies identifying maternal characteristics associated with needing pharmacological management of GDM, have used conventional regression analysis, which will identify confounding variables by testing for statistically significant relationships between them. This methodology may therefore misinterpret the relationship between variables by failing to identify all confounders or including bias - an incorrect interpretation of a relationship. Consequently, conventional regression analysis can only conclude associational, rather than causal, relationships. Causal Inference (Pearl Citation2009) is a statistical technique which utilises domain expertise, often in the form of directed acyclic graphs (DAGs), to draw causal rather than associational conclusions. This method is increasingly being used to handle observational data (Tennant et al. Citation2021) to identify casual, rather than just associational, conclusions.
The aim of this study was therefore to investigate if any causal relationships exist between maternal characteristics, including hemodynamics, and the need for pharmacological management of GDM. The null hypothesis was that there would be no causal relationships between the variables and treatment requirement.
Methods
We conducted a study of maternal haemodynamics in women with GDM commenced on dietary management, and compared women who remained on dietary intervention only with those who went on to require pharmacological management. Ethical approval was obtained from the East Midlands Research Ethics Committee (15/EM/0469, IRAS 182250) and the University Hospitals of Leicester (UHL) Research and Innovation Department prior to commencement. The study was conducted in accordance with the principles of Good Clinical Practice, and the Declaration of Helsinki (World Medical Association Citation2013). All women provided written consent to take part. The Strengthening the Reporting of Observational Studies in Epidemiology (STROBE) statement according to the Enhancing the Quality and Transparency of Health Research (EQUATOR) guidelines (Von Elm et al. Citation2007) were used in the production of the manuscript.
2.1. Study population
Participants for this study were identified as an opportunistic sample from women recruited to a larger study of longitudinal maternal haemodynamics conducted at UHL NHS Trust in the United Kingdom. The current study presents results not considered in our previous report of this project [Anness et al. Citation2023]. The previous report includes a full description of the eligibility criteria for inclusion. In short, women with GDM were eligible for inclusion if they had a viable singleton pregnancy without known foetal aneuploidy or anomaly. In contrast to the inclusion criteria for the previous study, where women were required to undergo serial assessments of their haemodynamics, women were eligible for inclusion in the current study if they underwent at least one assessment of their haemodynamics, whilst on dietary management for GDM, between 20+0 and 33+6 weeks gestation. All participants were followed up until delivery to establish whether they remained on dietary intervention throughout the pregnancy, or if they required additional pharmacological management, as well as their pregnancy outcomes.
The screening protocol for GDM at the UHL is adapted from UK national guidance (National Institute for Health and Care Excellence, Citation2015) and is presented in Table S1. GDM was defined as a fasting glucose of ≥5.6 mmol/L, and/or a serum glucose level of ≥7.8 mmol/L 2 hours after a 75 g oral glucose load. Women were started on a dietary intervention plan within 2 weeks of diagnosis. Adequate glucose control was defined as a fasting glucose of <5.3 mmol/L and 1 hour post prandial glucose levels of <7.8 mmol/L (National Institute for Health and Care Excellence, Citation2015). Women in whom 3 consecutive blood glucose readings (either all within one day, or across three days at the same testing time) remained above these targets after completing 2 weeks of dietary management, or who did not maintain their blood glucose levels within these limits, were commenced on metformin or insulin therapy, at the discretion of the consulting physician.
2.2. Study measurements
We collected demographic data including maternal age, ethnicity, height, parity, and BMI at booking, as well as fasting and post prandial glucose levels at diagnosis of GDM. Gestational age at diagnosis and at haemodynamic assessment was calculated based on the dating scan performed between 11+0 and 13+6 weeks gestation.
Maternal measurements of heart rate (HR), stroke volume (SV), CO, MAP, TPR, AoAIx, BrAIx, and pulse wave velocity (PWV) were assessed using the Arteriograph® (TensioMed Ltd, Budapest, Hungary) and a non-invasive bioreactance method (NICOM®, Cheetah Medical, Portland, Oregon, USA), using methodology described in previous descriptions of the study (Anness et al. Citation2023).
2.3. Statistical and Causal analysis
Baseline data and unadjusted values for each of the haemodynamic variables were first compared using parametric and non-parametric tests performed using Stata (Version 15.0, StataCorp LLC, College Station, TX, USA). Results were considered statistically significant if p < 0.05.
Causal analysis was then performed using a graphical causal inference approach. Conventional logistic regression utilises an adjustment set identified through mathematically significant relationships between the variables. It is therefore unable to conclude causality as this process is subject to Type I and Type II errors, and it does not account for systematic biases. These occur when confounding variables are not identified, causing an overestimation of the relevance of the relationship between the variables of interest, and when a variable is falsely identified as a confounder, which may cause an underestimate of the relationship.
The graphical causal inference method aims to eliminate this potential for misinterpretation of the relationship between two variables, by using DAGs to visually represent the relationships between the considered variables (nodes) and the direction of known relationships between them (edges). Parent nodes are those at the start of an arrow, and child nodes are those are the end. Rather than identifying the adjustment set for use in logistic regression analysis mathematically, this method of casual analysis identifies the adjustment set from the graphical model.
Directed paths, in which all arrows point in the same direction, represent potential causal pathways. Open, or backdoor, pathways in which two or more child nodes share the same parent node represent relationships that are non-casual, where the two child nodes are associated with each other, and the effect mediated by the parent node. The variable identified as the parent node is a confounder which need to be controlled for in the logistic regression analysis. Closed, or blocked, pathways in which two or more parent nodes have the same child node, also represent a non-causal relationship, but there is no association between the two parent nodes via the child node. Controlling for the child nodes would introduce bias and create a false interpretation of the relationship, and so they should be excluded from the adjustment set.
In this way, the adjustment set selected from a DAG for the regression analysis mitigates all sources of confounding and bias by identifying necessary confounders and omitting unnecessary ones, allowing the analysis to identify relationships which are causal in nature. We used the R package Dagitty (Textor et al. Citation2016) to electronically select a suitable adjustment set for each relationship of interest from our casual DAG. Due to the difficulty in adjusting a small data set for categorical variables, we converted ethnicity into a binary value defined as White or Non-White ethnicity. We also used the smallest adjustment identified by Daggity for each variable. We then used logistic regression models (Sharma & DoWhy Citation2020) to investigate the relationship between each maternal characteristic or haemodynamic measurement and the need for pharmacological management of GDM. Women with missing data for a particular variable were excluded from the logistic regression analysis involving that particular variable. To quantify the size of the effect of the variable on the need to initiate pharmacological treatment, we converted the logistic regression coefficients into an odds ratio using the following equation: Odds Ratio = elogistic regression coefficient. Logistic regression coefficients and odds ratios were considered statistically significant if the 95% confidence interval did not include zero, or 1.0, respectively.
Results
The recruitment and inclusion processes are shown in . A total of 120 women with GDM were included in the analysis. Data regarding BrAIx, AoAIx, and PWV were missing for 4 women, and regarding CO and TPR for 7 women. After assessment, 62 women remained on dietary intervention alone, and 58 required pharmacological management. Of these 58 women, 44 were treated with metformin alone, 2 with insulin alone, and 12 with metformin and insulin in combination.
3.1. Baseline characteristics and pregnancy outcomes of the study groups
Baseline characteristics of the women in each group are described in . Compared to women who remained on dietary intervention alone, those who required pharmacological management were significantly heavier (p = 0.004) and had higher BMI at booking (p = 0.003), but there was no significant difference in maternal age, height, parity, or ethnicity between the two groups. Fasting oral glucose tolerance test (OGTT) results were significantly higher in women who went on to require pharmacological management (p < 0.001) but there was no significant difference in 2-hour OGTT glucose levels or in gestation at diagnosis. None of the women had chronic hypertension and there was no difference in the rates of gestational hypertensive disorders between the two groups.
Table 1. Baseline demographic details, maternal haemodynamics, and pregnancy outcomes of the study groups. Data presented as mean + standard deviation or n (percentage).
3.2. Haemodynamic measurements
Unadjusted mean haemodynamic variables for each group are described in . On comparison of the raw values, mean maternal heart rate (87.6 ± 11.7 vs. 92.9 ± 11.90 bpm, p = 0.014) and aortic PWV (7.8 ± 1.04 vs. 8.4 ± 1.61 m/s, p = 0.029) were significantly higher amongst women who required pharmacological management compared to those managed on dietary intervention alone but there was no significant difference between the two groups in maternal CO, SV, TPR, MAP, BrAIx or AoAIx.
3.3. Causal inference analysis
The table of evidence used to construct the DAG is shown in Table S1. The DAG, showing the variables (nodes) of interest and the relationships between them (edges), is shown in . Variables included in the DAG were maternal age, height, weight, and BMI at booking; parity; ethnicity; history of GDM; gestational age at diagnosis and at haemodynamics assessment; fasting and 2 hour OGTT result; and haemodynamic measures, including: CO, HR, TPR, MAP, Brachial Augmentation Index (BrAIx), Aortic augmentation index (AoAIx) and Pulse Wave Velocity (PWV). The adjustment set identified by the Daggity program for each variable is provided in the Supplementary Material.
Figure 2. Causal directed acyclic graph (DAG). A directed acyclic graph (DAG) representing the causal relationships amongst demographics, haemodynamic variables, need for pharmacological treatment of GDM. Boxes represent variables of interest (nodes) and arrows (edges) represent the direction of known relationships between these variables. The dotted lines represent relationships we wanted to investigate.
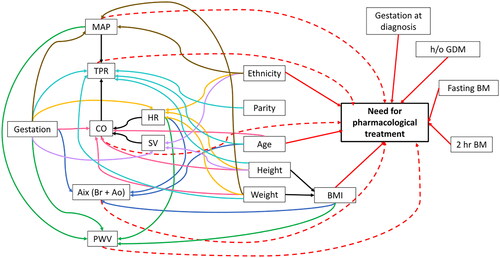
Regression coefficients for the effect of each haemodynamic variable on the need for pharmacological management are shown in . Amongst the demographic variables, maternal BMI at booking significantly influenced the need for pharmacological treatment of GDM, although interestingly, the effect was significant only amongst the women of non-White ethnicity. The size of the effect was relatively small, with each unit increase in BMI increasing the odds of needing metformin and/or insulin therapy by 20% [OR 1.20 (1.03 − 1.39)]. There was no significant causal relationship between fasting glucose, 2-hour OGTT result or any of the haemodynamic variables and requirement of pharmacological treatment ().
Table 2. Effect of maternal characteristics, diagnostic factors, and haemodynamic variables on need for pharmacological management. Logistic regression coefficients were converted into the odds ratio using the following equation: (Odds Ratio = elogistic regression coefficient) to quantify the effect size.
Secondary regression analysis using a smaller DAG, demonstrated in Figure S2, was carried out to explore how a different causal structure with a smaller variable set would affect the results. The adjustment set identified by the Daggity program for each variable on this DAG is also provided in the Supplementary Material. Results of this secondary analysis are demonstrated in . The causal influence of maternal BMI on the need for pharmacological treatment remained significant, with each unit increase in BMI increasing the odds of needing metformin and/or insulin therapy by 12% [OR 1.12 (1.02 − 1.22)]. This secondary analysis did not alter the findings for the maternal haemodynamic variables, as none of the relationships between the haemodynamic measurements and the need to initiate pharmacological management reached statistical significance.
Table 3. Effect of maternal characteristics, diagnostic factors, and haemodynamic variables on need for pharmacological management (based on secondary analysis DAG shown in Figure S1) Logistic regression coefficients were converted into the odds ratio using the following equation: (Odds Ratio = elogistic regression coefficient) to quantify the effect size.
Discussion
4.1. Summary of main findings
This observational study of maternal characteristics amongst women with GDM who required dietary management alone compared to those requiring pharmacological management is the first to apply a causal inference approach to this topic. We have identified that maternal booking BMI has a causal, rather than merely associational, relationship on the need for pharmacological treatment. To our knowledge this is also the first study to investigate the relationship between maternal haemodynamics and treatment requirement for GDM. Despite both maternal HR and PWV both being significantly higher amongst women requiring pharmacological treatment, the relationships did not remain significant in a causal logistic regression analysis.
4.2. Interpretation of main findings and comparison with the literature
Our findings agree with several previous studies which have identified increasing maternal BMI as an independent risk factor for needing supplemental insulin in addition to dietary management of GDM (Eleftheriades et al. Citation2021; Martine-Edith et al. Citation2021; Pertot et al. Citation2011; Sapienza et al. Citation2010; Wang et al. Citation2021). Our study, however, is the first to employ a causal analysis, meaning that the significant association identified can be interpreted as a causative and not just an associative relationship. Since obesity is associated with insulin resistance through increased fatty acid release and altered adipokine secretion (Westphal Citation2008), this causal relationship is not unexpected.
The results of the regression analysis derived from the larger DAG (Figure S1) found that the causal influence of BMI on the need for pharmacological treatment of GDM was limited to women of non-White ethnicity only, which was unexpected. Maternal BMI has a differential effect on the prevalence of GDM, with GDM occurring at lower BMIs in women of East and South Asian ethnicity compared to other ethnicities (Read et al. Citation2021) and so it is possible that BMI might also have a contrasting effect on the treatment requirements according to ethnicity. Larger studies will be able to assess our findings.
Previous investigators (Eleftheriades et al. Citation2021; Martine-Edith et al. Citation2021; Pertot et al. Citation2011; Wang et al. Citation2021) have also identified higher fasting and 2-hour OGTT results as independent risk factors for requiring pharmacological management of GDM. Although fasting OGTT levels were higher amongst the women in our cohort who required pharmacological treatment, logistic analysis within our statistical model did not identify a causal relationship.
Mansukhani et al. (Citation2022) reported higher PWV in women requiring insulin treatment, compared to diet alone, at 35–37 weeks of gestation. Similarly, when we compared the raw values of aortic PWV between the two groups, mean PWV was significantly higher amongst the women who went on to require pharmacological treatment, compared to those who remained on dietary management. However, when the raw values were entered into the logistic regression analysis, using the adjustment sets identified by the Daggity package, the difference was no longer significant. Our hypothesis that maternal haemodynamics may influence the need for pharmacological management of GDM was therefore not supported.
4.3. Strengths and limitations
Previous studies (Eleftheriades et al. Citation2021; Martine-Edith et al. Citation2021; Pertot et al. Citation2011; Sapienza et al. Citation2010; Wang et al. Citation2021) which have investigated factors associated with the need for pharmacological treatment amongst women with GDM have been able to conclude only association, and not causation. A strength of this study is that we have employed a causal inference analysis, meaning that significant relationships can be interpreted not just as associative, but as causative. By using BMI as a continuous variable, we have also been able to quantify the increase in treatment requirement caused by each unit increase in maternal BMI.
However, a limitation of this study is that since this was a sample of convenience, we had small participant numbers in each group and we were therefore unable to perform a sub-group analysis to investigate if requiring metformin alone versus requiring combination metformin with insulin therapy made any difference to the results. We also assessed women after initiation of dietary management, and so further work in this area may wish to assess women at diagnosis, prior to the initiation of any intervention.
4.4. Clinical and research implications
Our findings of a causative relationship between maternal BMI and requiring pharmacological management of GDM highlight the importance of pre- and peri-conception weight control. Meta-analyses have shown that physical activity interventions reduce the risk of developing GDM (Russo et al. Citation2015) and improve blood glucose levels in women with established GDM (Li et al. Citation2022), although neither study has commented on whether these outcomes were associated with reduced gestational weight gain. We are not aware of any interventions which have reported on gestational outcomes following pre-pregnancy lifestyle interventions, but the results of the forthcoming INTER-ACT study (Bogaerts et al. Citation2017) may be able to shed light on this.
Whilst the benefits of earlier, rather than delayed, achievement of good glycaemic control in the management of GDM have not been investigated, earlier screening and initiation of treatment for GDM has been associated with an improved primary composite outcome of emergency caesarean section, neonatal hypoglycaemia and macrosomia (Ryan et al. Citation2018). Enabling earlier identification of women with GDM likely to fail to achieve euglycaemia with dietary intervention alone may allow earlier initiation of pharmacological treatment and therefore improve maternal and foetal outcomes. Our results do not currently suggest a role for maternal haemodynamics in the prediction of women who are likely to require pharmacological management of GDM.
4.5. Conclusion
In this study, we have identified a causal relationship between maternal BMI at booking and the need for pharmacological treatment of GDM. No significant causal relationships were found between maternal haemodynamics and the need for pharmacological treatment.
Supplemental Material
Download MS Word (22.6 KB)Acknowledgements
We are grateful to Baxter Healthcare, United States, for the contribution of the double electrode stickers for use with the NICOM® system. TGR is a NIHR Senior Investigator. NW and MF are supported by the EPSRC CITCOM project (EP/T030526/1).
Data availability statement
The data that support the findings of this study are available from the corresponding author, [AA], upon reasonable request.
Additional information
Funding
References
- Anness, A.R., et al., 2022. Arterial stiffness throughout pregnancy: arteriograph device-specific reference ranges based on a low-risk population. Journal of Hypertension, 40 (5), 870–877.
- Anness, A.R., et al., 2023. Does treatment modality affect measures of arterial stiffness in women with gestational diabetes? Ultrasound in Obstetrics & Gynecology: The Official Journal of the International Society of Ultrasound in Obstetrics and Gynecology, 62 (3), 422–429.
- Anzoategui, S., et al., 2022. Midgestation cardiovascular phenotype in women who develop gestational diabetes and hypertensive disorders of pregnancy: comparative study. Ultrasound in Obstetrics & Gynecology: The Official Journal of the International Society of Ultrasound in Obstetrics and Gynecology, 60 (2), 207–214.
- Bogaerts, A., et al., 2017. INTER-ACT: prevention of pregnancy complications though an e-health driven interpregnancy lifestyle intervention – study protocol of a multicentre randomised controlled trial. BMC Pregnancy and Childbirth, 17 (1), 154.
- Eleftheriades, M., et al., 2021. Prediction of insulin treatment in women with gestational diabetes mellitus. Nutrition & Diabetes, 11 (1), 30.
- Guy, G.P., et al., 2017. Maternal cardiovascular function at 35-37 weeks’ gestation: relation to maternal characteristics. Ultrasound in Obstetrics & Gynecology: The Official Journal of the International Society of Ultrasound in Obstetrics and Gynecology, 49 (1), 39–45.
- Kim, J.A., et al., 2006. Reciprocal relationships between insulin resistance and endothelial dysfunction: molecular and pathophysiological mechanisms. Circulation, 113 (15), 1888–1904.
- Kintiraki, E., et al., 2018. Blunted cerebral oxygenation during exercise in women with gestational diabetes mellitus: associations with macrovascular function and cardiovascular risk factors. Metabolism: Clinical and Experimental, 83, 25–30.
- Li, X., et al., 2022. Exercise intervention improves blood glucose levels and adverse pregnancy outcomes in GDM patients: a meta-analysis. Computational and Mathematical Methods in Medicine, 2022, 9287737. Online ahead of print.
- Ling, H.Z., et al., 2021. Effect of maternal age on cardiac adaptation in pregnancy. Ultrasound in Obstetrics & Gynecology: The Official Journal of the International Society of Ultrasound in Obstetrics and Gynecology, 58 (2), 285–292.
- Mansukhani, T., et al., 2022. Vascular phenotype at 35-37 weeks’ gestation in women with gestational diabetes mellitus. Ultrasound in Obstetrics & Gynecology: The Official Journal of the International Society of Ultrasound in Obstetrics and Gynecology, 61 (3), 386–391.
- Martine-Edith, G., et al., 2021. Associations between maternal characteristics and pharmaceutical treatment of gestational diabetes: an analysis of the UK Born in Bradford (BiB) cohort study. BMJ Open, 11 (11), e053753.
- Meah, V.L., et al., 2016. Cardiac output and related haemodynamics during pregnancy: a series of meta-analyses. Heart (British Cardiac Society), 102 (7), 518–526.
- Mecacci, F., et al., 2022. Maternal hemodynamic chances in gestational diabetes: a prospective case-control study. Archives of Gynecology and Obstetrics, 306 (2), 357–363.
- National Institute for Health and Care Excellence 2015. Diabetes in pregnancy: management from preconception to the postnatal period.
- Osman, M.W., et al., 2018. Haemodynamic differences amongst women who were screened for gestational diabetes in comparison to healthy controls. Pregnancy Hypertension, 14, 23–28.
- Pearl, J., 2009. Causality. (2nd ed.). New York: Cambridge University Press.
- Perry, H., et al., 2019. Influence of maternal characteristics and gestational age on hemodynamic indices: NICOM device-specific reference ranges. Ultrasound in Obstetrics & Gynecology: The Official Journal of the International Society of Ultrasound in Obstetrics and Gynecology, 54 (5), 670–675.
- Pertot, T., et al., 2011. Can common clinical parameters be used to identify patients who will need insulin treatment in gestational diabetes mellitus? Diabetes Care, 34 (10), 2214–2216.
- Petry, C.J., et al., 2019. Temporal trends without seasonal effects on gestational diabetes incidence relate to reductions in indices of insulin secretion: the Cambridge baby growth study. Acta Diabetologica, 56 (10), 1133–1140.
- Read, S.H., et al., 2021. BMI and the risk of gestational diabetes among women of South Asian and Chinese ethnicity: a population-based study. Diabetologia, 64 (4), 805–813.
- Russo, L.M., et al., 2015. Physical activity interventions in pregnancy and risk of gestational diabetes mellitus: a systematic review and meta-analysis. Obstetrics and Gynecology, 125 (3), 576–582.
- Ryan, D.K., et al., 2018. Early screening and treatment of gestational diabetes in high-risk women improves maternal and neonatal outcomes: a retrospective clinical audit. Diabetes Research and Clinical Practice, 144, 294–301.
- Sapienza, A.D., et al., 2010. Factors predicting the need for insulin therapy in patients with gestational diabetes mellitus. Diabetes Research and Clinical Practice, 88 (1), 81–86.
- Savvidou, M.D., et al., 2010. Maternal arterial stiffness in pregnancies complicated by gestational and type 2 diabetes mellitus. American Journal of Obstetrics and Gynecology, 203 (3), 274.e1-274–e7.
- Sharma, A., DoWhy, K. E., 2020. An end-to-end library for causal inference. https://arxiv.org/abs/2011.04216. [Online]
- Tennant, P.W.G., et al., 2021. Use of directed acyclic graphs (DAGs) to identify confounders in applied health research: review and recommendations. International Journal of Epidemiology, 50 (2), 620–632.
- Tensiomed 2011. Arteriograph Users Manual. https://www.tensiomed.com/devices/arteriograph/ [Accessed + downloaded November 2018
- Textor, J., et al., 2016. Robust causal inference using directed acyclic graphs: the R package ‘dagitty. International Journal of Epidemiology, 45 (6), 1887–1894.
- Von Elm, E., et al., 2007. The strengthening the reporting of observational studies in epidemiology (STROBE) statement: guidelines for reporting observational studies. BMJ (Clinical Research ed.), 335 (7624), 806–808.
- Wang, T., et al., 2021. Identification of significant predictors for the need of insulin therapy and onset of postpartum impaired glucose tolerance in gestational diabetes mellitus patients. Diabetes, Metabolic Syndrome and Obesity: Targets and Therapy, 14, 2609–2617.
- Westphal, S.A., 2008. Obesity, abdominal obesity, and insulin resistance. Clinical Cornerstone, 9 (1), 23–31.
- World Medical Association. 2013. World medical association declaration of Helsinki: ethical principles for medical research involving human subjects. JAMA, 310 (20), 2191–2194.