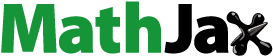
Abstract
Although scholars have developed simulation models and analyzed bankability criteria in numerous papers to enhance the financial outcomes of Public-Private Partnerships (PPPs), the intersection between both fields considering alternatives to assess the financial impact of flexibility in both scope and financing, remains underdeveloped. To address this gap, this paper introduces a financial model that assesses the financial impact of suitable life-cycle flexibility alternatives while coexisting with revenue performance alterations in each project phase within toll road PPPs. This model serves as a tool to gain insight into the planning of strategic actions to implement flexibility in both scope and financing. Based on system dynamics and validated using financial data from two toll road PPPs in India and East Europe, simulation results suggest that two key drivers (capital expenditures and debt repayment period) have the greatest influence on financial performance. Findings support the implementation of flexible scopes to effectively address the most influential exogenous factor impacting the financial performance of PPPs, namely traffic shortfalls. This model provides a suitable tool for assessing the life-cycle economic sustainability of PPPs in uncertain and complex environments.
Introduction
Besides the potential strengths of Public-Private Partnerships (PPPs), the success of this kind of delivery methodology relies significantly on addressing uncertainties across the project’s life-cycle and avoiding the diminishment of the private sector’s expected financial returns (Damnjanovic et al. Citation2016, Nguyen Citation2017). Uncertainties associated with debt-related conditions, construction expenditures, and operation costs in PPP projects can potentially threaten the private sector’s financial expectations and jeopardize projects’ bankability (i.e. lenders’ willingness to finance PPPs after due examination of their related returns and risks) (Nguyen Citation2017). These conditions are particularly relevant for toll road PPPs, as they incorporate traffic demand as an additional uncertainty for revenues throughout their life-cycle (Burke and Demirag Citation2015, Castelblanco et al. Citation2023a). In fact, different PPP toll projects have been met with financial distress since the traffic levels were significantly lower than that forecasted for the projects (Liu et al. Citation2017) and public authorities may offer forms of guarantee to relieve exogenous risks to make the project more attractive for private investors (Brandao and Saraiva Citation2008, Zapata Quimbayo et al. Citation2019).
In this context, the economic sustainability of toll road PPPs relies significantly on the private sector’s financial performance when uncertainties take place (Narbaev Citation2022). For toll road PPPs, private partners must comprehensively understand the financial implications of diverse and complex scenarios to prioritize suitable strategies for optimizing life-cycle revenues and expenditures (Yuan et al. Citation2019, Ortiz-Mendez et al. Citation2023, Castelblanco et al. Citation2023b). To do so, the private sector’s decision-makers must be aware of the relations between sources of payment (e.g. user fees), financing variables (e.g. interest rates and repayment periods), and investment parameters (e.g. capital expenditures and operational expenses), in conjunction with their implications for PPP life-cycle costs (Damnjanovic et al. Citation2016).
The existing literature offers a range of simulation models and bankability criteria to evaluate the financial outcomes of PPPs (Xiong and Zhang Citation2014, Xiong et al. Citation2016, Ullah et al. Citation2018, Bae et al. Citation2019, Shalaby and Hassanein Citation2019, Jin et al. Citation2020). However, there is a critical research gap in understanding the intersection between these models and the identification of strategies to implement flexibility comprehensively.
Most previous financial models in the PPP context have focused primarily on establishing concession parameters before tendering or dealing with renegotiations during the operation phase. This approach neglects the need for a holistic and longitudinal that encompasses all stages of the PPP life-cycle. For example, Ullah et al. (Citation2018) developed a financial model that overlooked important factors such as debt and financial costs, limiting its applicability to determining concession periods before procurement. Similarly, Yuan et al. (Citation2019) concentrated solely on modeling the concession period and public subsidies without considering bankability aspects or the impact of uncertainty effects on construction and operational costs. Other models have predominantly focused on renegotiations during the operation phase (Xiong and Zhang Citation2014, Xiong et al. Citation2016, Bae et al. Citation2019, Shalaby and Hassanein Citation2019, Jin et al. Citation2020).
Financial outcomes of PPP projects are often far from financial modeling estimations, and there have been numerous instances of PPPs failing on a global scale due to the established financial models falling short in covering operational costs and ensuring profitability. Such failures have led to financial losses for private equity investors and, in some cases, have had adverse repercussions for debt financiers, including bondholders and governmental bodies. An illustrative case in point is the State Highway 130 project in Texas, USA, which ultimately declared bankruptcy when the project was unable to guarantee a minimum bankability criteria. Consequently, the federal government was compelled to step in and acquire an equity stake in the new toll road operator, resulting in reduced government exposure to debt and facilitating cash payments. Nonetheless, the repayment status of the federal government’s extended loan remains uncertain (Congress of the United States Citation2020). Another notable example is the South Bay Expressway in San Diego, California, USA, where the private partner filed for bankruptcy (Gifford et al. Citation2023). Subsequently, the bankruptcy court introduced a revised financing and ownership structure to completely redefine the original financial model, incorporating a blend of secured debt and equity, which amounted to only 58% of the initial investment (Jensen Citation2011).
To bridge this gap, this study introduces a novel financial model that evaluates the financial impact of flexibility in scope and financing throughout the different phases of toll road PPPs. By integrating concepts from life-cycle cost assessment (LCCA), system thinking, and project finance, this model quantitatively assesses the potential cost and revenue performance alterations. It aims to offer strategies to implement flexibility in scope and financing in toll road PPPs. The validity and effectiveness of the model are demonstrated through its application to two distinct toll road PPP projects in India and Eastern Europe.
While extant literature has emphasized the impact of exogenous determinants on the financial performance of PPPs and the frequency of renegotiations (Cruz and Sarmento Citation2021), this study takes a step further by assessing the financial life-cycle impact of alterations to these parameters. Previous research has extensively analyzed the relevance of traffic to the financial performance of road PPPs from a general perspective (Flyvbjerg et al. Citation2007, Vassallo et al. Citation2012, Carpintero et al. Citation2013, Cruz et al. Citation2014). In contrast, this paper quantitatively evaluates the effect of demand alterations on PPP financial performance, considering important exogenous parameters such as debt interest rate and CAPEX overruns resulting from external factors.
Overall, the proposed model is a tool for gaining insight into designing real-time strategic actions when facing potential underperformances during each of the life-cycle PPP phases, by decoding multiple interacting feedback processes to self-correct or self-reinforce specific behaviors within complex systems.
The structure of this paper is as follows: Initially, a literature review on project finance and SD models applied to toll road PPPs is presented. The methodology for the construction of the model and the validation process are outlined. Next, the results are analyzed and discussed, with a focus on practical and theoretical implications. Finally, the paper concludes with a summary of findings and suggestions for future research.
Literature review
A comprehensive literature review was conducted to establish the relationship between financial drivers and their corresponding mathematical expressions. This systematic analysis aimed to provide a strong theoretical and mathematical foundation for the Causal Loop Diagram (CLD) and the SD Stock and Flow Diagram (SFD) presented in this study.
As shown in , the search encompassed terms related to PPPs, LCCA, and project finance. The search was focused on the Scopus database, covering the period between 2008 and 2022. Scopus was chosen as it is widely regarded as one of the most comprehensive bibliometric databases for scientific and technical peer-reviewed literature (Lagorio et al., Citation2022). The selected timespan aimed to consider publications after the global financial crisis in 2008, as researchers have emphasized the incorporation of impacts from unprecedented uncertainty on financial drivers during this period (Reis and Sarmento Citation2019). The search strategy resulted in 290 publications. Unrelated research areas were excluded resulting in the removal of 229 publications, leaving 61. Finally, a screening process was conducted by reviewing the titles and abstracts to remove manuscripts that were unrelated to the goal of this research, resulting in a final selection of 40 relevant publications. These included case studies, financial models, and theoretical approaches.
Table 1. Search strategy.
This section is organized into two parts, each addressing a specific aspect of the literature related to toll road PPPs. First, it examines the use of project finance in toll road PPPs, exploring the financial aspects involved, as well as their payment and funding sources. Second, it provides an overview of the financial models commonly employed in PPPs, including LCCA as a tool for evaluating of long-term cost-effectiveness of infrastructure projects.
Toll road PPPs through the project finance lens
Project finance constitutes the financing mechanism established for PPP infrastructure projects worldwide (Yescombe and Farquharson Citation2018, Salazar et al. Citation2024). Under a project finance mechanism, a legally independent project company (i.e. concessionaire), is created to finance the required investment in a single-purpose capital asset (i.e. PPP project) (Higham et al. Citation2017). This project company is financed with equity from sponsoring firms and nonrecourse debt within the concession period (Weber et al. Citation2016, El Kawam et al. Citation2024). Under project finance principles, the investment return relies on PPP revenues to generate cash flow during the concession period (Higuchi Citation2019, Khallaf et al. Citation2024).
Based on the payment mechanisms, there are two main alternative road PPP models, namely, service-fee and usage-based payments. A service-fee road PPP establishes its payment source in the public sector based on the operational availability of the infrastructure during the life-cycle (i.e. availability payments) (Yescombe and Farquharson Citation2018). Conversely, a usage-based payment PPP relies on user payments (e.g. toll roads) (Yescombe and Farquharson Citation2018). The increase in fiscal pressure and public budget constraints worldwide has led to a growth in the toll road as the preferred type of PPPs for developing highways in several developing and developed economies (Carpintero et al. Citation2015).
Toll roads’ financial structure relies on the project’s funding sources (i.e. user and government payments) and financing sources (i.e. equity and debt). Consequently, a financial structure based on project finance principles should be able to assess the cash flows required for guaranteeing CAPEX, OPEX, financial repayments, and equity return at a concessionaire’s rate of return, under pre-established risk allocation conditions (Weber et al. Citation2016, Higham et al. Citation2017, Higuchi Citation2019). Toll road projects require high leverage through long-term debt financing to access a larger pool of funds required for capital-intensive projects (Sanchez Soliño et al., Citation2018). This approach reduces the reliance on substantial equity contributions and results in a lower cost of capital compared to relying solely on equity financing to enhance user fees’ (Yescombe and Farquharson Citation2018).
The financial performance of PPPs is influenced by various factors, including capital structure (De Marco and Mangano Citation2017), unforeseen events (Domingues and Zlatkovic, 2015), traffic flow volumes (Akomea-Frimpong et al. Citation2021), traffic forecasts’ inaccuracies (Villalba-Romero and Liyanage Citation2016), and stakeholder alignment (Verweij and van Meerkerk, 2021). To improve PPP performance, it is crucial to adopt holistic approaches that address the complex interplay of socio-technical systems (Warsen et al., Citation2018). By considering these factors and adopting a comprehensive perspective, decision-makers can enhance the overall financial outcomes of PPP projects.
Financial models and LCCA in PPPs
Financial models are suitable tools for assessing projects’ financial performance considering uncertainty and the stakeholders’ interests (Biziorek et al. Citation2023, Castelblanco and Guevara Citation2024, Ottaviani et al. Citation2024). The need for PPP financial models has increased as the investment in this project delivery strengthened during the last three decades especially after global crises to address unforeseen events (Kumar et al. Citation2021).
Traditionally, PPP financial models have focused on specific project phases, lacking a life-cycle perspective (Mohamad et al., Citation2018). This study addresses this gap by quantitatively analyzing suitable alternatives for rebalancing financial underperformance at any PPP stage, considering bankability and uncertainty. Common financial models concentrate on the feasibility stage, determining the concession period, capital structure, or revenue model (Hanaoka and Palapus Citation2012, Khanzadi et al. Citation2012, Ma et al. Citation2018). Other focus on the operation phase, tackling ex-post renegotiations due to private sector bankruptcy or government-led modifications (Gong and Tian Citation2012, Xiong and Zhang Citation2014). By adopting a comprehensive approach, this research fills the void, enabling decision-makers to effectively manage financial underperformance throughout the entire PPP lifecycle.
For the feasibility stage of PPPs, researchers have proposed financial models to establish an optimal concession period. Several models have been focused on determining optimal concession periods based on approaches such as bargaining game theory (Ma et al. Citation2018), real options (Ma et al. Citation2018), Monte Carlo simulation (Hanaoka and Palapus Citation2012), and fuzzy logic (Khanzadi et al. Citation2012). Nevertheless, these models only establish the optimal concession period rather than providing decision-makers with alternatives to tackle uncertainties once the procurement phase has finished.
For the implementation and operation phases of toll roads, previous financial models have been focused on renegotiations. These models have been focused on assessing renegotiation alternatives in PPPs based on approaches such as net present value (Bae et al. Citation2019, Shalaby and Hassanein Citation2019), time series analysis (Xiong and Zhang Citation2014), game theory (Jin et al. Citation2020), and real options (Xiong and Zhang Citation2016). However, these models are focused only on assessing alternatives by increasing the public subsidies or the concession period, which results in detrimental effects for the public sector or the users.
Research methodology
This study develops a SD model for assessing suitable alternatives for implementing flexibility in scope and financing while considering potential cost and revenue performance alterations under a life-cycle perspective in toll roads. SD is used to expose the concurrent feedback loops that drive the concessionaire’s equity flow throughout its lifecycle. An analysis of the potential concessionaire’s interventions was developed to improve the understanding of the main drivers of flexibility in scope and financing that affect PPPs’ financial performance by conducting counterfactual experiments.
This study developed a five-stage methodology as shown in . A comprehensive literature review focused on project finance allowed for the development of the CLD, the SFD, and the quantitative model equations. Once the formulation of the model was completed, the model was assessed twofold, based on real case study data from India and East Europe. Finally, an analysis of the concessionaire’s interventions was conducted to propose specific actions to address PPPs’ financial underperformance.
Data sources
Once the scientific literature was analyzed, one of the most significant challenges for the data collection was obtaining financial data from real PPP projects. This is not a minor issue given the lack of transparency of financial data of PPP projects evidenced by several academics and practitioners (Agyenim-Boateng et al. Citation2017, Guevara et al. Citation2020). The authors gathered financial data from two real PPP projects, the road to Kolkata NH-34 (Yog and Eppler Citation2017) and a BOT toll road in Eastern Europe (PPIAF Citation2008). These data sets were chosen because they included consistent data regarding the project’s debt, and the concessionaire’s equity cash flow as a reference for the model outputs, so as the complete input parameters required for the model and improving model formulation for including increasing debt tranches, vehicles categories, and the number of tolls, among others. A twofold validation process contributed to strengthening the model’s reliability, considering the different configurations of each project.
The Indian project (Project 1) has a concession period of 25 years including 3 years of the construction phase () (Yog and Eppler Citation2017). This road had an initial overall traffic volume of almost 15,000 vehicles per day distributed in two tolls, each including six categories of vehicles. The CAPEX of US 425 million was financed by 18% with private equity, 32% with public subsidies, and the remaining 50% with loans.
Table 2. Exogeneous parameters.
The Eastern Europe project (Project 2) has a concession period of 50 years including 4 years in the construction phase () (PPIAF Citation2008). This project has a debt structure more complex than the Indian project because the debt is composed of three different tranches each with different interest rates, grace periods, and repayment periods. Conversely, the traffic structure of this project is simpler than the Indian project because there are only two categories of vehicles in one toll, whose initial overall traffic volume is 10,000 vehicles per day. This PPP project does not consider public subsidies; consequently, the CAPEX of US 687.5 million was financed 10% with private equity and the remaining 90% with loans.
Simulation model definition
To propose suitable alternatives for addressing financial underperformance, the financial model proposed in this study employs a simulation approach for revealing the complex relations among the most significant drivers of the concessionaire’s equity flow, based on an LCCA perspective. This approach allows modeling and simulating of this system to obtain scenarios that feed redesign concessionaire’s interventions into PPP programs.
The simulation model examines the financial performance of the toll road considering the benefits, pre-operative costs, depreciated costs, financial, and operation and maintenance (O&M) costs to assess alternatives for re-equilibrate the concessionaire’s equity flow during the PPP life-cycle under potential underperformance scenarios. The LCCA approach allows the concessionaire to assess the financial implications of multiple scenarios to define suitable strategies for optimizing their financial returns. From the perspective of the private sector, the concessionaire’s equity flow can be written mathematically in the following form (Sánchez-Silva and Klutke Citation2016):
(1)
(1)
EquationEq. (1)(1)
(1) defines the concessionaire’s equity flow objective function as a function of the benefits, costs, and the internal rate of return during the concession life-cycle. The term
is equivalent to all the benefits expected from the existence of the project. The term
indicates the CAPEX (i.e. planning, designing, and construction) and
is equivalent to all the financial and OPEX costs. The vector parameters
represent the decision variables,
is equivalent to the project lifetime (i.e. concession period), and IRR the concessionaire’s indicates the internal rate of return.
From the perspective of the concessionaire, the decision objective is to reach a non-negative Z(p) at the end of the concession period. Moreover, to calculate the benefits expected [B(p)], the CAPEX [Co(p)], and the Financial & OPEX costs [CL(p)], the model comprehends more than 120 equations.
The financial model relies on several exogenous parameters including general, toll, traffic, CAPEX, debt, and OPEX parameters. The main parameters for each of the two projects employed for the validation are shown in . Detailed information from each of the projects was collected from the World Bank and Harvard Business School (Toolkit for Public-Private Partnerships in Highways 2008, Yog and Eppler Citation2017).
CLD
The CLD () was developed through the use of arrows as links that represent the influence of one variable on another (Sterman Citation2000). The polarity in each causal link shows whether the relationship between the variables connected varies in the same direction (i.e. positive polarity) or behaves in contrary ways (i.e. negative polarity) (Biziorek et al. Citation2023). Moreover, variables can be linked to form closed loops, indicating feedback structures that may be either reinforcing (R) or balancing (B) (Sterman Citation2000).
CLDs are suitable tools for depicting the underlying structure of influencing elements through feedback loops and improving the understanding of the behavior of the systems. To create the CLD, the causal interactions between the drivers were examined in the literature review conducted.
The CLD depicts one reinforcing loop (R) and four balancing loops (B) explaining the main suitable alternatives for adjusting financial gaps under uncertainty. Each of them is focused on specific project phases in the PPP life-cycle. B1 is focused on the construction phase where the financial performance of the concessionaire will be reflected in the CAPEX, which implies that the concessionaire will increase its efforts to achieve efficiency in the construction activities, under Equity Discrepancy aiming for compensating and avoiding cost overruns. B3 is focused on the operation phase, therefore, if there is an Equity Discrepancy the concessionaire will acknowledge an increasing Need for OPEX reduction, which can be achieved through increased efficiencies and by taking advantage of innovative maintenance strategies.
B2 and B4 are focused on the financing conditions (i.e. Repayment Period and Interest Rate) negotiated by the concessionaire in the shaping phase, which in some cases can be renegotiated once the construction phase has been accomplished and the risk is lower if the project is under financial stress. Therefore, these balancing loops show that when there is underperformance in the Free Cash Flow to Equity leads to a higher Equity Discrepancy, which will trigger an increase in the efforts invested by the concessionaire in renegotiation (Cruz and Marques Citation2013). In B2 the Concessionaire’s Effort for Debt Renegotiation leads to an increase in the Repayment Period (Demirag et al. Citation2015), which aims to alleviate the pressure on the cash flow during the initial operation years. Alternatively, in B4 the Concessionaire’s Effort for Debt Renegotiation may result in a lower Interest Rate (Yescombe and Farquharson Citation2018), which reduces Debt Service. In either case, the yearly Financial Cost and Taxes drop, and, consequently; the yearly Free Cash Flow to Equity escalates. Extant literature establishes that when a PPP is under a significant financial underperformance (i.e. high Net Equity Discrepancy), the concessionaire invests increasing efforts in renegotiating debt conditions to reduce Interests to be paid during the life cycle (Demirag et al. Citation2015). The lenders may be willing to decrease the Interest Rate for PPPs under significant financial stress in B2 to avoid the project’s bankruptcy which may constitute a higher detrimental aftermath (Yescombe and Farquharson Citation2018). Alternatively, under a floating interest rate scheme, projects with no significant financial stress (i.e. low Net Equity Discrepancy) may face an increasing Interest Rate according to updated current-market rates (Zhang Citation2005).
R1 represents the traditional support from the public sector through increasing subsidies to compensate for financial underperformances out of the risk allocated to the private partner. Moreover, R1 depicts the undesirable behavior of renegotiations triggered by CAPEX increases (De Marco and Narbaev Citation2021, Marcellino et al. Citation2023). This kind of renegotiation looks for higher Subsidies to compensate and even, in extreme cases, exceed the CAPEX increases. This undesirable behavior is related to the unbalanced profit pool syndrome, which refers to a PPP profit pool skewed to the construction contract returns in the short term (Ho et al. Citation2015). Given the significant financial leverage in project finance projects, PPP promoters may prioritize short-term construction returns.
Model components
Based on the causal model shown previously, an SFD was developed in Vensim® DSS7.3.5 (). Four main components are nested within the model influencing the Free Cash Flow to Equity according to Equationeq. (1)(1)
(1) . The benefits component increases the Free Cash Flow to Equity according to the payment and financing sources. The CAPEX component depletes the Free Cash Flow to Equity during the construction period according to the planning, designing, and construction costs. The financial costs and taxes component reduces the Free Cash Flow to Equity according to the debt costs (including different debt tranches) and the taxes (including depreciation). The last is the OPEX component that diminishes the Free Cash Flow to Equity according to the fixed and variable operating costs.
Model validation
Despite the complexity of the financial model, it is, like all the models, a simplification of reality. To gain confidence regarding the model’s usefulness, the model was validated based on several confidence tests recommended such as behavior reproduction (accuracy of the model in replicating the real system’s behavior), sensitivity analysis (effect of parameters’ changes on the model’s outputs), integration error (accuracy of the model’s numerical solution), extreme conditions (behavior of the model under extreme inputs), parameter assessment (consistency of parameters with the real system), dimensional consistency (consistent units of the model’s variables), structure assessment (internal consistency of the model’s structure), and boundary adequacy (validity of the model’s boundaries and assumptions) (Sterman Citation2000). The sensitivity analysis is carried out to accurately evaluate the impact of changes on a set of input variables following different statistical distributions. In this way, the robustness of the model is evaluated and, at the same time, the uncertainty issue is considered (Rafew and Raziful, Citation2023). Behavior reproduction was focused on both stocks of the system –Free Cash Flow to Equity and Debt – in nominal terms for testing if the model reproduces the behavior of the real system.
Results
This section highlights the SD model results, focusing on evaluating suitable alternatives for addressing financial underperformance in toll road PPPs by considering potential cost and revenue alterations throughout the project phases. A comprehensive analysis of the SD model was conducted to understand the dynamics of the diffusion process and identify critical driving factors for PPP financial performance, while also assessing the robustness of the model. present a sensitivity analysis, showcasing how output values change when input parameters vary.
To assess the effectiveness of balancing loops identified in the SD model in addressing financial underperformance in realistic scenarios, multiple simulations were performed. Counterfactual experiments were conducted to explore “what-if” scenarios from a life-cycle perspective.
This section is organized as follows: firstly, the baseline scenario is presented, providing a foundation for comparison. Then, the analysis focuses on improvements resulting from proposed interventions by the concessionaire. Following that, underperformance scenarios stemming from exogenous risks are examined. Finally, the impact of intervention implementation is assessed in each underperformance scenario. These findings contribute to understanding the potential strategies for addressing financial underperformance in toll road PPPs.
Baseline scenario
The results of the base run simulation are shown in , which demonstrates that the simulated results (solid lines) follow the historical data (discrete data points). In this scenario, the Debt (green line) increases significantly in the short-term during the construction phase, while in the middle- and long-term decreases until is completely paid once the payment period is finished. Conversely, the Free Cash Flow to Equity shows a short- and middle-term declining trend where the Revenues are lower than the CAPEX, OPEX, and Debt Repayment but a positive trend drives recovery in the Free Cash Flow to Equity in the long-term to reach non-negative values once the concession period finishes.
According to , during the first few years, there was a significant decrease in the Free Cash Flow. This initial behavior is entirely driven by the CAPEX balancing loop (B1 in ). The CAPEX is entirely financed by equity, debt (B2 & B4), and public subsidies (R1). Then, there is a momentary slight improvement in the cash flow in both projects, which is produced by the first revenues derived from tolls but the decreasing trend resumes due to the debt payments. Once the repayment periods finish, the cash flow increases more slowly as time passes because of the attenuation effect of the IRR on the balancing loops B2, B3, and B4 ().
Concessionaire’s interventions through the PPP life-cycle
This study differentiates scenarios, which represent underperformance settings resulting from alterations in exogenous parameters beyond the concessionaire’s control, from improvement interventions that the concessionaire can undertake to mitigate these underperformance issues.
Three concessionaire’s interventions were established in the model (i.e. P1, P2, and P3) to analyze their impact in different phases of the project, considering the loops depicted in . R1, B1, B2, B3, and B4). This helped to establish suitable life-cycle alternatives focused on addressing potential underperformance issues in each of the project phases. The interventions were tested for the whole concession period to assess their impact on the Free Cash Flow to Equity and Debt Service Coverage Ratio (DSCR) -i.e. a measure of the cash available to pay the debt obligations, computed as the ratio between the net operating income and total debt service-. presents the three concessionaire’s interventions under analysis and shows the impact of the concessionaire’s interventions on the Free Cash Flow to Equity and DSCR.
Table 3. Description of concessionaire’s interventions.
P1 is an early proactive intervention resulting from CAPEX reductions in the initial phases of the project. This proactive intervention primarily focuses on advocating the adoption of a modular scope approach. This approach aims to introduce flexibility into PPP projects, mitigating the adverse impacts of traffic shortfalls while simultaneously reducing the initial CAPEX investment. This intervention considers a CAPEX reduction of 20% from the baseline scenario, which is within the range that has been previously considered in the literature on financial models for PPPs (Xu et al. Citation2012). Consequently, this intervention is evidenced during the pre-construction phase resulting in improvements in the life-cycle financial performance through the balancing loop B1 ().
P2 is a reactive intervention driven by renegotiating debt conditions with debt providers once the construction phase ends. In contrast to a proactive approach, a reactive intervention implies that the concessionaire waits until financial underperformance becomes noticeable before taking action by implementing cost savings by renegotiating debt conditions. This reactive intervention only may be considered to address financial underperformance noticed before the operation phase, allowing the concessionaire to balance the Net Equity Discrepancy through B2 (). This intervention is focused on modifying the repayment period by adding 4 years, which is suitable for debt providers considering that the rate of return of the debt will not be affected. Conversely, this model neglects to consider renegotiating the interest rate because of its inconvenience for debt providers and the further negative implications for the future of the debt market for PPPs.
P3 represents a late proactive intervention driven by OPEX reductions resulting from the concessionaire’s strategic decisions during the life-cycle. This is achieved through strategic maintenance planning throughout the project’s life-cycle to optimize costs based on strategies such as adopting a condition-based maintenance approach instead of rigid maintenance schedules through the balancing loop B3 (). This intervention considers an OPEX reduction of 20% from the baseline scenario, which is within the range that has been previously achieved as OPEX savings in multiple PPPs (Reis and Sarmento Citation2019).
According to , the analysis of the interventions implemented over the Baseline scenario demonstrated that all of them produced significant improvements in the DSCR and Free Cash Flow to Equity for Projects 1 and 2. In respect to the DSCR, P2 allowed Project 1 to always exhibit values higher than 1.0 across the repayment period. Similarly, P2 helped Project 2 to increase its outcomes from 0.81 in year 7 to values above 1.0 after year 10. This is important because high DSCR values are required to guarantee both fulfillment of debt obligations and profitability (Damnjanovic et al. Citation2016). On the other hand, in respect to interventions’ impacts on the Free Cash Flow to Equity, there are differences in the long- and short-term for both projects. While OPEX reductions (i.e. represented by P3) produced the lowest positive effect in the long-term; P1 generated the highest positive impact across the early stages. These behaviors show that short-term financial improvements (B1) must be preferred over long-term enhancements (B3), considering that the attenuating effect of the IRR on potential positive alterations of financial outcomes increases as time passes. Overall, P2 constituted the most effective intervention for the improvement of the DSCR in Project 2, while P1 was more effective in Project 1.
Scenarios based on underperformance conditions
Three scenarios were analyzed by considering realistic underperformance issues affecting the Free Cash Flow to Equity, namely: worse debt conditions, CAPEX escalation, and traffic underperformance. For doing so, base-run values of key exogenous parameters were modified to assess their impact on the system outcomes. These outcomes were compared against the baseline scenario of Projects 1 and 2, as shown in and and .
Figure 7. Results baseline vs. scenarios on the free cash flow to equity for project 1 (a) and Project 2 (b).
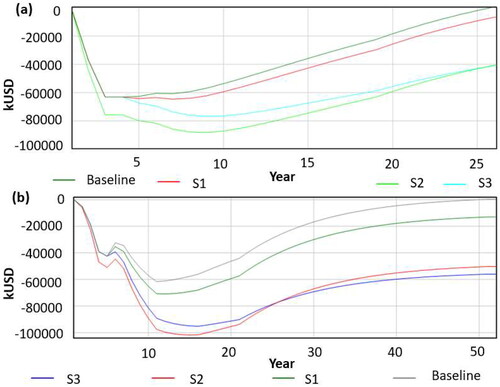
Table 4. Simulation results for the scenarios.
Scenario 1 (S1) was established to understand the impact of underperformance on the debt arrangement according to the intended conditions. The parameter considered for this scenario was the Interest Rate. S1 considers an Interest Rate increase of 1% from the baseline scenario, which keeps the range of this parameter inside the interval of 4% and 12% reported in previous literature (Xu et al. Citation2012).
Scenario 2 (S2) considers CAPEX escalations derived from exogenous risks, which is significantly different from CAPEX escalations taking place due to the concessionaire’s decisions or mistakes. In this sense, this model considers that all the construction risk is allocated to the concessionaire, and, consequently, no contractual renegotiations would be allowed to compensate for this underperformance. This is a suitable assumption in countries such as the UK, China, Colombia, and Chile (Bing et al. Citation2005, Castelblanco et al. Citation2024a, Citation2024b, Citation2024c, Guevara et al. Citation2024, Rojas et al. Citation2023). Accordingly, for this scenario, the CAPEX was increased by 20% from the baseline scenario, which is consistent with CAPEX overruns up to 50% as suggested by Carpintero & Barcham (Citation2012).
Scenario 3 (S3) was established to understand the impact of the variations of real traffic according to the forecasts. Such discrepancies were modeled by modifying the values of Initial Daily Traffic and Traffic Growth. This implies that the traffic risk is completely allocated to the private sector without any traffic guarantees, which is a common traffic risk allocation strategy in countries such as Brazil and Mexico (Carpintero & Petersen Citation2015). Based on that, this scenario assumed an Initial Daily Traffic decrease of 20% from the baseline scenario, which is a value inside the interval of variations between −80% to 50% reported in extant case studies as stated by Alasad & Motawa (Citation2016).
The analysis of the underperformance scenarios demonstrated that S3 constituted the most detrimental effect on the Free Cash Flow to Equity and DSCR for Projects 1 and 2 ( and ). Consequently, the traffic risk (S3) constitutes the most meaningful risk for the financial outcomes respecting the financial and construction risks. However, risks affecting the CAPEX (S2) represented the most significant underperformance for the Free Cash Flow to Equity in the middle term, which potentially may lead the concessionaire to implement reactive interventions during the operation phase. Conversely, worsening in debt conditions (S1) resulted in the least detrimental effect, but still harmful, on the financial outcomes.
Interventions and scenarios through the PPP life-cycle
The impact of the concessionaire’s interventions on the underperformance derived from exogenous events (scenarios) is examined to understand the implications of early proactive, late proactive, and reactive improvements on the life-cycle financial performance, as shown in and and .
Figure 9. Effect of interventions on the free cash flow to equity for: (a) Scenario 1- Project 1. (b) Scenario1- Project 2. (c) Scenario 2- Project 1. (d) Scenario 2- Project 2. (e) Scenario 3- Project 1. (f) Scenario3- Project 2.
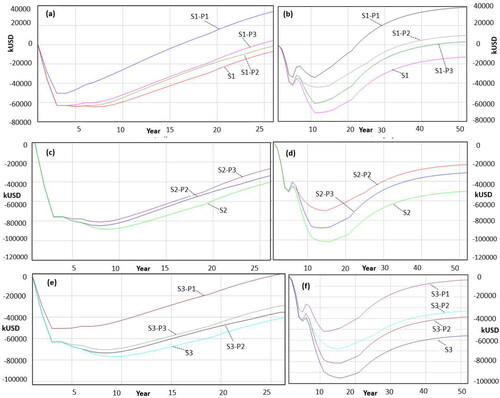
Figure 10. Effect of interventions on the DSCR for: (a) Scenario 1 -– Project 1. (b) Scenario 1 -– Project 2. (c)Scenario2- Project 1. (d) Scenario 2- Project 2. (e) Scenario 3- Project 1. (f) Scenario3- Project 2.
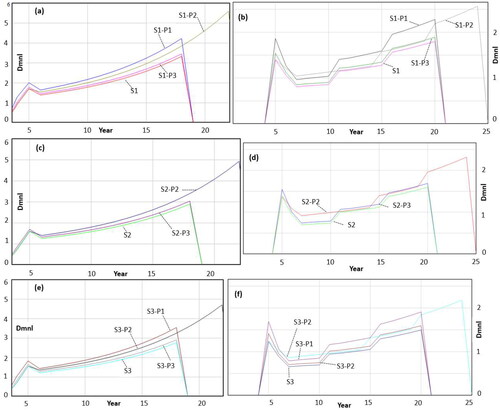
Table 5. Description of concessionaire’s interventions.
The analysis of the interventions implemented over the underperformance scenarios demonstrated that the concessionaire should prioritize early proactive intervention (CAPEX reductions-P1) driven by the balancing loop B1 to mitigate the affectations on its Free Cash Flow to Equity. Implementing an early proactive intervention resulted in the best performance for this outcome for all the scenarios in both projects analyzed ( and ). Consequently, short-term savings allow for a significant improvement of cash available during the construction phase (when it is more required) improving bankability and profitability.
Conversely, the effectiveness of early proactive intervention (CAPEX reductions-P1) for improving the DSCR depends on the level of debt of the project. In this regard, early proactive improvements (B1) only represented the highest positive impact on bankability in low debt-leveraged projects (Project 1 – where the debt constitutes 50% of the CAPEX), as shown in and . Alternatively, high debt-leverage projects (Project 2 – where the debt constitutes 90% of the CAPEX) achieved the most significant bankability improvements based on middle-term reactive interventions (B2-increasing the debt’s repayment period). The dependence of B2 dominance on the level of debt should allow the concessionaire to prioritize reactive actions considering the specific features of the project.
The effectiveness of implementing a late proactive intervention (OPEX reductions-P3) also demonstrated a high dependence on the level of debt of the project for bankability and the concessionaire’s profitability. Consequently, late proactive improvements (B3) represented the worst financial performance regarding the Free Cash Flow to Equity and DSCR for high debt-leveraged projects (Project 2) among all the interventions analyzed, as shown in . In contrast, for low debt-leveraged projects (Project 1) this intervention resulted in a better financial performance than reactive concessionaire’s measures (increasing the debt’s repayment period-P2), which demonstrates that the dominance of B3 also depends on the level of debt.
Discussion
Although the existing literature contains studies focused on improving model forecast accuracy to mitigate uncertainty in long-term PPP projects and their financial performance impacts, the success of these efforts is limited due to the inherent bounded rationality linked with complex interdependencies and rapid external condition changes. Instead of exploring traditional forecasting models, this research focuses on an SD simulation tool aimed at enhancing decision-makers’ comprehension of the system’s intrinsic structure influencing the financial performance of user-pay PPPs. The proposed SD model integrates endogenous causal structures directly affecting project financial outcomes. It incorporates 'what-if’ scenarios based on toll road projects with varying debt levels to quantitatively identify thresholds for specific policies to enhance flexibility within contractual frameworks.
Unlike existing literature, which often examines flexibility solely in terms of scope or financing parameters and is typically confined to specific project phases, results show the coexistence and mutual influence of flexibility in both scope and financing throughout the entire PPP life cycle—encompassing pre-construction, construction, operation, and maintenance phases. Accordingly, the significance of this research lies in addressing a gap in the current literature, where forecast models are restricted by inherent bounded rationality and flexibility has remained relatively unexplored and fragmented when considering user-pay road PPPs.
Elaborating on the concept of flexibility (Martins et al. Citation2014, Sismanidou and Tarradellas Citation2017), the proposed SD model extends its application to toll road PPPs by integrating flexibility into both scope and financing structure. In this way, findings shed light on interventions that the public and private sectors may prioritize to mitigate potential financial underperformance, considering balancing loops tied to traffic, financing costs, CAPEX, and OPEX across the lifecycle phases. Consequently, the SD model allows for the examination of three financial improvement avenues in user-pay PPPs: reactive flexible debt, early CAPEX savings, and early scope flexibility through modularity
Flexibility in debt leverage
Reactive financial improvements (P2 - increasing the debt’s repayment period) constitute the most effective intervention for improving the DSCR for PPPs, especially those with a higher significance of debts in the financial structure. The comparison between the two projects demonstrated that when the significance of debt in a PPP is higher, the impact of flexibility in debt conditions outweighs that of early proactive improvements (scope flexibility), from a bankability perspective.
The relevance of debt flexibility, particularly through extending the Repayment Period, carries significant implications for concessionaires. In instances of financial stress within a PPP project, concessionaires may prioritize renegotiating the debt repayment period as a more feasible option compared to renegotiating interest rates. This intervention’s implications are particularly noteworthy for financing entities, emphasizing that addressing bankability issues in ongoing PPPs lies within the control of banking institutions themselves, without necessitating the renegotiation of interest rates, which is often impractical (Lenferink et al. Citation2014, Yescombe and Farquharson Citation2018). However, it is important to acknowledge potential barriers to implementing this intervention, such as constraints on the Repayment Period for certain types of debt, like bonds. These barriers could potentially be addressed with support from the public sector and multilateral financing entities (Yescombe and Farquharson Citation2018).
Early CAPEX savings
The analysis of concessionaire interventions demonstrates that, from a life-cycle perspective, early proactive financial improvements (B1-reducing CAPEX) should take precedence over late proactive improvements (B3-reducing OPEX). This finding may appear counterintuitive given that the nominal value of Fixed Operation Costs is nearly three times that of CAPEX. However, this behavior arises from the role of the IRR as an attenuating factor in the long-term impact on OPEX (B3), compared to the short-term effect of CAPEX, which amplifies causal loops affecting immediate outcomes (B1). Life-cycle cost theory emphasizes the greater impact of costs during the operational phase compared to the construction phase (Galar et al. Citation2017, Hartwell et al. Citation2019). Conversely, this study demonstrates that due to the high private equity IRR in PPPs, short-term financial performance exhibits a higher incidence of private return compared to long-term financial behavior.
The significance of early proactive financial improvement through CAPEX is highlighted by model demonstrations revealing that CAPEX overruns, common in megaprojects, have a substantial financial impact comparable to that of traffic shortfalls and are even more critical than the effects of higher interest rates. Achieving early proactive CAPEX savings is feasible for the SPV through alternative mechanisms such as promoting competitive procurement for subcontractors (Ahmed et al. Citation2022), optimizing the construction supply chain (Koc and Gurgun Citation2021), and maximizing economies of scale (Sherratt et al. Citation2020). However, these intended proactive behaviors for the construction phase must be carefully tailored due to potential conflicts of interest. Practices such as SPVs becoming construction subcontractors may lead to inappropriate subcontract arrangements and a lack of rigor in construction supervision (Yescombe and Farquharson Citation2018). Sponsors can mitigate these conflicts by involving other sponsors in construction subcontract negotiation and supervision. Additionally, financing entities should enforce preventive measures and thoroughly consider the cost performance of previous PPPs in which Concessionaire’s partners have participated to assess the risk of payment default.
Early scope flexibility through modularity
Scope flexibility through modularity involves the first intervention proposed in the SD model (B1-reducing CAPEX) focused on establishing a minimum upfront scope that can be expanded during the operation phase if actual demand exceeds specific thresholds. While this has been proposed in airport PPPs to optimize infrastructure capacity in response to demand fluctuations, recent literature indicates that its implementation in road PPPs is limited (Martins et al. Citation2014). Specifically, this practice has been mostly reduced to toll-managed lanes overseen by the public sector or to post-PPP expansions, rather than being integrated from the outset within contractual frameworks for interurban toll road PPPs managed by SPVs. For example, the absence of contractual provisions for lane additions in response to traffic changes was noted in the II Coen Tunnel in the Netherlands (Benitez-Avila and Hartmann Citation2023).
Procurement practices for interurban toll road PPPs typically favor inflexible long-term contracts that mandate upfront expansion. This preference often leads to issues such as excessive initial capacity expansion and costly renegotiations (Chung et al. Citation2010, Ullah et al. Citation2018, Acerete et al. Citation2019). Recent case studies have provided evidence of this kind of early renegotiation, particularly occurring between 5 and 10 years after contract commencement (Cruz and Sarmento Citation2021). The detrimental effects of these early alterations in CAPEX on cashflow and bankability, as demonstrated by the interventions and scenarios analyzed by the SD model underscore the necessity for a flexible approach in the scoping and contractual design of PPP projects to enhance long-term sustainability of such projects.
This adaptability is particularly crucial during times of crisis and for greenfield projects where traffic forecasts may be less reliable. To successfully implement this approach, a modular scope must be clearly established in the contractual arrangement, defining specific demand thresholds within certain time intervals during the operational phase. Additionally, it should be noted that the adoption of this approach in toll road projects presents distinct challenges compared to other types of transportation infrastructure, such as airports. While airports can expand capacity by constructing new terminals, toll roads are required to engage in strategic planning from the outset, addressing the full project scope to avoid adverse impacts over the project’s life cycle. For instance, essential tasks like securing land and rights-of-way, conducting tunnel excavation, and constructing primary structural components should be undertaken early to accommodate the project’s maximum potential scope, which may become necessary 15 years into the future based on traffic patterns. Decisions regarding lane expansion should be made based on reaching specific traffic thresholds. Adding extra lanes during the operational phase, while potentially affecting service levels, poses challenges akin to those faced during periods of intensive maintenance.
To effectively address these challenges, it is essential to deploy adaptive strategies that prevent traffic interruptions while prioritizing user safety. Leveraged on the interplay among traffic flow, revenues, and CAPEX demonstrated in the proposed model, lane expansions could be strategically planned for execution during extended off-peak periods. This approach is particularly beneficial for toll roads subject to annual cyclical traffic variations. By scheduling construction during off-peak months, toll road operators can guarantee lane expansion needs while minimizing service disruptions, thereby bolstering the project’s success and sustainability.
Conclusions
The proposed financial model enhances the understanding of the impact of interventions that the concessionaire may prioritize in different project phases and their effects on financial performance. The model reveals that two significant life-cycle drivers for addressing financial underperformance without contract renegotiations are early proactive improvements (CAPEX savings) and middle-term reactive actions (increasing the debt repayment period). CAPEX reductions represent the only intervention capable of effectively addressing the most influential exogenous factor impacting the financial performance of PPPs, which is traffic shortfalls. PPP decision-makers can leverage this finding to promote flexible scopes through the implementation of modularity, allowing for a reduction in CAPEX during the early stages of the project while still ensuring acceptable service levels in the presence of traffic shortfalls or forecast inaccuracies. Conversely, middle-term reactive actions (increasing the debt repayment period) prove to be the most relevant alternative for improving bankability (minimum DSCR) in projects with higher debt leverage.
This paper strengthens the body of knowledge on PPPs in several ways. Firstly, the approach adopted in this study allows for an integrated life-cycle perspective. While various models have been proposed for assessing the financial performance of PPPs, they often focus on specific project phases and lack alternatives for addressing underperformances in each phase of the life-cycle. Secondly, the interventions analyzed from the concessionaire’s perspective enable the development of a LCCA approach, aiming to establish suitable strategies for addressing underperformance based on specific practical interventions, such as modular scope approaches that have been previously proposed for airport PPPs. However, scope modularity has received relatively less attention in toll road PPPs, although there have been some promising initial attempts. Dividing the scope into functional units that can be built at different paces and times based on the evolution of traffic patterns is one possible implementation.
Thirdly, this study proposes increasing the repayment period to reduce bankruptcy risk in stressed PPPs without sacrificing profitability. Although there may be barriers to renegotiating the repayment period for certain types of debt, the support of the public sector and multilateral financing entities can help address these barriers when prioritizing the avoidance of early termination in stressed PPPs.
While this study provides significant findings to concessionaire decision-makers, it also has some limitations derived from the assumptions and scope established. The current financial model focuses on the concessionaire’s perspective within toll road PPPs. Future extensions of this study could assess the public sector’s perspective, considering social, institutional, and environmental benefits such as social welfare, taxes paid by the concessionaire, and reduction of CO2 emissions, among others. Additionally, further research can focus on different categories of PPP infrastructure, such as social infrastructure. Moreover, future studies can incorporate diverse payment mechanisms, such as shadow tolls or availability payments, to compare the implications of non-user-pay PPPs. Lastly, future research should explore the financial ramifications of adopting a flexible scope, including the costs of additional works required in later stages, their effects on service levels during operation, and the potential for reducing initial capital outlays. This analysis will provide valuable insights into optimizing the financial and operational performance of toll road projects.
Disclosure statement
No potential conflict of interest was reported by the authors.
Data availability statement
Data generated or analyzed during the study are available from the corresponding authors by request.
References
- Acerete, B., Gasca, M., and Stafford, A., 2019. Two decades of dbfo roads in the uk and spain: an evaluation of the financial performance. Annals of Public and Cooperative Economics, 90, 269–289.
- Agyenim-Boateng, C., Stafford, A., and Stapleton, P., 2017. The role of structure in manipulating PPP accountability. Accounting, Auditing & Accountability Journal, 30 (1), 119–144.
- Ahmed, M.O., El-adaway, I.H., and Coatney, K.T., 2022. Solving the negative earnings dilemma of multistage bidding in public construction and infrastructure projects: a game theory–based approach. Journal of Management in Engineering, 38 (2), 1–19.
- Akomea-Frimpong, I., Jin, X., and Osei-Kyei, R., 2021. A holistic review of research studies on financial risk management in public–private partnership projects. Engineering, Construction and Architectural Management, 28 (9), 2549–2569.
- Alasad, R., and Motawa, I., 2016. Dynamic demand risk assessment for toll road projects. Construction management and economics, 33 (10), 839. https://doi.org/10.1080/01446193.2016.1143561
- Bae, D.S., Damnjanoic, I., and Kang, D.H., 2019. PPP renegotiation framework based on equivalent NPV constraint in the case of BOT project: incheon airport highway, South Korea. KSCE Journal of Civil Engineering. 23 (4), 1473–1483.
- Benitez-Avila, C., and Hartmann, A., 2023. Managerial agency (re)producing project governance structure and context: Public-private partnerships in the Netherlands. International Journal of Project Management, 41 (4), 102468.
- Bing, L., et al., 2005. The allocation of risk in PPP/PFI construction projects in the UK. International Journal of Project Management, 23 (1), 25–35.
- Biziorek, S., A. De Marco, J. Guevara, and G. Castelblanco. 2023. Enhancing the public investment in public-private partnerships using system dynamics modeling. In: 2023 Winter Simulation Conference, IEEE, 2777–2788.
- Brandao, L.E.T., and Saraiva, E., 2008. The option value of government guarantees in infrastructure projects. Construction Management and Economics, 26, 1171–1180.
- Burke, R., and Demirag, I., 2015. Changing perceptions on PPP games: Demand risk in Irish roads. Critical Perspectives on Accounting, 27, 189–208.
- Carpintero, S., and Barcham, R., 2012. Private toll roads: lessons from Latin America. 2012 International Conference on Traffic and Transportation Engineering (ICTTE 2012).
- Carpintero, S., and Petersen, O.H., 2015. Bundling and unbundling in public–private partnerships: implications for risk sharing in urban transport projects. Project management journal, 46, 35–46. https://doi.org/10.1002/pmj.21508
- Carpintero, S., Vassallo, J.M., and Sánchez, A., 2013. Dealing with traffic risk in latin american toll roads. Journal of Management in Engineering, 31 (2), 1–10.
- Castelblanco, G., et al., 2024a. Combining stakeholder and risk management: Multilayer network analysis for complex megaprojects. Journal of Construction Engineering and Management, 150 (2), 1–12.
- Castelblanco, G., and Guevara, J., 2024. Stakeholder dynamics: rethinking roles and responsibilities in user-pay transport PPP projects. Journal of Construction Engineering and Management,
- Castelblanco, G., Guevara, J., and De Marco, A., 2024b. Crisis management in public-private partnerships: lessons from the global crises in the XXI century. Built Environment Project and Asset Management, 14 (1), 56–73.
- Castelblanco, G., et al., 2023a. Environmental impact assessment effectiveness in public-private partnerships: study on the colombian road program. Journal of Management in Engineering, 39 (2), 19.
- Castelblanco, G., et al., 2024c. Pandemic risks in PPPs: comparative analysis within developing countries. International Journal of Construction Management.
- Castelblanco, G., Safari, P., and De Marco, A., 2023b. Driving factors of concession period in healthcare public private partnerships. Buildings, 13 (10), 2452.
- Chung, D., Hensher, D.A., and Rose, J.M., 2010. Toward the betterment of risk allocation: Investigating risk perceptions of Australian stakeholder groups to public e private-partnership tollroad projects. Research in Transportation Economics, 30 (1), 43–58.
- Congress of the United States 2020. Public-Private Partnerships for Transportation and Water Infrastructure. Congressional Budget Office.
- Cruz, C.O., and Marques, R.C., 2013. Exogenous determinants for renegotiating public infrastructure concessions: evidence from Portugal. Journal of Construction Engineering and Management, 139 (9), 1082–1090.
- Cruz, C.O., Marques, R.C., and Cardoso, P., 2014. Empirical evidence for renegotiation of PPP contracts in the road sector. Journal of Legal Affairs and Dispute Resolution in Engineering and Construction, 7 (2), 05014003. American Society of Civil Engineers (ASCE).
- Cruz, C. O., and Sarmento, J. M., 2021. The Renegotiations of Public Private Partnerships in Transportation.
- Damnjanovic, I.D., Johnson, S.T., and Ford, D.N., 2016. Financial stress testing of toll road projects: the effect of feedback loop dynamics. The Journal of Structured Finance, 21 (4), 51–64.
- Demirag, I., Khadaroo, I., and Stapleton, P., 2015. A changing market for PFI financing: Evidence from the financiers. Account. Forum, 39 (3), 188–200.
- Domingues, S., Zlatkovic, D., and Roumboutsos, A., 2014. Contractual flexibility in transport infrastructure PPP. European Transport Conference 2014.
- Flyvbjerg, B., Holm, M.K.S., and Buhl, S.L., 2007. Inaccuracy in traffic forecasts. Transport Reviews, 26 (1), 1–24.
- Galar, D., Sandborn, P., and Kumar, U., 2017. Maintenance Costs and Life Cycle Cost Analysis. Boca Raton, FL: CRC Press.
- Gifford, J.L., et al., 2023. What triggers public-private partnership (PPP) renegotiations in the United States? Public Management Review, Routledge.
- Gong, L., and Tian, J. 2012. Model for renegotiation in infrastructure projects with government guarantee. In: Proceedings of 2012 5th International Conference, Business Intelligence and Financial Engineering, BIFE 2012. IEEE, 646–648.
- Guevara, J., Garvin, M.J., and Ghaffarzadegan, N., 2020. The forest and the trees: a systems map of governance interdependencies in the shaping phase of road public-private partnerships. Journal of Management in Engineering,
- Guevara, J., et al., 2024. Navigating PPP renegotiations in the Wake of COVID-19: insights from a toll road program. Journal of legal affairs and dispute resolution in engineering and construction, 16 (1), 1–13.
- Hanaoka, S., and Palapus, H.P., 2012. Reasonable concession period for build-operate-transfer road projects in the Philippines. International Journal of Project Management, 30 (8), 938–949. Elsevier LtdAPM and IPMA
- Hartwell, J.C., Upadhyay, Y., and Sourani, A., 2019. Influential factors of life cycle management in education PFI projects. Built Environment Project and Asset Management, 9 (2), 302–314.
- Higham, A., Bridge, C., and Farrell, P., 2017. Project Finance for construction. Routledge.
- Higuchi, T., 2019. Natural Resource and PPP Infrastructure Projects and Project Finance: Business Theories and Taxonomies. Singapore: Springer.
- Ho, S.P., et al., 2015. Opportunism-focused transaction cost analysis of public-private partnerships. Journal of management in engineering, 31 (6), 1–11. https://doi.org/10.1061/(ASCE)ME.1943-5479.0000361
- Jensen, R., 2011. Tollway exits chapter 11. Bond Buy.
- Jin, H., et al., 2020. A game-theoretic approach to developing a concession renegotiation framework for user-pays PPPs. International Journal of Construction Management, 20 (6), 642–652.
- El Kawam, K., et al., 2024. Decoding eastern european national public-private partnership infrastructure programs. Eurasian Studies in Business and Economics,
- Khallaf, R., et al., 2024. A system dynamics model for a national ppp program: the Egyptian project portfolio. Construction Research Congress, 2024, 507–516.
- Khanzadi, M., Nasirzadeh, F., and Alipour, M., 2012. Integrating system dynamics and fuzzy logic modeling to determine concession period in BOT projects. Automation in Construction. 22, 368–376.
- Koc, K., and Gurgun, A.P., 2021. Stakeholder-associated life cycle risks in construction supply chain. Journal of Management in Engineering, 37 (1), 04020107.
- Kumar, A., Srivastava, V., and Tabash, M.I., 2021. Infrastructure project finance: a systematic literature review and directions for future research. Qualitative Research in financial Markets, 13 (3), 295–327.
- Lagorio, A., et al., 2022. A systematic literature review of innovative technologies adopted in logistics management. International journal of logistics research and applications, 25 (7), 1043–1066. https://doi.org/10.1080/13675567.2020.1850661
- Lenferink, S., Tillema, T., and Arts, J., 2014. Lifecycle driven planning of infrastructure: Public and private experiences with more integrated approaches for managing project complexity. European Journal of Transport and Infrastructure Research, 14 (2), 82–101.
- Liu, T., et al., 2017. Sharing the big risk : assessment framework for revenue risk sharing mechanisms in transportation public-private partnerships. Journal of Construction Engineering and Management, 143 (12), 1–12.
- Ma, G., Du, Q., and Wang, K., 2018. A concession period and price determination model for PPP projects: Based on real options and risk allocation. Sustain,
- Marcellino, M., Castelblanco, G., and De Marco, A. 2023. Contract renegotiation in PPPs: evidence from Italy. In: AIP Conference Proceedings, No.2 The Distillery, Glassfields, Avon Street, Bristol, IOP Publishing.
- De Marco, A., and Mangano, G., 2017. Risk factors influencing the debt leverage of project financing initiatives in the energy industry. International Journal of Energy Sector Management, 11 (3), 444–462.
- De Marco, A., and Narbaev, T., 2021. Factors of schedule and cost performance of tunnel construction megaprojects. The Open Civil Engineering Journal, 15 (1), 38–49.
- Martins, J., Cunha, R., and Cruz, C.O., 2014. Maximizing the value for money of PPP arrangements through flexibility: An application to airports. Journal of Air Transport Management, 39, 72–80.
- Mohamad, R., Ismail, S., and Mohd Said, J., 2018. Performance indicators for public private partnership (PPP) projects in Malaysia. Journal of economic and administrative sciences, 34 (2), 137–152. https://doi.org/10.1108/jeas-04-2017-0018
- Narbaev, T., 2022. A meta-analysis of the public-private partnership literature reviews: exploring the identity of the field. International Journal of Strategic Property Management, 26 (4), 318–331.
- Nguyen, D. A., 2017. Improving Public-Private Partnership Contracts through Risk Characterization, Contract Mechanisms, and Flexibility. Blacksburg, Virginia: Virginia Polytechnic Institute and State Univeristy.
- Ortiz-Mendez, L., De Marco, A., and Castelblanco, G., 2023. Building information modeling for risk management: a literature review. In: B. Alareeni, A. Hamdan, R. Khamis, and R. E. Khoury, eds. Digitalisation: Opportunities and Challenges for Business. ICBT 2022. Lecture Notes Networks System, vol 620, 8. Springer.
- Ottaviani, F.M., et al., 2024. Risk perception-based project contingency management framework. Systems, 12 (3), 93.
- PPIAF. 2008. Numerical financial model eastern Europe BOT project. Toolkit Public-Private Partnerships Highw. https://ppiaf.org/sites/ppiaf.org/files/documents/toolkits/highwaystoolkit/6/financial_models/Graphical_model_Operis_v3.xls.
- Rafew, S.M., and Rafizul, I.M., 2023. Application of system dynamics for municipal solid waste to electric energy generation potential of Khulna city in Bangladesh. Energy reports, 9, 4085–4110. https://doi.org/10.1016/j.egyr.2023.02.087
- Reis, R.F., and Sarmento, J.M., 2019. Cutting costs to the bone”’: the Portuguese experience in renegotiating public private partnerships highways during the financial crisis. Transportation, 46 (1), 285–302.
- Rojas, D., et al., 2023. NLP and SNA for understanding renegotiations of toll road PPPs amid the COVID-19 pandemic. In: Proceedings of International Structural Engineering and Construction. Fargo, ND, USA: ISEC Press.
- Salazar, J., Guevara, J., and Castelblanco, G., 2024. Network structures and project complexity in environmental impact assessment outcomes: a Colombian case study. Construction Research Congress, 2024, 127–137.
- Sánchez-Silva, M., and Klutke, G.-A., 2016. Reliability and Life-Cycle Analysis of Deteriorating Systems. H. Pham, ed. Piscataway, USA: Springer.
- Sánchez Soliño, A., Lara Galera, A.L., and Cabero Colín, F., 2018. Valuation of the option of early reversion in road concessions. European journal of transport and infrastructure research, 18 (2), 239–249. https://doi.org/10.18757/ejtir.2018.18.2.3232
- Shalaby, A., and Hassanein, A., 2019. A decision support system (DSS) for facilitating the scenario selection process of the renegotiation of PPP contracts. Engineering, Construction and Architectural Management, 26 (6), 1004–1023.
- Sherratt, F., Sherratt, S., and Ivory, C., 2020. Challenging complacency in construction management research: the case of PPPs. Construction Management and Economics, 0 (0), 1–15. Routledge
- Sismanidou, A., and Tarradellas, J., 2017. Traffic demand forecasting and flexible planning in airport capacity expansions: Lessons from the Madrid-Barajas new terminal area master plan. Case Studies on Transport Policy, 5 (2), 188–199.
- Sterman, J. D., 2000. Business dynamics. S. Isenberg, ed. Irwin Mc Graw Hill.
- Ullah, F., et al., 2018. System dynamics model to determine concession period of PPP infrastructure projects: overarching effects of critical success factors. Journal of legal affairs and dispute resolution in engineering and construction, 10 (4), 1–12.
- Vassallo, J.M., Ortega, A., and Baeza, M. d. Á., 2012. Impact of the economic recession on toll highway concessions in Spain. Journal of Management in Engineering, 28 (4), 398–406. https://doi.org/10.1061/%28ASCE%29ME.1943-5479.0000108.
- Villalba-Romero, F., and Liyanage, C., 2016. Evaluating success in PPP road projects in europe: a comparison of performance measurement approaches. Transportation Research Procedia. 14, 372–381.
- Warsen, R., et al., 2018. What makes public-private partnerships work? Survey research into the outcomes and the quality of cooperation in PPPs. Public management review, 20 (8), 1165–1185. https://doi.org/10.1080/14719037.2018.1428415
- Weber, B., Staub-Bisang, M., and Alfen, H. W., 2016. Infrastructure as an Asset Class: Investment Strategy, Sustainability, Project Finance and PPP. Cornwall, UK: John Wiley & Sons, Ltd.
- Xiong, W., and Zhang, X., 2014. Concession renegotiation models for projects developed through public-private partnerships. Journal of Construction Engineering and Management, 140 (5), 04014008. https://doi.org/10.1061/%28ASCE%29CO.1943-7862.0000843.
- Xiong, W., and Zhang, X., 2016. The real option value of renegotiation in public–private partnerships. Journal of construction engineering and management, 142 (8), 04016021. https://doi.org/10.1061/(asce)co.1943-7862.0001130
- Xiong, W., Zhang, X., and Chen, H., 2016. Early-termination compensation in public–private partnership projects. Journal of Construction Engineering and Management, 142 (4), 04015098.
- Xu, Y., et al., 2012. System Dynamics (SD) -based concession pricing model for PPP highway projects. International Journal of Project Management, 30 (2), 240–251.
- Yescombe, E. R., and Farquharson, E., 2018. Public-Private Partnerships for infrastructure: Principles of policy and finance. Butterworth-Heinemann.
- Yog, S., and B. Eppler. 2017. The road to Kolkata: NH-34 and PPP in India. Harvard Business School, Case 215-007. https://www.hbs.edu/faculty/Pages/item.aspx?num=47660.
- Yuan, J., et al., 2019. Simulation-based dynamic adjustments of prices and subsidies for transportation PPP stakeholders’ satisfaction. Transportation (Amst), 46, 2309–2345.
- Zapata Quimbayo, C.A., Mejía Vega, C.A., and Marques, N.L., 2019. Minimum revenue guarantees valuation in PPP projects under a mean reverting process. Construction Management and Economics, 37 (3), 121–138.
- Zhang, X., 2005. Financial viability analysis and capital structure optimization in privatized public infrastructure projects. Journal of construction engineering and management, 131 (6), 656–668. https://doi.org/10.1061/ASCE0733-93642005131:6656