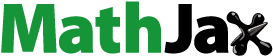
ABSTRACT
The national-scale British Groundwater Model (BGWM) is implemented to simulate groundwater dynamics and budgets in Great Britain. Notwithstanding the challenges of integrating a very large amount of data, finding a trade-off between computational efficiency and realism, performing automatic calibration, and addressing multiple sources of structural and parameter uncertainty, a quantitative–qualitive evaluation approach showed that the BGWM provides a reasonably accurate digital representation of groundwater systems and processes at a national scale. In this work, the model was applied to understand the variability of budget components across multiple spatial and temporal scales. Comparisons showed regional differences linked to lithological and climatic factors, which in turn can be associated with more or less groundwater resilience to extreme climatic events. There is confidence that the current and future versions of the BGWM can become valuable tools for effective water resources management and adaptation strategies under future climatic and population changes.
Editor A. Fiori; Associate Editor (not assigned)
1 Introduction and motivation
Groundwater contained in aquifer systems accounts for the largest volume of liquid freshwater on Earth, providing 50% of the water used in domestic settings and about 25% of all the water withdrawn for agriculture (United Nations Citation2022). Although groundwater is globally abundant (Gleeson et al. Citation2016), natural and anthropogenic factors such as intensification of climatic extremes (Taylor et al. Citation2013), increased demand (Bierkens and Wada Citation2019), and a combination of these (Liu et al. Citation2022) are threatening the resilience of groundwater resources and the sustainability of abstractions in many areas of the globe (Jasechko and Perrone Citation2021, Nair and Indu Citation2021). Effective management strategies are key to avoid overexploitation, depletion, and deterioration of groundwater resources (Famiglietti Citation2014, Zheng and Guo Citation2022, Scanlon et al. Citation2023), which damage freshwater ecosystems (Rohde et al. Citation2017) and cause socio-economic losses (Guzy and Malinowska Citation2020, Jain et al. Citation2021). The implementation of these strategies requires understanding the dynamics of aquifer systems through a quantification of groundwater budgets and their variability across multiple spatial and temporal scales.
Because measuring all budget components directly is not feasible at regional and larger scales, estimations often rely on numerical modelling. At the global scale, models have been applied, for instance, to estimate total amounts of groundwater storage (Gleeson et al. Citation2016), recharge (Reinecke et al. Citation2021) and lateral groundwater flows (de Graaf and Stahl Citation2022) or to assess the impact of groundwater abstractions on global river flows (de Graaf et al. Citation2019). Interactions between surface and groundwater systems (Maxwell et al. Citation2015), groundwater budget components (Alattar et al. Citation2020), coupled surface–subsurface system climatology (Furusho-Percot et al. Citation2019), and groundwater flow in deep basins (Mather et al. Citation2022) have also been modelled at very large or continental scales.
Numerical models have been developed for simulating groundwater processes at the national or large (> 40 000 km2) scale in several countries. For example, an integrated three-dimensional (3-D) multi-layer groundwater-surface water model (Henriksen et al. Citation2003) has been applied to simulate the surface and subsurface hydrology of Denmark (Sechu et al. Citation2022, Seidenfaden et al. Citation2022). In Germany, modelling has been applied to simulate large-scale groundwater flows and understand the impact of droughts (Hellwig et al. Citation2020). Other examples of national-scale groundwater models include France (Vergnes et al. Citation2022), New Zealand (Westerhoff et al. Citation2018), China (Lancia et al. Citation2022), and the Netherlands (Delsman et al. Citation2023).
In England and Wales, the application of groundwater modelling started in the 1970s to assess groundwater development (see review by Rushton and Skinner Citation2012). The growing importance of models as regulatory and management tools in the 1990s was the driver for the creation of a national modelling framework led by the Environment Agency (EA) with the objectives of developing numerical models covering strategically important aquifers and promoting nationally consistent best modelling practices (Whiteman et al. Citation2012). Several regional models have been developed under this framework, but the total modelled area still covers less than 40% of England. Moreover, because each model was independently developed, there are inconsistencies in the conceptualization and parameterization of neighbouring models. Other numerical models of a number of English aquifers (e.g. Medici and West Citation2022, Streetly Citation2023) have also been developed to study a number of topics including the effects of climate (Jackson et al. Citation2011) and land use changes (Zhang and Hiscock Citation2010), as well as the sustainability of groundwater abstractions (Bianchi et al. Citation2023). A two-dimensional steady-state numerical model simulating the average annual groundwater table across England and Wales was developed very recently (Rahman et al. Citation2023). In Scotland, only a few small-scale models have been developed (Jackson et al. Citation2005, Mansour et al. Citation2012).
This work presents the first integrated groundwater model covering Britain (British Groundwater Model, BGWM) to simulate transient groundwater dynamics and surface water/groundwater interactions at the national scale. The main motivation is to provide a tool for a holistic assessment of groundwater resources in British aquifers and for improving current representations of groundwater flow in existing UK-focussed land surface (Best et al. Citation2011) and water quality models (Bell et al. Citation2021). The BGWM also represents the basis for future developments to be applied to a variety of critical ongoing and planned national-scale hydrogeological studies aimed at: (1) understanding the effect of climate projections on groundwater and budgets for evaluating impact and developing adaptation policies (Hannaford et al. Citation2023); (2) characterizing the temporal and spatial dynamics of groundwater flooding and droughts for effective management and risk assessment (Hughes et al. Citation2011, Ascott et al. Citation2021); (3) evaluating the sustainability of current abstractions under scenarios of climate change and population growth (Rameshwaran et al. Citation2022).
The focus of this paper is to detail the conceptualization and implementation of the first version of the BGWM and to demonstrate its application for the estimation of national- and regional-scale groundwater budgets. Understanding temporal and spatial variability in the budget components can potentially provide unprecedented insight into the sustainable management of British aquifers at the national scale. Emphasis is given to the approach used to represent 3-D geological heterogeneity, which is a challenge for global and large-scale models (Condon et al. Citation2021) and not always included in national-scale models (e.g. Hellwig et al. Citation2020). The approaches used for calibration, uncertainty quantification, and model performance evaluation represent elements of novelty compared to previous national or large-scale groundwater models. In particular, the evaluation of the reliability of large-scale models is not trivial given the high degree of structural and parameter uncertainty. The generally coarse resolution also introduces errors when simulated values are compared to point observations. To overcome these difficulties, model performance should be assessed not just with comparisons with hard observations, but also with previous hydrogeological conceptual understanding (Gleeson et al. Citation2021). This mixed quantitative–qualitative evaluation approach was applied to the BGWM to provide a comprehensive evaluation of the model accuracy and highlight limitations and areas for future upgrades.
2 Modelling approach and data
The BGWM combines a 3-D numerical groundwater flow model with a distributed runoff and potential recharge model to simulate transient groundwater heads and flows. The integrated groundwater model covers Great Britain including the countries of England, Wales, and Scotland (). A parsimonious approach was used for parameter calibration based on the minimization of the residuals between measured and simulated groundwater heads and groundwater discharges to rivers (i.e. baseflow). Details about the construction, calibration, and evaluation of the BGWM are provided in the following sections.
Figure 1. (a) Map of Great Britain showing the domain covered by the BGWM. (b) Geological structure and distribution of the HUs at selected depths.

2.1 Hydrostratigraphic zonation
The landmass of Great Britain is underlain by very heterogeneous bedrock, with some representation of nearly every geological period. Lateral and vertical variations in rock properties created by the long-ranging and complex stratigraphy are further complicated by multiple episodes of tectonic rifting and compression, as well as physical and chemical weathering across geological time.
An extensive database of borehole logs, geological cross sections, geological maps, and seismic data were brought together and used to construct a 3-D geological model of Great Britain extending for up to 3 km into the subsurface (Newell Citation2018). Due to the complexity of the input data and faulted bedrock geology, a semi-automated implicit modelling method was used to generate the 3-D gridded distribution of the main geological units, which were combined into 15 hydrostratigraphic units (HUs) based on their bulk geological properties (lithology, stratigraphy), typical groundwater flow type, and hydrogeological properties (). The 3-D spatial distribution of the HUs up to a depth of 500 m below ground surface provided the geological structure for the zonation-based parameterization of the BGWM. A series of cross-sections illustrating the distribution of the HUs at different depths is shown in .
Table 1. Hydrostratigraphic units simulated in the BGWM.
The first version of the BGWM considers only bedrock HUs, while unconsolidated superficial Quaternary aquifers (HU-1) will be added in future developments. Six HUs represent major aquifers in England and Wales (Allen et al. Citation1997). These include the Chalk (HU-4), the most productive aquifer in Great Britain, supplying about one half of the total licensed groundwater abstractions; the Permo–Triassic sandstones (HU-9), the second most productive aquifer, with one quarter of the licensed abstractions; the Greensand aquifers (HU-5); and three major limestone aquifers (Middle Jurassic limestones HU-8; Magnesian Limestone HU-10; Carboniferous Limestone HU-12). The other HUs represent minor aquifers (Jones et al. Citation2000), such as the Palaeogene deposits of southern England (HU-2), or the Lower Cretaceous/Upper Jurassic sandstones in southeast England (HU-6). The calcareous sandstones and limestones of the Corallian Group (Jurassic) in northeast and central England (HU-7) are of variable importance as aquifers. HU-3 mostly comprises mudstones of Triassic to Palaeogene age with subordinate siltstones and sandstones, which are generally considered not to form productive aquifers, although they are locally exploited for domestic and agricultural use. Carboniferous sedimentary rocks mainly from the Millstone Grit and Coal Measures units are represented in HU-11. Productive strata from these units have been largely exploited by the mining industry in the last century and they are still an important industrial water supply. Minor aquifers consisting of older rocks are represented by HU-13 in Wales; HU-14 in southwest and northwest England, Wales, and southern Scotland; and HU-15 in northern Scotland.
2.2 Spatial and temporal discretization
The governing equation of transient groundwater flow coupling Darcy’s flow and fluid mass conservation was solved with a finite-volume numerical scheme in MODFLOW 6 (Langevin et al. Citation2017). The model domain, which covers an area of 218 424 km2, was discretized using an unstructured quadtree grid consisting of 300 750 active cells and four vertical layers. The grid was generated with different levels of refinements and smoothing in proximity to significant hydrogeological features (i.e. rivers, abstractions, and observation boreholes) resulting in cell sizes of 1 km (64.1% of the total number of cells), 2 km (28.9%), 4 km (6.5%), and 8 km (0.5%). The highest percentages of 1 km cells are in the two shallowest layers (85.1% and 62.4%). Further details about the grid are reported in Table S1 and Fig. S1 in the Supplementary material. The top elevation of grid cells in layer 1 corresponds to the minimum of all values within the cell boundaries obtained from a hydrologically consistent integrated hydrological digital terrain model (IHDTM, Morris et al. Citation1990) at 50 m resolution. Layer thickness is equal to 50 m in the two shallowest layers (layer 1 and 2), 100 m in the layer below these (layer 3), and 300 m in the deepest layer (layer 4). The first two layers represent shallow aquifers where active recharge, vertical and lateral groundwater flows, and surface water/groundwater interactions occur. The third layer represents confined portions or deeper parts of unconfined aquifers containing older groundwater, and layer 4 represents deep aquifers. Values for bottom elevations of the layers were based on data showing that 95% of groundwater abstractions in Great Britain are within 200 m below ground surface, and that the transition between fresh (total dissolved solids < 1625 mg/L) and brackish groundwater occurs at around 500 m below the surface (Bloomfield et al. Citation2020).
Regarding the temporal discretization, the full transient run of the BGWM considers a simulation period of 49 years from January 1970 to December 2018, discretized into 588 monthly stress periods. Each stress period is further divided into 15 time steps to facilitate convergence.
2.3 Hydrogeological parameterization
Transmissivity and storage coefficient data from field tests (Allen et al. Citation1997, Jones et al. Citation2000) indicate remarkably high variability within the Hus, with values ranging over two to four orders of magnitude (Figs S2 and the SI). This variability is mainly due to the control exerted by secondary porosity on groundwater flow in the majority of the HUs, which means borehole hydraulic testing data are highly influenced by local conditions of fracturing and dissolution in carbonate rocks. The available data are also spatially biased, not only towards the most productive aquifers (more than 60% of the entire database refers to HU-4 i.e. Chalk and HU-9 i.e. Permo–Triassic sandstones) but also towards historically productive areas. Given the difficulty in finding representative parameter values from the data and the impossibility of resolving small-scale heterogeneity at the scale of the BGWM, HUs were parameterized with effective uniform values of horizontal and vertical hydraulic conductivity (Kh and Kv), specific yield (Sy), and storage coefficient (S). These values were determined from model calibration, but within the range of hydraulic parameters resulting from pumping test analysis for each HU.
To transfer the 3-D hydrostratigraphic zonation onto the numerical grid, the 3-D geological model was sampled every 25 m along the vertical direction at locations corresponding to the centres of the cells of the numerical grid, and uniform parameter values were assigned to the HUs along each vertical profile. The resulting array of values were then averaged to compute upscaled equivalent values according to the arithmetic mean for the horizontal diagonal components of the hydraulic conductivity tensor (Kxx = Kyy = Kh) and to the harmonic mean for the vertical (Kv) component (Anderson et al. Citation2015). Arithmetic averaging was applied for the storage parameters S and Sy. An exponential relationship with depth similar to that assumed in previous large-scale hydrogeological models (Fan et al. Citation2007) was also applied to the 3-D K field. The e-folding depth (i.e. the depth at which the superficial K values decrease by a factor of e = 2.718) was assumed to be 75 m based on the analysis of packer test data in sandstone aquifers and in the Chalk (Allen et al. Citation1997). The resulting field with calibrated values is shown in .
2.4 Boundary and initial conditions
A head-dependent boundary condition (general-head boundary – GHB) was applied to the grid cells along the coastline to represent coastal groundwater discharge to the sea. The seawater water level was assumed to be constant over time and equal to 0 m above ordnance datum (m aOD). A no-flow boundary condition was applied at the bottom of the modelled domain.
Spatially distributed recharge rates were applied to the top surface of the model grid at the beginning of each stress period. These monthly rates were estimated with a previously developed national-scale potential recharge model at a resolution of 2 km implemented with the code ZOODRM (Mansour and Hughes Citation2004). This recharge model is based on gridded estimates of daily rainfall and potential evaporation for the UK (Hough and Jones Citation1997). A detailed description of the methodology and input data (i.e. rainfall, potential evaporation, land cover, vegetation, and surface topography) can be found in Mansour et al. (Citation2018). The surface runoff coefficients were manually calibrated by comparing simulations to reference runoff values obtained from subtraction of baseflow estimates (Gustard et al. Citation1992) from measured river flows. The ZOODRM recharge and MODFLOW 6 models were coupled offline, using spatially distributed monthly averages of the gridded daily potential recharge datasets.
River/aquifer interactions were simulated with the Streamflow Routing Package (SFR), which applies Darcy’s law to calculate the flow between a river cell and the adjacent aquifer cells and then routes river discharge downstream. The flow is calculated according to the following equation (Prudic et al. Citation2004):
where KRB and BRB are the hydraulic conductivity and thickness of the riverbed sediments, respectively; LR and WR are the river length and width in the cell, respectively; hR is the river stage elevation; and hc is the hydraulic head at the river cell. Negative QSFR values represent groundwater discharging from the aquifer to the river and therefore were considered to represent baseflow to rivers. For the implementation of the SFR package, a river network was extracted from the IHDTM using hydrologic terrain analysis software (TauDEM; Tarboton and Ames Citation2012). Tributaries with Strahler order lower than 6 and baseflow index lower than 0.50 were not included in the final SFR network, which consists of more than 50 000 river reaches (i.e. river channels within a grid cell) representing 477 catchments (Fig. S3). Given the spatial extent and complexity of the river network, assumptions were required to parameterize the river network and EquationEquation (1)(1)
(1) . In particular, the thickness and the hydraulic conductivity of the riverbed were combined into a lumped parameter (
), which was assumed to be different for each of the HUs and considered as a parameter in the calibration of the BGWM. The width of each river segment was calculated with an empirical formula developed for UK catchments (Bell et al. Citation2009), while the lengths of the segments were calculated from the length of the river channels within the grid cell. Given the scale and resolution of the river network once mapped onto the numerical grid, river stage elevations were assumed to be equal to the top elevation of the grid cells in layer 1 and kept constant during the simulation.
Estimates of groundwater abstractions from more than 35 000 boreholes were included in the BGWM (Fig. S4). Average abstraction rates were calculated from annual abstraction data from individual boreholes, and then assigned to grid cells corresponding to borehole locations. Data sources include the relevant environment agencies for England (EA), Scotland (SEPA) and Wales (NRW). Because of the lack of actual abstraction data in some areas as well as data licensing issues, the current version of the BGWM assumes only constant abstraction rates over the simulation period.
A simplified model was developed to estimate initial conditions for the full transient run of the BGWM. This model consists of 12 stress periods forced by averaged monthly recharge values for the period 1970–2018 (Fig. S5). Initial conditions for this model were generated with a steady-state initial stress period considering the long-term average recharge over the 1970–2018 period. This simplified model, which was run with the parameter values resulting from the calibration procedure (see below), was run recursively for several years until dynamic equilibrium, i.e. identical groundwater levels and flows, was attained for a particular month for successive years at various observation points. The head distribution at the end of the recursive simulation was used as the initial condition for the full BGWM transient simulation.
2.5 Observation data, model calibration, and evaluation
Two sets of observations were considered for model calibration and evaluation. The first consists of time series of manually and automatically measured groundwater levels for 253 boreholes sourced from the National Groundwater Level Archive (NGLA). These boreholes, which include data for all the HUs except HU-2, were selected because they were located in zones least affected by significant groundwater abstraction and used to produce a monthly hydrogeological bulletin for the UK (e.g. Sefton et al. Citation2023). Notwithstanding the high quality of these data, coverage varies substantially among the HUs with a significant bias towards major aquifers, especially HU-4 (43% of the observation boreholes) and HU-9 (19%) as shown in Fig. S6. Coverage is particularly scarce in Northern and Southwest England and in Wales and Scotland (HU-14 and HU-15). Observed groundwater levels were compared to simulated values from grid cells corresponding to borehole locations and depths. Given the resolution of the grid, spatial discrepancies in the order of a few hundred metres between the borehole location and the grid centres were inevitable.
The second set of observations was generated from baseflow separation analysis (Gustard et al. Citation1992) applied to daily river flow data downloaded from the National River Flow Archive from 522 gauging stations (Fig. S7). Naturalized discharge values adjusted to net abstractions and discharges upstream were available for only 14 gauging stations. The resulting time series of baseflow estimates were compared to simulated downstream discharge at SFR cells corresponding to the location of the gauges. As with the groundwater level data, some level of spatial discrepancy exists between the actual and simulated locations.
A total of 57 input parameters including Kh, S, Sy, and RRB values for each HU, and a general horizontal to vertical conductivity ratio Kv/Kh, were estimated using a parallel version of the parameter estimation code PEST (Doherty Citation2018). Singular value decomposition (SVD) was used to improve numerical stability and reduce parameter dimensionality (Doherty and Hunt Citation2010). A simplified lower-fidelity model consisting of only 14 stress periods and simulating the average conditions over the 1970–2018 period was developed to be used as the forward model in PEST. This simplified model has significantly faster running time compared to the full transient model (0.5 vs. 11 h) thus allowing non-linear estimation of optimal parameter values with reasonable computational effort. The temporal discretization of the simplified model includes an initial steady-state period considering the 1970–2018 average recharge. This is followed by a transient-state period driven by average recharge values for the month of December over the 1970–2018 period. The purpose of these two initial stress periods is to initialize the model with each parameter update before running 12 additional stress periods, starting from January (1st stress period) and ending in December (12th stress period), driven by monthly averaged recharge data for each month. Updates to the 57 adjustable parameters were calculated using the Levemberg-Marquard algorithm in PEST which minimizes the following objective function ():
where ,
,
, and
are the weights assigned to the following four “observation groups”:
average values of the observed hydraulic heads for the month of January over the 1970–2018 period (
) and corresponding simulated values (
);
differences between subsequent monthly averaged (1970–2018) observed hydraulic heads (
) and corresponding simulated values (
);
average values of the observed baseflow estimates for the month of January over the 1970–2018 period (
) and corresponding simulated values (
);
differences between subsequent monthly averaged (1970–2018) observed baseflows (
) and corresponding simulated values (
).
Values for the weights ,
,
, and
in EquationEquation (2)
(2)
(2) were calculated such that the four observation groups contribute equally to the Φ value resulting from the initial parameter values. For simplicity, weights were assumed to be uniform within each group of observations.
Parameter values resulting from the calibration of the simplified model were evaluated by comparing groundwater levels and baseflows simulated by the full transient BGWM with corresponding monthly observed values obtained through averaging daily time-series to monthly resolution. Several performance metrics were used to quantify model accuracy (see equations in the Appendix).
2.6 Calibration uncertainty
The calibration of groundwater flow models is problematic due to the non-uniqueness in the solution of the history-matching problem. Highly parameterized approaches considering thousands of parameters and regularization have been shown to be successful in mitigating this issue (Doherty and Hunt Citation2010, White Citation2018, Hunt et al. Citation2020). For the first version of the BGWM, given the scale and complexity of the 3-D hydrostratigraphic setting and the uneven distribution of groundwater level observations within the modelled area, a traditional zonation-based parameterization approach was used. With this approach, which is still the most common for large-scale models (e.g. Vergnes et al. Citation2022), only the large-scale hydrostratigraphic zonation was imposed as a form of regularization on the parameter fields.
To explore the uncertainty in the calibration results, a numerical experiment was also conducted to investigate the parameter space with the objective to find alternative solutions to the calibration problem. This experiment consisted of performing more than 190 000 runs of the simplified model used for calibration on a 32-node High-performance computer (HPC) with 512 Central Processing Unit (CPU) cores. Each run considered a quasi-random set of the 57 input parameters generated by sampling (Sobol) uniform distributions with ranges equal to the interquartile ranges of the available field test data (Allen et al. Citation1997, Jones et al. Citation2000; Fig. S2). The 24 sets producing the lowest values of EquationEquation (2)(2)
(2) , and therefore the most accurate models, were then selected as acceptable alternative solutions for full BGWM transient simulations. Notably, only one of these sets produced slightly more accurate results than the optimal parameter set estimated by PEST. The standard deviations of the gridded groundwater heads from an ensemble including these 24 models plus the PEST solution were then calculated and mapped. This numerical experiment, although it does not provide a rigorous quantification of the posterior model prediction uncertainty (e.g. Hunt et al. Citation2021), provides some insights regarding the variability of the model outputs within the ensemble of the best 25 solutions of the calibration problem.
2.7 Calculation of groundwater budgets
National and regional groundwater budgets for each HU were extracted from the MODFLOW cell-by-cell flow file (Harbaugh Citation1990) of the calibrated BGWM. The hydrostratigraphic zonation was used to identify groups of cells belonging to each HU. For the identification, a cell was assigned to a certain HU if this was the most frequent hydrostratigraphic unit within the cell. Inflow components () include recharge from precipitation (namely “recharge”), contribution to groundwater from rivers (“river recharge”), lateral or vertical groundwater inflows (“GW inflow”). Outflows (
) include groundwater discharge to rivers or baseflow (“river discharge”), groundwater discharge to sea along the coastline (“coastal discharge”), lateral or vertical groundwater outflows (“GW outflow”), and groundwater abstraction. The total groundwater budget equation considering n inflow components QIN (units L3/T) and m outflow components QOUT (L3/T) can therefore be defined as:
The change in storage (L3/T) is the difference between the amount of water stored in the cell during the associated stress period (“storage intake”) and the amount drained (“storage release”) and added to the flow. In groundwater modelling, storage release is typically counted as an inflow component whereas storage intake is considered an outflow component, although no actual transfer of water into or out of the groundwater system occurs with either of these processes (Anderson et al. Citation2015, Langevin et al. Citation2017). In an aquifer, water surplus occurs when the sum of the inflows is greater than the outflows and therefore ΔS is positive (i.e. excess of groundwater stored in the aquifer and groundwater levels rising). Conversely, during periods of water deficit the left-hand side of EquationEquation (3)
(3)
(3) becomes negative and so is ΔS (i.e. groundwater released from the aquifer and groundwater levels declining).
To facilitate comparisons, monthly volumetric rates were multiplied by the number of days for each month and then normalized by the area of each HU outcropping at the ground surface or sub-cropping under Quaternary (HU-1) deposits ().
3 Results and discussion
3.1 Observation-based accuracy and calibration uncertainty
Results of the PEST-based calibration of the simplified model are shown in , and estimated parameter values are reported in Table S2. The cumulative distribution of 3084 groundwater head residuals (i.e. the difference between measured and simulated heads) has a mean error (ME) of 1.33 m with 43% and 63% of the values falling within ±5 m and ±10 m intervals, respectively. Overall, there is high linear correlation between simulated and measured heads (R2 = 0.93), while the Root Mean Squared Error (RMSE) (20.21 m, Equation A3 in the Appendix) is in line with the values estimated for other large-scale models (e.g. Lancia et al. Citation2022). The RMSE is influenced by large overestimation errors in excess of 20 m in 6% of the observations and, similarly, by large underestimations in 9% of the observations. The cumulative distribution of 6744 baseflow residuals has an ME of 0.45 m3/s, with 73% of the values falling within a ± 1 m3/s interval. The distribution is slightly skewed towards underestimation, as shown by the difference between the 5th and 95th percentiles of the residuals (−2.3 vs. 4.0 m3/s). The R2 and RMSE values of the simulated baseflows are also in line with other national-scale models (e.g. Vergnes et al. Citation2022).
Figure 3. Calibration results (simplified model): cumulative distributions of monthly averaged bias (left) and scatter plots of measured versus simulated values (right) of groundwater head and river discharge.

Maps of standard deviation of simulated heads from the ensemble of 25 BGWM model realizations () indicate that the areas with the highest uncertainty in the simulated heads and consequently in the calibrated parameters correspond to the zones of HU-12 (Carboniferous Limestone Supergroup), HU-14 (Lower Palaeozoic units), and HU-15 (Precambrian units). For the other HUs, as well as in general, higher uncertainty, up to 10 m in standard deviation, is calculated for grid cells characterized by high topographic relief and slope. Since these cells represent areas where steeper hydraulic gradients induce a high range of variability in the actual groundwater levels within the cell, the estimated standard deviations, which are rather stable between different stress periods, can represent a proxy for the scaling error occurring when simulated heads from a model cell are compared to borehole observations. In lowland and relatively flat areas the standard deviation of heads is in the range of a few centimetres to a few metres, which is consistent with the scaling errors estimated by Henriksen et al. (Citation2003) for the national groundwater model of Denmark, which has relatively flat topography.
Evaluation results of the full transient BGWM simulation are presented in . Cumulative distributions of the residuals and scatterplots of measured versus simulated outputs show that the calibrated parameter values produce satisfactory accuracy for the full transient simulation. The distribution of groundwater head residuals (n = 95 615) is comparable to that of the simplified model, with 64% of the values falling within a ± 10 m error, as well as the values of other performance metrics (RMSE = 19.02 m, R2 = 0.94). For baseflows, the ME is 0.28 m3/s while the mean absolute error (MAE, Equation A2) is 1.28 m3/s. Although the correlation between measured and simulated baseflow is generally strong (R2 = 0.90), the underestimation of higher flows (> 100 m3/s) is evident, highlighting the generally low accuracy of simulated peak baseflows in large catchments.
Figure 5. Evaluation results (full transient model): cumulative distributions of monthly averaged bias (left) and scatter plots of measured versus simulated values (right) of groundwater head (top) and river discharge (bottom).

The accuracy of the full transient BGWM was also evaluated for each observation borehole and gauging station. Plots of simulated versus observed hydrographs for selected boreholes and gauging stations are presented in Fig. S8. Satisfactory values of standard model performance metrics represented in green in were identified from guidelines for hydrological model evaluation (e.g. Moriasi et al. Citation2007) and after consideration of all sources of uncertainty and types of errors. For instance, a possible source of error in the evaluation of the simulated baseflows is the separation method, which in the case of the BGWM is standard practice for UK rivers (Gustard et al. Citation1992), but other methodologies producing different estimates could have been used (e.g. Piggott et al. Citation2005). Values of the percentage bias (PBIAS, Equation A6) show that groundwater heads are reasonably well reproduced in most boreholes and for most HUs (). According to the Nash-Sutcliffe efficiency (NSE, Equation A5), the BGWM is particularly accurate in reproducing groundwater levels in the unconfined Chalk aquifer (HU-4), while for boreholes in other major and minor aquifers the accuracy of the simulated groundwater heads is split between about half of the boreholes having positive NSE and the other half negative (). However, values of the Pearson correlation coefficient (Equation A7) indicate that the model is effective in reproducing observed seasonal and multi-annual groundwater level trends in 68% of the observation boreholes (). The PBIAS values of the simulated baseflows () show satisfactory levels of accuracy in about half of the gauging stations and confirms the model tendency towards underestimation (37% of the stations) rather than overestimation (only 16%). NSE values are positive in 57% of the stations, providing further confirmation of the generally satisfactory accuracy level of the baseflow predictions (). Moderate to high correlation coefficient values are calculated for 95% of stations, indicating that the BGWM can very successfully reproduce the temporal variations in baseflow for the simulated river network ().
3.2 Qualitive assessment of model reliability
Evaluating the performance of large-scale models based only on the reproducibility of the observations is rarely robust due to issues with data availability, spatial bias, and scaling errors (Gleeson et al. Citation2021). This is also the case for the BGWM, which include areas where high-quality groundwater observations are limited, such as Scotland, Wales, northern England, and in general the minor aquifers (Fig. S6). Therefore, the observation-based evaluation of the model outputs was complemented by a qualitive analysis of the reliability of the modelled predictions with respect to general and local conceptual hydrogeological understanding. The following are the key results of this analysis, highlighting current limitations of the model and areas for further development.
The tendency towards underestimation of baseflow data in larger catchments, particularly of peak values during intense flooding events, could be the effect of the resolution of the simulated river network – the network does not include the contribution of small tributaries (Strahler index < 6) – and/or due to the monthly temporal discretization, causing an excessive smoothing of the recharge signal. Anthropogenic modifications of the natural flow not considered in the model, such as surface water abstractions, diversions, or sewage discharges, could also impact the accuracy of the baseflow predictions. In the evaluation of the model performance it is also worth considering that groundwater discharge to rivers is not the only process contributing to baseflow (Gnann et al. Citation2021). Moreover, the contributions from different hydrological processes cannot be distinguished by the separation method used to generate baseflow observations. To improve the representation of surface water–groundwater interactions and address these issues, the simulation of total river flow and the inclusion of river stage dynamics at least for major rivers (e.g. Alattar et al. Citation2020) will be considered in future updates of the BGWM.
Another source of error for the baseflow simulations is likely to be the missing contribution from unconsolidated superficial Quaternary deposits that overlie the bedrock. Over two-thirds of the bedrock surface in Great Britain is covered by a veneer of natural superficial deposits of highly variable lithology, heterogeneity, and thickness (Lee et al. Citation2018), and these deposits can in turn possess highly variable hydraulic properties. However, there are areas where superficial deposits form productive aquifers contributing to baseflow, particularly at low elevations and in major river valleys (Tetzlaff and Soulsby Citation2008, Bloomfield et al. Citation2011). This unit also includes an important minor aquifer in eastern England.
The model assumes groundwater flows effectively across the entire thickness of each HU to a depth of 500 m. Although this assumption is supported by borehole data for the sandstone aquifers such as HU-9 (Medici et al. Citation2017, Bloomfield et al. Citation2020), it may not be valid for limestones (HU-4, HU-6, HU-7, HU-10, and HU-12) due to dissolution or older indurated sediments, or for crystalline and metamorphic rock units (HU-13–HU-15) in which groundwater flow and storage is entirely linked to larger fractures typically more persistent within a few tens of metres below ground surface (O’Dochartaigh et al. Citation2015). This discrepancy between modelled and effective active aquifer thickness has implications for the parameterization of the HUs, potentially causing an overestimation of the groundwater potential and storage.
In the Chalk (HU-4), spatial variability in transmissivity and storage coefficients are traditionally linked to the topography, according to a pattern in which values in the valleys are at least one order of magnitude higher than those in the interfluves (e.g. Price Citation1987). It has also been observed that transmissivity varies non-linearly with saturated depth, with a zone of high values within the range of depth of seasonal fluctuation of the water table and a rapid decline below (Butler et al. Citation2012). Although including this smaller-scale heterogeneity (tens to hundreds of metres) in the BGWM would have improved the accuracy of the simulated outputs (e.g. Rushton et al. Citation1989), it also would have required ad hoc changes in the discretization and parameterization of HU-4, causing unevenness in the resolution of the different HUs and a general bias towards this unit.
In general, all the HUs are heterogeneous at a range of scales smaller than the hydrostratigraphic zonation assumed in the BGWM. For instance, some HUs include a mixture of different lithologies with very different hydrogeological properties. At smaller scales, karstic features and interbedded clay-rich layers can greatly change the hydraulic properties of limestone aquifers (e.g. Medici et al. Citation2019). Likewise, tectonic and sedimentary features have been shown to impact groundwater flow in sandstone aquifers (Medici et al. Citation2016). Resolving at least some of the smaller scale heterogeneities within the HUs would certainly improve the accuracy of the BGWM outputs. Refining the parameterization by introducing higher complexity will be considered in the future developments of the BGWM to improve the reliability and reduce the uncertainty of model predictions. Further comparison with existing regional groundwater flow models, e.g. the EA models in England, could prove beneficial in developing more sophisticated parameter distributions.
Simulated groundwater levels are not accurate in areas of significant past exploitation of groundwater resources because of inaccurate initial model conditions. This is, for instance, the case in the confined Chalk (HU-4) within the London Basin, where measured heads of several metres below sea level are rebuilding from the drawdown induced by intense industrial abstractions in the 19th and 20th centuries. Without considering historical pumping rates and given the current set of boundary and initial conditions, it is not possible to accurately reproduce these largely negative heads, and indeed the simulated values are more in line with the original conditions predating industrial abstraction (i.e. a few metres aOD). The lack of time series of actual groundwater abstraction rates is also a limitation of the current model that affects model performance. The inclusion of more realistic abstraction data in the BGWM will improve the calculation of monthly water budgets in heavily pumped and agricultural land areas (e.g Rameshwaran et al. Citation2022).
The recharge model simulates potential recharge without considering the modification of the signal induced by infiltration through overlying deposits and the unsaturated zone of the HU being recharged. This assumption introduces an error when rainfall infiltration through the vadose zone is slower than the duration of the stress period (i.e. one month). This depends on the depth of the water table and on the speed of infiltration through the unsaturated zone. Across Great Britain, the depth of the water table is generally shallow, averaging about 14 m below ground (McKenzie Citation2015). Deeper values (> 20 m) can be observed in the Chalk hills and generally in the high lands of Wales, southwest and northern England, and Scotland. Cross-correlation analyses between groundwater levels and rainfall (Bloomfield and Marchant Citation2013, Mackay et al. Citation2015, Lafare et al. Citation2021) also showed that the lag in the recharge signal is generally within 1 month or slightly slower in the Chalk (HU-4) and limestones aquifers (HU-7, HU-8, HU-10, HU-12). Conversely it can be significant (1–3 months) in the Permo–Triassic sandstones (HU-9), which can explain some of the errors in the simulated groundwater levels. Including a more realistic representation of the recharge process accounting for the unsaturated zone would potentially increase the accuracy of the BGWM in areas where either the water table is deep or infiltration is slow. Lee (Citation2021) identified the distribution of a simplified conceptual understating for the Quaternary deposits distributed on a 1 km2 grid. The next generation of the model will include these conceptualizations to modify the potential recharge by relating the distribution of the hydraulic properties identified by the mapping. However, a comparative study between recharge models in the Chalk, including a physically based Richard’s equation model, showed that the performances are comparable for monthly outputs and for periods of average precipitation (Ireson and Butler Citation2013).
3.3 Simulated national and regional groundwater budgets
The simulated monthly-averaged national groundwater budget for Great Britain () shows that recharge represents the greatest inflow component, followed by storage release, with river recharge as the third component. Recharge rates vary significantly over the year, with highest values (> 25 mm/month) from October to March, and the lowest (≈ 10 mm/month) in the months of May, June, and July. Water released from storage is significant during the summer months, accounting for about 40% of the total inflow. The contribution of river recharge is generally low (≈ 3 mm/month) and unaffected by seasonality. Among the outflows, river discharge is the major component, being proportionally the highest all year round. In the winter months, the amount of groundwater discharged to rivers is equivalent to about 80% of the recharge, while from April to August, this component exceeds recharge by a minimum of 14% in August and a maximum of 70% in May. The components of coastal discharge and abstractions are generally minor compared to river discharge. The absolute amount of groundwater discharged along the British coastline is higher in winter and autumn. For instance, 9 mm/month, corresponding to approximately 63 × 106 m3/day, of groundwater is discharged to the sea on average in January, while in June the discharge is reduced to a third of this value. However, the maximum percentages relative to recharge (≈ 30%) are estimated for the spring and summer months. As for the inflows, the temporal distribution of the outflows follows a very smooth December to January sinusoidal cycle with minima between May and July. Accordingly, groundwater stored in autumn/winter is released in spring/summer to support almost 50% of the groundwater discharged to rivers.
Figure 7. Modelled seasonal groundwater budgets for Britain and the major aquifers. Flows were normalized by the outcropping area of the HUs. A description of the HUs is presented in .

Monthly water budgets for major () and minor () aquifers indicate notable regional and temporal variability. For the Chalk aquifer, for instance (HU-4), recharge accounts for more than 50% of total inflows only in autumn and winter, while it accounts for less than 10% from June to August. In the summer months, river discharge, abstractions, and the other outflows are sustained mostly by groundwater released from storage (≈47% of the total on average) and lateral groundwater inflows from neighbouring units (≈28%). The amount of water exchanged to and from neighbouring units is particularly relevant for the budgets of other major (HU-5, HU-8, HU-12) and minor (HU-7) aquifers, while in certain HUs (e.g. HU-13) the contributions from different components to the total inflow are roughly equal. Interestingly, the HUs can be roughly classified into two groups according to the more or less evident seasonality in the river discharge signal. The first group includes HUs (HU-2, HU-4, HU-8, HU-12, HU-14, and HU-15) where the minimum rate of groundwater discharge to rivers during summer months is less than half of the maximum rate during the winter months, while the second group is characterized by relatively uniform rates over the year. The balance for the Magnesium limestone (HU-10) is an example of this second group. As expected, storage components are more significant in HUs characterized by a higher intragranular flow regime and storage capacity, such as sandstones (HU-5, HU-6, HU-11, HU-13). For instance, the budget of Permo–Triassic sandstones (HU-9) indicates that more than 25% of the average total inflows estimated for the months of November, December, and January are stored in the porous matrix. The balances for HU-14 and HU-15 are rather similar and show the predominance of high rates of recharge among inflow components and of river and coastal discharges, equivalent to more than 50% of the total recharge, among the outflows.
Figure 8. Modelled seasonal groudnwater budgets for minor aquifers. Flows were normalized by the outcropping area of the HUs. A description of the HUs is presented in .

The average monthly water surplus and deficit were calculated for Great Britain and the HUs for the simulated period () to better understand and quantify the role of the storage component. At the national scale, an average pattern of 5 months of water surplus corresponding to positive variations in storage ( in EquationEquation 3)
(3)
(3) and increasing groundwater levels is observed, from October of one year to February of the next year. These are separated by 7 months of deficit when negative changes in storage occurs, and groundwater levels decline to compensate for the insufficiency of inflows in matching the outflows. On average, the maximum surplus is registered in December (10.3 mm) while the minimum is in February (1.2 mm). The maximum and minimum surplus values correspond to approximately 19% and 4% of the average monthly recharge. The maximum water deficit (−8.8 mm) is in May, which corresponds to almost the average recharge amount for the same month (86%). This means that on average the outflows in May are sustained by almost equal amounts of water from recharge and from groundwater released from storage.
This pattern is generally replicated in the majority of the HUs, with some exceptions. For instance, HU-2 (Palaeogene sands) has only 4 months of water surplus, while HU-6 (Cretaceous and Jurassic sandstones), HU-14 (Lower Palaeozoic units) and HU-15 (Precambrian units) have 6 months. In the latter two units, the shift between deficit and surplus tends to occur earlier in the year at the end of August, while the maximum water surplus is expected in October/November. In general, the reason for different patterns of water surplus/deficit are linked to regional climatic differences across Great Britain. Aquifers in Scotland (HU-15) in particular can exhibit prolonged recharge seasons compared to other areas in England (Jackson et al. Citation2005). Other exceptions include the Magnesian limestone aquifer (HU-10), where the onset of periods of water surplus and deficit are shifted forward by a month compared to other HUs.
3.4 Impact of climatic extremes on groundwater budgets
Climatic extremes such as droughts and floods in groundwater systems are spatio-temporal phenomena. Previous studies have examined the extent and impact of droughts in British aquifers at a regional scale based on statistical analysis of groundwater levels in selected boreholes (Marchant and Bloomfield Citation2018, Marchant et al. Citation2022). At the national scale, the BGWM can provide a more comprehensive and physically based understanding of the spatial and temporal dynamics of these phenomena and impacts on groundwater systems. Rather than quantifying drought severity from the calculation of standardized drought indices (Hellwig et al. Citation2020), the focus in this work is on evaluating changes from average conditions in the groundwater balance components during historically significant droughts and floods to gain insight about impacts of future events of comparable extent and severity.
Two examples of historically significant droughts in the UK include the periods from May 1975 to August 1976 (Rodda and Marsh Citation2011) and from February 2004 until October 2006 (Marsh Citation2007). Simulated groundwater recharge and river discharge rates during these two events are compared in for three lithologically different HUs. The 1975–1976 drought was a country-wide extreme event representing one of the driest 12-month rainfall periods and the driest 16-month period ever recorded. The reduction in autumn and winter recharge is noticeable for all the HUs. For the Chalk aquifer (HU-4), simulations shows that the recharge received during the period from October 1975 and March 1976 is only between 20% and 50% of the respective monthly averages. These simulated values match with estimates based on the amount of infiltration reported by Rodda and Marsh (Citation2011). Little to no recharge is received by the aquifer until September 1976, when the drought period ends quite abruptly due to a significant recharge in October. In the Jurassic limestones (HU-8), the reduction in recharge for the same period is even more extreme (79% to 91%), while the Permo–Triassic sandstones (HU-9) experienced a relatively less severe reduction (33% to 75%), particularly for the month of January 1976.
Figure 10. Simulated recharge and river discharge during historical droughts: 1976 (left) and 2004–2006 (right) in the Chalk (HU-4, top), Middle Jurassic (HU-8, middle) and Permo–Triassic sandstone aquifers (HU-9, lower). Flows were normalized by the outcropping area of the HUs.

To understand the impact of the 1976 drought on river flows, the simulated recharge rate is compared to the rate of groundwater discharge to rivers or baseflow. Chalk streams and rivers are particularly important because they are almost entirely groundwater fed and support diverse and unique ecological communities (e.g. Berrie Citation1992). In the Chalk, from October 1975 until September 1976 a reduction in baseflow between 18% and 58% of the average monthly values is estimated. These percentages correspond to a reduction in total volumetric baseflow between 1.01 and 3.7 million m3/day. Maps of simulated baseflow in the river network across the region () show that by January 1976 there is little to no baseflow in most of the Chalk streams and rivers and that this condition progressively worsened over the year, culminating with the virtual disappearance of most of the Chalk streams and rivers in August 1976. These simulated results match observations of ceased flow or extremely reduced flow in a number of rivers and of the drying up of their traditional sources (Rodda and Marsh Citation2011). Losses ranging from 36% to 73% are also estimated for Jurassic limestones, while simulated baseflow rates in the Permo–Triassic sandstones (HU-9) are relatively less affected (36% of maximum reduction, 22% on average) demonstrating higher resilience due to a higher storage capacity.
Figure 11. Simulated groundwater discharge into rivers (baseflow) in the southeast of England. Comparison between average values for January (top, left) and August (top, right) and corresponding values for 1976 (bottom). The white line indicates the boundary of the outcrop of the Chalk aquifer (HU-4).

The 2004–06 drought differs from the 1976 event because it had a more regional focus and was characterized by lower-than-average rainfall over two successive winter/spring periods instead of one. Drought conditions developed through the late autumn of 2004, mainly across southeast England, and lasted until the early winter of 2006–2007 (Marsh Citation2007). The regional focus of this event is evident also in the simulated groundwater budgets (). For instance, reductions in recharge of between 23% and 80% of the monthly averages are estimated for the two winter/spring periods of 2004–2005 and 2005–2006 in the Chalk aquifer (HU-4). The lack of two successive periods of autumn/winter recharge has an impact on the simulated baseflow rates, which stay consistently below monthly average values (63% on average) from November 2004 to November 2005. Conversely, in the Permo–Triassic sandstones (HU-9), whose main outcrops occur in central and northern England, the combined location and storage properties are responsible for a much lower impact, as shown by the relatively unaffected simulated baseflow rates.
Simulated recharge and baseflow rates during two historical groundwater flood events for different HUs are compared in . The 2000–2001 event caused long-lasting groundwater flooding, especially in Chalk areas, causing significant damage to homes and transport infrastructure (Hughes et al. Citation2011). The event was generated by exceptional rainfall over the period September 2000 to April 2001, particularly in England and Wales, where areas received more than 180% of the 1961–1990 average (Marsh and Dale Citation2002). Simulated groundwater budgets for this period show recharge rates higher than average for all the HUs. For instance, in the Chalk aquifer (HU-4), the estimated rate for October 2000 is 476% above the monthly average (13.5 mm vs. 64.3 mm). Two peaks of exceptional recharge rates are estimated between November and December 2000 (324% and 181%, respectively) and between February and March 2001 (215% and 312%, respectively). This double-peak event is observed also in the Jurassic limestones (HU-8), while the recharge signal is less intense in early 2001 in the Permo–Triassic sandstones (HU-9), indicating spatial granularity in the flood event. The anomalous recharge values correspond to very high simulated baseflow rates in the Chalk and Jurassic limestones aquifers, where baseflow rates remain significantly above average (180% on average) at least until October 2001, notwithstanding a very dry summer in 2001. Higher than average albeit not extreme rates (maximum 69%, 23% on average) are observed in the Permo–Triassic sandstones until August 2001, again indicating a smoothed response and higher resilience to extreme climatic events in this aquifer.
Figure 12. Simulated recharge and river discharge during historical groundwater floods: 2000–2001 (left) and 2013–2014 (right) in the Chalk (HU-4, top), Middle Jurassic (HU-8, middle) and Permo–Triassic sandstone aquifers (HU-9, lower). Flows were normalized by the outcropping area of the HUs.

Another historical flood event occurred in winter 2013–2014, caused by repeated storms and heavy rainfall from December 2013 to February 2014. During this period, flows in excess of 200% of the long-term averages were measured across many major rivers in southeast England and parts of Scotland, while groundwater flooding occurred in many areas of southern and central England (Huntingford et al. Citation2014). Simulated budgets for this period show higher than average recharge from December 2013 to February 2014, peaking in January 2014 () when recharge rates are more than double the corresponding monthly average. In absolute terms, values simulated for January 2014 are the highest estimated for the 1970–2018 simulation period in seven HUs in England (HU-2–HU-5, HU-7–HU-9) or among the highest. In the Chalk (HU-4) and Jurassic limestones (HU-8) aquifers, the recharge accumulated over the three winter months sustained higher than average baseflow for more than 6 months after February 2014 although recharge rates over this period were near or below average. This timing is an important measure of how these aquifer systems can respond to relatively short but very intense climatic events. In sandstone aquifers with hydraulic properties similar to the Permo–Triassic Sandstones (HU-9), the impact of these type of events is lessened.
4 Conclusions
The first national-scale groundwater model of Great Britain (BGWM) was implemented with the integration of a very large amount of data from multiple sources regarding the geology, hydrology, hydrogeology, land use, and climate. Although simplifications in the parameterization were necessary given the complexity and scale of the domain, it was shown that the BGWM can simulate groundwater dynamics at monthly and mostly 1 km resolutions with generally satisfactory levels of accuracy.
A combination of observation-based and qualitive analysis was used to assess the model performance and build confidence in the reliability of the simulated outputs. Identified key sources of error and uncertainty are mainly oversimplifications in the representation of the hydrogeological systems such as the relatively coarse parameterization omitting finer-scale heterogeneity within the hydrostratigraphic zonation, not considering the contribution of unconsolidated superficial aquifers to baseflow, ignoring temporal modifications of the recharge signal due to infiltration through the unsaturated zone, and inaccurate or omitted representations of hydraulic stresses from human activities in the boundary and initial conditions. Standard deviation of simulated heads from an ensemble of alternative solutions of the calibration problem showed ranges on the order of few centimetres to a few metres in relatively flat lowland areas of the domain, while higher uncertainties, in excess of 10 m of head, are associated with certain HUs and areas with high topographic relief and slope. Insight from the mixed quantitative-qualitive evaluation approach, which is the suggested strategy in recent modelling guidelines (Gleeson et al. Citation2021), not only allowed the main limitations of the current model to be identified, but will also serve to plan future upgrades of the BGWM targeting the main sources of uncertainty and error.
With the confidence built on the BGWM as a reasonably accurate digital representation of groundwater resources at the national scale, groundwater budgets were estimated for Great Britain and selected major and minor aquifers. Quantified inflows, outflows, and storage components show differences in the relative importance of the different components at the national and regional scale as well as seasonal variability. Seasonality is evident, for instance, in the alternation of periods of water surplus during autumn/winter months and deficit during spring/summer months, which correspond to periods with positive or negative changes in storage.
A key result of the budget assessment is that the BGWM provides unprecedented quantitive data which confirm that prolonged dry conditions causing insufficient recharge during the autumn/winter months are a triggering factor for the onset of droughts in British aquifers; also, they allow researchers to identify the spatial and temporal variability of this process at the national scale. Regional differences in the budget components were in fact found by analysing their variations during historically significant droughts and floods that occurred over the period considered by the simulations. Comparisons show that lithological and geographic factors, which in turn control hydrogeological properties and climatic components, determine the intensity and the duration of the impact of these events on groundwater resources, particularly with respect to changes in net recharge and river–aquifer interactions. Understanding differences in resilience to extreme climatic events is fundamental to designing effective national management and adaptation strategies to avoid potential future water crises or mitigate socio-economic impacts.
A model as complex as the BGWM will be under continual improvement and as such, the next phase of development will involve increasing the sophistication of the parameterization, including Quaternary deposits both to modify recharge processes and for their role in providing localized storage, as well a more realistic simulation of aquifer–marine interactions (coastal processes). By undertaking this future work, the BGWM will be improved so that it will become a robust decision support tool to test different solutions in the context of a changing population and climate.
Supplemental Material
Download MS Word (6.7 MB)Acknowledgements
We sincerely thank the editor, Giacomo Medici, and an anonymous reviewer for their insightful comments and suggestions, which improved the quality of the manuscript. This work was funded through the Hydro-JULES research programme of the Natural Environment Research Council (NERC) (NE/S017380/1). All the BGS authors publish with the permission of the Executive Director of the British Geological Survey (NERC).
Disclosure statement
No potential conflict of interest was reported by the author(s).
Supplementary material
Supplemental data for this article can be accessed online at https://doi.org/10.1080/02626667.2024.2320847
Additional information
Funding
References
- Alattar, M.H., et al., 2020. Modeling the surface water and groundwater budgets of the US using MODFLOW-OWHM. Advances in Water Resources, 143, 103682. doi:10.1016/j.advwatres.2020.103682
- Allen, D.J., et al., 1997. The physical properties of major aquifers in England and Wales. Keyworth, Nottingham: British Geological Survey. Technical Report No. WD/97/34.
- Anderson, M.P., Woessner, W.W., and Hunt, R.J., 2015. Applied groundwater modeling, 2nd edition. 2nd. San Diego: Academic Press.
- Ascott, M.J., et al., 2021. Managing groundwater supplies subject to drought: perspectives on current status and future priorities from England (UK). Hydrogeology Journal, 29 (3), 921–924. doi:10.1007/s10040-020-02249-0.
- Bell, V.A., et al., 2009. Use of soil data in a grid-based hydrological model to estimate spatial variation in changing flood risk across the UK. Journal of Hydrology, 377 (3), 335–350. doi:10.1016/j.jhydrol.2009.08.031.
- Bell, V.A., et al., 2021. Long term simulations of macronutrients (C, N and P) in UK freshwaters. Science of the Total Environment, 776, 145813. doi:10.1016/j.scitotenv.2021.145813
- Berrie, A.D., 1992. The chalk-stream environment. Hydrobiologia, 248 (1), 3–9. doi:10.1007/BF00008881.
- Best, M.J., et al., 2011. The Joint UK Land Environment Simulator (JULES), model description – part 1: energy and water fluxes. Geoscientific Model Development, 4 (3), 677–699. doi:10.5194/gmd-4-677-2011.
- Bianchi, M., et al., 2023. Using numerical modelling to test the geological and groundwater conceptual understanding of a complex, layered aquifer: a case study from the Fell Sandstone, Northumbria. Quarterly Journal of Engineering Geology and Hydrogeology, (ja), qjegh2022–077.
- Bierkens, M.F.P. and Wada, Y., 2019. Non-renewable groundwater use and groundwater depletion: a review. Environmental Research Letters, 14 (6), 63002.
- Bloomfield, J.P., Bricker, S.H., and Newell, A.J., 2011. Some relationships between lithology, basin form and hydrology: a case study from the Thames basin, UK. Hydrological Processes, 25 (16), 2518–2530. doi:10.1002/hyp.8024.
- Bloomfield, J.P., et al., 2020. Characterising variations in the salinity of deep groundwater systems: a case study from Great Britain (GB). Journal of Hydrology: Regional Studies, 28. doi:10.1016/j.ejrh.2020.100684.
- Bloomfield, J.P. and Marchant, B.P., 2013. Analysis of groundwater drought building on the standardised precipitation index approach. Hydrology and Earth System Sciences, 17 (12), 4769–4787. doi:10.5194/hess-17-4769-2013.
- Butler, A.P., et al., 2012. Advances in modelling groundwater behaviour in Chalk catchments. Geological Society, London, Special Publications, 364 (1), 113–127. doi:10.1144/SP364.9.
- Condon, L.E., et al., 2021. Global groundwater modeling and monitoring: opportunities and challenges. Water Resources Research, 57 (12), e2020WR029500. doi:10.1029/2020WR029500.
- de Graaf, I.E.M., et al., 2019. Environmental flow limits to global groundwater pumping. Nature, 574 (7776), 90–94. doi:10.1038/s41586-019-1594-4.
- de Graaf, I.E.M. and Stahl, K., 2022. A model comparison assessing the importance of lateral groundwater flows at the global scale. Environmental Research Letters, 17 (4), 44020. doi:10.1088/1748-9326/ac50d2.
- Delsman, J.R., et al., 2023. Reproducible construction of a high-resolution national variable-density groundwater salinity model for the Netherlands. Environmental Modelling and Software, 164, 105683. doi:10.1016/j.envsoft.2023.105683
- Doherty, J., 2018. PEST - model-independent parameter estimation. User manual. 7th ed. Brisbane, Australia: Watermark Numercal Computing.
- Doherty, J.E. and Hunt, R.J., 2010. Approaches to highly parameterized inversion-A guide to using PEST for groundwater-model calibration. Report No. 2010–5169.
- Famiglietti, J.S., 2014. The global groundwater crisis. Nature Climate Change, 4 (11), 945–948. doi:10.1038/nclimate2425.
- Fan, Y., et al., 2007. Incorporating water table dynamics in climate modeling: 1. Water table observations and equilibrium water table simulations. Journal of Geophysical Research: Atmospheres, 112 (D10). doi:10.1029/2006JD008111.
- Furusho-Percot, C., et al., 2019. Pan-European groundwater to atmosphere terrestrial systems climatology from a physically consistent simulation. Scientific Data, 6 (1), 320. doi:10.1038/s41597-019-0328-7.
- Gleeson, T., et al., 2016. The global volume and distribution of modern groundwater. Nature Geoscience, 9 (2), 161–167. doi:10.1038/ngeo2590.
- Gleeson, T., et al., 2021. GMD perspective: the quest to improve the evaluation of groundwater representation in continental- to global-scale models. Geoscientific Model Development, 14 (12), 7545–7571. doi:10.5194/gmd-14-7545-2021.
- Gnann, S.J., et al., 2021. Including regional knowledge improves baseflow signature predictions in large sample hydrology. Water Resources Research, 57 (2), e2020WR028354. doi:10.1029/2020WR028354.
- Gustard, A., Bullock, A., and Dixon, J.M., 1992. Low flow estimation in the United Kingdom. Wallingford, UK: Institute of Hydrology. IH Report No. 108.
- Guzy, A. and Malinowska, A.A., 2020. State of the art and recent advancements in the modelling of land subsidence induced by groundwater withdrawal. Water, 12 (7), 2051. doi:10.3390/w12072051.
- Hannaford, J., et al., 2023. The enhanced future Flows and Groundwater dataset: development and evaluation of nationally consistent hydrological projections based on UKCP18. Earth System Science Data, 15, 2391–2415. doi:10.5194/essd-15-2391-2023.
- Harbaugh, A.W., 1990. A computer program for calculating subregional water budgets using results from the U.S. geological survey modular three-dimensional finite- difference ground-water flow model. Report No. 90–392.
- Hellwig, J., et al., 2020. Large-scale assessment of delayed groundwater responses to drought. Water Resources Research, 56 (2), e2019WR025441. doi:10.1029/2019WR025441.
- Henriksen, H.J., et al., 2003. Methodology for construction, calibration and validation of a national hydrological model for Denmark. Journal of Hydrology, 280 (1), 52–71. doi:10.1016/S0022-1694(03)00186-0.
- Hough, M.N. and Jones, R.J.A., 1997. The United Kingdom meteorological office rainfall and evaporation calculation system: MORECS version 2.0-an overview. Hydrology and Earth System Sciences, 1 (2), 227–239. doi:10.5194/hess-1-227-1997.
- Hughes, A.G., et al., 2011. Flood risk from groundwater: examples from a Chalk catchment in southern England. Journal of Flood Risk Management, 4 (3), 143–155. doi:10.1111/j.1753-318X.2011.01095.x.
- Hunt, R.J., et al., 2021. Evaluating lower computational burden approaches for calibration of large environmental models. Groundwater, 59 (6), 788–798. doi:10.1111/gwat.13106.
- Hunt, R.J., Fienen, M.N., and White, J.T., 2020. Revisiting “An exercise in groundwater model calibration and prediction” after 30 years: insights and new directions. Groundwater, 58 (2), 168–182. doi:10.1111/gwat.12907.
- Huntingford, C., et al., 2014. Potential influences on the United Kingdom’s floods of winter 2013/14. Nature Climate Change, 4 (9), 769–777. doi:10.1038/nclimate2314.
- Ireson, A.M. and Butler, A.P., 2013. A critical assessment of simple recharge models: application to the UK Chalk. Hydrology and Earth System Sciences, 17, 2083–2096. doi:10.5194/hess-17-2083-2013.
- Jackson, C.R., et al., 2005. Numerical testing of conceptual models of groundwater flow: a case study using the Dumfries Basin aquifer. Scottish Journal of Geology, 41 (1), 51–60. doi:10.1144/sjg41010051.
- Jackson, C.R., Meister, R., and Prudhomme, C., 2011. Modelling the effects of climate change and its uncertainty on UK chalk groundwater resources from an ensemble of global climate model projections. Journal of Hydrology, 399 (1), 12–28. doi:10.1016/j.jhydrol.2010.12.028.
- Jain, M., et al., 2021. Groundwater depletion will reduce cropping intensity in India. Science Advances, 7 (9), eabd2849. doi:10.1126/sciadv.abd2849.
- Jasechko, S. and Perrone, D., 2021. Global groundwater wells at risk of running dry. Science, 372 (6540), 418–421. doi:10.1126/science.abc2755.
- Jones, H.K., et al., 2000. The physical properties of minor aquifers in England and Wales. Keyworth, Nottingham: British Geological Survey, Technical Report No. WD/00/4.
- Krause, P., Boyle, D.P., and Bäse, F., 2005. Comparison of different efficiency criteria for hydrological model assessment. Advances in Geosciences, 5, 89–97. doi:10.5194/adgeo-5-89-2005
- Lafare, A.E.A., Peach, D.W., and Hughes, A.G., 2021. Use of point scale models to improve conceptual understanding in complex aquifers: an example from a sandstone aquifer in the Eden valley, Cumbria, UK. Hydrological Processes, 35 (5), e14143. doi:10.1002/hyp.14143.
- Lancia, M., et al., 2022. The China groundwater crisis: a mechanistic analysis with implications for global sustainability. Sustainable Horizons, 4, 100042. doi:10.1016/j.horiz.2022.100042
- Langevin, C.D., et al., 2017. Documentation for the MODFLOW 6 groundwater flow model. Reston, VA: U.S. Geological Survey, USGS Numbered Series No. 6-A55.
- Lee, J.R., 2021. Permeability classification for UK natural superficial deposits – a hydro-JULES dataset. Nottingham, UK: British Geological Survey. Open Report No. OR/21/050.
- Lee, J.R., Candy, I., and Haslam, R., 2018. The neogene and quaternary of England: landscape evolution, tectonics, climate change and their expression in the geological record. Proceedings of the Geologists’ Association, 129 (3), 452–481.
- Liu, P.-W., et al., 2022. Groundwater depletion in California’s Central Valley accelerates during megadrought. Nature Communications, 13 (1), 7825. doi:10.1038/s41467-022-35582-x.
- Mackay, J.D., et al., 2015. Seasonal forecasting of groundwater levels in principal aquifers of the United Kingdom. Journal of Hydrology, 530, 815–828. doi:10.1016/j.jhydrol.2015.10.018
- Mansour, M.M. and Hughes, A.G., 2004. User’s manual for the distributed recharge model ZOODRM. Keyworth, Nottingham: British Geological Survey. Internal Report No. IR/04/150.
- Mansour, M.M., et al., 2012. The role of numerical modelling in understanding groundwater flow in Scottish alluvial aquifers. Geological Society, London, Special Publications, 364 (1), 85–98. doi:10.1144/SP364.7.
- Mansour, M.M., et al., 2018. Estimation of spatially distributed groundwater potential recharge for the United Kingdom. Quarterly Journal of Engineering Geology and Hydrogeology, 51 (2), 247–263. doi:10.1144/qjegh2017-051.
- Marchant, B.P., et al., 2022. Temporal interpolation of groundwater level hydrographs for regional drought analysis using mixed models. Hydrogeology Journal, 30 (6), 1801–1817. doi:10.1007/s10040-022-02528-y.
- Marchant, B.P. and Bloomfield, J.P., 2018. Spatio-temporal modelling of the status of groundwater droughts. Journal of Hydrology, 564, 397–413.
- Marsh, T., 2007. The 2004–2006 drought in Southern Britain. Weather, 62 (7), 191–196. doi:10.1002/wea.99.
- Marsh, T.J. and Dale, M., 2002. The UK floods of 2000–2001: a hydrometeorological appraisal. Water and Environment Journal, 16 (3), 180–188. doi:10.1111/j.1747-6593.2002.tb00392.x.
- Mather, B., et al., 2022. Constraining the response of continental-scale groundwater flow to climate change. Scientific Reports, 12 (1), 4539. doi:10.1038/s41598-022-08384-w.
- Maxwell, R.M., Condon, L.E., and Kollet, S.J., 2015. A high-resolution simulation of groundwater and surface water over most of the continental US with the integrated hydrologic model ParFlow v3. Geoscientific Model Development, 8 (3), 923–937. doi:10.5194/gmd-8-923-2015.
- McKenzie, A.A., 2015. User guide for the British geological survey national depth to groundwater dataset. Keyworth, Nottingham: British Geological Survey, Open Report No. OR/15/006.
- Medici, G. and West, L.J., 2022. Review of groundwater flow and contaminant transport modelling approaches for the Sherwood Sandstone aquifer, UK; insights from analogous successions worldwide. Quarterly Journal of Engineering Geology and Hydrogeology, 55 (4), qjegh2021–176. doi:10.1144/qjegh2021-176.
- Medici, G., West, L.J., and Banwart, S.A., 2019. Groundwater flow velocities in a fractured carbonate aquifer-type: implications for contaminant transport. Journal of Contaminant Hydrology, 222, 1–16. doi:10.1016/j.jconhyd.2019.02.001
- Medici, G., West, L.J., and Mountney, N.P., 2016. Characterizing flow pathways in a sandstone aquifer: tectonic vs sedimentary heterogeneities. Journal of Contaminant Hydrology, 194, 36–58. doi:10.1016/j.jconhyd.2016.09.008
- Medici, G., West, L.J., and Mountney, N.P., 2017. Characterization of a fluvial aquifer at a range of depths and scales: the Triassic St Bees Sandstone Formation, Cumbria, UK. Hydrogeology Journal, 26, 565–591. doi:10.1007/s10040-017-1676-z.
- Moriasi, D.N., et al., 2007. Model evaluation guidelines for systematic quantification of accuracy in watershed simulations. Transactions of the ASABE, 50 (3), 885–900. doi:10.13031/2013.23153.
- Morris, D., Flavin, R., and Morris, R., 1990. A digital terrain model for hydrology. In: Proceedings of 4th International Symposium on Spatial Data Handling. Presented at the 4th International Symposium on Spatial Data Handling, Zurich, 250–262.
- Nair, A.S. and Indu, J., 2021. Assessment of groundwater sustainability and identifying factors inducing groundwater depletion in India. Geophysical Research Letters, 48 (3), e2020GL087255. doi:10.1029/2020GL087255.
- Newell, A.J., 2018. Implicit geological modelling: a new approach to 3D volumetric national-scale geological models [online]. Available from: https://nora.nerc.ac.uk/id/eprint/527473/ [ Accessed 18 April 2023].
- O’Dochartaigh, B.E., et al., 2015. Scotland’s aquifers and groundwater bodies. Edinburgh: British Geological Survey. Open Report No. OR/15/028.
- Piggott, A.R., Moin, S., and Southam, C., 2005. A revised approach to the UKIH method for the calculation of baseflow/Une approche améliorée de la méthode de l’UKIH pour le calcul de l’écoulement de base. Hydrological Sciences Journal, 50 (5), null–920. doi:10.1623/hysj.2005.50.5.911.
- Price, M., 1987. Fluid flow in the Chalk of England. Geological Society, London, Special Publications, 34 (1), 141–156. doi:10.1144/GSL.SP.1987.034.01.10.
- Prudic, D.E., Konikow, L.F., and Banta, E.R., 2004. A new Streamflow-Routing (SFR1) package to simulate stream-aquifer interaction with MODFLOW-2000. U.S. Geological Survey. Open-File Report No. 2004–1042.
- Rahman, M., Pianosi, F., and Woods, R., 2023. Simulating spatial variability of groundwater table in England and Wales. Hydrological Processes, 37 (3), e14849. doi:10.1002/hyp.14849.
- Rameshwaran, P., et al., 2022. Use of abstraction and discharge data to improve the performance of a national-scale hydrological model. Water Resources Research, 58 (1), e2021WR029787. doi:10.1029/2021WR029787.
- Reinecke, R., et al., 2021. Uncertainty of simulated groundwater recharge at different global warming levels: a global-scale multi-model ensemble study. Hydrology and Earth System Sciences, 25 (2), 787–810. doi:10.5194/hess-25-787-2021.
- Rodda, J.C. and Marsh, T.J., 2011. The 1975-76 drought – a contemporary and retrospective review. Wallingford, UK: Centre for Ecology & Hydrology. Open Report.
- Rohde, M.M., Froend, R., and Howard, J., 2017. A global synthesis of managing groundwater dependent ecosystems under sustainable groundwater policy. Groundwater, 55 (3), 293–301. doi:10.1111/gwat.12511.
- Rushton, K.R., Connorton, B.J., and Tomlinson, L.M., 1989. Estimation of the groundwater resources of the berkshire downs supported by mathematical modeling. Quarterly Journal of Engineering Geology and Hydrogeology, 22 (4), 329–341. doi:10.1144/GSL.QJEG.1989.022.04.06.
- Rushton, K.R. and Skinner, A.C., 2012. A national approach to groundwater modelling: developing a programme and establishing technical standards. Geological Society, London, Special Publications, 364 (1), 7–17. doi:10.1144/SP364.2.
- Scanlon, B.R., et al., 2023. Global water resources and the role of groundwater in a resilient water future. Nature Reviews Earth & Environment, 4, 87–101. doi:10.1038/s43017-022-00378-6.
- Sechu, G.L., et al., 2022. Mapping groundwater-surface water interactions on a national scale for the stream network in Denmark. Journal of Hydrology: Regional Studies, 40, 101015.
- Sefton, C., et al., 2023. Hydrological summary for the United Kingdom: February 2023 [online]. Available from: http://nrfa.ceh.ac.uk/monthly-hydrological-summary-uk [ Accessed 25 April 2023].
- Seidenfaden, I.K., et al., 2022. Quantification of climate change sensitivity of shallow and deep groundwater in Denmark. Journal of Hydrology: Regional Studies, 41, 101100.
- Streetly, M.J., 2023. A personal perspective of 20 years of regional groundwater resource modelling of the Permo–Triassic Sandstone aquifers in England. Quarterly Journal of Engineering Geology and Hydrogeology, 56 (3), qjegh2022–090. doi:10.1144/qjegh2022-090.
- Tarboton, D.G. and Ames, D.P., 2012. Advances in the mapping of flow networks from digital elevation data. Civil and Environmental Engineering Faculty Publications. Paper 2577. https://digitalcommons.usu.edu/cee_facpub/2577
- Taylor, R.G., et al., 2013. Ground water and climate change. Nature Climate Change, 3 (4), 322–329. doi:10.1038/nclimate1744.
- Tetzlaff, D. and Soulsby, C., 2008. Sources of baseflow in larger catchments – using tracers to develop a holistic understanding of runoff generation. Journal of Hydrology, 359 (3), 287–302. doi:10.1016/j.jhydrol.2008.07.008.
- United Nations, 2022. The United Nations world water development report 2022: groundwater: making the invisible visible. Paris, France.
- Vergnes, J.-P., Caballero, Y., and Lanini, S., 2022. Assessing climate change impact on French groundwater resources using a spatially distributed hydrogeological model. Hydrological Sciences Journal, 68 (2), 209–227. doi:10.1080/02626667.2022.2150553.
- Westerhoff, R., White, P., and Miguez-Macho, G., 2018. Application of an improved global-scale groundwater model for water table estimation across New Zealand. Hydrology and Earth System Sciences, 22 (12), 6449–6472. doi:10.5194/hess-22-6449-2018.
- White, J.T., 2018. A model-independent iterative ensemble smoother for efficient history-matching and uncertainty quantification in very high dimensions. Environmental Modelling and Software, 109, 191–201. doi:10.1016/j.envsoft.2018.06.009
- Whiteman, M.I., et al., 2012. Start, development and status of the regulator-led national groundwater resources modelling programme in England and Wales. Geological Society, London, Special Publications, 364 (1), 19–37. doi:10.1144/SP364.3.
- Zhang, H. and Hiscock, K.M., 2010. Modelling the impact of forest cover on groundwater resources: a case study of the Sherwood Sandstone aquifer in the East Midlands, UK. Journal of Hydrology, 392 (3), 136–149. doi:10.1016/j.jhydrol.2010.08.002.
- Zheng, C. and Guo, Z., 2022. Plans to protect China’s depleted groundwater. Science, 375 (6583), 827. doi:10.1126/science.abn8377.
Appendix
The following metrics were used to assess the model performance (Krause et al. Citation2005, Moriasi et al. Citation2007). In all the formulas below, and
indicate measured and simulated quantities (i.e. groundwater levels or river discharge), respectively, while
indicate the mean values.
Mean error (ME):
Mean absolute error (MAE):
Root mean squared error (RMSE):
Coefficient of determination (R2):
Nash-Sutcliffe efficiency (NSE):
Percent bias (PBIAS):
Pearson correlation coefficient (r):