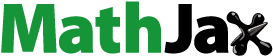
Abstract
Biological modeling for anti-cancer treatments using mathematical models can be very supportive in gaining more insight into dynamic processes responsible for cellular response to treatment, and predicting, evaluating and optimizing therapeutic effects of treatment. This review presents an overview of the current status of biological modeling for hyperthermia in combination with radiotherapy (thermoradiotherapy). Various distinct models have been proposed in the literature, with varying complexity; initially aiming to model the effect of hyperthermia alone, and later on to predict the effect of the combined thermoradiotherapy treatment. Most commonly used models are based on an extension of the linear-quadratic (LQ)-model enabling an easy translation to radiotherapy where the LQ model is widely used. Basic predictions of cell survival have further progressed toward 3 D equivalent dose predictions, i.e., the radiation dose that would be needed without hyperthermia to achieve the same biological effect as the combined thermoradiotherapy treatment. This approach, with the use of temperature-dependent model parameters, allows theoretical evaluation of the effectiveness of different treatment strategies in individual patients, as well as in patient cohorts. This review discusses the significant progress that has been made in biological modeling for hyperthermia combined with radiotherapy. In the future, when adequate temperature-dependent LQ-parameters will be available for a large number of tumor sites and normal tissues, biological modeling can be expected to be of great clinical importance to further optimize combined treatments, optimize clinical protocols and guide further clinical studies.
1. Introduction
Biological modeling can be very supportive to enhance insight into tumor dynamics and response to anti-cancer treatments. In radiotherapy, dedicated software packages have been developed to predict treatment outcomes by radiobiological plan evaluation, such as Bioplan [Citation1], with a variety of practical tools, e.g., tumor control probability (TCP) and normal tissue complication probability (NTCP) models with parametric sensitivity analysis, dose statistics, equivalent uniform dose (EUD) and individualized dose prescription. Furthermore, the possible consequences of ‘cold’ and ‘hot’ radiation dose regions can be evaluated using the ΔTCP method [Citation2]. Such biological modeling software can be very useful for individualized dose prescription. For example, a potential gain of ∼10% increase in TCP could be achieved from dose individualization strategies in prostate cancer patients, with comparable NTCP [Citation3]. Presently, modern commercial radiotherapy treatment planning systems, such as Eclipse and RayStation, provide tools for biological optimization and evaluation of treatment plans. The use of biological modeling in radiotherapy is widely accepted to determine and evaluate fractionation schedules by comparing equivalent doses for different fractionation schemes. Using biological modeling, biologically equivalent doses (BED) can be calculated, which can be applied for example when optimizing treatment schedules for hypofractionation or hyperfractionation [Citation4,Citation5].
Mild hyperthermia (i.e., heating to 39–44 °C) induces radio and chemosensitization via a large number of biological mechanisms, such as DNA damage repair inhibition, direct cell kill, reoxygenation, reperfusion and also immunologic effects [Citation6–9]. Some of these mechanisms already become active at a relatively low temperature (>39 °C), e.g., reperfusion, while other mechanisms, such as DNA repair inhibition, need higher temperature levels for activation, and show a dose-effect relationship [Citation10]. The effectiveness of a hyperthermia treatment depends thus on the tumor temperatures achieved. This has been confirmed in several studies that found clinical outcome and thermal dose to be correlated [Citation11–17]. In combined radiotherapy and hyperthermia (thermoradiotherapy) treatments, evaluation of treatment quality is generally performed for the individual modalities separately, based on the delivered (biological) dose and achieved temperature, respectively.
Hyperthermia treatment quality is often expressed as a thermal iso-effect dose, CEM43, i.e., the cumulative equivalent minutes at 43 °C. This relatively simple biological model was originally introduced by Sapareto and Dewey [Citation18] as a concept to represent the effect of the entire heat exposure during treatment on cell death. Although this is an elegant concept and some studies reported CEM43 as a predictive parameter [Citation11,Citation16,Citation17], there are several weaknesses when CEM43 is used to predict treatment efficacy for combined radiotherapy plus hyperthermia treatments [Citation10]. One important issue is that CEM43 accounts for the direct cytotoxic effect of heat via an Arrhenius relationship, thus assuming the amount of cell death depends on the temperature and exposure time. This direct cytotoxicity does not account for the biological (sensitization) mechanisms of hyperthermia and thus CEM43 has no predictive value for the radiosensitivity of tumors. That being said, some sensitization mechanisms depend on disabling certain cellular proteins, a temperature-dependent process that may follow an Arrhenius-like relationship [Citation10]. This may explain why a direct relation between CEM43 and clinical endpoints cannot always be established [Citation19]. Furthermore, the extent of radiosensitisation is also influenced by the number of fractions and the timing of the combined treatment. The thermal enhancement ratio, i.e., the ratio between the radiation dose required to achieve a specific endpoint with radiotherapy alone and in combination with hyperthermia, is largest when radiotherapy and hyperthermia are applied simultaneously and decreases with increasing time interval between the two modalities [Citation20]. Thus, because of the complex synergy between radiotherapy and hyperthermia, CEM43 is not the sole predictor and does not per se predict treatment results and establishing a reliable predictive dose parameter would require multi-parameter biological prediction models.
Reliable biological models for thermoradiotherapy treatments that account for the complex synergy between hyperthermia and radiotherapy would be very useful to obtain insight into the overall treatment quality and compare the effectiveness of different treatment strategies, similar to biological models used for radiotherapy alone. To be able to compare results of thermoradiotherapy treatments, the need for an adequate equivalent dose parameter is evident, especially since clinically achieved temperatures during each treatment can vary largely within patients and also between patients. Multi-parameter models incorporating the biological effects of hyperthermia are thus essential to predict the level of heat-induced cell death in multi-modality treatments. Over the past decades, significant progress has been made in understanding the biological mechanisms of hyperthermia and developing adequate mathematical formulations to predict hyperthermic effects in thermoradiotherapy treatments. In this review, we present an overview of the current status of biological modeling for thermal enhancement of radiation response and we also discuss ongoing developments toward biological thermoradiotherapy treatment planning for routine clinical use.
2. Biological mechanisms of hyperthermia
Biological modeling aims to capture the biological effects of hyperthermia in a mathematical model capable of predicting the effectiveness of treatment delivery. This is a very challenging task, as hyperthermia is known to work via a plethora of mechanisms. Based on current knowledge, Issels et al. proposed the definition of 6 important hallmarks of hyperthermia [Citation21]:
Induced cell killing: Heat can disrupt key cellular processes within the cell. Cells can thus be killed when exposed to heat and the fraction of cells that do not survive heating depends on the cell type, origin, achieved the temperature and the duration of the heat exposure. Assuming a typical clinical mild hyperthermia treatment of 1h, normally oxygenated cells can usually stand the elevated temperatures. Direct cytotoxic effects of heat at hyperthermic temperature levels occur especially in chronically hypoxic cells suffering from nutrient deprivation and low pH [Citation22,Citation23]. This enhanced cytotoxicity is helpful since chronically hypoxic cells are less sensitive to radiotherapy [Citation23]. Cells may die due to apoptosis, provided that this pathway is present and the cells are not inactivated by the heat dose. Otherwise, inactivated cells will stop proliferating via permanent cell cycle arrest, necrosis or secondary apoptosis after S-phase or mitotic failure [Citation24].
Inhibition of DNA repair: Radiotherapy and some cytotoxic agents aim at inducing lethal damage in tumor DNA. DNA double-strand breaks are the most lethal lesions. Cells start the repair of double-strand breaks immediately after induction of damage. The repair can be done by non-homologous end-joining (NHEJ) or homologous recombination (HR). NHEJ is normally responsible for the majority of the repair since it is active throughout the whole cell cycle. Heat affects the NHEJ repair process through the inhibition of DNA-dependent protein kinase catalytic subunit (DNA-PKcs) activity, which is thermal dose-dependent [Citation25]. Essential repair proteins Ku70, Ku80, BRCA1 and 53BP1 are decreased [Citation25]. HR is the second most important repair pathway and is only active in S-phase. At temperatures exceeding 41 °C BRCA2, a key protein in HR is temporarily depleted, thereby inhibiting the HR repair pathway [Citation26]. Furthermore, the level and duration of BRCA2 depletion, and thus HR inhibition, increases with both temperature and heating time [Citation27]. After radiotherapy, the majority of DNA damage is repaired within 2h, which means that the effectiveness of subsequent hyperthermia decreases with increasing time intervals between radiotherapy and hyperthermia [Citation28]. After hyperthermia, HR remains inhibited for up to 4 hours [Citation26], which means that subsequent radiotherapy is preferably given within that time frame.
Changing tumor microenvironment: The tumor microenvironment is often chronically hypoxic, poorly perfused and exhibits abnormally high interstitial fluid pressure (IFP). These negative environmental characteristics induce treatment resistance in tumor cells to radiotherapy and chemotherapy. Hypoxia is caused by an imbalance between oxygen delivery and consumption rate. Furthermore, dynamic changes in oxygenation occur due to changes in red blood cell flux, vascular changes and thermoregulation. These effects lead to cyclic or intermittent hypoxia [Citation29]. The application of heat induces physiological changes and affects the microenvironment [Citation30]. Hyperthermia at temperatures between 39 and 43 °C yields a temporary increase in blood flow, and a positive alteration of pO2 and IFP [Citation31,Citation32]. Heat-induced vasodilation yields enhanced local perfusion and reoxygenation [Citation33]. Note that this reoxygenation already occurs at relatively low temperatures starting from ∼39 °C, easily achieved in the clinic [Citation34]. In addition, reoxygenation is also caused indirectly by hyperthermia by a reduced oxygen consumption rate due to the killing of (chronic) hypoxic cells at somewhat higher temperatures [Citation35]. The improved tumor oxygenation and decreased IFP may last for at least 24h [Citation30,Citation31,Citation34].
Inducing cellular stress response: Heat transiently up-regulates heat shock genes that encode a class of proteins known as heat shock proteins (HSP) [Citation24]. HSPs act as molecular chaperones of other proteins, thereby assisting in the recovery from stress either by repairing damaged proteins (protein refolding) or by degrading them [Citation24]. Released HSPs can stimulate the immune response (see hallmark V), but HSP up-regulation is also associated with thermotolerance, i.e., transient resistance of cells to a second heat shock within 1–2 days, which is the reason why in clinical protocols hyperthermia is not applied daily, but just once or twice a week.
Modulating immune response: Hyperthermia can increase the visibility of tumor cells to the immune system and activates tumor-specific immune cells [Citation36]. There is direct heat-induced activation of natural killer (NK) cells, CD8+ T cells and dendritic cells (DCs). HSPs also activate NK cells and antigen presenting cells (APCs) [Citation37]. Surface expression of MICA and MHCI is increased after heating, making the cells more vulnerable to NK cells and CD8+ T cells, respectively [Citation38,Citation39]. Heating makes the tumor cells release exosomes, which transfer potential tumor antigens to APCs, cross-presenting them to CD8+ T cells. Increased perfusion may facilitate the trafficking of immune cells between tumors and draining lymph nodes. This immune cell trafficking may be further improved through changes in the vasculature adhesion molecules by increased permeability of the tumor vasculature [Citation40].
Sensitization to radiotherapy, chemotherapy and immunotherapy: Mechanisms described in II-V lead to sensitization to radiotherapy and chemotherapy. For example, inhibition of repair of potentially lethal or sublethal DNA damage increases the effectiveness of radiotherapy and some chemotherapeutics in causing lethal double-strand breaks and also increased oxygenation after hyperthermia makes these modalities more cytotoxic. Hyperthermia may also enhance vascular leakage, thereby improving the uptake of chemotherapeutic agents [Citation41]. Furthermore, hyperthermia enhances radiation-induced immunomodulation [Citation42,Citation43]. However, cancer cells are unfortunately also able to exploit the immune checkpoints that prevent the immune system from accidentally destroying healthy cells during an immune response, which enables the cancer cells to escape from immune detection and elimination. Checkpoint inhibitor immunotherapy is therefore a very promising treatment modality and local vasodilation by hyperthermia can further improve effectiveness by enhancing the delivery of these inhibitors to the tumor [Citation44,Citation45]. Thus, there is a clear rationale to combine radiotherapy with hyperthermia and checkpoint inhibitor immunotherapy [Citation46] and very promising preclinical results were recently reported e.g., for pancreatic cancer [Citation47]. In a recent review, Hurwitz discusses the rationale for clinical investigation of hyperthermia and immunotherapy for several tumor sites, including melanoma, bladder, and head and neck cancers [Citation48]. Ongoing research should provide more (pre)clinical data to determine the optimal temperature and timing [Citation45,Citation49].
All above hyperthermia mechanisms are temperature and thermal dose-dependent. Though temperature dependence of blocking cell survival is fully covered by the CEM43 model and some other mechanisms also conform with the Arrhenius model (e.g., DNA repair and HSP70 promotor activation [Citation10,Citation50]) and are therefore at least partly covered by the CEM43 model, it is quite clear that the huge complexity of both direct and synergistic effects makes it extremely challenging to quantitatively predict the effect of hyperthermia in combined treatments using mathematical/biological models. Especially capturing changes in microenvironment and immune response modulation in a predictive model will be most challenging. The next section first describes the basics of mathematical modeling of heat-induced cell killing, followed by a discussion of work that has been done on modeling to describe the effect of hyperthermia combined with radiation. This review ends with our vision of required future developments toward routine clinical use.
3. Mathematical modeling of hyperthermic effects
3.1. Modeling heat induced cell killing
In Petri dishes, most heat survival curves have a very similar shape as those observed after exposure to ionizing irradiation: typically, there is an initial ‘shoulder’ region followed by a relatively steep decline in cell survival, as depicted schematically in . The shape of the survival curves is temperature-dependent: the initial shoulder becomes less pronounced with increasing temperatures, and the slope becomes steeper. As a basis for biological modeling of hyperthermia treatments, the earliest models predicted heat-induced thermal damage after exceeding certain time-temperature thresholds, based on an Arrhenius formulation. For example, Moritz and Henriquez used this concept to describe the thermal dose-dependent development of skin burns in pigs [Citation51]. In an extensive review, Pearce discusses mathematical models of cell death and thermal damage processes [Citation52]. A drawback of these Arrhenius-type models is that they often fail to represent thermally induced cell damage or death at relatively moderate hyperthermic temperatures by showing a significant over-prediction of the thermal damage fractions in the early stages of heating, i.e., during the early stage ‘shoulder’ region. Therefore, models have been designed to better describe the characteristic ‘shoulder’ shape of tumor cell heat survival curves.
Figure 1. Schematic cell survival curves for heat alone, radiation alone and combined radiation with heat; the vertical axes have a logarithmic scale. The shape of the survival curves is temperature-dependent: the initial shoulder becomes less pronounced with increasing temperatures, and the slope becomes steeper. Note that exact survival fractions at specific dose levels and temperatures are cell-line dependent.
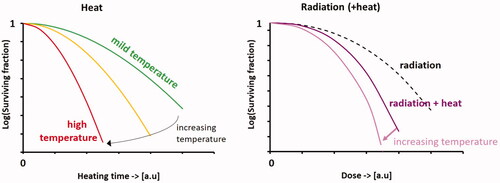
A model that provides essential basic insight and can predict heat survival curves very well, was proposed in 1986 by Jung [Citation53]. This model predicts cellular inactivation by heat and quantitatively describes cell killing after single heating, as well as after consecutive heat treatments at different temperatures. Cellular inactivation by heat is considered a 2-step process. First, heating produces non-lethal lesions, which are then converted (by heat) to lethal events. This yields two temperature-dependent parameters p and c, representing the rate constant for the production of non-lethal lesions per cell and per unit of time, and the rate constant for the conversion of a non-lethal lesion into a lethal event per unit of time, respectively. The survival fraction S/S0 after heating for time t is then given by:
(1)
(1)
Similarly, for subsequent heat treatment at two different temperatures for times t1 and t2, the survival fraction can be calculated using
(2)
(2)
The parameters p and c obey the Arrhenius law and p shows an inflection point at 42.5 °C for Chinese hamster ovary (CHO) cells. For human cells, an inflection point will probably occur at slightly higher temperatures [Citation54]. This model perfectly explains why heat survival curves exhibit a shoulder. At the start of treatment, there are no non-lethal lesions to turn into lethal events, so the survival curve starts with a zero slope. After some time, the number of non-lethal lesions increases, as well as the probability of inactivation. This leads to an initial shoulder, followed by an increase in steepness of the heat survival curve when initially non-lethal lesions turn into lethal lesions. In addition, this model is generally useful to predict thermosensitization of different treatment strategies. It provides very relevant insight into how complex heating protocols like step-up and step-down heating affect cellular inactivation in a different manner. For example with step-down heating, i.e., heating at high temperature followed by heating at a lower temperature, a certain number of non-lethal lesions are produced by pre-heating, which may readily convert to lethal lesions during the second treatment. This greatly enhances thermosensitization, which leads to the disappearance of the shoulder with increasing pre-heating time and a steeper survival curve. Furthermore, this model can also predict negatively modified thermal response by induced thermotolerance [Citation55].
Given the similarity between cell survival curves after exposure to heat and ionizing irradiation, also the multi-target single-hit model and the linear-quadratic (LQ) model, as used in radiotherapy, were proposed to predict cell killing by hyperthermia alone [Citation56]. The multi-target single-hit model predicts the survival fraction of cells treated by heat using:
(3)
(3)
where tT is the exposure time at temperature T, t0 is the activation energy for cell killing at temperature T and n is the extrapolation number. In this model, the t0 parameter is temperature dependent and obeys the Arrhenius law. The other parameter, n, is cell-line dependent. Using the LQ-model, the survival fraction is expressed as:
(4)
(4)
With tT again the exposure time at temperature T and α and β arbitrary constants. According to Arrhenius analysis, α is a linear function of the reciprocal of the temperature and β of temperature squared. Thus, both parameters obey the Arrhenius law and the LQ-model can completely define cell killing as a function of both time and temperature.
3.2. Modeling effects of radiation combined with hyperthermia
To model the effect of hyperthermia in combination with radiotherapy, various models have been proposed, with varying complexity. Some of these model frameworks primarily focus on obtaining more insight into dynamic processes responsible for cellular response to treatment, while other models aim toward the prediction of therapeutic effects.
3.2.1. The multi-hit repair model
The multi-hit repair (MHR) model, as proposed by Scheidegger et al. [Citation57], is a dynamic population model using variables of state, and is based on a chain of cell populations. The initial population (N0, counted by state variable N0) is clonogenic and can undergo mitosis. Cells accumulate ‘hits’, which are lesions induced by radiation. This causes cell damage and hinders cells from mitosis. These damaged cells move to the next population in the chain. Cells with lethal damage are removed from the population and successful repair allows to move cells to a population upward in the chain. This basic concept of the MHR model is illustrated in .
Figure 2. Schematic illustration of the multi-hit repair approach to model response of tumor cells to radiation and heat [Citation57]. The model is based on a chain of cell populations (N 0, …, N k), characterized by the number of radiation-induced damages (‘hits’). At the start of the simulation, the initial population (N0, counted by state variable N0) is clonogenic and can undergo mitosis. During treatment, cells accumulate radiation-induced damage (‘hits’). These damaged cells move to the next population in the chain (green arrows). Damage will be repaired with a certain repair probability, and after successful repair cells move back to a population upward in the chain (black arrows). Hyperthermia reduces this repair capacity. When the damage becomes lethal, cells are removed from the population (red arrows). The final population represents the cells that survived after treatment.
![Figure 2. Schematic illustration of the multi-hit repair approach to model response of tumor cells to radiation and heat [Citation57]. The model is based on a chain of cell populations (N 0, …, N k), characterized by the number of radiation-induced damages (‘hits’). At the start of the simulation, the initial population (N0, counted by state variable N0) is clonogenic and can undergo mitosis. During treatment, cells accumulate radiation-induced damage (‘hits’). These damaged cells move to the next population in the chain (green arrows). Damage will be repaired with a certain repair probability, and after successful repair cells move back to a population upward in the chain (black arrows). Hyperthermia reduces this repair capacity. When the damage becomes lethal, cells are removed from the population (red arrows). The final population represents the cells that survived after treatment.](/cms/asset/a59af299-fed7-4bd7-806f-2a8d4cf3f9fc/ihyt_a_2113826_f0002_c.jpg)
For practical implementations, the chain length k is limited, and typically between 5 and 10. The core differential equation for population Ni is given by:
(5)
(5)
where α (Gy−1) is a radiosensitivity parameter (unrelated to the LQ-parameter α), ce (h−1) is the elimination rate constant, R(t) the dose rate and r a function describing repair. State variables count population sizes N0 to Nk, and determine the repair probability. Another state variable describes the initial impedance of repair due to radiation-induced damage to repair proteins. Time-dependency of this phenomenon yields a differential equation, which is coupled to the repair function r. To account for hyperthermia, two additional state variables represent the amount of active and inactive repair proteins. Their time dynamics are governed by specific differential equations, and also coupled to the repair function r. This design yields a relatively large number of model parameters: 5 radiobiological parameters and 3 additional parameters to model synergistic effects between radiation and hyperthermia, which need to be optimized using dedicated algorithms.
Since the state variable N0 is tracking clonogenic cells, the MHR model can be used to fit experimental cell survival curves. Although good quality fits of cell survival curves were obtained, unambiguous interpretation of the results can be quite challenging, since very different sets of parameters, representing very different repair dynamics, can fit a set of experimental results almost equally well [Citation57,Citation58]. Furthermore, sometimes unexpected parameter values were found; calibration and validation of the model are thus quite challenging.
Since the MHR model has 8 free parameters and clonogenic assays typically have only 5 data points, additional data are needed for reliable model calibration. To this end, Weyland et al. implemented methods to map the model to comet assay readouts to be combined with clonogenic assay data [Citation58]. Results showed that unambiguous model parameters can only be obtained by combining information from both assays in a holistic approach. However, even with this combination, some parameters remain unidentified. Furthermore, model calibration is computationally expensive, and experimental temperatures did not exceed 42 °C. This implies that the model does not account for direct cytotoxicity by hyperthermia and does not correctly describe inhibition of DNA damage repair at higher temperatures [Citation58].
Although the predictive value of the MHR model is limited, a clear advantage is that it offers a tool for testing various concepts and ideas about the cellular repair process. The cell population-based approach allows the inclusion of the mitotic cycle, with variations in radiosensitivity. This can be extended to a tumor model with subpopulations of different radiosensitivity, which can help understand tumor response.
3.2.2. Artificial immune − tumor ecosystem
To investigate the dynamic behavior of immune-tumor ecosystems in different scenarios, Scheidegger et al. developed a dedicated model representing an artificial adaptive immune system [Citation59]. The artificial immune-tumor ecosystem consists of two major components: a tumor-host ecosystem with host tissue and immune cells, and a perceptron for antigen pattern recognition. The combination of a danger signal and antigens then activate the immune system. Danger signal generation is assumed to be proportional to the amount of necrotic or immune system-activating apoptotic cells. Hyperthermia-induced radio-sensitization and increased blood perfusion are accounted for by basic biophysical models. The dynamic interactions are described by differential equations; for a detailed description, the reader is referred to Scheidegger et al. [Citation59].
Results of simulations for combined radiation and hyperthermia suggested that the main effect of hyperthermia is related to radiosensitization and no tumor vaccination effect was observed. However, conclusions are restricted to the investigated scenarios and the proposed artificial immune–tumor ecosystem. As for the multi-hit repair model, this model is also not primarily meant as a predictive model covering all relevant aspects of therapy response, but more as a tool to evaluate ideas about dynamics and to generate and refine hypotheses. A drawback also of this model is that a similar behavior of the system is observed for a large range of very different parameter values.
3.2.3. The temperature dependent LQ model
In radiotherapy, the LQ-model is commonly used for biological modeling. The standard LQ-model is defined as [Citation60,Citation61]:
(6)
(6)
In EquationEquation (6)(6)
(6) α and β are the coefficients of the non-repairable and the repairable components of radiation damage respectively [Citation62]. The β-component represents both misrepair and repair: a high value of β indicates that the cell is good at both. The degree of curvature is frequently defined in terms of the α/β ratio. For a low α/β ratio, the survival curve falls rapidly, which is characteristic of slowly proliferating cells [Citation61,Citation62]. The β-component fades with a half-time of minutes or hours so that a very low dose rate is close to the α-curve [Citation62].
The shape of the cell survival curve after radiotherapy combined with hyperthermia is very similar to the shape of the survival curve for radiotherapy alone (). Therefore, the LQ model, as used in radiotherapy, can be used to describe these survival curves, with adjusted LQ-parameters [Citation63,Citation64]:
(7)
(7)
With α* and β* adjusted temperature-dependent LQ-parameters to reflect the radiosensitizing effect of hyperthermia on cell survival. Xu et al. found that simultaneous radiation and heating of (heat resistant) human colon adenocarcinoma cells for 1 h at 41.1 °C resulted in a 2.5–3 fold increase in the parameter α, without a significant change in the β parameter [Citation64]. Sequential heating immediately after radiation for 2 h was needed to realize the same effect.
Using this model, Myerson et al. evaluated response rates for a cohort of 60 patients treated with radiotherapy and simultaneous superficial hyperthermia, as well as 4 randomized studies using superficial hyperthermia (the ESHO melanoma trial [Citation15] and 3 recurrent breast cancer trials reported by Vernon et al. [Citation65]) [Citation63]. Assuming α*= α + Δα, and β*=β, it was observed that the incidence of complete response as a function of the number of effective hyperthermia sessions could be fitted with a Δα of about 0.05–0.1 Gy−1 [Citation63].
Franken et al. reviewed the modulation of the LQ-parameters for several radiosensitizing agents, including hyperthermia [Citation66]. Radiation plus hyperthermia for 1 h at 41 °C and 43 °C was evaluated for human cervical cancer, colon cancer and lung cancer cell lines and LQ-parameters were determined. It was found that 1 h at 41 °C mainly increased the β parameter, while 1 h at 43 °C increased both α and β. An increase of β consequently lowered the α/β ratio, which makes the tumor more sensitive to higher fraction doses.
Van Leeuwen et al. used a temperature-dependent LQ-model, with a term added to account for direct cell kill, to generate a model that describes cell survival as a function of radiation dose, temperature and time interval [Citation67]. To this end, cell survival assays were performed at various combinations of radiation dose (0–8 Gy), temperature (37–42 °C for a standard treatment duration of 60 min), time interval (0–4 h) and treatment sequence (hyperthermia before/after radiotherapy) for two human cervical cancer cell lines. The LQ-model was fitted to the data using maximum likelihood estimation. The model described the dependency of cell survival on the dose, temperature and time interval very well (R2 = 0.9), which makes it suitable for biological modeling and evaluation of the impact of different temperatures and time intervals as observed in the clinic.
3.2.4. The AlphaR model
Brüningk et al. proposed the AlphaR model, an empirical cell survival model for heat-induced radiosensitization by DNA-repair inhibition, adapted from the LQ-model [Citation68]. The AlphaR model calculates the surviving fraction by:
(8a)
(8a)
(8b)
(8b)
Here, the parameter α0 reflects cellular damage, αR the damage repair and β the reduction of repair capacity with increasing dose. The model assumes that damage repair is only possible up to a threshold dose D = αR/(2β), and the survival is then described by an LQ-function with α = α0–αR. The model was fitted to in vitro experimental data with good results for both heating alone and heat combined with radiation. Especially at very high temperatures (43.5–57 °C), when heat is applied without radiation, the AlphaR model correctly describes both the initial shoulder and the exponentially linear decay, while the LQ-model yields an overestimation of the latter. Also for the combined radiation and heat the authors hypothesize that this AlphaR model might be preferable to a conventional LQ-model. They suggest that considering that hyperthermic radiosensitization is due to inhibition of DNA double-strand break repair, the parameter α0 could be assumed constant. An increase of α with thermal dose might then be due to a reduction in the repair capacity, i.e., a reduction of αR. However, the authors were not able to provide data to support this hypothesis. Their analysis was limited to the LQ-arm of the model because the fit of α0 and αR were prone to large uncertainties.
Next, based on the model of Powathil [Citation69,Citation70] and the AlphaR model, Brüningk et al. proposed a hybrid cellular automaton model to predict both the number and distribution of viable cells over time [Citation71]. To allow growth modeling, cells were represented as 2 D or 3 D voxels, with the edge length equal to the cell diameter. These digital cells follow the four-stage cell cycle through G1, S, S1 and M-phases and are assigned to the appropriate stage, depending on a cell cycle timer. When the doubling time is reached, a cell in stage G1 will divide, or enter the reversible quiescent stage G0 when there are no free neighboring spaces. Cell survival after treatment was modeled using the AlphaR model, with a cell cycle-dependent weight factor γ added to account for differences in radiation sensitivity at each stage of the cell cycle (i.e., the exponentials in Equation (8) are multiplied with this factor γ) [Citation71]. Model calibration for the cell cycle distribution and growth requires additional flow cytometry and cellular growth curves. Results showed that this model can predict the dynamic growth of a treated cell population when adequate model parameters have been determined based on in vitro experimental data. This simulation framework was mainly designed to simulate the experimental procedure of an in vitro focused ultrasound experiment, and to analyze the expected treatment response in order to achieve relevant basic knowledge about the promising treatment approach to combine focused ultrasound-mediated heating with radiotherapy.
3.2.5. Thermodynamic modeling based on the LQ-model
As indicated by Myerson et al., the LQ-model could also be applied to evaluate the thermal enhancement ratio (TER) [Citation63]. Suppose that d1 is the fraction dose with hyperthermia and d2 is the fraction dose that, without hyperthermia, would produce the same effect as d1 with hyperthermia. Using EquationEquation (7)(7)
(7) we can write:
(9a)
(9a)
Since TER = d2/d1, this yields:
(9b)
(9b)
which yields a formula of TER in terms of fraction dose d1, α/β ratio and Δα/α:
(9c)
(9c)
and shows that TER decreases with increasing d1, and increases with α/β and Δα/α. The fact that TER decreases with decreasing α/β suggests a therapeutic benefit when thermoradiotherapy is compared with higher doses of radiotherapy. This implies that increasing the radiation dose to realize an equivalent effect to thermoradiation in acutely reacting tumor tissue would lead to more toxicity in late reacting normal tissue with a lower α/β. Such evaluations could be very useful in the design of future prospective trials.
De Mendoza et al. proposed a thermodynamic model for simultaneous application of heat and radiation, in which the TER is related to the cell fraction that is radiosensitized by heat-induced sublethal damage [Citation72]. Hyperthermia-induced damage will elevate cells from an alive state A to a more vulnerable sensitized state A’. The radiation dose DRT+HT to realize the same surviving fraction with hyperthermia is reduced and the LQ-model can then be written as:
(10)
(10)
EquationEquation (10)(10)
(10) has thus modulated LQ-parameters α·TER and β·TER2. The TER is assumed to be dependent on the energy absorbed in this transition from state A to A’:
(11)
(11)
With c1 the baseline of TER and c2 account for the radio- and thermal sensitivity. The function k(T) represents the transition rate from state A to A’ and assumes protein denaturation to be the mechanism responsible for heat-induced cell damage, depending on temperature T, which is modeled by means of Eyring’s transition state theory.
This model provides a theoretical basis to understand hyperthermia-induced radiosensitization. Results showed that sensitization rates were exponentially dependent on temperature, which is in line with empirical observations [Citation72]. Furthermore, it was shown that the model can reproduce clonogenic survival curves, as well as TER values observed in vitro and in vivo.
3.2.6. 3 D equivalent dose prediction
Kok et al. proposed to use the LQ-model with temperature-dependent parameters α and β to estimate the radiosensitization effect by hyperthermia as a 3 D equivalent radiation dose distribution [Citation73]. This equivalent radiation dose is the radiation dose that would be needed without hyperthermia to achieve the same biological effect as the combined radiotherapy and hyperthermia treatment. The model uses voxel-based calculations with local LQ-parameters. These LQ-parameters are affected by the local temperature (T), exposure time and the time interval (tint) between radiotherapy and hyperthermia. Considering a standard 60 min hyperthermia treatment duration, the (tumor) cell surviving fraction can be calculated as:
(12)
(12)
where D (Gy) is the total dose and G is the Lea-Catcheside protraction factor that accounts for repair during irradiation, which approaches 1 for high dose rates. The equivalent radiation dose EQDRT for each voxel can then be calculated as:
(13)
(13)
where α37 and β37 are the ‘regular’ LQ-parameters at 37 °C and dref (Gy) is the fraction size of the reference radiotherapy treatment [Citation73,Citation74]. Based on this concept, the X-Term software has been developed by van Leeuwen et al. [Citation74], which enables 3 D biological evaluation of thermoradiotherapy plans. X-Term also accounts for hyperthermic cytotoxicity using an Arrhenius relationship [Citation67]. The survival fraction according to EquationEquation (12)
(12)
(12) is then multiplied with a term for hyperthermic cytotoxicity (SHT) given by:
(14)
(14)
where t (s) is the heating time, which is typically 60 min (3600s) for standard hyperthermia treatment, and k is the reaction rate as a function of T, given by:
(15)
(15)
with ΔS (cal/°C/mol) the entropy of inactivation and ΔH (cal/mol) is the inactivation energy of the critical rate-limiting molecules that cause cell lethality. Including EquationEquation (15)
(15)
(15) , the equivalent dose EQDRT is then calculated by [Citation67]:
(16)
(16)
By importing a CT image data set, a DICOM structure set and (registered) radiotherapy and hyperthermia dose and temperature distributions, X-Term performs a voxel-wise calculation of the EQDRT, based on the user-defined treatment schedule. Individual model parameters can be assigned to each delineated structure, enabling to include also organs at risk. Results of two treatment schemes are visualized by X-Term, typically radiation alone and radiation plus hyperthermia, but the user can also compare for example the effect of different time intervals. The predicted EQDRT distributions can be evaluated according to conventional radiotherapy planning criteria, i.e., by dose distributions, dose volume histograms and tumor control probability. illustrates the workflow of X-Term. Results based on X-Term using predicted but clinically realistic temperature distributions and temperature-dependent LQ-parameters showed that adding hyperthermia to a radiation treatment schedule is typically equivalent to an extra ∼10 Gy dose escalation to the tumor [Citation73,Citation75].
Figure 3. Workflow of X-Term to predict and evaluate equivalent dose distributions for thermoradiotherapy treatments in patients.
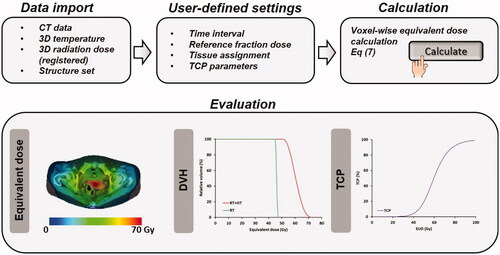
The functionality of X-Term allows qualitative use to predict differences in effectiveness for different treatment strategies, and to investigate the impact on the outcome for relevant clinical questions. Biological modeling can predict the feasibility of hyperthermia as a treatment option, as was done for pediatric patients with in-field recurrent sarcoma in the pelvic region or an extremity after previous irradiation, and limited treatment options [Citation76]. Results indicated that low dose re-irradiation (23x2Gy) plus weekly locoregional hyperthermia could effectively realize a possibly curative equivalent dose of 54 Gy [Citation76]. A clinical feasibility study would be a logical next step to demonstrate validity, although patient numbers will be quite low and thus accrual might be problematic.
Another biological planning study using X-Term suggested hyperthermia can substantially increase the local control rate in high-risk prostate cancer patients [Citation73]. Based on clinical dose-response curves, an extra hyperthermia-equivalent 10 Gy (from 76 to 86 Gy) would increase the local control probability from ∼50% to ∼90% [Citation73]. Several non-randomized clinical studies have been performed for radiotherapy combined with hyperthermia in prostate cancer patients. A recent systematic literature review also concluded that hyperthermia would be a promising treatment strategy to enhance the therapeutic ratio for these patients [Citation77]. Prospective phase II trials are ongoing, e.g., the HT-Prostate trial (NCT0415905), including patients with biochemical relapse after radical prostatectomy. Results of the planned interim analysis (n = 50) showed feasibility; safety criteria were met and a promising short-term prostate-specific antigen response was observed with no significant changes in quality of life [Citation78].
Biological modeling with the X-Term software was also applied to evaluate the impact of the time interval between radiotherapy and hyperthermia treatments. Investigating this in a prospective clinical trial is ethically quite difficult, and biological modeling provides an excellent tool to answer such questions. A biological planning study in cervical cancer patients showed that the thermal enhancement ratio (TER) decreases with increasing time interval (range 1.16–1.05), while the TER for organs at risk remained rather constant and close to 1, independent of the time interval [Citation79]. Thus, the shorter the time interval, the larger the therapeutic gain [Citation79]. This conclusion was supported by a retrospective analysis of clinical data, which showed a 3-year in-field recurrence rate of 18% and 53% in the short (≤79.2 min) and long (>79.2 min) time-interval group, respectively; the 5-year overall survival was 52% and 17%, respectively [Citation28]. However, another large retrospective analysis by Kroesen et al. could not demonstrate an impact of the time interval [Citation80]. A possible explanation could be that inhibition of DNA damage repair was exploited less in their cohort due to either different patient selection, lower temperature levels and/or longer time intervals achieved. Direct comparison was difficult as the definition of time interval was different (time until steady state versus start power on), affecting the interpretation; scientific discussions on this topic are still ongoing [Citation81–83]. Nevertheless, hyperthermia exhibits multiple other, less time-dependent working mechanisms besides inhibition of DNA repair. Thus a clinically relevant benefit of hyperthermia was observed also for patients treated with longer time intervals [Citation13].
Ghaderi Aram et al. implemented a similar approach for 3 D voxel-wise biologically equivalent dose (BED, i.e., EQDRT multiplied with the relative effectiveness) calculation to evaluate the potential of combined Gamma Knife radiosurgery and hyperthermia for pediatric neuro-oncology [Citation84]. A single medulloblastoma patient case was evaluated for stereotactic radiotherapy combined with a dedicated microwave device for brain tumors that is currently under development [Citation85]. Different α and β parameter distributions were applied based on clinically representative pO2 histograms, to represent the LQ-parameters for generic, well-oxygenated, moderately and poorly oxygenated tumors. Evaluation of BED suggested the feasibility of this treatment approach, with an increase of typically ∼10 Gy by hyperthermia [Citation84].
Androulakis et al. implemented the EQDRT-based biological modeling approach for high-dose-rate (HDR) brachytherapy combined with simultaneous interstitial hyperthermia for prostate cancer [Citation86]. In addition, rather than optimizing both treatment modalities individually, they developed a method to optimize the temperature distribution, based on the expected EQDRT of the combined treatment. This allows for treatment planning using radiotherapeutic dose constraints and objectives, which makes the combined treatment plan easier to interpret. Theoretical evaluation of this method was performed using implant geometries, anatomical data and clinical HDR brachytherapy dose distributions of 10 previously treated patients. Results suggested that using biological optimization to optimize the temperature distribution would permit realization of the same equivalent prostate target dose with 80% of the original HDR brachytherapy dose, together with a significantly lower EQDRT in organs at risk (urethra, rectum and bladder) [Citation86]. This is a promising tool for further development of interstitial hyperthermia in combination with HDR brachytherapy.
4. Future developments toward routine clinical use
The work described in the previous sections shows there has been significant progress in biological modeling for hyperthermia combined with radiotherapy, resulting in valuable models that help to improve understanding the effects of thermoradiotherapy treatments. Since complex combinations of dose, sequencing and timing of thermoradiotherapy treatments are of utmost importance to realize maximal tumor control and minimal normal tissue toxicity, advanced and robust biological treatment planning models are indispensable.
Future research will focus on the further development of such models, eventually aiming at routine clinical use and at personalized treatment using biological treatment planning to optimize the effectiveness of thermoradiotherapy treatments. Realizing this goal requires a multi-disciplinary approach combining biology, physics and clinical aspects with cross-disciplinary feedback (). To this end, the large European Hyperboost consortium has been initiated (European Horizon 2020 MSCA-Innovative training network grant 955625). The research as schematically shown in will be performed within this consortium and the main strategies will be discussed in the next paragraphs.
Figure 4. Multidisciplinary research combining biology, physics and clinical aspects will lead to personalized treatment using biological modeling to optimize thermoradiotherapy treatments. This reflects the ongoing research within the large European Hyperboost consortium (European Horizon 2020 MSCA-Innovative training network grant 955625).
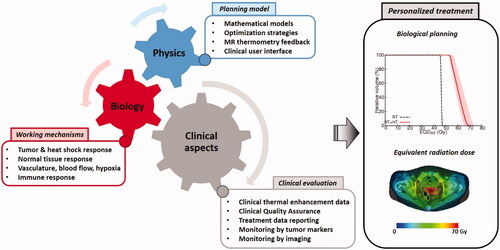
Most existing thermal enhancement data presently used in biological models were derived from in vitro experiments. Such experiments often yield thermal enhancement data at one or two specific temperature levels, but since clinical temperature distributions are heterogeneous, 3 D equivalent dose calculations require extensive data at various temperature levels in the hyperthermic range and multiple clinically relevant time intervals. Only an extensive data set allows the derivation of a detailed mathematical model, describing the dependency of cell survival on radiation dose, temperature/thermal dose and time interval, as basic input for equivalent dose calculations. Such a model has been developed for cervical cancer [Citation67], and a first mandatory step toward more extensive use of biological models in treatment planning would be generating extensive experimental (in vitro/in vivo) data for other tumor sites regularly treated with hyperthermia, e.g., breast, head & neck, pancreas, rectum and bladder, including the normal tissues at risk in these regions. Combination with clinical thermal enhancement data when available would further improve reliability.
Once extensive thermal enhancement data are available for tumor sites treated with radiotherapy and hyperthermia, a standard clinical application of basic biological modeling becomes feasible. Predicted equivalent doses based on measured temperatures and reported time intervals could provide very relevant insight into the quality of different treatment series and treatment sessions. Standard clinical thermometry is based on data acquired with a limited number of thermometry probes implanted in or near the tumor target, as prescribed in the QA guidelines [Citation87,Citation88]. Assuming a relatively uniform radiotherapy dose distribution, reported indexed temperatures T10, T50 and T90 could be used to calculate an estimate of the equivalent dose EQDRT10, EQDRT50 and EQDRT90. If treatment operators have additional treatment planning and/or MR thermometry data available, more detailed 3-D temperature distribution information could then be used to further improve the accuracy of the equivalent dose estimate.
Mechanisms incorporated in the biological models so far were typically limited to DNA-damage repair inhibition and direct cell kill, as these are more easily determined in in vitro tumor models. Although these mechanisms are very important, the incorporation of other mechanisms is essential to realize higher accuracy and personalized treatment (planning). This also requires further research on understanding and quantifying the timing/dose-effect relationship of other relevant mechanisms, such as heat shock response, normal tissue response, vascular effects, blood flow, hypoxia and immunological effects. Dedicated in vivo studies are required to assess the contribution of each mechanism, depending on temperature/thermal dose and timing of hyperthermia. This should involve quantification of multiple clinically relevant end-points in several tumor sites, as well as in normal tissues. Some of this research is foreseen in the framework of Hyperboost.
Incorporating other hyperthermia working mechanisms in biological prediction models is very challenging because of the complex pleiotropic nature of interactions and the different time scales for tumor and normal tissue responses. Also, the incorporation of e.g., hypoxia, re-oxygenation and immune response in biological models is so complex that it is still a subject of research to establish and incorporate those effects for radiotherapy alone. Although the LQ-model can be extended to account for re-oxygenation [Citation89], additional parameters increase complexity, and thereby the number of experiments needed to derive reliable input data. Nahum et al. presented a practical approach to characterize radioresistance in hypoxic tissue by adapted LQ-parameters and especially significantly lower α-values [Citation90]. The first approach to model the effect of hyperthermia on chronic hypoxic tumors in clinical applications could then be to define a reduction of the hypoxic fraction, with specific LQ-parameters for aerobic and hypoxic regions. This would also require additional imaging to visualize the hypoxic fraction, e.g., by PET imaging using a hypoxia tracer, or by blood oxygenation level-dependent (BOLD) MRI [Citation91,Citation92]. and, preferably, also the dynamics over the treatment course. For example, using Cu-ATSM PET imaging it could be possible to determine the effect of hyperthermia on tumor hypoxia, independent of the increase of perfusion [Citation93]. Adequate incorporation of cyclic tumor hypoxia in biological prediction models will be much more challenging, also because our understanding of cyclic hypoxia processes is still relatively limited and therefore also the clinical consequences. Pre-clinical data showed that biological response to cyclic conditions differs from chronic hypoxia [Citation29], so fluctuations in oxygen levels do have very important biological consequences. Interestingly, glioblastomas irradiated in mice exposed to cyclic hypoxia were more radioresistant compared to the controls with chronic hypoxia [Citation29,Citation94].
Several studies suggest that re-oxygenation after hyperthermia, which lasts up to 24–48 h, is not only caused by increased blood flow but also by a decrease in oxygen consumption, possibly due to heat-induced tumor cell death or a heat-induced change in tumor metabolism [Citation30,Citation35,Citation95–97]. These dual effects are likely caused by the generally heterogeneous temperature distributions achieved in clinical hyperthermia. The relatively low temperatures will then enhance perfusion, thereby causing re-oxygenation, while temperatures at the higher end of the range directly kill chronic hypoxic cells, which reduces oxygen consumption [Citation35]. These effects are difficult to study in classical in vivo experiments, since these typically use water-bath heating, yielding a homogeneous tumor temperature distribution. To improve the clinical translation of pre-clinical research, it would thus be essential to mimic clinical heating in in vivo experiments, including realistic heterogeneous temperature distributions. To that end, realistic miniature heating equipment has been developed, e.g., the ALBA micro8 phased array system (Med-Logix SRL, Rome, Italy), which enables realistic locoregional hyperthermia treatments in mice models. Such dedicated heating equipment allows the design of pre-clinical experiments to further elucidate the impact of different mechanisms on local tumor control. Translating the three-dimensional intratumoral variation in biological effects of hyperthermia into mathematical models will be extremely challenging, though important when modeling is eventually aiming to predict treatment outcomes under realistic conditions.
To account also for systemic tumor control, the immune response should be included in the prediction models, which is perhaps also one of the most challenging aspects, since tumor interactions with the immune system are very complex and also not yet completely understood. Recent research on breast cancer showed that thermoradiotherapy upregulates immune suppressive immune checkpoint molecules [Citation49]. The sequence of hyperthermia and radiotherapy did not strongly affect the immune phenotype [Citation49]. Dedicated pre-clinical experiments will be needed to further determine the response of tumor-infiltrating immune cells for various tumors to different thermoradiotherapy combinations. For example, analyzing the activation of natural killer cells, and the ability of dendritic cells to induce T cell activation/suppression would provide valuable insights. The release of damage-associated molecular patterns (DAMPs) on exposure of cells to thermoradiotherapy could be quantified and used in more advanced biological models. Furthermore, patient biopsies, serum and plasma could be used for the analysis of alarmins such as Hsp70, HMGB1 and Calreticulin by immunohistochemistry and DAMPs could be evaluated in the tumor and peripheral blood of patients. This could eventually provide a personalized immune status for patients treated with hyperthermia.
As mentioned earlier, interactions can be very complex; e.g., the immunosuppressive state of the tumor microenvironment is also supported by hypoxia [Citation9]. Because of the complex interactions and the dynamics of the mechanisms, ideally, models should account for the dynamics of thermoregulation and hypoxia changes, and process feedback data of hypoxia imaging, temperature and immune markers during hyperthermia treatment sessions and treatment series. Noninvasive MR imaging during treatment could provide very valuable additional information to support this. Dedicated MR methods can be applied to map perfusion and hypoxia, but also 3 D temperature (estimations) during treatment [Citation91,Citation98,Citation99]. These data can be very useful to further improve the quantitative reliability of temperature predictions by hyperthermia treatment planning, using the advanced discrete vasculature (DIVA) thermal model [Citation100,Citation101] and also to improve the reliability of on-line adaptive strategies during hyperthermia treatment [Citation102,Citation103]. Furthermore, feedback by tumor and immune response monitoring during the treatment course will permit individualized re-planning during the treatment course and show the effective and dynamic contribution of each hyperthermia working mechanism for individual patients.
Next to sophisticated biological planning models to predict clinical outcomes for various treatment strategies, another important step would be combined optimization of the thermoradiotherapy treatment, instead of optimizing each treatment modality separately. Androulakis et al. recently showed that when biological modeling is used to optimize the temperature distribution, the prostate HDR brachytherapy dose could be reduced by 20%, together with a significantly lower EQDRT in neighboring organs at risk [Citation86]. This indicates that joint multi-objective optimization can be expected to further improve treatment quality. Sophisticated robust optimization strategies are essential to ensure treatment outcome, and this will be very challenging, especially with external beam radiotherapy. Patient-specific optimization is aimed at, capable of dynamic adaptive strategies based on feedback data during hyperthermia treatment, as discussed above.
Such advanced planning tools, embedded in a user-friendly graphical user interface, would eventually minimize, or even eliminate, the current user-dependent quality of hyperthermia treatments. Temperatures achieved during treatments can show a large variation, and for example for superficial heating of breast cancer recurrences, the minimum goal temperatures as indicated in the quality assurance (QA) guidelines (T90 > 40 °C and T50 > 41 °C) are not always realized [Citation11,Citation88]. However, a high temperature is associated with significantly higher locoregional control [Citation11]. Reliable planning can effectively guide the use of hyperthermia in mainstream clinics and it could also help to reach a consensus about the optimal thermoradiotherapy treatment schedules, thereby homogenizing treatment protocols. To realize this, QA guidelines are essential to achieve better-controlled temperature distributions, with more uniform and higher EQDRT and less normal tissue hot spots. Clinical implementation of thermoradiotherapy planning requires tight QA protocols to ensure that the actual settings of the heating device match the planning prescription. Furthermore, sufficient technical and clinical data should be reported to allow adequate analysis, thereby avoiding also institutional variation in the calculation of thermal dose parameters. This requires consensus on guidelines for combined thermoradiotherapy treatment data reporting suitable for multi-center clinical trials, and guidelines on thermal dose reporting to accurately determine the effective equivalent dose during treatments.
These expected developments will eventually set the stage for clinical (registration) studies on the clinical benefit of personalized thermoradiotherapy planning. Biological treatment planning may become very useful to guide further clinical studies in hyperthermia, e.g., as an alternative to dose escalation. The expected outcome of hyperthermia-mediated vs. standard dose escalation can be estimated using advanced biological models. Biological treatment planning can also be very useful to investigate the possible effectiveness of hyperthermia for different patient cohorts and thereby aid the design of promising feasibility studies to further expand the role of hyperthermia in clinical oncology. Next, extension toward modeling the combination of hyperthermia and proton therapy, which could mimic the effect of carbon ion therapy, would be a logical step further [Citation7,Citation104], but also modeling triple-modality treatment strategies, e,g, adding chemotherapy, poly(ADP-ribose) polymerase-1 (PARP-1) inhibitors, immune checkpoint inhibitors, or halogenated pyrimidines [Citation49,Citation105,Citation106].
Especially evaluation of thermochemoradiation (i.e., hyperthermia, chemotherapy and radiotherapy) would be a very valuable next step, since an increasing number of standard treatment protocols include both radiotherapy and chemotherapy. Expressing the effect of chemoradiation as an equivalent radiation dose is relatively straightforward [Citation107], but when adding hyperthermia the synergy with both radiation and chemotherapy should be taken into account [Citation108]. Such biological models can eventually be expected to be very useful to further optimize treatment effectiveness and to guide further clinical studies.
5. Conclusion
Significant progress has been made in understanding the biological mechanisms of hyperthermia and developing adequate mathematical formulations to predict hyperthermic effects in combined treatments with radiotherapy. Patient-specific 3 D equivalent dose predictions allow for prediction of differences in effectiveness for different treatment strategies, and to answer relevant clinical questions. Mechanisms incorporated in the biological models so far were typically limited to DNA-damage repair inhibition and direct cell kill. Further research will focus on including other important mechanisms in advanced biological planning models, such as re-oxygenation in hypoxic tumors and immune response, eventually aiming for integrated radiotherapy + hyperthermia planning to achieve joint temperature/radiotherapy dose optimization for the combined treatment. In the future, when adequate temperature-dependent LQ-parameters will be available for a large number of tumor sites and normal tissues, biological modeling can be expected to be of great clinical importance to further optimize the effectiveness of combined radiotherapy and hyperthermia treatments and to guide further clinical studies.
Disclosure statement
No potential conflict of interest was reported by the author(s).
Additional information
Funding
References
- Sanchez-Nieto B, Nahum AE. BIOPLAN: software for the biological evaluation of radiotherapy treatment plans. Med Dosim. 2000;25(2):71–76.
- Sanchez-Nieto B, Nahum AE. The Delta-TCP concept: a clinically useful measure of tumor control probability. Int J Radiat Oncol Biol Phys. 1999;44(2):369–380.
- Sanchez-Nieto B, Nahum AE, Dearnaley DP. Individualization of dose prescription based on normal-tissue dose-volume and radiosensitivity data. Int J Radiat Oncol Biol Phys. 2001;49(2):487–499.
- Jones B, Dale RG, Deehan C, et al. The role of biologically effective dose (BED) in clinical oncology. Clin Oncol. 2001;13(2):71–81.
- Stavrev PV, Stavreva N, Ruggieri R, et al. Theoretical investigation of the impact of different timing schemes in hypofractionated radiotherapy. Med Phys. 2021;48(7):4085–4098.
- Peeken JC, Vaupel P, Combs SE. Integrating hyperthermia into modern radiation oncology: what evidence is necessary? Front Oncol. 2017;7:132.
- Datta NR, Kok HP, Crezee H, et al. Integrating loco-regional hyperthermia Into the current oncology practice: SWOT and TOWS analyses. Front Oncol. 2020;10(819):819.
- Kampinga HH, Dikomey E. Hyperthermic radiosensitization: mode of action and clinical relevance. Int J Radiat Biol. 2001;77(4):399–408.
- Repasky EA, Evans SS, Dewhirst MW. Temperature matters! And why it should matter to tumor immunologists. Cancer Immunol Res. 2013;1(4):210–216.
- van Rhoon GC. Is CEM43 still a relevant thermal dose parameter for hyperthermia treatment monitoring? Int J Hyperth. 2016;32(1):50–62.
- Bakker A, Tello Valverde CP, van Tienhoven G, et al. Post-operative re-irradiation with hyperthermia in locoregional breast cancer recurrence: temperature matters. Radiother Oncol. 2022;167:149–157.
- Bakker A, Van der Zee J, van tienhoven G, et al. Temperature and thermal dose during radiotherapy and hyperthermia for recurrent breast cancer are related to clinical outcome and thermal toxicity: a systematic review. Int J Hyperth. 2019;36(1):1024–1039.
- Kroesen M, Mulder HT, Van Holthe JML, et al. Confirmation of thermal dose as a predictor of local control in cervical carcinoma patients treated with state-of-the-art radiation therapy and hyperthermia. Radiother Oncol. 2019;140:150–158.
- Rau B, Wust P, Tilly W, et al. Preoperative radiochemotherapy in locally advanced or recurrent rectal cancer: regional radiofrequency hyperthermia correlates with clinical parameters. Int J Radiat Oncol Biol Phys. 2000;48(2):381–391.
- Overgaard J, Gonzalez DG, Hulshof MCCH, et al. Hyperthermia as an adjuvant to radiation therapy of recurrent or metastatic malignant melanoma. A multicentre randomized trial by the European Society for Hyperthermic Oncology. Int J Hyperth. 1996;12(1):3–20.
- Ohguri T, Harima Y, Imada H, et al. Relationships between thermal dose parameters and the efficacy of definitive chemoradiotherapy plus regional hyperthermia in the treatment of locally advanced cervical cancer: data from a multicentre randomised clinical trial. Int J Hyperth. 2018;34(4):461–468.
- Franckena M, Fatehi D, de Bruijne M, et al. Hyperthermia dose-effect relationship in 420 patients with cervical cancer treated with combined radiotherapy and hyperthermia. Eur J Cancer. 2009;45(11):1969–1978.
- Sapareto SA, Dewey WC. Thermal dose determination in cancer therapy. Int J Radiat Oncol Biol Phys. 1984;10(6):787–800.
- de Bruijne M, van der Holt B, van Rhoon GC, et al. Evaluation of CEM43 degrees CT90 thermal dose in superficial hyperthermia: a retrospective analysis. Strahlenther Onkol. 2010;186(8):436–443.
- Overgaard J. Simultaneous and sequential hyperthermia and radiation treatment of an experimental tumor and its surrounding normal tissue in vivo. Int J Radiat Oncol Biol Phys. 1980;6(11):1507–1517.
- Issels R, Kampmann E, Kanaar R, et al. Hallmarks of hyperthermia in driving the future of clinical hyperthermia as targeted therapy: translation into clinical application. Int J Hyperth. 2016;32(1):89–95.
- Gerweck LE, Nygaard TG, Burlett M. Response of cells to hyperthermia under acute and chronic hypoxic conditions. Cancer Res. 1979;39(3):966–972.
- Suit HD, Gerweck LE. Potential for hyperthermia and radiation therapy. Cancer Res. 1979;39(6 Pt 2):2290–2298.
- Kampinga HH. Cell biological effects of hyperthermia alone or combined with radiation or drugs: a short introduction to newcomers in the field. Int J Hyperth. 2006;22(3):191–196.
- Ihara M, Takeshita S, Okaichi K, et al. Heat exposure enhances radiosensitivity by depressing DNA-PK kinase activity during double strand break repair. Int J Hyperth. 2014;30(2):102–109.
- Krawczyk PM, Eppink B, Essers J, et al. Mild hyperthermia inhibits homologous recombination, induces BRCA2 degradation, and sensitizes cancer cells to poly (ADP-ribose) polymerase-1 inhibition. Proc Natl Acad Sci USA. 2011;108(24):9851–9856.
- van den Tempel N, Laffeber C, Odijk H, et al. The effect of thermal dose on hyperthermia-mediated inhibition of DNA repair through homologous recombination. Oncotarget. 2017;8(27):44593–44604.
- Van Leeuwen CM, Oei AL, Chin KWTK, et al. A short time interval between radiotherapy and hyperthermia reduces in-field recurrence and mortality in women with advanced cervical cancer. Radiat Oncol. 2017;12(1):75.
- Bader SB, Dewhirst MW, Hammond EM. Cyclic hypoxia: an update on its characteristics, methods to measure it and biological implications in cancer. Cancers. 2020;13(1):23.
- Vujaskovic Z, Song CW. Physiological mechanisms underlying heat-induced radiosensitization. Int J Hyperth. 2004;20(2):163–174.
- Winslow TB, Eranki A, Ullas S, et al. A pilot study of the effects of mild systemic heating on human head and neck tumour xenografts: analysis of tumour perfusion, interstitial fluid pressure, hypoxia and efficacy of radiation therapy. Int J Hyperth. 2015;31(6):693–701.
- Song CW, Shakil A, Osborn JL, et al. Tumour oxygenation is increased by hyperthermia at mild temperatures. Int J Hyperth. 1996;12(3):367–373.
- Song CW. Effect of local hyperthermia on blood flow and microenvironment: a review. Cancer Res. 1984;44(10 Suppl):4721s–4730s.
- Dewhirst MW, Vujaskovic Z, Jones E, et al. Re-setting the biologic rationale for thermal therapy. Int J Hyperth. 2005;21(8):779–790.
- Dewhirst MW, Oleson JR, Kirkpatrick J, et al. Accurate three-dimensional thermal dosimetry and assessment of physiologic response are essential for optimizing thermoradiotherapy. Cancers. 2022;14(7):1701.
- Toraya-Brown S, Fiering S. Local tumour hyperthermia as immunotherapy for metastatic cancer. Int J Hyperth. 2014;30(8):531–539.
- Multhoff G, Hightower LE. Cell surface expression of heat shock proteins and the immune response. Cell Stress Chaper. 1996;1(3):167–176.
- Ostberg JR, Dayanc BE, Yuan M, et al. Enhancement of natural killer (NK) cell cytotoxicity by fever-range thermal stress is dependent on NKG2D function and is associated with plasma membrane NKG2D clustering and increased expression of MICA on target cells. J Leukoc Biol. 2007;82(5):1322–1331.
- Suzue K, Zhou X, Eisen HN, et al. Heat shock fusion proteins as vehicles for antigen delivery into the major histocompatibility complex class I presentation pathway. Proc Natl Acad Sci USA. 1997;94(24):13146–13151.
- Fisher DT, Chen Q, Skitzki JJ, et al. IL-6 trans-signaling licenses mouse and human tumor microvascular gateways for trafficking of cytotoxic T cells. J Clin Invest. 2011;121(10):3846–3859.
- Seynhaeve ALB, Amin M, Haemmerich D, et al. Hyperthermia and smart drug delivery systems for solid tumor therapy. Adv Drug Deliv Rev. 2020;163-164:125–144.
- Werthmoller N, Frey B, Ruckert M, et al. Combination of ionising radiation with hyperthermia increases the immunogenic potential of B16-F10 melanoma cells in vitro and in vivo. Int J Hyperth. 2016;32(1):23–30.
- Ruckert M, Deloch L, Fietkau R, et al. Immune modulatory effects of radiotherapy as basis for well-reasoned radioimmunotherapies. Strahlenther Onkol. 2018;194(6):509–519.
- Adnan A, Munoz NM, Prakash P, et al. Hyperthermia and tumor immunity. Cancers. 2021;13(11):2507.
- Bull JMC. A review of immune therapy in cancer and a question: can thermal therapy increase tumor response? Int J Hyperth. 2018;34(6):840–852.
- Hader M, Frey B, Fietkau R, et al. Immune biological rationales for the design of combined radio- and immunotherapies. Cancer Immunol Immunother. 2020;69(2):293–306.
- Mahmood J, Shukla HD, Soman S, et al. Immunotherapy, radiotherapy, and hyperthermia: a combined therapeutic approach in pancreatic cancer treatment. Cancers. 2018;10(12):469.
- Hurwitz MD. Hyperthermia and immunotherapy: clinical opportunities. Int J Hyperth. 2019;36(sup1):4–9.
- Sengedorj A, Hader M, Heger L, et al. The effect of hyperthermia and radiotherapy sequence on cancer cell death and the immune phenotype of breast cancer cells. Cancers. 2022;14(9):2050.
- Deckers R, Debeissat C, Fortin PY, et al. Arrhenius analysis of the relationship between hyperthermia and Hsp70 promoter activation: a comparison between ex vivo and in vivo data. Int J Hyperth. 2012;28(5):441–450.
- Moritz AR, Henriques FC. Studies of thermal injury: II. The relative importance of time and surface temperature in the causation of cutaneous burns. Am J Pathol. 1947;23(5):695–720.
- Pearce JA. Comparative analysis of mathematical models of cell death and thermal damage processes. Int J Hyperth. 2013;29(4):262–280.
- Jung H. A generalized concept for cell killing by heat. Radiat Res. 1986;106(1):56–72.
- Roizin-Towle L, Pirro JP. The response of human and rodent cells to hyperthermia. Int J Radiat Oncol Biol Phys. 1991;20(4):751–756.
- Jung H. A generalized concept for cell killing by heat. Effect of chronically induced thermotolerance. Radiat Res. 1991;127(3):235–242.
- Roti JL, Henle KJ. Comparison of two mathematical models for describing heat-induced cell killing. Radiat Res. 1980;81(3):374–383.
- Scheidegger S, Fuchs HU, Zaugg K, et al. Using state variables to model the response of tumour cells to radiation and heat: a novel multi-hit-repair approach. Comput Math Methods Med. 2013;2013:587543.
- Weyland MS, Thumser-Henner P, Nytko KJ, et al. Holistic view on cell survival and DNA damage: how model-based data analysis supports exploration of dynamics in biological systems. Comput Math Methods Med. 2020;2020:5972594.
- Scheidegger S, Barba SM, Gaipl US. Theoretical evaluation of the impact of hyperthermia in combination with radiation therapy in an artificial immune-tumor-ecosystem. Cancers. 2021;13(22):5764.
- Fowler JF. The linear-quadratic formula and progress in fractionated radiotherapy. Br J Radiol. 1989;62(740):679–694.
- McMahon SJ. The linear quadratic model: usage, interpretation and challenges. Phys Med Biol. 2018;64(1):01TR01.
- Fowler JF. Development of radiobiology for oncology-a personal view. Phys Med Biol. 2006;51(13):R263–86.
- Myerson RJ, Roti Roti JL, Moros EG, et al. Modelling heat-induced radiosensitization: clinical implications. Int J Hyperth. 2004;20(2):201–212.
- Xu M, Myerson RJ, Straube WL, et al. Radiosensitization of heat resistant human tumour cells by 1 hour at 41.1 degrees C and its effect on DNA repair. Int J Hyperth. 2002;18(5):385–403.
- Vernon CC, Hand JW, Field SB, et al. Radiotherapy with or without hyperthermia in the treatment of superficial localized breast cancer: results from five randomized controlled trials. International collaborative hyperthermia group. Int J Radiat Oncol Biol Phys. 1996;35:731–744.
- Franken NA, Oei AL, Kok HP, et al. Cell survival and radiosensitisation: modulation of the linear and quadratic parameters of the LQ model (review). Int J Oncol. 2013;42(5):1501–1515.
- Van Leeuwen CM, Oei AL, Ten Cate R, et al. Measurement and analysis of the impact of time-interval, temperature and radiation dose on tumour cell survival and its application in thermoradiotherapy plan evaluation. Int J Hyperth. 2018;34(1):30–38.
- Bruningk SC, Ijaz J, Rivens I, et al. A comprehensive model for heat-induced radio-sensitisation. Int J Hyperth. 2018;34(4):392–402.
- Powathil GG, Adamson DJA, Chaplain MAJ. Towards predicting the response of a solid tumour to chemotherapy and radiotherapy treatments: clinical insights from a computational model. PLOS Comput Biol. 2013;9(7):e1003120.
- Powathil GG, Swat M, Chaplain MAJ. Systems oncology: towards patient-specific treatment regimes informed by multiscale mathematical modelling. Semin Cancer Biol. 2015;30:13–20.
- Bruningk S, Powathil G, Ziegenhein P, et al. Combining radiation with hyperthermia: a multiscale model informed by in vitro experiments. J R Soc Interface. 2018;15(138):20170681.
- De Mendoza AM, Michlikova S, Berger J, et al. Mathematical model for the thermal enhancement of radiation response: thermodynamic approach. Sci Rep. 2021;11(1):5503.
- Kok HP, Crezee J, Franken NAP, et al. Quantifying the combined effect of radiation therapy and hyperthermia in terms of equivalent dose distributions. Int J Radiat Oncol Biol Phys. 2014;88(3):739–745.
- van Leeuwen CM, Crezee J, Oei AL, et al. 3D radiobiological evaluation of combined radiotherapy and hyperthermia treatments. Int J Hyperth. 2017;33(2):160–169.
- Crezee J, van Leeuwen CM, Oei AL, et al. Biological modelling of the radiation dose escalation effect of regional hyperthermia in cervical cancer. Radiat Oncol. 2016;11:14.
- Kok HP, Van Dijk I, Crama KF, et al. Reirradiation plus hyperthermia for recurrent pediatric sarcoma; a simulation study to investigate feasibility. Int J Oncol. 2019;54(1):209–218.
- Le Guevelou J, Chirila ME, Achard V, et al. Combined hyperthermia and radiotherapy for prostate cancer: a systematic review. Int J Hyperth. 2022;39(1):547–556.
- Beck M, Ghadjar P, Mehrhof F, et al. Salvage-Radiation therapy and regional hyperthermia for biochemically recurrent prostate cancer after radical prostatectomy (results of the planned interim analysis). Cancers. 2021;13(5):1133.
- van Leeuwen CM, Crezee J, Oei AL, et al. The effect of time interval between radiotherapy and hyperthermia on planned equivalent radiation dose. Int J Hyperth. 2018;34(7):901–909.
- Kroesen M, Mulder HT, van Holthe JML, et al. The effect of the time interval Between radiation and hyperthermia on clinical outcome in 400 locally advanced cervical carcinoma patients. Front Oncol. 2019;9:134.
- Crezee J, Oei AL, Franken NAP, et al. Response: commentary: the impact of the time interval Between radiation and hyperthermia on clinical outcome in patients with locally advanced cervical cancer. Front Oncol. 2020;10(528):528.
- Crezee H, Kok HP, Oei AL, et al. The impact of the time interval between radiation and hyperthermia on clinical outcome in patients with locally advanced cervical cancer. Front Oncol. 2019;9:412.
- Kroesen M, Mulder HT, van Rhoon GC, et al. Commentary: the impact of the time interval between radiation and hyperthermia on clinical outcome in patients with locally advanced cervical cancer. Front Oncol. 2019;9:1387.
- Ghaderi Aram M, Zanoli M, Nordstrom H, et al. Radiobiological evaluation of combined gamma knife radiosurgery and hyperthermia for pediatric neuro-oncology. Cancers. 2021;13(13):3277.
- Schooneveldt G, Dobsicek Trefna H, Persson M, et al. Hyperthermia treatment planning including convective flow in cerebrospinal fluid for brain tumour hyperthermia treatment using a novel dedicated paediatric brain applicator. Cancers. 2019;11(8):1183.
- Androulakis I, Mestrom RMC, Christianen M, et al. A novel framework for the optimization of simultaneous ThermoBrachyTherapy. Cancers. 2022;14(6):1425.
- Bruggmoser G, Bauchowitz S, Canters R, et al. Quality assurance for clinical studies in regional deep hyperthermia. Strahlenther Onkol. 2011;187(10):605–610.
- Trefna HD, Crezee H, Schmidt M, et al. Quality assurance guidelines for superficial hyperthermia clinical trials: I. Clinical requirements. Int J Hyperth. 2017;33(4):471–482.
- Brenner DJ, Hlatky LR, Hahnfeldt PJ, et al. A convenient extension of the linear-quadratic model to include redistribution and reoxygenation. Int J Radiat Oncol Biol Phys. 1995;32(2):379–390.
- Nahum AE, Movsas B, Horwitz EM, et al. Incorporating clinical measurements of hypoxia into tumor local control modeling of prostate cancer: implications for the alpha/beta ratio. Int J Radiat Oncol Biol Phys. 2003;57(2):391–401.
- Niendorf T, Pohlmann A, Arakelyan K, et al. How bold is blood oxygenation level-dependent (BOLD) magnetic resonance imaging of the kidney? Opportunities, challenges and future directions. Acta Physiol. 2015;213(1):19–38.
- Wijsman R, Kaanders JH, Oyen WJ, et al. Hypoxia and tumor metabolism in radiation oncology: targets visualized by positron emission tomography. Q J Nucl Med Mol Imaging. 2013;57(3):244–256.
- Myerson RJ, Singh AK, Bigott HM, et al. Monitoring the effect of mild hyperthermia on tumour hypoxia by Cu-ATSM PET scanning. Int J Hyperth. 2006;22(2):93–115.
- Hsieh CH, Lee CH, Liang JA, et al. Cycling hypoxia increases U87 glioma cell radioresistance via ROS induced higher and long-term HIF-1 signal transduction activity. Oncol Rep. 2010;24(6):1629–1636.
- Moon EJ, Sonveaux P, Porporato PE, et al. NADPH oxidase-mediated reactive oxygen species production activates hypoxia-inducible factor-1 (HIF-1) via the ERK pathway after hyperthermia treatment. Proc Natl Acad Sci USA. 2010;107(47):20477–20482.
- Vaupel PW, Kelleher DK. Pathophysiological and vascular characteristics of tumours and their importance for hyperthermia: heterogeneity is the key issue. Int J Hyperth. 2010;26(3):211–223.
- Horsman MR, Overgaard J. Can mild hyperthermia improve tumour oxygenation? Int J Hyperth. 1997;13(2):141–147.
- Gellermann J, Hildebrandt B, Issels R, et al. Noninvasive magnetic resonance thermography of soft tissue sarcomas during regional hyperthermia: correlation with response and direct thermometry. Cancer. 2006;107(6):1373–1382.
- Boehm C, Goeger-Neff M, Mulder HT, et al. Susceptibility artifact correction in MR thermometry for monitoring of mild radiofrequency hyperthermia using total field inversion. Magn Reson Med. 2022;88(1):120–132.
- Kok HP, Van den Berg CAT, Bel A, et al. Fast thermal simulations and temperature optimization for hyperthermia treatment planning, including realistic 3D vessel networks. Med Phys. 2013;40(10):103303.
- Kotte AN, van Leeuwen GM, Lagendijk JJ. Modelling the thermal impact of a discrete vessel tree. Phys Med Biol. 1999;44(1):57–74.
- Kok HP, Crezee J. Adapt2Heat: treatment planning-assisted locoregional hyperthermia by on-line visualization, optimization and re-optimization of SAR and temperature distributions. Int J Hyperth. 2022;39(1):265–277.
- Kok HP, Korshuize - van Straten L, Bakker A, et al. On-line adaptive hyperthermia treatment planning during locoregional heating to suppress treatment limiting hot spots. Int J Radiat Oncol Biol Phys. 2017;99(4):1039–1047.
- Datta NR, Puric E, Schneider R, et al. Could hyperthermia with proton therapy mimic carbon ion therapy? Exploring a thermo-radiobiological rationale. Int J Hyperth. 2014;30(7):524–530.
- Franken NA, Barendsen GW. Enhancement of radiation effectiveness by hyperthermia and incorporation of halogenated pyrimidines at low radiation doses as compared with high doses: implications for mechanisms. Int J Radiat Biol. 2014;90(4):313–317.
- Eppink B, Krawczyk PM, Stap J, et al. Hyperthermia-induced DNA repair deficiency suggests novel therapeutic anti-cancer strategies. Int J Hyperth. 2012;28(6):509–517.
- Plataniotis GA, Dale RG. Use of concept of chemotherapy-equivalent biologically effective dose to provide quantitative evaluation of contribution of chemotherapy to local tumor control in chemoradiotherapy cervical cancer trials. Int J Radiat Oncol Biol Phys. 2008;72(5):1538–1543.
- Crezee J, Barendsen GW, Westermann AM, et al. Quantification of the contribution of hyperthermia to results of cervical cancer trials: in regard to plataniotis and dale (int J radiat oncol biol phys 2009;73:1538-1544). Int J Radiat Oncol Biol Phys. 2009;75(2):634–635.