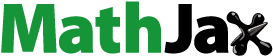
Abstract
Purpose
We studied the differences between planning and treatment position, their impact on the accuracy of hyperthermia treatment planning (HTP) predictions, and the relevance of including true treatment anatomy and position in HTP based on magnetic resonance (MR) images.
Materials and methods
All volunteers were scanned with an MR-compatible hyperthermia device, including a filled waterbolus, to replicate the treatment setup. In the planning setup, the volunteers were scanned without the device to reproduce the imaging in the current HTP. First, we used rigid registration to investigate the patient position displacements between the planning and treatment setup. Second, we performed HTP for the planning anatomy at both positions and the treatment mimicking anatomy to study the effects of positioning and anatomy on the quality of the simulated hyperthermia treatment. Treatment quality was evaluated using SAR-based parameters.
Results
We found an average displacement of 2 cm between planning and treatment positions. These displacements caused average absolute differences of ∼12% for TC25 and 10.4%–15.9% in THQ. Furthermore, we found that including the accurate treatment position and anatomy in treatment planning led to an improvement of 2% in TC25 and 4.6%–10.6% in THQ.
Conclusions
This study showed that precise patient position and anatomy are relevant since these affect the accuracy of HTP predictions. The major part of improved accuracy is related to implementing the correct position of the patient in the applicator. Hence, our study shows a clear incentive to accurately match the patient position in HTP with the actual treatment.
Introduction
During hyperthermia treatment, the temperature of the target volume is increased to sensitize the tumor cells for chemotherapy and radiotherapy [Citation1,Citation2] without increasing late toxicity to the healthy tissue [Citation3–5]. For a group of 420 patients with locally advanced cervical cancer (LACC), Franckena et al. [Citation6] have shown a correlation between the thermal dose and treatment outcome. Recently, Kroesen et al. confirmed this in an independent cohort of 227 patients with LACC [Citation7]. These studies demonstrated the importance of precise heat delivery to improve hyperthermia treatment efficacy. Magnetic resonance (MR) guided hyperthermia has great potential for improving treatment precision since it allows real-time monitoring of the three-dimensional (3D) temperature and continuous thermal-dose optimization. Furthermore, the complete treatment setup can be imaged, allowing position verification, retrospective evaluation of treatment quality, and investigations into the accuracy of hyperthermia treatment modeling. Even though MR-hyperthermia is relatively recent and applied only to a selected group of users, it is proving its potential to facilitate a more adaptive and accurate strategy for treatment [Citation8–12]. While MR thermometry can be helpful to (retrospective) establish treatment quality [Citation13–16], hyperthermia treatment planning (HTP) enables (prospective) prediction and optimization of SAR and thermal dose [Citation17–20]. The accuracy of HTP is dependent on patient modeling uncertainties [Citation21–27], such as the position and anatomy changes between and during the hyperthermia treatments. Several efforts have been made to understand the importance of patient modeling and how the patient should be positioned to get the best treatment quality. However, there are limited assessments about how the patient’s position and anatomy affect treatment planning accuracy. MR-compatible hyperthermia systems give the unique possibility of imaging the complete treatment setup. Consequently, it offers an ideal environment to investigate the impact of treatment anatomy and position on the accuracy and quality of HTP predictions. Note that the benefit of imaging the patient in the real treatment setup to assess the quality and accuracy to adapt the standard plan is still unexplored research in hyperthermia.
HTP starts with generating an anatomical patient model using the segmentation of tissue volumes based on computerized tomography (CT) or MR images. The applicator’s 3 D model is added, and the 3 D electric field distributions per antenna are simulated. The power and phase of the signals applied to each antenna are optimized for maximizing the predicted heat delivery in the tumor without overheating healthy tissues. Note that the HTP description corresponds to the regular treatment planning workflow for regional radiofrequency hyperthermia [Citation19,Citation28]. In HTP, heat delivery can be described as the predicted power absorption, usually expressed in the specific absorption rate (SAR) or the predicted temperature. Several studies have validated a qualitative agreement between HTP simulations and clinical observations [Citation29–31]. HTP-guided steering has become particularly important in adjusting the treatments to hotspots by temperature measurements or patient feedback [Citation32–36]. Unfortunately, there is insufficient information regarding the quantitative accuracy of the current treatment plans because of uncertainties in the modeling parameters, such as position changes and thermal and dielectric properties [Citation37–40]. Hence, it is essential to assess the accuracy of the treatment plans compared to the HT dose applied to the patient.
The generation of the patient model is based on CT or MR images, which are generally taken at the planning stage at least a week before the treatment. In the time between patient imaging and the hyperthermia treatment, anatomy may change: variable bladder filling, differences in the bending of the support hammock, and the patient may be slightly compressed due to the inflation of the surrounding water bolus. In the current clinical protocol, patient positioning is defined in HTP, and before treatment starts, the patient’s position is checked to confirm compliance with HTP. The position is verified by measuring the distances between the body and the hyperthermia device using a measuring tape, laser light, and ultrasound. This process is facilitated by taking an MR scan when using MR-compatible devices. Franckena et al. [Citation35] showed the challenges and influence of the inaccurate patient’s position from the CT-based computer model compared to the real treatment position. Gellermann et al. [Citation41] indicated a dependency between temperature, patient displacement, and evident differences in patient position between hyperthermia treatment sessions. Canters et al. [Citation40] did a simulation study on the impact of patient position on hyperthermia treatment quality. This study indicated that HTP-guided steering was only effective if the patient’s position during the treatment matched the patient’s position in the HTP. All studies did not include the entire treatment setup; thus, all these studies have specific limitations in predictive value. Even though these suggest the importance of correct patient positioning, the true impact of real treatment position and anatomy on the predicted treatment quality is unknown, and the positioning procedure’s accuracy is unclear.
MR–compatible hyperthermia devices are a unique prospect for providing a more personalized hyperthermia treatment since the full hyperthermia treatment setup can be monitored from the start to the end of the treatment. The benefits of this technology in enabling 3 D noninvasive temperature monitoring have been extensively studied [Citation9,Citation42–44]. Besides temperature monitoring, the current treatment protocols include MR imaging at the beginning of the treatment to verify the patient position [Citation13,Citation15]. As mentioned before, Gellermann et al. [Citation41] conducted a retrospective study where the patient positions inside the MR-compatible device were evaluated among several hyperthermia treatments. They concluded that MR imaging of the complete hyperthermia treatment setup offers the opportunity to improve the modeling of the treatment setup. To our knowledge, no study has exploited MR imaging and MR-compatible hyperthermia devices to evaluate patient positioning and anatomy to assess HTP accuracy and the need to adapt the plan to true treatment anatomy and position.
In this simulation study, we evaluated the accuracy of the current HTP predictions compared to the simulated applied HT dose during treatment and the benefit of adapting the treatment based on the true patient position and anatomy. We conducted a healthy volunteer study where the full-body image of volunteers was taken inside and outside the MR-compatible hyperthermia device. We evaluated position displacements between the two setups and, consequently, the impact of the hyperthermia device on the positioning and anatomy. We simulated the administered hyperthermia treatment and assessed the differences between the HTP predictions and the applied HT dose in the simulated treatment. Finally, we assessed the potential value of re-planning based on the true treatment position and anatomy.
Materials and methods
Healthy volunteer study
In total, 14 healthy female volunteers participated in this study and gave written informed consent after the study was explained to them. Our institutional review board approved this study using the protocol 'MRI technology healthy volunteers’ (MEC-2014-096). The examinations were conducted in a 1.5 T GE Signa Excite scanner (General Electric Healthcare, Waukesha, WI, USA) from November 2018 and April 2021. During the experiments, the volunteers were scanned and positioned inside the BSD-2000-3D MR-compatible system (Pyrexar Medical Cop., Salt Lake City, UT, USA) [Citation9,Citation10]. presents the volunteer information, and the same details are given regarding the patients treated in the MR-compatible system [Citation13].
Table 1. Characterization of the volunteers participating in this study and the patients treated with the MR-compatible device.
presents the experiment timeline. The experiment’s two first steps (a and b) consisted of positioning and scanning the volunteer in the hammock without the hyperthermia device to mimic the imaging setup used for the current HTP. We describe the volunteer position in this setup as the planning position. We used the laser light landmark from the MR scanner to position the volunteer so that the pelvic region was in the center of the field of view. In step c, we prepared the treatment setup, in which the height and position of the hammock were not modified. In the last two steps (d and e), we acquired MR images of the volunteer inside the MR-compatible device to reproduce approximately the hyperthermia treatment setup. Thus, we defined this position as the real treatment position. During hyperthermia treatment, an open transurethral catheter is used during treatment to remove urine from the bladder. Hence, we asked the volunteers to go to the bathroom to resemble the patient’s anatomy (empty bladder) before the start of the experiment, as well as an optional time in the middle of the experiment (step c in ). However, in some volunteers, we identified urine in the bladder in the second part (d and e) of the experiment. After conducting the experiments, the acquired MR images were processed to reproduce the HTP and simulate the administered treatment.
Figure 1. Schematic representation of volunteer experiment timeline. The experiment started by (a) positioning the volunteer in the hammock without the hyperthermia device (planning setup); (b) implementing the MR protocol with the volunteer in the planning setup; (c) experiment pause for the volunteer; (d) position the volunteer in the hammock and place the MR-compatible hyperthermia device with a filled water bolus; (e) execute the MR protocol with the volunteer inside the MR-compatible hyperthermia device.

MRI protocol
The MR imaging protocol was optimized for air pockets visualization, motion compensation, soft tissue contrast, and geometrical precision of the applicator position without significant image wrapping. We used two types of sequences in the protocol. The first scan was to visualize the gastrointestinal air; thus, T1-weighted images were acquired using a 3 D spoiled gradient recalled echo (SPGR) pulse sequence. The acquisition parameters were: TE/TR: 0.62/1.44 ms; slice thickness of 5 mm; flip angle of 2°; the spacing between slices was 2.5 mm; the scan duration was 26 s, and the volume of interest (VOI) was 50 × 50 × 60 cm3. Second, a high resolution anatomic image was taken to capture the volunteer position and the anatomic information. These were T2-weighted MR images acquired using the PROPELLER sequence with the following acquisition parameters: TE/TR: 81/4200 ms; slice thickness of 5.5 mm; flip angle of 125°; there was no spacing between slices; and the total scan duration was 7 min. In order to capture the anatomy inside the applicator, a total of seven scans using the PROPELLER sequence, where a cylindrical VOI of 42.0 × 100.5 cm3 was acquired and reconstructed using the AutoBind tool from GE scanner software. Note that this tool was only used for the PROPELLER sequence since the SPGR sequence takes 3 D volumetric acquisition.
presents the images to visualize the gastrointestinal air from different views in two positions. presents the anatomic images with (treatment setup) and without (planning setup) and the hyperthermia device. The images in the planning setup were used to create the standard treatment plans. In contrast, the MR images from the treatment setup will be used to simulate the administrated treatment and mimic a treatment plan for the true treatment anatomy and position.
Figure 2. Illustration of the MR images taken during a volunteer experiment. (a) T1-weighted images were acquired using the 3 D SPGR pulse sequence; (b) T2-weighted images were acquired using the PROPELLER sequence. The top MR images from (a) and (b) capture the planning setup, while the bottom MR images present the treatment setup. For each MR image set, three different views are shown: axial (left image), coronal (middle image), and sagittal (right image).

Treatment modeling
Patient model generation
The first steps to generate a HTP include imaging the patient and segmenting the images into different tissues. The tissue delineation for bone, muscle, lungs and fat was based on thresholding combined with manual segmentation in the Propeller MR images. The software used for delineating was MIM Maestro (MIM Software Inc., USA). Because of the lack of homogeneity of the B1 field, also known as the ‘bias field’, we used a bias field correction tool from MIM Maestro before delineating the tissues. The bias field correction enabled an automatic segmentation tool based on intensity levels. Note that all high water content tissues were assigned as muscle, such as intestines, bladder, and its lumen. As mentioned, the bladder was not empty in some volunteers, and some urine was identified, which was defined as muscle. After delineating the gastrointestinal air in the SPGR MR images, we used MIM Maestro to perform rigid registration to align the two sets of MR images. The air delineation was transferred to the initial tissue segmentation. presents the rigid registration using the MIM Maestro, where the lower-contrast MR images and the air delineation overlap with the high-resolution MR image. Since the time between the two sets of MR images was 3–5 min, we manually corrected the air delineation in some volunteers. The total time to generate the final segmentation, including delineation and registration, was 2.5 h. Note that the delineation of the bone structure was the longest process (∼1.5 h) because of the low tissue contrast in the MR images.
Figure 3. Propeller MR images of a volunteer with the hyperthermia device and the delineated tissues. (a) Rigid registration of gastrointestinal air delineation on top of the Propeller MR image. The red-colored image represents the SPGR MR image; (b) Final segmentation after all tissues were delineated and the GTV and HTV were added. The distances illustrated by the pink arrows are defined during HTP. The images correspond to the middle slice, which is shown in three different views: axial (left), coronal (middle), and sagittal (right).

After the 3 D patient model was generated (), a gross target volume (GTV) and hyperthermia target volume (HTV) was added. The former is the structure used to attribute the tumor properties. The latter covers the GTV plus a margin for microscopic extensions and is used for treatment optimization. These structures were created using the existing structures from HTP of a patient treated with thermoradiotherapy for locally advanced carcinoma at our institution. To improve the variability of treated tumors, an experienced HT medical doctor artificially created GTVs of three different sizes (small, medium, and large), and the corresponding HTV was drawn. The small, medium and large GTV sizes were ∼19.5, 83.6 and 293.5 ml.
At the start of the MR-guided hyperthermia treatments, MR images are taken to verify if the patient’s position is according to HTP. This process involves measuring the distances shown in and verifying if the position complies with HTP. Note that the MR images taken at the start of the treatment had lower spatial resolution and a limited VOI (50 × 50 × 25 cm3) [Citation13]; thus, these images are insufficient to create an accurate HTP. During the positioning procedure, the patient is mainly shifted in the z- and y-direction. Despite any repositioning, the patient model from the HTP is not modified in the current clinical process. We aligned the MR images of the planning setup to the treatment setup using rigid registration to replicate such repositioning of the patient at the start of the treatment. presents the body contours in both setups and how these look when overlapped. As shown in , the pubic symphysis was delineated and served as a reference structure for registration. After registration, the contours from the planning setup were in the treatment position, as shown in . These were used to create an additional treatment plan. Furthermore, this process enabled the evaluation of the impact of the pressure of the water bolus on the anatomy and how these changes affected HTP accuracy.
Figure 4. Schematic representation of the body and pubic symphysis contours before and after rigid registration. (a) Contours in treatment position (treatment setup) and planning position (planning setup). (b) Contours overlapped, and after re-positioning the volunteer to the treatment position. The pubic symphysis was used as a reference structure to perform rigid registration.

Electromagnetic (EM) simulation
The EM field for each antenna was calculated using the Finite-Difference-Time-Domain solver in Sim4life (v6.2 Zurich MedTech AG, Zurich, Switzerland). We used a nonuniform grid in which we considered a maximum grid step of 2.5 mm inside the applicator and a maximum of 10 mm outside the applicator. The EM field per antenna was computed for 1 V sinusoidal signal excitation, and we considered a harmonic signal of 15 periods at 100 MHz. Literature-based dielectric tissue properties were assigned to each delineated tissue, as indicated in .
Table 2. Literature values of EM tissue properties [Citation51, Citation71].
The energy absorbed in each tissue was described by the SAR, which corresponds to the rate at which EM energy is absorbed per unit of mass of tissue:
(1)
(1)
where
(S/m) is the electric conductively,
(kg/m3) is the mass density and
(V/m) is the magnitude of the local electric field vector. Furthermore, the resulting 3 D EM field distributions were imported into the treatment planning software, VEDO, that is, a custom-made tool developed at Erasmus MC [Citation32]. In this process, cubic filtered (cf)-SAR distribution was optimized, which aims at maximizing the Target-Hotspot-Quotient (THQ) [Citation19,Citation45,Citation46], which is expressed as:
(2)
(2)
where
is the average SAR within the HTV and
is the average SAR in the hotspots, that is 50 ml of the healthy tissue with the highest SAR outside the HTV.
HTPs and simulated treatment
We assessed the differences between the HTP predictions and the simulated treatment. Furthermore, we evaluated the benefit of including the treatment position and anatomy to adapt the treatment. In clinical practice, an HTP is conducted to prescribe how to start and adjust the treatment settings during the treatment to deliver an effective SAR. We created three plans and evaluated these compared to the simulated hyperthermia treatment. Plan A1 and Plan A2 represent the best and worst-case scenarios of the current HTP, respectively. We design a treatment plan based on the correct real patient position and anatomy (Plan B), representing the envisioned situation. Finally, we simulated the administered hyperthermia treatment and calculated the applied HT doses using the amplitude and phase settings of the signals per antenna (‘antenna settings’) from the standard HTP (Plan A1 and A2). The applied HT doses were compared with the plan B predictions to assess the need to include the anatomy of the treatment. presents the steps in each scenario which are described below:
Figure 5. Description of the three plans (plan A1, A2 and B) and the applied HT dose during the simulated hyperthermia treatment (applied HT doses A1 and A2). Plan A1 starts with obtaining the patient data without the MR-compatible hyperthermia device. After tissue delineation and generation of the 3 D patient model are performed, SAR-based optimization in which antenna settings are defined. In the last step of the HTP, the SAR distributions are obtained (dose predictions) using the optimized antenna settings. Plan A2 follows the same steps; however, rigid registration is performed to adjust the MR images from the planning position to the treatment position (). In Plan B, the volunteer is scanned with the MR-compatible hyperthermia device and a filled water bolus (treatment setup). In the simulated hyperthermia treatment, the applied HT dose was calculated using Plan A1 and Plan A2 antenna settings. Five SAR distributions were obtained, where three represent the dose predictions from the treatment plans, and two denote the applied HT dose in the simulated treatment. The symbol’s color of the antenna settings denotes the plan in which these were optimized. Overall, Plan A1 and A2 represent the current HTP, Plan B is the idealized treatment plan, and Applied HT doses A1 and A2 denote the current treatment in clinical practice.

Hyperthermia treatment plannings
Plan A1: denotes the standard HTP in which the MR imaging intends to mimic the CT image acquisition performed in clinical practice. We tried to reproduce the patient’s position from the CT-based computer model. Note that the model’s position is presented in the first scheme of .
Plan A2: represents the intended HTP done in the clinic, in which the volunteer’s position is corrected to match the treatment position. Hence, the model used to create the treatment plan is the same as in plan A1; however, the volunteer’s position changed, as shown in the second scheme of .
Plan B: symbolizes what we would consider the ideal treatment plan since it is based on the true patient anatomy and position inside the hyperthermia device.
Simulation of the administered hyperthermia treatments
Applied HT dose A1: characterizes the SAR distribution obtained in the simulated hyperthermia treatment. Hence, the administered hyperthermia treatment was simulated using the settings from the standard plan (plan A1). The full hyperthermia treatment setup was incorporated to assess the applied HT dose when the position and anatomy are not accurately represented.
Applied HT dose A2: corresponds to the SAR distribution acquired during the simulated treatment when the correct positioning was considered during the HTP. The antenna settings used to calculate the applied HT dose are from plan A2, which corresponds to the standard plan after the volunteer was re-position to the treatment position.
Evaluation
Positioning assessment
As shown in , the displacements between planning and treatment positions were done using the body contour. We calculated these displacements using the mean surface distance (MSD) and 95th percentile Hausdorff distance (HD). The former is the average of all the distances between the surface of the body planning position and the real treatment position [Citation47,Citation48], and the latter is the maximum distance between the two contours [Citation49,Citation50]. Since HD is sensitive to outliers, we considered the 95th percentile of the ordered distance measures (95th HD). For 95th HD and MSD, lower values (in cm) correspond to a lower displacement between the compared positions. presents an example of the top and bottom distances between the two contours, and as shown before, the schematic representation can be found in .
Figure 6. (a) Representation of the body contour and pubic symphysis contour in the planning and treatment position (as in ); (b) illustration of the closest distances from all surface points on the body contour in the planning position to the points on body contour in the real treatment position. Note that the top and bottom distances are defined as surface distances. In addition, the x-, y- and z-direction are demonstrated together with the different anatomical planes.

As mentioned before and shown in , we performed rigid registration to align the two body contours. After registration, we re-computed the MSD and 95th HD. We reported the translation in the x-, y- and z-directions to adjust the two sets of MR images in the same position. The translation reported in the x-, y- and z-directions denotes the distance from left to right, posterior to anterior, and inferior to superior. Hence, the same distances were computed when the two contours were aligned, as shown in .
Dosimetric assessment
The SAR-based quality indicators that we used to understand the clinical significance of changes were THQ and target coverage of 25% (TC25) and 50% (TC50), as these have been related to temperature or clinical outcome [Citation19,Citation24,Citation46]. TC25 and TC50 are defined as the percentage of the HTV in which the SAR level is higher than 25% and 50% of the maximum SAR. For THQ, we quantified the relative difference, while for TC25 and TC50, we calculated the difference.
(4)
(4)
(5)
(5)
where
denotes 25% (TC25) and 50% (TC50), and plan represents treatment plans A1, A2 and B.
In the first evaluation, any difference between standard HTP predictions (plan A1 and plan A2) and the applied HT doses is unwanted since this will cause planning inaccuracies. Hence, we quantified these changes using absolute values:
and
Because the absolute values do not meet the normality assumptions, we used the bootstrapping technique [Citation51] to understand which range the average absolute differences belong. In summary, we resampled the data 100 times, calculated the mean value for each sample, and in the end, we calculated the mean and 95th confidence interval of the bootstrap distribution.
In the second evaluation, we were interested to know whether plan adaptations improved or degraded the results, so we did not use absolute differences. Plan adaptation impact was studied using the differences between plan B predictions and applied HT doses A1 and A2. Since the objective is to evaluate the benefit, we conducted a paired t-test to determine the significance and used p < 0.05 as the threshold for significance.
Results
Positioning evaluation
presents the spatial distance metrics between the body contour in the treatment and the planning position (). Before rigid registration, the average distance between the two positions was 2.1 cm (MSD), and the maximum distance was 4.3 cm (95th HD). The displacement between the two positions might be caused by the lifting power of the water when filling the water bolus. Furthermore, these shifts may be produced by the intrascan movements or shifts during the volunteer’s reposition (step c in ).
Table 3. MSD and 95th HD between the body contour in the planning and treatment positions.
After the alignment (), MSD and 95th HD values characterized the impact of the water bolus pressure in the body. As presented in , the water from the water bolus compressed the abdomen of the patient’s body such that the MSD was ∼0.5 cm and 95th HD was equal to 2.0 cm. The translation needed to match the two positions was 0.1, 3.0 and 0.7 cm in the x-, y- and z-direction, respectively. Additionally, we quantified the body volume in both setups. We observed a volume reduction of 4.5% when the volunteers were placed inside the hyperthermia device. The latter is in line with the assumed compressing effect of the water bolus. Furthermore, we quantify the volume of the volunteer inside the MR-compatible hyperthermia device, and we define it as body volume. This volume was calculated after the volunteer models were placed inside the applicator model during HTP. Between the planning and treatment setup, we observed an absolute decrease of 1.4 ± 0.3 L, corresponding to a 4.6% decrease.
Comparison of HTP (plan A1 and A2) with the simulated treatment (applied HT doses A1 and A2)
presents the average absolute differences of the SAR-based quality indicators acquired from the standard plan predictions (plan A1 and A2) and the simulated treatment after and before adjusting the volunteer position to the treatment position. The plan A1 predictions and applied HT dose A1 are based on volunteers’ anatomy and position. As mentioned, the volunteer’s position was modified to the treatment position when creating plan A2. Therefore, the differentiating modeling parameter between plan A2 predictions and applied HT dose A2 is the anatomy of the volunteer.
Table 4. The average absolute difference and confidence intervals of the SAR-quality indicators parameters between plan A and applied HT dose A.
The results indicate significant differences between the standard plan predictions and the simulated treatment since we found an average absolute error higher than 10% for all SAR-indicators. The rigid registration improved the plan A2 predictions since we found lower differences in | THQ| and |
TC50| compared to plan A1. The average absolute difference after registration is still substantial, indicating the significance of incorporating the real treatment anatomy in HTP. Based on the confidence intervals, we believe that the variation between the HTP predictions and the simulated hyperthermia treatment is likely between 8.3% and 22.6%. The SAR-metric parameters used to calculate the average differences between plan A1 and A2, and applied HT doses are illustrated in Appendix A.
shows the cf-SAR in healthy and tumor tissue in the predictions of simulated treatment and treatment plans (A1 and A2). Even though we observed clear differences between plan predictions and the applied HT doses, we identified fewer differences between the curves of plan A2 and the applied HT dose A2. These results agree with the observations in , where the error between HTP and simulated treatment was decreased when the treatment position was considered. However, the mismatch between the curves from plan A2 and applied HT dose A2 is still significant since there are anatomical differences between the two scenarios, as expressed in .
Figure 7. (a) Average cf-SAR – volume histograms from plans A and applied HT dose A and (b) details the differences between the different scenarios. The histograms with a gray background (right histogram from a)) correspond to the zoomed region from the original cf-SAR – volume histograms (left histogram from a)). Note that the histograms are averages of the 14 volunteers and 3 tumor sizes; thus, 42 scenarios are considered. Four tissues were considered: GTV, HTV, muscle and fat. Plan A1 and A2 denote the standard treatment plans conducted in the clinical routine, where the patient’s imaging does not include the hyperthermia device and its effect on the anatomy.

Evaluate the improvement between the ideal plan (plan B) and the simulated treatment (applied HT doses A1 and A2)
The results in show that plan B significantly improved the THQ by about 10% compared to the applied HT dose A1. Regarding the predicted TC25 and TC50, we observed an improvement of 2.3% and 4.0% when adapting the plan to true anatomy and position. Although different antenna settings were used, the differences between plan B and applied HT dose A2 were smaller because true treatment position was considered in both. Generally, Plan B improved the quality indicators in most volunteer data (14 volunteers: 42 situations). Compared to applied HT dose A1, plan B improved the SAR-metrics in 100% (THQ), 67% (TC25), and 71% (TC50) of the volunteer data, while compared to applied HT dose A2, the improvement was 71%(THQ), 64% (TC25), and 60% (TC50) of the data. Even though the variation between applied HT dose A2 and plan B was small, the results suggest that incorporating the treatment anatomy is still significantly important (improvement in THQ of 4.6%). The SAR-metric parameters used to calculate the average differences between plan B and applied HT doses are illustrated in Appendix A.
Table 5. Description of the improvement in the SAR-quality indicators parameters between plan B and the applied HT dose A1 and A2.
shows that plan B can improve the treatment quality since higher coverage is seen in the target region, and lower cf-SAR is observed in the healthy tissues. We observed that applied HT dose A2 shows slightly better results than applied HT dose A1, which agrees with the previous observations. Overall, there is a clear benefit in using plan B, which considers the correct treatment position and anatomy for optimization.
Figure 8. (a) Average cfSAR – volume histograms from plans B and applied HT doses and (b) details about the differences between the scenarios. The histograms with a gray background (right histogram from a)) correspond to the zoomed region from the original cfSAR – volume histograms (left histogram from a)). Note that the histograms are averages of the 14 volunteers and 3 tumor sizes; thus, 42 scenarios are considered. Four tissues were considered: GTV, HTV, muscle and fat. As mentioned, the applied HT doses denote the simulated treatments using the antenna settings from standard HTP, and plan B characterized the ideal treatment plan based on accurate patient modeling.

Example: SAR distributions of a volunteer
shows a volunteer’s cf-SAR distributions and the corresponding SAR-based quality indicators. Qualitatively, the distributions acquired from the standard plans (A1 and A2) do not look similar to the applied HT doses. When looking at the cf-SAR distributions between these plans and the applied HT doses, clear differences show how altered the predictions made during HTP are compared with the simulated treatment.
Figure 9. Illustration of axial and sagittal cross-sections of the normalized cf-SAR on top of Propeller MR image for the three plans and applied HT doses. The SAR distribution acquired from plan A2 is not overlapped with the MR image since the alignment shifted the model. The SAR distributions are normalized; a dotted black line indicates the HTV. The white arrows indicate the regions in the applied HT dose where higher SAR was observed than predictions from the standard and ideal plan.

As seen in section C, the differences between plan B and applied HT dose A2 decrease when considering treatment position. These results indicate that a correct patient position (plan A2) improves the cf-SAR distribution in the simulated treatment (applied HT dose A2). Furthermore, shows that the SAR level in the target region is similar in applied HT doses A2 and A1, while the SAR level in the healthy tissues decreased. These distributions corroborate the THQ values found since it suggests that plan B reduces the level of SAR in the healthy tissues without losing heating efficacy in the target. Hence, adapting the treatment and creating an HTP based on an accurate representation of the treatment position and anatomy leads to lower energy deposition in healthy tissues.
We observed a 3 cm and 0.4 cm displacement for this volunteer in the y- and z-direction, respectively. These dislocations can be observed in in the SAR distribution from plan A2, where the SAR distribution does not coincide with the MR image because of the alignment. In the x-direction, as with most other volunteers, no substantial displacement was observed.
Discussion
In this study, we simulated the impact of position and anatomy deformations between treatment and HTP using images of healthy volunteers. MR imaging was used to evaluate the accuracy of the current HTP and whether including the proper treatment setup would regain the accuracy and enable a more precise treatment. To replicate the current clinical practice, we developed an MR protocol that enables reproducing and simulating all the current standard stages, from the imaging and generation of HTP to the final treatment implementation. All volunteers were placed in the hammock and scanned with and without the MR-compatible hyperthermia device (). The scan in the first setup was intended to mimic the CT imaging used to generate the CT-based patient model. In contrast, the last scan aimed to image the volunteer in the true (most accurate) treatment position to generate and model the treatment setup.
Patient positioning
MR imaging of the volunteers enabled us to assess the impact of the water bolus filling on the volunteer between the planning and treatment setup position. We found minor displacements in the x-direction (0.1 cm), which we attributed to the fact that the movement of the volunteer in that direction was limited by the hammock, which was validated by other studies [Citation35,Citation40,Citation41]. Gellermann et al. [Citation41] found shifts lower than 1 cm for the x-direction and greater than 2 cm for the y-direction and z-direction. Franckena et al. [Citation35] indicated that the patients presented a strong displacement in the z-direction between the CT scan, which was later corrected when special attention was paid to the positioning of the patient in the hammock in the z-direction. Note that the patients included in the study by Franckena et al. were treated with the BSD-2000 using the Sigma-60 applicator [Citation35]. The impact of the waterbolus pressure of this device on the anatomy and the patient’s position is different because of the leg support, whereby the uncertainty in Y-positioning, as indicated by Canters et al. is >1 cm when only rulers are used [Citation45]. Even though the displacements we report for the x- and y-directions are consistent with the literature values, we observed lower displacements in the z-direction. We attribute the different results to not all volunteers wanting to step out of the hammock between the treatment and planning setup. The re-positioning procedure was avoided, and the anatomy likely was more stable, resulting in fewer movements and anatomical differences. Another possible reason for lower z-direction displacements is the improvement of the clinical protocol with the assistance of laser lights, making patient positioning simpler and reducing positioning shifts. Contrary to the clinical study by Franckena et al. [Citation35], we exploited these tools and MR imaging to verify the volunteer’s position during this volunteer study. The current ESHO quality assurance guidelines for deep hyperthermia provide generic instructions on positioning for x-, y- and z-direction but is not prescribe maximum values for deviations [Citation52,Citation53]. The precision and accuracy of the current clinical procedure of positioning the patient used by the various hyperthermia centers are unknown. Hence, our findings are unique since they show the benefit of MR imaging to adjust positioning and assess the 3 D accuracy of this process.
The water bolus is an essential component of the hyperthermia device since it provides an efficient transfer of EM energy from the antennas and enables patient cooling [Citation54,Citation55]. After we performed rigid registration and aligned the models, we found that the water bolus compressed the abdomen by 0.5 cm (MSD) on average, with a maximum of 2.0 cm (95th HD). Additionally, we found that water pressure reduced the patient volume inside the hyperthermia device by 4.5% on average. Even if the patient’s position from the HTP to the actual treatment position is reproduced successfully, body and anatomic deformations caused by the water bolus are not considered in HTP. Even though several studies have evaluated position displacements in patients and have conducted simulations studies to understand their impact, the level of anatomic deformation and its impact on HTP predictions has not been studied. This study is the first to demonstrate the improvement in HTP accuracy when translating the predicted 3 D SAR distribution for the true treatment position and anatomy instead of using HTP based on CT images of the pretreatment anatomy and position.
Evaluation of the accuracy of HTP translation
One of the main focuses of this study was to assess the accuracy between the predictions from the current HTP (plan A1 and plan A2) and the treatment given (applied HT doses), that is, to understand whether the translated HTP properly represented the treatment condition. To establish this accuracy, we used MR imaging to reproduce the patient’s position from the CT scans to mimic the current state of art protocol for HTP. Second, MR images of the volunteer in the real treatment setup were collected to simulate the treatment administered to the patient. The rigid registration enabled replicating patient re-positioning in MR-guided hyperthermia treatments, where MR images are used to ensure that the patient’s position is according to the HTP. This verification is done within a few minutes since the patient is ready for treatment. Note that the accuracy of the re-positioning of the patient is unknown. Therefore, the rigid registration performed in this study intends to represent the best case scenario when translating the planning position during clinical treatment. The modeling of plans A1 and A2 represent the worst- and best scenarios, which differences can be seen in the schematic representation of . The results show that the average absolute differences between plans A1 and A2 and the applied HT doses were significant in all SAR-based quality indicators (). Even if the position matched the treatment configuration, the actual absolute difference between the standard HTP predictions and simulated treatment is between 8.3%–18.3%, and 8.6%–22.6% for TC25 and TC50. These results demonstrate how far-off the clinical predictions are compared with the administered treatment.
Finally, we assessed the need to adapt the plan based on treatment position and anatomy. The results indicated an improvement in all SAR-based quality indicators using plan B ( and ). We confirmed that cf-SAR in healthy tissues was reduced, and the cf-SAR in the tumor was improved in most cases (). Compared to the applied HT dose A2, the benefit of plan B was reduced since the antenna settings used to calculate the applied HT dose considered the real treatment position. Nevertheless, plan B still showed improvement compared to the applied HT dose A2, reflecting the effect of incorporating the anatomy changes in the treatment setup.
Clinical relevance
This simulation study assessed the impact of the water bolus on the patient’s position and anatomy under true treatment conditions. This study is unique because we used volunteers to demonstrate the effect of water pressure from the water bolus on the position and anatomy. Furthermore, we investigated the impact of this effect on the HTP predictions when the volunteer is on the correct treatment configuration versus the one based on the pretreatment CT-based configuration. Interestingly, the position changes observed in this study are comparable with those reported by Franckena et al., Gellerman et al. and Canters et al. [Citation35,Citation40,Citation41]. As in these studies, we observed higher displacements in the y-direction and lower in the z-direction.
According to the thermal dose-effect relationships for treating LACC by thermoradiotherapy [Citation6,Citation7], the effectiveness of hyperthermia depends on the tumor’s temperature rise and the heating duration. Furthermore, higher SAR levels in healthy tissues are more likely to translate into patient complaints that may limit RF-power input. Together, these results suggest that plan B is beneficial because it leads to lower energy deposition in healthy tissues, potentially enabling higher patient tolerance during treatment and, thus, more effective energy deposition in the target region.
Although we have no quantified clinical evidence of the benefit, we should not underestimate the relevance of improved patient comfort on the quality of the treatment. Earlier, Canters et al. [Citation40] reported that position errors exceeding 1 cm would cause a SAR deviation higher than 5% in hotspots SAR to tumor SAR quotient (1/THQ). The reported findings in our study on the changes between plans A1 and A2, and the applied HT doses agree with the study by Canters et al. In that study [Citation40], the displacements were artificially implemented, and the same model was used between the different positions. Applying the Canters et al. relation between THQ and T50 on the observed differences between standard HTP (plan A1 and A2) and applied HT doses would translate into a variation in T50 of about 0.6 °C. Regarding plan B, the improvement in T50 would be ∼0.3 °C. Hence, the anticipated temperature improvements when incorporating true treatment position and anatomy in HTP are associated with more effective hyperthermia treatment. Note that this association was based on a simulation study using the BSD-2000; thus, its validation for other systems is still needed, and the impact of the relation between THQ and T50 on clinical relevance is still unknown.
Clinical impact and future work
It is well known that the accuracy of the HTP is dependent on the uncertainties in the modeling. Canters et al. [Citation26] indicated that the uncertainty in dielectric parameters influences the HTQ (=1/THQ) up to 25%. Furthermore, uncertainties in the patient’s anatomy can also influence SAR distributions. Earlier, we showed that the effect of tissue delineation could impact the THQ by up to 20% [Citation22]. Even though other parameters can cause higher uncertainties, we believe strategies to improve patient positioning, and anatomic representation should be prioritized based on their practicability to implement and because can contribute to increasing treatment quality. Although the position and anatomic changes seem to affect treatment quality, correcting for positioning deviations contributes more to improving treatment plan predictions. When the correct treatment position was considered, the error between HTP predictions and simulated treatment decreased by 5.5% in THQ (). The benefit of including accurate treatment position and anatomy decrease 4% () when the standard HTP (plan A2) incorporated treatment position. Hence, in our quest to improve the quality of deep hyperthermia treatment using HTP, it is logical to prioritize improving the precision and accuracy of patient positioning. The focus on developing better procedures for accurate patient position is further justified by the fact that majority of the deep hyperthermia treatments are performed in a non-MR setting. Unfortunately, the current practice to control the patients vertical position in the BSD2000 Sigma-60 and Sigma-Eye applicator is based on ruler measurements of the distance between applicator ring and the patient back at both the cranial and caudal edge of the applicator. The accuracy of these ‘ruler’ measurements is poor and presents an important obstacle regarding accurate translation of HTP predicted SAR distributions during clinical treatment [Citation35,Citation41]. Measurement via ultrasound technology may provide a solution but other options should be investigated.
For the MR-compatible hyperthermia devices available in several academic clinical centers, the current study’s results should encourage including MR imaging as part of the standard practice of HTP. As MR imaging will enable the complete treatment setup to be included precisely, it will result in a more accurate translation of HTP results, which is an ultimate requirement to set the pathway for adaptive treatment. Hence, the added value of using MR imaging when treating MR-compatible devices is incontestable since it enables real-time temperature monitoring and improves the translation of HTP to the clinic. This study shows that improving this translation can start with matching the treatment and planning configuration, such as patient position and anatomy. Furthermore, introducing MR imaging provides the advantage of higher tissue contrast, enabling the delineation of more tissues and generating a more personalized treatment plan. As most of the current HTP workflows use CT images, the tissue delineation is based on thresholding. Applying the same process to MR images is not trivial and can be time-consuming and labor-intensive. Hence, using MR imaging in the current HTP workflow requires successful used segmentation methods such as atlas-based techniques [Citation56–64] or deep learning strategies [Citation65–69] that enable fast and accurate segmentation.
Finally, it is relevant to realize that most patients are treated in non-hybrid deep hyperthermia systems because of the patient’s dimensions and the availability of such devices. So far, state of the art about using MR hybrid systems has been focused mainly on the ability to monitor the 3 D temperature measurement noninvasively. However, considering the different MR imaging techniques, the key benefit of MR-hybrid systems is that these enable validation of the current HTP research to investigate improvements in patient modeling (tissue properties and their uncertainties) as well as assessment of treatment response. No doubt will exist that the benefits of the ‘academic’ MR-guided hyperthermia research will eventually find their use in the many treatments conducted in non-MR-compatible devices.
Limitations of the study
The findings of this study have to be seen in light of some considerations. During the registration, we did not quantify elastic deformations in the anatomy and did not consider possible deformations in the GTV and HTV. The internal anatomic architecture was preserved because rigid registration is limited to rotational and translational transformations. Due to the unpredictability nature of the deformation and the aim of this study, we considered this limitation insignificant.
The clinical relevance of considering the true treatment anatomy and position in HTP is still unclear since the significance of these results is based on a simulation study conducted on healthy volunteers. Nevertheless, we establish that when the patient’s position and anatomy are known accurately, Plan B can correct the SAR distribution without losing heating efficiency in the target while sparing the healthy tissues. An important aspect to consider when using volunteer data is how the results translate to the clinic. The volunteers in this study were younger and heavier than patients treated with the MR-compatible device, as shown in . Because of the restrictive patient size guidelines of the MR-compatible device, the number of healthy volunteers following the requirements was restrictive for young female adults. Although the volunteers presented higher weight and likely higher muscle percentage [Citation22], we expect that these differences do not or barely affect the results since the analysis is based on relative differences. Further, the displacements we found coincide with patient displacements reported in previous clinical studies [Citation35,Citation41]. Another limitation is that we did not study the impact of dielectric property uncertainties. For locoregional hyperthermia, studies have shown that the impact of these uncertainties can be up to 20% in SAR and temperature distributions [Citation26,Citation70], making tissue properties uncertainties an important aspect to consider in future studies. Another important aspect is the translation of SAR-quality indicator parameters to thermal dose and, subsequently, clinical outcome. Although our results showed that positioning strongly impacts THQ, only a theoretical association with temperature has been established for this metric. Hence, the true impact on temperature and treatment outcome when adapting for patient anatomy and position in the applicator needs further evaluation.
Conclusion
In this study, we assessed the impact of patient position and anatomy on the accuracy of the current HTP and the importance of accurate treatment position and anatomy to improve hyperthermia treatment. We found that the displacements between the planning and real treatment position are predominantly in the y-direction, that is, the pressure of the water bolus can deform the patient’s body up to 2 cm. Our study also indicates the benefit of (MR) imaging of the entire treatment setup to account for position changes and deformation.
Precise positioning contributed most strongly to the improved HTP accuracy since we found an average absolute difference above 12% (anatomy and position different) and 10% (only different anatomy) for all SAR-based quality indicators (THQ, TC25 and TC50). Furthermore, we observed that the confidence interval for THQ in both scenarios was still substantial: 13.5%−19.8% (anatomy and position different) and 8.4%−14.4% (only different anatomy). MR imaging of the patient during the hyperthermia treatment device can provide the required anatomical information to adjust HTP and improve HTP-guided steering. We found that plan B, considered the ideal plan, showed improvements in THQ above 4.6% and TC25 above 2.3%.
While our results should be confirmed during clinical treatments in MR hybrid hyperthermia systems, they also suggest the importance of a better understanding of the current positioning accuracy. Hence, the results indicate that introducing methods to accurately measure the patient’s true treatment position in non-MR-hybrid systems will provide a more effective translation of the HTP predictions.
Acknowledgments
The authors thank Theresa Feddersen, Ioannis Androulakis and Gennaro Bellizzi for their assistance during the volunteer experiments. Furthermore, the authors thank Pelle ter Haar for his help in the process of tissue delineation, Piotr A. Wielopolski for his support in the development of the MR protocol, and Erik van Werkhoven for his advice in the statistical analysis.
Disclosure statement
No potential conflict of interest was reported by the author(s).
Additional information
Funding
References
- van der Zee J. Heating the patient: a promising approach? Ann Oncol. 2002;13(8):1173–1184.
- Wust P, Hildebrandt B, Sreenivasa G, et al. Hyperthermia in combined treatment of cancer. Lancet Oncol. 2002;3(8):487–497.
- Yea JW, Park JW, Oh SA, et al. Chemoradiotherapy with hyperthermia versus chemoradiotherapy alone in locally advanced cervical cancer: a systematic review and Meta-Analysis. Int J Hyperthermia. 2021;38(1):1333–1340.
- Franckena M, Stalpers LJA, Koper PCM, et al. Long-Term improvement in treatment outcome After radiotherapy and hyperthermia in locoregionally advanced cervix cancer: an update of the dutch deep hyperthermia trial. Int J Radiat Oncol Biol Phys. 2008;70(4):1176–1182.
- Zee J, Van Der; González DG, Rhoon GC, et al. Comparison of radiotherapy alone with radiotherapy plus hyperthermia in locally advanced pelvic tumors. Lancet. 2000;355:1119–1125.
- Franckena M, Fatehi D, Bruijne M D, et al. Hyperthermia dose-effect relationship in 420 patients with cervical cancer treated with combined radiotherapy and hyperthermia. Eur J Cancer. 2009;45(11):1969–1978.
- Kroesen M, Mulder HT, van Holthe JML, et al. Confirmation of thermal dose as a predictor of local control in cervical carcinoma patients treated with state-of-the-Art radiation therapy and hyperthermia. Radiother Oncol. 2019;140:150–158.
- VilasBoas‐Ribeiro I, Nouwens SAN, Curto S, et al. POD–kalman filtering for improving noninvasive 3D temperature monitoring in MR‐guided hyperthermia. Med. Phys. 2022;49(8):4955–4970.
- Adibzadeh F, Sumser K, Curto S, et al. Systematic review of pre-clinical and clinical devices for magnetic resonance-guided radiofrequency hyperthermia. Int J Hyperthermia. 2020;37(1):15–27.
- Gellermann J, Wlodarczyk W, Ganter H, et al. A practical approach to thermography in a hyperthermia/magnetic resonance hybrid system: validation in a heterogeneous phantom. Int J Radiat Oncol Biol Phys. 2005;61(1):267–277.
- Gellermann J, Wlodarczyk W, Feussner A, et al. Methods and potentials of magnetic resonance imaging for monitoring radiofrequency hyperthermia in a hybrid system. Int J Hyperthermia. 2005;21(6):497–513.
- Nouwens SAN, Paulides MM, Fölker J, et al. Integrated thermal and magnetic susceptibility modeling for Air-Motion artifact correction in proton resonance frequency shift thermometry. Int J Hyperthermia. 2022;39(1):967–976.
- VilasBoas-Ribeiro I, Curto S, van Rhoon GC, et al. MR thermometry accuracy and prospective Imaging-Based patient selection in MR-Guided hyperthermia treatment for locally advanced cervical cancer. Cancers (Basel). 2021;13(14):3503.
- Unsoeld M, Lamprecht U, Traub F, et al. MR thermometry data correlate with pathological response for soft tissue sarcoma of the lower extremity in a single center analysis of prospectively registered patients. Cancers (Basel). 2020;12(4):959.
- Gellermann J, Wlodarczyk W, Hildebrandt B, et al. Noninvasive magnetic resonance thermography of recurrent rectal carcinoma in a 1.5 tesla hybrid system. Cancer Res. 2005;65(13):5872–5880.
- Gellermann J, Hildebrandt B, Issels R, et al. Noninvasive magnetic resonance thermography of soft tissue sarcomas during regional hyperthermia: correlation with response and direct thermometry. Cancer. 2006;107(6):1373–1382.
- Cheng KS, Stakhursky V, Craciunescu OI, et al. Fast temperature optimization of multi-source hyperthermia applicators with reduced-order modeling of “virtual sources. Phys Med Biol. 2008;53(6):1619–1635.
- Kok HP, Navarro F, Strigari L, et al. Locoregional hyperthermia of Deep-Seated tumours applied with capacitive and radiative systems: a simulation study. Int J Hyperthermia. 2018;34(6):714–730.
- Paulides MM, Rodrigues DB, Bellizzi GG, et al. ESHO benchmarks for computational modeling and optimization in hyperthermia therapy. Int J Hyperthermia. 2021;38(1):1425–1442.
- Aklan B, Zilles B, Paprottka P, et al. Regional deep hyperthermia: quantitative evaluation of predicted and direct measured temperature distributions in patients with High-Risk extremity Soft-Tissue sarcoma. Int J Hyperthermia. 2019;36(1):170–185.
- Wust P, Stahl H, Löffel J, et al. Clinical, physiological and anatomical determinants for radiofrequency hyperthermia. Int J Hyperthermia. 1995;11(2):151–167.
- Vilasboas-Ribeiro I, van Rhoon GC, Drizdal T, et al. Impact of number of segmented tissues on SAR prediction accuracy in deep pelvic hyperthermia treatment planning. Cancers (Basel). 2020;12(9):2646.
- Wust P, Nadobny J, Seebass M, et al. Influence of patient models and numerical methods on predicted power deposition patterns. Int. J. Hyperth. 1999;15(6):519–540.
- Bellizzi GG, Sumser K, VilasBoas-Ribeiro I, et al. Standardization of patient modeling in hyperthermia simulation studies: introducing the erasmus virtual patient repository. Int J Hyperthermia. 2020;37(1):608–616.
- Schooneveldt G, Kok HP, Bakker A, et al. Clinical validation of a novel thermophysical bladder model designed to improve the accuracy of hyperthermia treatment planning in the pelvic region. Int J Hyperthermia. 2018;35(1):383–397.
- Canters RAM, Paulides MM, Franckena M, et al. Benefit of replacing the sigma-60 by the Sigma-Eye applicator: a monte Carlo-Based uncertainty analysis. Strahlenther Onkol. 2013;189(1):74–80.
- Paulides MM, Stauffer PR, Neufeld E, et al. Simulation techniques in hyperthermia treatment planning. Int J Hyperthermia. 2013;29(4):346–357.
- Kok HP, Wust P, Stauffer PR, et al. Current state of the art of regional hyperthermia treatment planning: a review. Radiat Oncol. 2015;10(1):1–14.
- van Haaren PMA, Kok HP, van den Berg CAT, et al. On verification of hyperthermia treatment planning for cervical carcinoma patients. Int J Hyperthermia. 2007;23(3):303–314.
- Sreenivasa G, Gellermann J, Rau B, et al. Clinical use of the hyperthermia treatment planning system HyperPlan to predict effectiveness and toxicity. Int J Radiat Oncol Biol Phys. 2003;55(2):407–419.
- Kok HP, Ciampa S, De Kroon-Oldenhof R, et al. Toward online adaptive hyperthermia treatment planning: correlation between measured and simulated specific absorption rate changes caused by phase steering in patients. Int J Radiat Oncol Biol Phys. 2014;90(2):438–445.
- Rijnen Z, Bakker JF, Canters RAM, et al. Clinical integration of software tool VEDO for adaptive and quantitative application of phased array hyperthermia in the head and neck. Int J Hyperthermia. 2013;29(3):181–193.
- Kok HP, Korshuize- van Straten L, Bakker A, et al. Online adaptive hyperthermia treatment planning During locoregional heating to suppress Treatment-Limiting hot spots. Int J Radiat Oncol Biol Phys. 2017;99(4):1039–1047.
- Kok HP, Korshuize-van Straten L, Bakker A, et al. Feasibility of On-Line Temperature-Based hyperthermia treatment planning to improve tumour temperatures during locoregional hyperthermia. Int J Hyperthermia. 2018;34(7):1082–1091.
- Franckena M, Canters R, Termorshuizen F, et al. Clinical implementation of hyperthermia treatment planning guided steering: a cross over trial to assess its current contribution to treatment quality. Int J Hyperthermia. 2010;26(2):145–157.
- Wiersma J, van Wieringen N, Crezee H, et al. Delineation of potential hot spots for hyperthermia treatment planning optimisation. Int J Hyperthermia. 2007;23(3):287–301.
- De Greef M, Kok HP, Correia D, et al. Uncertainty in hyperthermia treatment planning: the need for robust system design. Phys Med Biol. 2011;56(11):3233–3250.
- Gavazzi S, van Lier ALHMW, Zachiu C, et al. Advanced Patient-Specific hyperthermia treatment planning. Int J Hyperthermia. 2020;37(1):992–1007.
- Ribeiro IVB, Van Holthe N, Van Rhoon GC, et al. Impact of segmentation detail in hyperthermia treatment planning. Cancer (Basel). 2020;12(9):2646.
- Canters RAM, Franckena M, Paulides MM, et al. Patient positioning in deep hyperthermia: influences of inaccuracies, signal correction possibilities and optimization potential. Phys Med Biol. 2009;54(12):3923–3936.
- Gellermann J, Göke J, Figiel R, et al. Simulation of different applicator positions for treatment of a presacral tumour. Int J Hyperthermia. 2007;23(1):37–47.
- Craciunescu OI, Stauffer PR, Soher BJ, et al. Accuracy of real time noninvasive temperature measurements using magnetic resonance thermal imaging in patients treated for high grade extremity soft tissue sarcomas. Med Phys. 2009;36(11):4848–4858.
- Stauffer PR, Craciunescu OI, Maccarini PF, et al. Clinical utility of magnetic resonance thermal imaging (MRTI) for realtime guidance of deep hyperthermia. Proceedings Volume 7181, Energy-based Treatment of Tissue and Assessment V. 2009. p 7181.
- Feddersen TV, Hernandez-Tamames JA, Franckena M, et al. Clinical performance and future potential of magnetic resonance thermometry in hyperthermia. Cancers (Basel). 2020;13(1):31.
- Canters RAM, Paulides MM, Franckena MF, et al. Implementation of treatment planning in the routine clinical procedure of regional hyperthermia treatment of cervical cancer: an overview and the rotterdam experience. Int J Hyperthermia. 2012;28(6):570–581.
- Canters RAM, Wust P, Bakker JF, et al. A literature survey on indicators for characterisation and optimisation of SAR distributions in deep hyperthermia, a plea for standardisation. Int J Hyperthermia. 2009;25(7):593–608.
- Kiser KJ, Barman A, Stieb S, et al. Novel autosegmentation spatial similarity metrics capture the time required to correct segmentations better than traditional metrics in a thoracic cavity segmentation workflow. J Digit Imaging. 2021;34(3):541–553.
- Yeghiazaryan V, Voiculescu I. Family of boundary overlap metrics for the evaluation of medical image segmentation. J Med Imag. 2018;5(01):1.
- Aydin OU, Taha AA, Hilbert A, et al. On the usage of average hausdorff distance for segmentation performance assessment: hidden error when used for ranking. Eur Radiol Exp. 2021;5(1):4.
- Taha AA, Hanbury A. Metrics for evaluating 3D medical image segmentation: analysis, selection, and tool. BMC Med Imaging. 2015;15(1).
- Hasgall P, Di Gennaro F, Baumgartner C, et al. IT’IS database for thermal and electromagnetic parameters of biological tissues itis.swiss/database 2018 [accessed 22 April 2020]. Available from: https://itis.swiss/virtual-population/tissue-properties/database/dielectric-properties/
- Bruggmoser G, Bauchowitz S, Canters R, et al. Quality assurance for clinical studies in regional deep hyperthermia. Strahlenther Onkol. 2011;187(10):605–610.
- Bruggmoser G, Bauchowitz S, Canters R, et al. Guideline for the clinical application, documentation and analysis of clinical studies for regional deep hyperthermia. Strahlenther Onkol. 2012;188(S2):198–211.
- Oberacker E, Kuehne A, Nadobny J, et al. Radiofrequency applicator concepts for simultaneous MR imaging and hyperthermia treatment of glioblastoma multiforme. Curr Dir Biomed Eng. 2017;3(2):473–477.
- Koo TK, Li MY. A guideline of selecting and reporting intraclass correlation coefficients for reliability research. J Chiropr Med. 2016;15(2):155–163.
- Iglesias JE, Sabuncu MR. Multi-Atlas segmentation of biomedical images: a survey. Med Image Anal. 2015;24(1):205–219.
- Fortunati V, Verhaart RF, Niessen WJ, et al. Automatic tissue segmentation of head and neck MR images for hyperthermia treatment planning. Phys Med Biol. 2015;60(16):6547–6562.
- Teguh DN, Levendag PC, Voet PWJ, et al. Clinical validation of atlas-based auto-segmentation of multiple target volumes and normal tissue (swallowing/mastication) structures in the head and neck. Int J Radiat Oncol Biol Phys. 2011;81(4):950–957.
- Ju Z, Wu Q, Yang W, et al. Automatic segmentation of pelvic organs-at-Risk using a fusion network model based on limited training samples. Acta Oncol. 2020;59(8):933–939.
- Kalantar R, Lin G, Winfield JM, et al. Automatic segmentation of pelvic cancers using deep learning: state-of-the-Art approaches and challenges. Diagnostics. 2021;11(11):1964.
- Estrada S, Lu R, Conjeti S, et al. FatSegNet: a fully automated deep learning pipeline for adipose tissue segmentation on abdominal dixon MRI. Magn Reson Med. 2020;83(4):1471–1483.
- O’Connor LM, Dowling JA, Choi JH, et al. Validation of an MRI-Only planning workflow for definitive pelvic radiotherapy. Radiat Oncol. 2022;17(1):1–11.
- Farjam R, Tyagi N, Deasy JO, et al. Dosimetric evaluation of an Atlas-Based synthetic CT generation approach for MR-Only radiotherapy of pelvis anatomy. J Appl Clin Med Phys. 2019;20(1):101–109.
- Guerreiro F, Burgos N, Dunlop A, et al. Evaluation of a Multi-Atlas CT synthesis approach for MRI-Only radiotherapy treatment planning. Phys Med. 2017;35:7–17.
- Bentvelzen LG, Cronholm RO, Karlsson A, et al. Auto-Segmentation of Pelvic Structures Using MRI Planner; A Quantitative Evaluation. No. 2020. 1–5.
- Savenije MHF, Maspero M, Sikkes GG, et al. Clinical implementation of MRI-Based organs-at-Risk Auto-Segmentation with convolutional networks for prostate radiotherapy. Radiat Oncol. 2020;15(1):1–12.
- Liu Z, Liu X, Guan H, et al. Development and validation of a deep learning algorithm for Auto-Delineation of clinical target volume and organs at risk in cervical cancer radiotherapy. Radiother Oncol. 2020;153:172–179.
- Chen M, Wu S, Zhao W, et al. Application of deep learning to auto-delineation of target volumes and organs at risk in radiotherapy. Cancer/Radiotherapie. 2022;26(3):494–501.
- Min H, Dowling J, Jameson MG, et al. Automatic radiotherapy delineation quality assurance on prostate MRI with deep learning in a multicentre clinical trial. Phys Med Biol. 2021;66(19):195008.
- Kamer JBVD, Wieringen NV, Leeuw aaCD, et al. The significance of accurate dielectric tissue data for hyperthermia treatment planning. Int J Hyperthermia. 2001;17(2):123–142.
- McIntosh R, Anderson V. A comprehensive tissue properties database provided for the thermal assessment of a human At rest. Biophys Rev Lett. 2013;08(01n02):99–100.
Appendix A
presents the SAR-based quality indicators from the different treatment plans and simulated treatment. The treatment plan A1 and A2 denote the standard treatment plans and plan B the envisioned plan. In plan A1, the planning anatomy and position were considered while in plan A2, the volunteer position was matched to the treatment position. Furthermore, for each treatment plan and applied HT dose, the average values of SAR-based metric are given in a red symbol in the scatter plots.