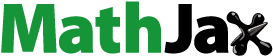
Abstract
Binding to acetylcholinesterase (AChE k1) may cause toxic effects in humans. Organophosphates simultaneously are both dangerous and useful substances. Dangerous since they are employed in chemical warfare and useful when they are applied as pesticides. Here, we suggest the models for organophosphates binding to AChE k1 developed via representing the molecular structure by a simplified molecular input-line entry system using so-called optimal descriptors calculated with the Monte Carlo technique using the Correlation and Logic (CORAL) free software available on the Internet (http://www.insilico.eu/coral). Quantitative structure-activity relationships (QSARs) serve to develop predictive models for organophosphates. The predictive potential of these models is quite good: the determination coefficient for the validation set ranged from 0.87 to 0.90. These models were built up according to the principle ‘QSARs is a random event’, that is, predictive potential of an approach should be checked up with several splits of available data into the training and test sets. The special scheme of mechanistic interpretation definition is represented. The mechanistic interpretation is based on probabilities of molecular features to be in the sub-group of promoters of increase for endpoint or in sub-group of promoters of it is decrease.
Introduction
Pesticides are widely used in agriculture for plant protection and to increase the yields and quality of agricultural products, but also in domestic applications (Naik, Singh, and Singh Citation2009). Organophosphate compounds (OPC) are the widely used choice as insecticides and are widely used across the world. For this reason, these substances are frequently encountered in surface water and groundwater due to their agricultural use. OPC are also used for ornamentals, in floriculture, and for residential treatments. Unfortunately, despite these advantages, these pesticides are highly toxic and also bio accumulate (Torres-Palma and Serna-Galvis Citation2018; Bora et al. Citation2020). Exposure to OPCs can affect the immune response and carbohydrate metabolism, and cause cardiovascular toxicity (Marimuthu et al. Citation2019).
Residues of these pesticides usually build up on the surface of the soil and ultimately find their way into food products. They may have adverse impacts on various groups of soil microbes, wild animals, and also humans, through environmental contamination, or residues in food and feedstuff (Torres-Palma and Serna-Galvis Citation2018; Bora et al. Citation2020; Toropov et al. Citation2017, Citation2018). Experimental data on these chemicals can be used to improve the design of new pesticides, moving toward safer, greener substances, considering their adverse effects on humans and ecosystems (Mudasir, Wibowo, and Pranowo Citation2013; Wibowo, Mudasir, and Pranowo Citation2017). Furthermore, OPC are chemical warfare tools (Mendonca et al. Citation2020).
Quantitative structure-activity relationships (QSARs) serve to develop predictive models for the toxicity of compounds in general and OPC in particular (Niraj, Saini, and Kumar Citation2015; Marimuthu et al. Citation2019; Bora et al. Citation2020; Maxwell and Brecht Citation1992; Ruark et al. Citation2011; Zhao and Yu Citation2013; Morrill et al. Citation2015; Lee and Barron Citation2016). The Correlation and Logic (CORAL) software (http://www.insilico.eu/coral) has been used up as a tool to develop QSAR models for different endpoints (Toropov et al. Citation2017, Citation2018; Veselinović et al. Citation2015; Kumar and Chauhan Citation2017; Kumar, Kumar, and Sindhu Citation2019; Islam and Pillay Citation2016). Here, we studied the ability of this software to build up predictive models for toxicity OPC binding to acetylcholinesterase (AChE k1).
Method
Data
A database of 278 molecular structures together with numerical data on toxicity represented by rate constants for human binding to AChE k1 were taken from Ruark et al. (Citation2013). These substances have been randomly distributed into an active training set (≈25%) used to construct the model, a passive training set (≈25%) independent of those used for building the model, a calibration set (≈25%) to identify situations under which the model is over-training, and a validation set (≈25%) to provide the final assessment of the statistical quality of the model (Toropov, Toropova, and Benfenati Citation2020). The molecular structure was represented with simplified molecular input-line entry systems (SMILES; Weininger Citation1988).
Optimal SMILES-based descriptors
The optimal SMILES-based descriptor of the correlation weights (DCW) applied here is calculated as follows:
(1)
(1)
Correlation weights (CW) are calculated with the Monte Carlo method using the CORAL software (http://www.insilico.eu/coral). T is an integer to define rare fragments and active ones. N is the number of epochs of the Monte Carlo optimization. Atom-pairs proportion (APP) is atom pair’s proportions self-organized vector.
In , examples of APP are given. Sk is SMILES atoms, that is, one symbol or a group of symbols that cannot be examined separately (‘Br’, ‘%11’, ‘@@’). SSk is fragments of SMILES containing two SMILES atoms. CW(APP), CW(Sk), and CW(SSk) are correlation weights for these SMILES attributes.
Table 1. An example of building a self-organized vector of APP for the following SMILES: CCC(Br)(CC)C(=O)NC(N)=O.
T* is the threshold, that is, a value to discriminate molecular features extracted from SMILES into two categories, viz. rare (frequency in the active training set is less than T*) and active (frequency in the active training set is equal or better than T*). N* is the number of epochs of the Monte Carlo optimization.
Monte Carlo optimization
QSAR-models were calculated with the Monte Carlo optimization based on the target functions:
(2)
(2)
and
are correlation coefficients between observed and predicted endpoint for the active training and passive training sets, respectively. The maximum given by EquationEquation 2
(2)
(2) is the target of the Monte Carlo calculations. Having the corresponding numerical data in the CWs, the endpoint is calculated as follows:
(3)
(3)
the CO and C1 are regression coefficients.
Results and discussion
Domain of applicability
The domain of applicability for these compounds is defined according to so-called SMILES defects (Toropova et al. Citation2020). The number of potential outliers in the validation set is 6, 7, and 5, respectively, for splits 1, 2, and 3. The SMILES defect is not a rigid categorization of compounds but only indicates that the representability of fragments of the corresponding SMILES in the active training set (Toropova et al. Citation2020) is not enough. gives examples of the statistical outliers. The outliers are not all similar for different splits though some are the same (e.g. #1 for splits 1 and 2; #198 for splits 2 and 3).
Mechanistic interpretation
In , promoters (Toropova et al. Citation2020) for increases and decreases for rate constants of human AChE k1 are listed. There are some promoters of an increase (double bonds indicated by ‘=’) since in all three runs of the Monte Carlo optimization this SMILES attribute obtains positive CWs () and some promoters of a decrease (brackets in SMILES) since this SMILES attribute obtains negative CWs in all three runs of the optimization. However, there are some more complex and more intriguing promoters, for example, oxygen and phosphorus in proportion 2:1 [SMILES attribute “(O.P).2.1.”] is a promoter of greater toxicity, while the fragment PO [SMILES attribute “P…O…….”] is a promoter of lower toxicity. These data can be useful from the heuristic point of view. It is to be noted that (O.P).2.1. means the presence oxygen and phosphoros atoms in ration 2:1 (maybe not connected directly); whereas P…O……. means connecting of these atoms by covalent bond.
Table 2. Mechanistic interpretation – promoters of increase and decrease for the toxicity of the pesticides.
Models
Computational experiments with three random splits give the following models:
Split 1
Split 2
Split 3
(6)
contains the statistical characteristics of models for three random splits. There is dispersion of the statistical characteristics, but not too much. In other words, the models suggested are robust and can be used for practical applications.
Table 3. Statistical characteristics of models for splits 1–3.
Comparison with models from the literature
In , the statistical quality of models suggested here is compared with some other models suggested. Despite the interest in OPCs (Devillers Citation2000; Mudasir, Wibowo, and Pranowo Citation2013; Ruark et al. Citation2013; Bora et al. Citation2020) it is impossible to find any QSAR studies on the rate constants of human AChE k1. Therefore the only comparison can be between the results here and those from the original article (Ruark et al. Citation2013). In our case, the original set of compounds was split into four sets: active training, passive training, calibration, and validation, while Ruark et al. (Citation2013) report the results on three subsets. However, it is possible to make a comparison. The results of the active training are quite similar. No results on the passive training are available in the original article, because the authors applied a different approach; however, the values for the passive training used only in our case are higher than those on the training set in the original article. The values for the calibration and validation sets are always better in our case, particularly for the validation set. The validation set values are of particular interest because they demonstrate that our model is not affected by overtraining and quite probably can be applied for substances not used in the modeling phase.
Table 4. Comparison with statistical characteristics of a model described in the literature.
Besides their good statistical results, our model is simpler, does not require the calculation of molecular descriptors as in Ruark’s article (2013), but only needs the SMILES to represent the molecular structure.
Supplementary materials section gives technical details of the suggested models.
Conclusions
We have developed new models for AChE binding for OPC that are robust and predictive. These models are accompanied by the domain of applicability and a mechanistic interpretation. The statistical quality of the models examined here is better than in models for the same endpoint obtained with the CODESSA software (Ruark et al. Citation2013). The approach we adopt is quite simple and uses the freely available CORAL software.
Ethical approval
The manuscript is not submitted to any other journal.
Consent to participate
The article did not involve any human participants.
Consent to publish
The submitted work is original.
Author contributions
APT was involved in formal analysis, data collection, and curation, investigation, methodology incl. statistics, software, visualization, writing of original draft, and revision. AAT was involved in conceptualization, data curation, formal analysis, investigation, methodology incl. statistics, software, visualization, writing of original draft, review, and editing. AR was involved in conceptualization, formal analysis, visualization, writing of original draft, review, and editing. EB was involved in conceptualization, formal analysis, visualization, review, and editing.
Supplemental Material
Download MS Excel (68.1 KB)Acknowledgments
The authors are grateful for the contribution of the project LIFE-CONCERT contract (LIFE17 GIE/IT/000461) and Optitox (OC/EFSA/SCER/2018/01) for support.
Disclosure statement
The authors declare that they have no known competing financial interests or personal relationships that could have appeared to influence the work reported in this article.
Data availability statement
All data generated or analyzed during this study are included in this article (and its supplementary information files).
Additional information
Funding
References
- Bora, A., L. Crisan, A. Borota, S. Funar-Timofei, and G. Ilia. 2020. “Ecotoxicological QSAR Modeling of Organophosphorus and Neonicotinoid Pesticides.” In Ecotoxicological QSARs, edited by Kunal Roy, 513–544. New York: Humana. doi:10.1007/978-1-0716-0150-1_21.
- Devillers, J. 2000. “Prediction of Toxicity of Organophosphorus Insecticides against the Midge, Chironomus Riparius, via a QSAR Neural Network Model Integrating Environmental Variables.” Toxicology Methods 10 (1): 69–79. doi:10.1080/105172300242562.
- Islam, M. A., and T. S. Pillay. 2016. “Simplified Molecular Input Line Entry System-Based Descriptors in QSAR Modeling for HIV-Protease Inhibitors.” Chemometrics and Intelligent Laboratory Systems 153: 67–74. doi:10.1016/j.chemolab.2016.02.008.
- Kumar, A., and S. Chauhan. 2017. “QSAR Differential Model for Prediction of SIRT1 Modulation Using Monte Carlo Method.” Drug Research 67 (3): 156–162. doi:10.1055/s-0042-119725.
- Kumar, P., A. Kumar, and J. Sindhu. 2019. “Design and Development of Novel Focal Adhesion Kinase (FAK) Inhibitors Using Monte Carlo Method with Index of Ideality of Correlation to Validate QSAR.” SAR and QSAR in Environmental Research 30 (2): 63–80. doi:10.1080/1062936X.2018.1564067.
- Lee, S., and M. G. Barron. 2016. “A Mechanism-Based 3D-QSAR Approach for Classification and Prediction of Acetylcholinesterase Inhibitory Potency of Organophosphate and Carbamate Analogs.” Journal of Computer-Aided Molecular Design 30 (4): 347–363. doi:10.1007/s10822-016-9910-7.
- Marimuthu, P., Y.-J. Lee, B. Kim, and S. S. Seo. 2019. “In Silico Approaches to Evaluate the Molecular Properties of Organophosphate Compounds to Inhibit Acetylcholinesterase Activity in Housefly.” Journal of Biomolecular Structure & Dynamics 37 (2): 307–320. doi:10.1080/07391102.2018.1426046.
- Maxwell, D. M., and K. M. Brecht. 1992. “Quantitative Structure-Activity Analysis of Acetylcholinesterase Inhibition by Oxono and Thiono Analogues of Organophosphorus Compounds.” Chemical Research in Toxicology 5 (1): 66–71. doi:10.1021/tx00025a011.
- Mendonca, M. L., D. Ray, C. J. Cramer, and R. Q. Snurr. 2020. “Exploring the Effects of Node Topology, Connectivity, and Metal Identity on the Binding of Nerve Agents and Their Hydrolysis Products in Metal-Organic Frameworks.” ACS Applied Materials & Interfaces 12 (31): 35657–35675. doi:10.1021/acsami.0c08417.
- Morrill, J. A., J. J. Topczewski, A. M. Lodge, N. Yasapala, and D. M. Quinn. 2015. “Development of Quantitative Structure Activity Relationships for the Binding Affinity of Methoxypyridinium Cations for Human Acetylcholinesterase.” Journal of Molecular Graphics & Modelling 62: 181–189. doi:10.1016/j.jmgm.2015.09.016.
- Mudasir, M., Y. M. Wibowo, and H. D. Pranowo. 2013. “Design of New Potent Insecticides of Organophosphate Derivatives Based on QSAR Analysis.” Indonesian Journal of Chemistry 13 (1): 86–93. doi:10.22146/ijc.21331.
- Naik, P. K., T. Singh, and H. Singh. 2009. “Quantitative Structure–Activity Relationship (QSAR) for Insecticides: Development of Predictive in Vivo Insecticide Activity Models.” SAR and QSAR in Environmental Research 20 (5-6): 551–566. doi:10.1080/10629360903278735.
- Niraj, R. R. K., V. Saini, and A. Kumar. 2015. “QSAR Analyses of Organophosphates for Insecticidal Activity and Its In-Silico Validation Using Molecular Docking Study.” Environmental Toxicology and Pharmacology 40 (3): 886–894. doi:10.1016/j.etap.2015.09.021.
- Ruark, C. D., C. E. Hack, P. J. Robinson, and J. M. Gearhart. 2011. “Quantitative Structure-Activity Relationships for Organophosphates Binding to Trypsin and Chymotrypsin.” Journal of Toxicology and Environmental Health. Part A 74 (1): 1–23. doi:10.1080/15287394.2010.501716.
- Ruark, C. D., C. E. Hack, P. J. Robinson, P. E. Anderson, and J. M. Gearhart. 2013. “Quantitative Structure-Activity Relationships for Organophosphates Binding to Acetylcholinesterase.” Archives of Toxicology 87 (2): 281–289. doi:10.1007/s00204-012-0934-z.
- Toropov, A. A., A. P. Toropova, and E. Benfenati. 2020. “QSAR Model for Pesticides Toxicity to Rainbow Trout Based on “Ideal Correlations.” Aquatic Toxicology (Amsterdam, Netherlands) 227: 105589. doi:10.1016/j.aquatox.2020.105589.
- Toropov, A. A., A. P. Toropova, E. Benfenati, and J. L. Dorne. 2018. “SAR for Gastro-Intestinal Absorption and Blood-Brain Barrier Permeation of Pesticides.” Chemico-Biological Interactions 290: 1–5. doi:10.1016/j.cbi.2018.04.030.
- Toropov, A. A., A. P. Toropova, M. Marzo, J. L. Dorne, N. Georgiadis, and E. Benfenati. 2017. “QSAR Models for Predicting Acute Toxicity of Pesticides in Rainbow Trout Using the CORAL Software and EFSA’s OpenFoodTox Database.” Environmental Toxicology and Pharmacology 53: 158–163. doi:10.1016/j.etap.2017.05.011.
- Toropova, A. P., A. A. Toropov, E. Carnesecchi, E. Benfenati, and J. L. Dorne. 2020. “The Using of the Index of Ideality of Correlation (IIC) to Improve Predictive Potential of Models of Water Solubility for Pesticides.” Environmental Science and Pollution Research International 27 (12): 13339–13347. doi:10.1007/s11356-020-07820-6.
- Torres-Palma, R. A., and E. A. Serna-Galvis. 2018. “Sonolysis.” In Advanced Oxidation Processes for Wastewater Treatment: Emerging Green Chemical Technology, edited by Ameta, S.C., Ameta, R., 177–213. Cambridge, MA: Academic Press. doi:10.1016/B978-0-12-810499-6.00007-3.
- Veselinović, A. M., J. B. Veselinović, J. V. Živković, and G. M. Nikolić. 2015. “Application of SMILES Notation Based Optimal Descriptors in Drug Discovery and Design.” Current Topics in Medicinal Chemistry 15 (18): 1768–1779. doi:10.2174/1568026615666150506151533.
- Weininger, D. 1988. “SMILES, a Chemical Language and Information System. 1. Introduction to Methodology and Encoding Rules.” Journal of Chemical Information and Modeling 28 (1): 31–36. doi:10.1021/ci00057a005.
- Wibowo, Y. M., M. Mudasir, and H. D. Pranowo. 2017. “Quantitative Structure-Activity Relationship Analysis of Organophosphate Insecticides Using Electronic and Molecular Parameters.” Makara Journal of Science 21 (3): 131–135. doi:10.7454/mss.v21i3.5965.
- Zhao, J., and S. Yu. 2013. “Quantitative Structure-Activity Relationship of Organophosphate Compounds Based on Molecular Interaction Fields Descriptors.” Environmental Toxicology and Pharmacology 35 (2): 228–234. doi:10.1016/j.etap.2012.11.018.