Abstract
Background: Deformable image registration (DIR) is increasingly used in the field of radiation therapy (RT) to account for anatomical deformations. The aims of this paper are to describe the main applications of DIR in RT and discuss current DIR evaluation methods.
Methods: Articles on DIR published from January 2000 to October 2018 were extracted from PubMed and Science Direct. Our search was restricted to articles that report data obtained from humans, were written in English, and address DIR methods for RT. A total of 207 articles were selected from among 2506 identified in the search process.
Results: At planning, DIR is used for organ delineation using atlas-based segmentation, deformation-based planning target volume definition, functional planning and magnetic resonance imaging-based dose calculation. In image-guided RT, DIR is used for contour propagation and dose calculation on per-treatment imaging. DIR is also used to determine the accumulated dose from fraction to fraction in external beam RT and brachytherapy, both for dose reporting and adaptive RT. In the case of re-irradiation, DIR can be used to estimate the cumulated dose of the two irradiations. Finally, DIR can be used to predict toxicity in voxel-wise population analysis. However, the evaluation of DIR remains an open issue, especially when dealing with complex cases such as the disappearance of matter. To quantify DIR uncertainties, most evaluation methods are limited to geometry-based metrics. Software companies have now integrated DIR tools into treatment planning systems for clinical use, such as contour propagation and fraction dose accumulation.
Conclusions: DIR is increasingly important in RT applications, from planning to toxicity prediction. DIR is routinely used to reduce the workload of contour propagation. However, its use for complex dosimetric applications must be carefully evaluated by combining quantitative and qualitative analyses.
Introduction
Deformable image registration (DIR) involves estimating the geometric transformation between two images to map them onto a common coordinate system (CCS). The process is deformable, or nonlinear, because the estimated transformation does not include only rigid transformations (i.e., translation and/or rotation) but also deformations (e.g., shrinking or stretching).
DIR has been extensively studied for radiation therapy (RT) applications, and its integration into clinical practice is currently the object of intensive research effort as shown by the report of the AAPM Task Group 132 [Citation1]. The main drivers behind the recent popularity of DIR for RT are the increasing number of acquired images, along with the development of multimodal imaging for planning, image-guided RT (IGRT) and adaptive RT (ART) during treatment delivery, and advances in image processing. Indeed, at planning, DIR is a particularly attractive tool to assist the segmentation when using an atlas-based model and for fusion in the case of multimodal imaging. Moreover, DIR can improve the definition of the planning target volume (PTV) through the use of population analysis. During fractionated treatment, in both external beam RT (EBRT) and brachytherapy (BT), managing organ deformations remains a significant clinical challenge. These deformations are caused by multiple factors such as patient motion, breathing, weight loss, tumors and organ at risk (OAR) shrinkage or organ filling. If not corrected, these anatomical variations in intra- or inter-fractions may lead to tumor underdosage and OAR overdosage, thus increasing the risk of recurrence or toxicity [Citation2]. In this context, DIR has been applied to monitor the deformations, and can therefore propagate the planning segmentations to per-treatment images (i.e., images acquired during the treatment delivery). DIR can then be used to estimate and monitor the cumulated dose in a deformable anatomical structure. Additionally, DIR has been used for toxicity prediction within a voxel-based analysis framework. Thus, it allows the identification of specific organ subregions that are associated with a high risk of toxicity.
These very promising applications of DIR have led many companies to include DIR tools in their commercial solutions. As these tools can be used for different purposes, the clinical applications have very different levels of maturity. From a clinician’s point of view, when considering the use of DIR for a specific application, it is important to understand its precise role, requirements and limitations, which differ markedly among applications.
This paper describes first the principle of DIR, the difficulties for DIR, the classic DIR methods used in RT and the DIR evaluation methods, in Supplementary Materials. The paper provides then a review of the main applications of DIR in RT: at planning to improve segmentation; during the treatment for IGRT, BT, ART and re-irradiation; and for toxicity prediction. It does not cover rigid registration.
Material and methods
We searched PubMed and Science Direct for articles published from January 2000 to October 2018 using the following query: (‘DIR’ OR ‘deformable image registration’ OR ‘elastic registration’ OR ‘non-rigid registration’) AND (‘radiotherapy’ OR ‘radiation therapy’). Our search was restricted to articles that report data obtained from humans, were written in English, and addressed DIR methods for EBRT and BT.
Results
The search returned a total of 2506 articles. As this paper is not intended as an exhaustive review, 207 articles were selected based on didactic considerations to present the most frequently used methods and the main clinical applications.
Deformable image registration: principle, difficulties, methods used in radiotherapy and evaluation
This chapter is provided in details as Supplementary Material.
DIR applications in RT
The following sections introduce the primary DIR applications in the RT workflow, along with their clinical benefits and limitations.
DIR at planning: atlas-based segmentation, multimodal image fusion, PTV definition, functional imaging and MRI-based dose calculation
The first step of treatment planning is the delineation of the organs of interest. Delineation is generally based on computed tomography (CT) images, although it can involve multimodal imaging. This task can be time consuming, requiring up to 2.5 h, for example, for the head and neck (H&N) region [Citation3].
Atlas-based segmentation
To perform automatic segmentation, atlas-based methods rely on DIR between the image to be segmented and the images of an atlas, for which the associated delineations are known. The atlas consists of a single image template or several templates (multi-atlas, typically at least 15 images, depending on the tumor localization) [Citation4] from patients with representative anatomy and organ delineations that have been validated by experts. The first step consists of identifying the most similar template image in terms of the image intensities, specific characteristics (e.g., patient gender, age, weight) or anatomical features (e.g., organ size, centroid position, orientation) [Citation5]. The DIR transformation between the template image and the image targeted for segmentation is then estimated and applied to the template delineations, allowing the contours to be propagated onto the anatomy of the new subject. Finally, the radiation oncologist must verify these contours and correct them if necessary. Atlas-based segmentation methods are useful for organ delineation, although they are not appropriate for the segmentation of tumors, which exhibit different shape characteristics and image features for different patients. However, atlas-based segmentation can be suitable for defining the nodal tumor volume, which is defined based on its anatomical localization. Selecting the right template is crucial, because a template that is more representative of the considered image will result in a transformation that is simpler and less prone to error. To reduce the uncertainties, the selection of a unique template can be replaced by the use of multiple templates [Citation6,Citation7]. In the latter case, the final segmentations over the multiple propagated contours is obtained by averaging or majority vote using the simultaneous truth and performance level estimation (STAPLE) or selective and iterative method for performance level estimation (SIMPLE) methods.
In prostate cancer, the selection of multiple templates with label fusion appears to be the best approach, yielding a Dice similarity coefficient (DSC) greater than 0.8 for the prostate, bladder and rectum [Citation7,Citation8]. In cervical cancer, the same approach can be used to assist the selection of a treatment plan from a planning library [Citation9]. The generated segmentations yielded a median DSC greater than 0.8, indicating the selection of the correct plan in 93% of tested cases. In H&N cancer, a standard atlas-based segmentation method resulted in OAR and nodal clinical target volume (CTV) segmentation with a mean DSC of around 0.7 [Citation10]. Manual correction helped to improve the mean DSC to above 0.8. Overall, the application of DIR to atlas-based methods has the ability to provide segmentations of a quality comparable to expert delineations [Citation6–10] while also reducing intra- and inter-observer delineation variability and decreasing the delineation time (e.g., up to 40% reduction for H&N [Citation10]). Atlas-based segmentation methods are currently offered in several treatment planning systems (TPSs) [Citation11,Citation12] and are routinely used in clinics.
Multimodal image fusion
Morphological and functional images, when considered as diagnostic images, play a crucial role in RT planning. For example, magnetic resonance imaging (MRI) and nuclear imaging (e.g., fluorodeoxyglucose-positron emission tomography (FDG-PET), single-photon emission-computed tomography (SPECT)) are used because of their ability to improve the tumor delineation [Citation13]. These multimodal images must therefore be fused to combine the associated information in a CCS. However, delineation could prove to be complex when anatomical deformations or body position variations occur during image acquisition, rendering ‘standard’ rigid registration inappropriate. For prostate delineation, MRI enables to clearly define the prostate contour as well as tumor volumes, whereas CT often overestimates this organ volume and does not show the tumor [Citation14]. DIR methods have therefore been used to propagate the delineation of the prostate from MRI to CT using a free-form deformation (FFD) [Citation15] or finite-element model (FEM) [Citation16]. In the treatment of cervical cancer, DIR has been used to propagate the pre-BT MRI to the planning CT. The resulting propagated high-risk CTV was shown to be smaller when using MRI than in CT [Citation17]. For H&N cancer, DIR has been used to propagate the gross tumor volume (GTV) from the diagnostic position MRI to the planning CT, thus improving the accuracy of the GTV delineation [Citation18]. DIR has also been applied in H&N cases to propagate CT/FDG-PET or MRI/FDG-PET to the planning CT [Citation19,Citation20], and is more accurate than rigid registration alone in terms of the target registration error (TRE), center of mass of the tumor, and normalized cross-correlation between the PET images. Finally, in lung cancer cases, DIR has been used to deform the static CT to a 4D MRI using a hybrid DIR method to generate a 4D CT for RT planning [Citation21].
DIR for deformation-based model PTV definition and functional planning
DIR has been used to quantify the mobility and deformation of organs within a population of patients. The deformations can then be quantified, at both the intra-patient scale (e.g., using 4D CT or early per-treatment imaging) and inter-patient scale (e.g., using planning or per-treatment imaging), thus generating a population-based deformation model. This model can then be applied to a given patient to define the PTV margins, dose coverage probability and functional imaging-based planning. In prostate cancer, the correspondence between inter- and intra-patient anatomies can be generated by DIR, allowing a statistical deformable motion model to be constructed [Citation22]. By accumulating the dominant deformations of the model, a population-based PTV could be defined based on prostate displacements, such as in a ‘standard’ approach population-based PTV, while accounting for deformations. The same method has been applied in cases of rectal cancer [Citation23]. The population-based PTV significantly improved sparing of OARs compared to uniform and nonuniform CTV-to-PTV margins. In cervical cancer, a DIR-based model has been generated to simulate different treatment scenarios and assess the dose coverage probability of the CTV and OAR [Citation24]. A similar DIR-based model was used to generate a patient-specific planning library that predicts systematic deformations of the uterus [Citation25]. A simpler use of surface-based DIR has been proposed to estimate intermediate positions of the uterus between full- and empty-bladder anatomies, allowing a planning library to be generated [Citation26]. The same approach has been used to construct a planning library for bladder cancer [Citation27]. Such adaptive planning library strategies, defined before RT treatment using DIR, improve the CTV coverage of numerous pelvic tumors [Citation28–30]. In the case of lung cancer, DIR can be used to estimate the deformation vector field (DVF) between each breathing phase of the 4D CT or the exhale/inhale images, resulting in functional imaging maps [Citation31,Citation32]. These maps can then be used to guide the intensity-modulated RT (IMRT) dose planning and spare functional regions, especially for patients who have high-functional lung adjacent to the PTV [Citation33]. However, the definition of these functional imaging maps is influenced by the choice of the DIR method, and should be used with caution [Citation34].
DIR for MRI-based dose calculation
With the development of the MRI-linear accelerator (LINAC), the dose calculation based on MRI appears to be especially crucial [Citation35–39]. Atlas-based approaches have been designed to generate, from a single MRI, a pseudo-CT (i.e., a virtual image that mimics CT densities on the MRI anatomy) from which the dose distribution can be calculated. The considered atlas consists of pairs of associated CT and MRI images, and the template MRI can be deformed toward that of the new patient. This deformation can therefore be used to propagate the electronic densities of the template CT onto the new patient MRI space, thus providing a pseudo-CT. Studies on prostate [Citation40,Citation41] and H&N [Citation42] have demonstrated that pseudo-CT images exhibit low Hounsfield unit differences compared with ground-truth CT images.
DIR in IGRT: contour propagation and fraction dose estimation
DIR for contour propagation
Three-dimensional per-treatment imaging (e.g., CT, cone-beam CT (CBCT) or MRI) is routinely employed for tumor positioning under the LINAC system. Per-treatment imaging using DIR can also be exploited to characterize anatomical deformations and their impact on the fraction dose. Thus, DIR has been used to propagate delineations from the planning CT to the per-treatment imaging (). A DIR transformation is computed between the planning CT and per-treatment image, thus providing a DVF. The DVF is then used to propagate the planning CT delineations to the per-treatment image. An expert must then validate and, if necessary, correct the delineations. For example, in prostate cancer, a non-parametric or FFD DIR method has been used to propagate the delineation from the planning CT to the per-treatment images (CT or CBCT) [Citation43–48]. Compared to rigid registration, DIR improved the accuracy of the organ delineation, achieving an increase in DSC. The largest and most complex deformations observed in the bladder and the rectum, however, cannot usually be fully handled by these DIR methods, and thus still require manual corrections [Citation47,Citation49]. Specific geometric approaches based on a B-spline interpolated transformation of the previously extracted salient points have therefore been developed, and these improve the registration to more than 90% accordance with manual contours [Citation50]. In cervical carcinoma, constrained FFD methods (using a prior deformable model or landmarks) have been used to propagate delineations between either intra-patient [Citation51,Citation52] or inter-patient MRIs [Citation53]. For intra-patient DIR, DSCs of approximately 0.85 were recorded for the bladder and uterus, which is comparable to expert delineation [Citation52]. For inter-patient DIR, large and complex deformations between inter-patient cervix-uterus shapes were poorly handled (mean DSC of 0.55 for the CTV) and required manual correction [Citation53]. In image-guided BT, DIR has been used to propagate high-risk CTV and OAR from fraction-to-fraction MRIs, producing clinically acceptable dose uncertainties [Citation54]. In liver cancer, a commercially available DIR method has been used to propagate abdominal organ delineations from the planning CT to the per-treatment CT with similar geometrical accuracy as inter-observer variability within 20 s per CT [Citation55]. A biomechanical-based DIR can propagate the GTV delineated on the planning CT to the per-treatment CBCT based on a liver surface mapping [Citation56]. This approach can be used to track the GTV during EBRT treatment for liver cancer when the per-treatment imaging contrast limits the visualization of the tumor. In H&N cancer, intra-patient DIR has been used to propagate the planning contour to the per-treatment CTs [Citation48,Citation57–59] and CBCTs [Citation60–62]. Additionally, when propagating the contours from the pretreatment MRI to the end of treatment MRI, DIR provided a contour error similar to the voxel size (2 mm) and a DSC of around 0.8 [Citation63]. Overall, DIR was consistently more accurate than rigid registration, with a mean DSC increase of 0.12–0.15 and better TRE (3 mm on average). In lung cancer and upper-abdominal malignancies, tumor volumes are manually delineated on each phase of the 4D CT [Citation64]. By enabling GTV propagation between the 4D CT phases [Citation65–70], DIR reduced the delineation time by a factor of 2 (from 40 min to 18 min) [Citation65,Citation66]. The propagated GTV delineations were similar to the manual delineations [Citation71], with a reported DSC greater than 0.76, which is similar to the reported intra-physician variation score [Citation65,Citation67]. Thus, DIR can reduce the time required for the delineation process while overcoming inter-observer variability. However, the DIR uncertainties require a physician to check and correct the propagated delineations. DIR can account for anatomical variations caused by breathing, which is particularly interesting for the incoming MRI-LINAC systems, as the delineated tumor at planning can be propagated onto the per-treatment 2D MRI for the purpose of gating the treatment beam [Citation72,Citation73].
Figure 1. Workflow of delineation propagation from the planning CT to per-treatment image (head-and-neck). The planning delineations are propagated by means of the deformation vector field estimated by DIR. The propagated delineations are validated by the physician and corrected if needed. DIR: deformable image registration.
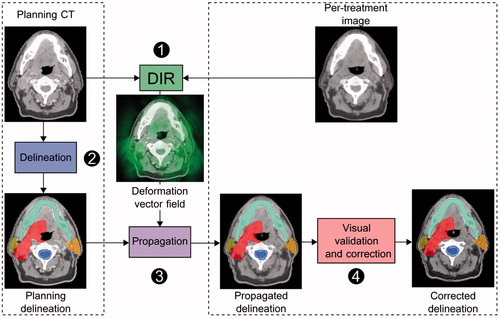
DIR for fraction dose calculation
If the planning contours can be effectively propagated to the per-treatment images, estimating the delivered fraction dose may become more difficult. Indeed, the planned dose distribution might not correspond to the delivered fraction dose distribution because of anatomical variations. In cases that involve large external contour and electronic density variations, the dose must be recalculated. When irradiating prostate cancer, the hypothesis of dose invariance (i.e., the dose map remains globally constant between the planning and the fraction) has been validated, except in the event of the significant appearance/disappearance of rectal gas or large external contour variations [Citation74,Citation75]. When irradiating locally advanced H&N cancer, weight loss and shrinking of the tumor and parotid gland mean that dose recalculation is needed. Thus, an image that represents the current anatomy with reliable electronic densities is required. DIR has therefore been used to propagate the Hounsfield unit from the planning CT to the per-treatment imaging (e.g., CBCT or megavoltage CT (MVCT)), generating a pseudo-CT that allows the fraction dose distribution to be calculated [Citation76,Citation77].
In terms of H&N cancer, several studies have evaluated the accuracy of DIR in generating the pseudo-CT in terms of the electronic density difference and dose calculation (i.e., dose difference and gamma index) [Citation60,Citation78–81]. The dose uncertainties were found to be small, and were considered ‘clinically acceptable.’ A similar approach to deform the planning CT toward the online CBCT was investigated in a cohort of five lung cancer patients. The approach was dosimetrically evaluated in a single patient to confirm the possibility of triggering a re-planning based on anatomical changes [Citation82]. However, the inherent noise, low contrast and limited field of view (FOV) in CBCT and MVCT make DIR methods challenging. Thus, preprocessing may be necessary to compute the dose on a corrected/modified CBCT [Citation78,Citation79,Citation83,Citation84].
DIR in ART for dose accumulation
Justification and principle of DIR for fraction dose accumulation
A crucial issue in RT is estimating the cumulated dose over the fractions, either to report the delivered dose or to compare with the planning dose (dose monitoring), to trigger, for example, re-planning. Indeed, in EBRT, the dose-volume histograms (DVHs) cannot be simply aggregated when considering deformable structures [Citation85–89]. Local anatomical variations must be accounted for in mapping the fraction doses to a CCS before summation. It is thought that DIR could be used to perform this mapping (). With the same approach as contour propagation from planning CT to per-treatment images, the DVF between the planning CT and per-treatment image can be applied to deform the fraction dose toward the planning anatomy. The deformed fraction doses propagated into a CCS in this way can then be summed. illustrates the difference between the direct addition of the DVH and the use of DIR to accumulate the DVH while using a numerical phantom of the pelvis [Citation90]. The significant difference in outcomes emphasizes the need for a DIR-based method to accumulate the doses. In BT, the direct addition of the DVHs overestimated the delivered dose compared to the more appropriate DIR approach [Citation91–95].
Figure 2. Workflow of cumulated dose estimation by deformable image registration. Step 1: a deformable image registration is computed between the per-treatment images and the planning CT image. Step 2: the fraction doses are calculated from the per-treatment images with the same treatment parameters as the planning. Step 3: the fraction dose distributions are propagated to the planning CT by means of the resulting deformation vector fields. Step 4: the propagated dose distributions are summed to compute the cumulated dose on the planning CT. The planned dose can be compared to the estimated cumulated dose.
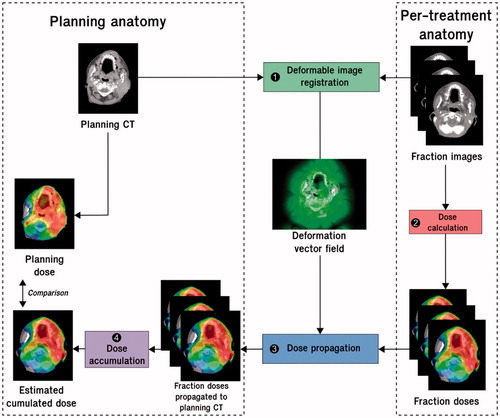
Figure 3. Cumulated DVH should not be estimated as the mean fraction DVH. DVH: Dose volume histogram. A pelvic numerical phantom was designed (A) to compare dose accumulation using ground truth accumulated dose (in red) and DVH averaging (in blue) in the bladder for prostate cancer irradiation. The fraction dose DVHs are in gray (normalized to the total dose). The ground truth accumulated dose was obtained by propagating the fraction doses to the planning using the reference DVF resulting from biomechanical laws (Supplementary Material). The cumulated dose appears superior to the planned dose. The mean fraction dose overestimates the dose received by the bladder.
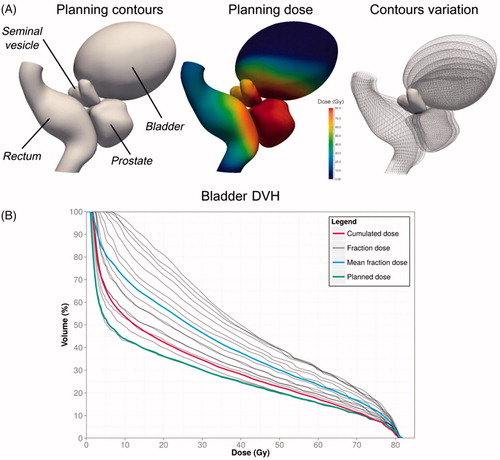
After the DVF estimation, two approaches can be used to propagate the dose accordingly. The first is to linearly interpolate the dose on the spatial grid of the fixed image; although, this does not consider the physical properties of the tissues in terms of the dose absorption [Citation96]. The second approach considers the tissue density when recalculating the deformed dose. Moreover, dose propagation methods should follow the principle of energy conservation [Citation97]. Monte Carlo-based dose propagation has proven to be accurate for regions that have heterogeneous electronic densities (e.g., the lungs) [Citation98], whereas linear interpolation-based dose propagation can be used for homogeneous regions (e.g., the pelvis).
Clinical applications of DIR for fraction dose accumulation in EBRT, BT and re-irradiation
DIR enables the accumulation of doses during EBRT and/or BT and, in the case of re-irradiation, in a large number of tumor localizations. In prostate cancer, OAR deformations mean that differences between the planning and cumulated dose have been quantified [Citation99,Citation100]. The mean dose difference is reportedly 7 Gy for the bladder and 2 Gy for the rectum [Citation99]. The use of DIR has also allowed the decrease in rectal dose when using a rectal balloon to be quantified (70% of patients showed a decrease of more than 5% in the normal tissue complication probability, NTCP) [Citation100]. Moreover, DIR can be used to accumulate the rectal dose of EBRT and high-dose-rate (HDR) BT [Citation101]. The accumulated dose was higher when using DIR (21.3% for D0.1cc, 6.3% for D1cc and 3.5% for D2cc) than that given by the direct addition of the DVH. In bladder cancer, DIR has been used to accumulate the treatment dose on the planning anatomy, allowing the comparison of several ART strategies [Citation102]. For cervical cancer, the use of DIR to accumulate the EBRT doses did not show large differences compared to the planned dose for the target; although, large dose differences have been observed for OARs [Citation103–105]. A recent study compared the performance of different RT and ART strategies in terms of accumulated dose for a single patient, showing good conformity with the geometric CTV-PTV coverage [Citation106]. In HDR-BT, DIR can also be useful to accumulate the fraction doses caused by the large deformations resulting from organs filling and intracavity applicator (re)insertion [Citation91,Citation107–109]. Moreover, DIR has been used to deform the EBRT planning CT anatomy toward the planning CT/MRI anatomy at the time of BT [Citation110], allowing the cumulated received dose to the rectum and bladder to be estimated [Citation111]. However, uncertainties in the dose accumulation are linked to the DIR accuracy and the EBRT technique (i.e., steep dose gradient) [Citation92,Citation112,Citation113]. Moreover, the ground-truth is unknown, thus making the evaluation of cumulated dose more difficult. The clinical benefit of DIR in this context, therefore, must be evaluated [Citation95]. In H&N cancer, weight loss along with the shrinking of the tumor and parotid gland during treatment can lead to large differences between planned and delivered doses [Citation114]. DIR methods estimated a mean overdose in excess of 3 Gy for at least 30% (up to 60%) of parotid glands [Citation3,Citation115–118]. However, a study on 133 H&N patients with MVCT image-guidance reported only a small deviation in the spinal cord delivered dose estimated by DIR in the case of large anatomical changes [Citation119]. In lung cancer, breathing causes anatomical variations during treatment delivery, which motivates the use of DIR to accumulate the dose for each individual fraction. Using biomechanical model-based DIR for the planning 4D CT and per-treatment 4D CBCT of 10 breathing phases, the average dose difference between the accumulated and planned dose, considering the minimum dose to 0.5 cm3 of the tumor, was quantified as 0.8 Gy; although, the difference was more than 2 Gy for 30% of patients [Citation120]. Other studies have investigated the impact of breathing on dose accumulation, suggesting large variations in the OARs [Citation121,Citation122] and none at the target [Citation71].
Finally, DIR has been used in the case of re-irradiation to propagate the dose from the first planning to the re-irradiation planning, such as in H&N, brain, liver, mediastinum and lung cancer [Citation85,Citation87,Citation123]. DIR appears, therefore, to be especially helpful for guiding radiation oncologists in irradiating the recurrence while avoiding increased toxicity. However, the use of DIR in this situation appears challenging because of the significant anatomical differences, especially when related to matter (dis)appearance between the two treatments.
DIR for toxicity prediction via voxel-wise population analysis
DIR can also be applied to investigate the local relationships between dose and side-effects by analyzing the dose at finer scales via voxel-wise population analysis, thus revealing local differences across individuals. Such methods are inspired by voxel-based morphometry (VBM), which typically assesses the differences between groups at each voxel and relates them to different covariates (e.g., age, gender, diagnosis and cognitive scores). As mentioned before, the toxicity studies have allowed the identification of more predictive subregions within the organs [Citation124–126]. Mapping the dose to a CCS remains a central question: the map can be obtained via a parametric representation of the anatomy in a spherical coordinate system [Citation124], or can be more precisely computed through tailored DIR [Citation127]. However, voxel-based methods require different steps, as shown in : (i) the mapping by DIR of a population of individuals onto an anatomical template (steps 1 and 2); (ii) the propagation of dose distributions according to the obtained DVFs (step 3); and (iii) a local statistical analysis of the dose–effect relationship (step 4) that allows anatomical subregions that are at high risk of toxicity to be identified.
Figure 4. Workflow of voxel-based analysis using deformable image registration for patient toxicity prediction. Step 1: feature extraction is done on the population data and anatomical template (i.e., preprocessing); step 2: a DIR method is used to compute the inter-individual deformation vector fields (DVF). Step 3: the resulting DVFs are used to propagate the 3D planning dose distributions of the population on the anatomical template coordinate system; step 4: a local statistical analysis of dose–effect relationship is performed.
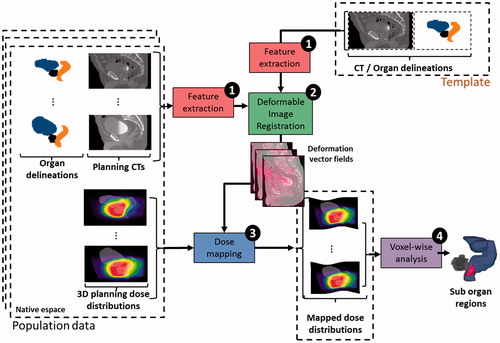
In prostate cancer IMRT, DIR has enabled antero-inferior parts of the anorectum to be identified as highly predictive of rectal bleeding [Citation125,Citation126]. This subregion was found to receive a significantly higher dose (up to 6.8 Gy) in patients with toxicity symptoms compared with nontoxic patients. In H&N cancer, inter-patient DIR was used to compare the local dose of patients with and without acute dysphagia. Two subregions (cricopharyngeus muscle and cervical esophagus) were found to have significant differences in terms of the received dose (by more than 10 Gy) [Citation128]. Finally, in lung cancer cases, DIR was used to perform voxel-by-voxel analysis to assess the relationship between a local received dose and lung injury [Citation129]. For each patient, a probability model was developed to represent the risk of severe lung injury under the received dose. Moreover, the peripheral medial-basal portion of the lungs was found to receive a higher mean dose in patients with lung damage [Citation130].
In summary, the identified anatomical subregions can be used for patient toxicity prediction and/or specific OAR definition, and must be particularly spared at the time of planning to decrease the toxicity.
Discussion
Uncertainties and perspectives of DIR in RT
DIR is validated in routine treatment for delineation at the planning and per-treatment contour propagation [Citation1]. Its accuracy appears to be similar to the inter-observer variability in prostate and H&N localization [Citation48,Citation131]. However, its contribution is still limited for CT-CBCT DIR of anatomical localization showing large deformations during EBRT. Moreover, because of the limited number of dosimetric studies and the absence of published clinical studies, the use of DIR for dose accumulation is still under evaluation and cannot be used directly in clinical practice [Citation3,Citation132]. Indeed, the use of DIR for dose accumulation is subject to uncertainties that are linked to multiple parameters, such as the DIR algorithm’s performance, the order in which the considered images are registered (i.e., inverse consistency), the lack of contrast between images, the conservation of mass, tissue sliding and the dose mapping method (linear interpolation or Monte-Carlo). The performance of various DIR algorithms has been compared to quantify dose accumulation in several anatomical localizations [Citation57,Citation88,Citation117,Citation133–138]. Although volume-of-interest-based metrics provide some idea of dose accumulation uncertainties [Citation135,Citation137], the dose mapping error remains very difficult to quantify [Citation136].
With the aim of improving the DIR accuracy, some commercial software packages now provide the ability to drive the DVF using contours [Citation127,Citation139] or corresponding points [Citation140]. For this purpose, specific tools, developed in academic institutions, are imported to commercial RT TPS [Citation12,Citation139,Citation141]. Therefore, DIR algorithms require further development to better meet clinical needs, such as accounting for the different imaging modalities between planning and treatment delivery [Citation133,Citation142], near-real-time algorithms with graphic processing unit-based frameworks [Citation139,Citation143–145], and anatomical properties simulated by finite element models [Citation146–150].
Issues in the choice of the DIR evaluation method
While numerous studies have compared the performance of different DIR methods (commercial, open access or homemade) for RT applications [Citation57,Citation117,Citation151–159], one of the main challenges that faces DIR evaluation is its adaptation to each considered application. For example, dose monitoring requires low point-to-point error, whereas delineation propagation generally only requires good correspondence between the organ boundaries. Moreover, DIR evaluation for dose monitoring must include dosimetric indices (e.g., mean dose, homogeneity index, dose–response indices), as any given geometric error could cause different dosimetric errors depending on the dose gradient (i.e., high dosimetric errors in cases of high dose gradients) [Citation149]. A perfect point-to-point matching is probably an unreachable goal considering the voxel size, artifacts and realistic deformation constraints. Owing to the large number of DIR methods, standard evaluation criteria must be defined and generalized [Citation153,Citation160]. The development of numerical phantoms is a first step toward comparing and validating DIR methods, particularly for dose accumulation. Moreover, challenge datasets are available online, enabling the evaluation and comparison of various homemade DIR methods [Citation161–165]. More advanced techniques, such as neural networks, could also be used to quantify DIR performance [Citation166].
However, a number of particular situations will always defeat DIR methods. Thus, even after a thorough evaluation study, each individual registration should be evaluated. In general, qualitative and quantitative evaluation methods should be combined to improve the robustness of the evaluation process, e.g., the quantitative scores may be used to guide the qualitative analysis. Moreover, geometric evaluation should always be verified by DVF analysis to ensure that the DIR provides a physically plausible solution.
Conclusions
Although, there has been significant progress in the development of DIR in RT, further developments are required to enable its exploitation in the clinical workflow. DIR in RT is complex in terms of both methods and applications. As recently recommended by the AAPM Task Group 132 [Citation1], DIR can be used at planning and during IGRT for autosegmentation and multimodality fusion to reduce the delineation workload, especially given the increasing number of images generated for each patient. Using DIR to assess the accumulated dose could be the answer to an unmet clinical need, namely the need to estimate the potential deviation from the planned dose. DIR also enables a comparison of different RT strategies, mainly ART, in terms of the delivered dose. Nevertheless, DIR must be used with caution, as this more complex application requires high local accuracy. Indeed, DIR evaluation must consider both geometrical and dosimetric metrics, which thus requires sophisticated dedicated tools such as numerical phantoms. Moreover, a consensus on the evaluation method and criteria is required before its potential contribution in the clinical workflow can be assessed. However, given that each considered situation is unique (e.g., patient anatomy, image noise), the evaluation must also be performed at the individual scale [Citation167].
Supplemental Material
Download Zip (16.4 MB)Disclosure statement
G. Cazoulat has a competing interest with RaySearch Laboratories (Stockholm, Sweden). All other authors have no potential conflict of interest.
References
- Brock KK, Mutic S, McNutt TR, et al. Use of image registration and fusion algorithms and techniques in radiotherapy: report of the AAPM Radiation Therapy Committee Task Group No. 132. Med Phys. 2017;44:e43–e76.
- de Crevoisier R, Tucker SL, Dong L, et al. Increased risk of biochemical and local failure in patients with distended rectum on the planning CT for prostate cancer radiotherapy. Int J Radiat Oncol Biol Phys. 2005;62:965–973.
- Castelli J, Simon A, Louvel G, et al. Impact of head and neck cancer adaptive radiotherapy to spare the parotid glands and decrease the risk of xerostomia. Radiat Oncol. 2015;10:6.
- Han X, Hoogeman MS, Levendag PC, et al., editors. Atlas-based auto-segmentation of head and neck CT images. International Conference on Medical Image Computing and Computer-assisted Intervention. Springer; 2008.
- Acosta O, Mylona E, Le Dain M, et al. Multi-atlas-based segmentation of prostatic urethra from planning CT imaging to quantify dose distribution in prostate cancer radiotherapy. Radiother Oncol. 2017;125:492–499.
- Acosta O, Simon A, Monge F, et al., editors. Evaluation of multi-atlas-based segmentation of CT scans in prostate cancer radiotherapy. 2011 IEEE International Symposium on Biomedical Imaging: From Nano to Macro. IEEE; 2011.
- Dowling JA, Fripp J, Chandra S, et al. Fast automatic multi-atlas segmentation of the prostate from 3D MR images. Prostate Cancer Imaging. Image Analysis and Image-Guided Interventions. Springer; 2011. p. 10–21.
- Acosta O, Dowling J, Drean G, et al. Multi-atlas-based segmentation of pelvic structures from CT scans for planning in prostate cancer radiotherapy. In: Abdomen and Thoracic Imaging. Boston, (MA): Springer; 2014. p. 623-656.
- Langerak T, Heijkoop S, Quint S, et al. Towards automatic plan selection for radiotherapy of cervical cancer by fast automatic segmentation of cone beam CT scans. Medical Image Computing and Computer-Assisted Intervention – MICCAI 2014. Springer; 2014. p. 528–535.
- Daisne J-F, Blumhofer A. Atlas-based automatic segmentation of head and neck organs at risk and nodal target volumes: a clinical validation. Radiat Oncol. 2013;8:154.
- La Macchia M, Fellin F, Amichetti M, et al. Systematic evaluation of three different commercial software solutions for automatic segmentation for adaptive therapy in head-and-neck, prostate and pleural cancer. Radiat Oncol. 2012;7:160.
- Bodensteiner D. RayStation: external beam treatment planning system. Med Dosim. 2018;43:168–176.
- Farina E, Ferioli M, Castellucci P, et al. 18F-Fdg-PET-guided planning and re-planning (adaptive) radiotherapy in head and neck cancer: current state of art. Anticancer Res. 2017;37:6523–6532.
- Seppälä T, Visapää H, Collan J, et al. Converting from CT- to MRI-only-based target definition in radiotherapy of localized prostate cancer. Strahlenther Onkol. 2015;191:862–868.
- Sabater S, del Rosario Pastor-Juan M, Berenguer R, et al. Analysing the integration of MR images acquired in a non-radiotherapy treatment position into the radiotherapy workflow using deformable and rigid registration. Radiother Oncol. 2016;119:179–184.
- Zhong H, Wen N, Gordon JJ, et al. An adaptive MR-CT registration method for MRI-guided prostate cancer radiotherapy. Phys Med Biol. 2015;60:2837.
- Tait LM, Hoffman D, Benedict S, et al. The use of MRI deformable image registration for CT-based brachytherapy in locally advanced cervical cancer. Brachytherapy. 2016;15:333–340.
- Chuter R, Prestwich R, Bird D, et al. The use of deformable image registration to integrate diagnostic MRI into the radiotherapy planning pathway for head and neck cancer. Radiother Oncol. 2017;122:229–235.
- Leibfarth S, Mönnich D, Welz S, et al. A strategy for multimodal deformable image registration to integrate PET/MR into radiotherapy treatment planning. Acta Oncol. 2013;52:1353–1359.
- Hwang AB, Bacharach SL, Yom SS, et al. Can positron emission tomography (PET) or PET/computed tomography (CT) acquired in a nontreatment position be accurately registered to a head-and-neck radiotherapy planning CT? Int J Radiat Oncol Biol Phys. 2009;73:578–584.
- Yang Y, Teo SK, Van Reeth E, et al. A hybrid approach for fusing 4D-MRI temporal information with 3D-CT for the study of lung and lung tumor motion. Med Phys. 2015;42:4484–4496.
- Thörnqvist S, Hysing LB, Zolnay AG, et al. Adaptive radiotherapy in locally advanced prostate cancer using a statistical deformable motion model. Acta Oncol. 2013;52:1423–1429.
- Bondar L, Intven M, Burbach JM, et al. Statistical modeling of CTV motion and deformation for IMRT of early-stage rectal cancer. Int J Radiat Oncol Biol Phys. 2014;90:664–672.
- Tilly D, van de Schoot AJ, Grusell E, et al. Dose coverage calculation using a statistical shape model—applied to cervical cancer radiotherapy. Phys Med Biol. 2017;62:4140.
- Rigaud B, Simon A, Gobeli M, et al. Statistical shape model to generate a planning library for cervical adaptive radiotherapy. IEEE Trans Med Imaging. 2019;38:406–416.
- Heijkoop ST, Langerak TR, Quint S, et al. Clinical implementation of an online adaptive plan-of-the-day protocol for nonrigid motion management in locally advanced cervical cancer IMRT. Int J Radiat Oncol Biol Phys. 2014;90:673–679.
- Lutkenhaus LJ, Visser J, de Jong R, et al. Evaluation of delivered dose for a clinical daily adaptive plan selection strategy for bladder cancer radiotherapy. Radiother Oncol. 2015;116:51–56.
- Ghose S, Holloway L, Lim K, et al. A review of segmentation and deformable registration methods applied to adaptive cervical cancer radiation therapy treatment planning. Artif Intell Med. 2015;64:75–87.
- Collins SD, Leech MM. A review of plan library approaches in adaptive radiotherapy of bladder cancer. Acta Oncol. 2018;57:566–573.
- Thörnqvist S, Hysing LB, Tuomikoski L, et al. Adaptive radiotherapy strategies for pelvic tumors – a systematic review of clinical implementations. Acta Oncol. 2016;55:943–958.
- Kipritidis J, Hugo G, Weiss E, et al. Measuring interfraction and intrafraction lung function changes during radiation therapy using four‐dimensional cone beam CT ventilation imaging. Med Phys. 2015;42:1255–1267.
- Yamamoto T, Kabus S, Klinder T, et al. Investigation of four-dimensional computed tomography-based pulmonary ventilation imaging in patients with emphysematous lung regions. Phys Med Biol. 2011;56:2279.
- Yamamoto T, Kabus S, Von Berg J, et al. Impact of four-dimensional computed tomography pulmonary ventilation imaging-based functional avoidance for lung cancer radiotherapy. Int J Radiat Oncol Biol Phys. 2011;79:279–288.
- Yamamoto T, Kabus S, Klinder T, et al. Four‐dimensional computed tomography pulmonary ventilation images vary with deformable image registration algorithms and metrics. Med Phys. 2011;38:1348–1358.
- Freyhardt P, Hartwig T, De Bucourt M, et al. MR-guided facet joint injection therapy using an open 1.0-T MRI system: an outcome study. Eur Radiol. 2013;23:3296–3303.
- Van Heijst TC, Den Hartogh MD, Lagendijk JJ, et al. MR-guided breast radiotherapy: feasibility and magnetic-field impact on skin dose. Phys Med Biol. 2013;58:5917.
- Lagendijk JJ, Raaymakers BW, van Vulpen M. The magnetic resonance imaging-linac system. Semin Radiat Oncol. 2014;24:207–209.
- Owrangi AM, Greer PB, Glide-Hurst CK. MRI-only treatment planning: benefits and challenges. Phys Med Biol. 2018;63:05TR01.
- Tenhunen M, Korhonen J, Kapanen M, et al. MRI-only based radiation therapy of prostate cancer: workflow and early clinical experience. Acta Oncol. 2018;57:902–907.
- Dowling JA, Lambert J, Parker J, et al. An atlas-based electron density mapping method for magnetic resonance imaging (MRI)-alone treatment planning and adaptive MRI-based prostate radiation therapy. Int J Radiat Oncol Biol Phys. 2012;83:e5–e11.
- Chen S, Quan H, Qin A, et al. MR image‐based synthetic CT for IMRT prostate treatment planning and CBCT image‐guided localization. J Appl Clin Med Phys. 2016;17:236–245.
- Burgos N, Cardoso MJ, Guerreiro F, et al., editors. Robust CT synthesis for radiotherapy planning: application to the head and neck region. International Conference on Medical Image Computing and Computer-Assisted Intervention. Springer; 2015.
- König L, Derksen A, Papenberg N, et al. Deformable image registration for adaptive radiotherapy with guaranteed local rigidity constraints. Radiat Oncol. 2016;11:122.
- Gardner SJ, Wen N, Kim J, et al. Contouring variability of human-and deformable-generated contours in radiotherapy for prostate cancer. Phys Med Biol. 2015;60:4429.
- Kim J, Kumar S, Liu C, et al. A novel approach for establishing benchmark CBCT/CT deformable image registrations in prostate cancer radiotherapy. Phys Med Biol. 2013;58:8077.
- Thor M, Bentzen L, Elstrøm UV, et al. Dose/volume-based evaluation of the accuracy of deformable image registration for the rectum and bladder. Acta Oncol. 2013;52:1411–1416.
- Thor M, Petersen JB, Bentzen L, et al. Deformable image registration for contour propagation from CT to cone-beam CT scans in radiotherapy of prostate cancer. Acta Oncol. 2011;50:918–925.
- Riegel AC, Antone JG, Zhang H, et al. Deformable image registration and interobserver variation in contour propagation for radiation therapy planning. J Appl Clin Med Phys. 2016;17:347–357.
- Thörnqvist S, Petersen JB, Høyer M, et al. Propagation of target and organ at risk contours in radiotherapy of prostate cancer using deformable image registration. Acta Oncol. 2010;49:1023–1032.
- Xie Y, Chao M, Lee P, et al. Feature-based rectal contour propagation from planning CT to cone beam CT. Med Phys. 2008;35:4450–4459.
- Van Der Put R, Kerkhof E, Raaymakers B, et al. Contour propagation in MRI-guided radiotherapy treatment of cervical cancer: the accuracy of rigid, non-rigid and semi-automatic registrations. Phys Med Biol. 2009;54:7135.
- Lu C, Chelikani S, Jaffray DA, et al. Simultaneous nonrigid registration, segmentation, and tumor detection in MRI guided cervical cancer radiation therapy. IEEE Trans Med Imaging. 2012;31:1213–1227.
- Berendsen FF, Van Der Heide UA, Langerak TR, et al. Free-form image registration regularized by a statistical shape model: application to organ segmentation in cervical MR. Comput Vision Image Understand. 2013;117:1119–1127.
- Chapman CH, Polan D, Vineberg K, et al. Deformable image registration-based contour propagation yields clinically acceptable plans for MRI-based cervical cancer brachytherapy planning. Brachytherapy. 2018;17:360–367.
- Gupta V, Wang Y, Romero AM, et al. Fast and robust adaptation of organs-at-risk delineations from planning scans to match daily anatomy in pre-treatment scans for online-adaptive radiotherapy of abdominal tumors. Radiother Oncol. 2018;127:332–338.
- Brock KK, Hawkins M, Eccles C, et al. Improving image-guided target localization through deformable registration. Acta Oncol. 2008;47:1279–1285.
- Rigaud B, Simon A, Castelli J, et al. Evaluation of deformable image registration methods for dose monitoring in head and neck radiotherapy. Biomed Res Int. 2015;2015:726268.
- Kumarasiri A, Siddiqui F, Liu C, et al. Deformable image registration based automatic CT‐to‐CT contour propagation for head and neck adaptive radiotherapy in the routine clinical setting. Med Phys. 2014;41:121712.
- Ramadaan IS, Peick K, Hamilton DA, et al. Validation of Varian’s SmartAdapt® deformable image registration algorithm for clinical application. Radiat Oncol. 2015;10:73.
- Veiga C, McClelland J, Moinuddin S, et al. Toward adaptive radiotherapy for head and neck patients: feasibility study on using CT-to-CBCT deformable registration for "dose of the day" calculations. Med Phys. 2014;41:031703.
- Hou J, Guerrero M, Chen W, et al. Deformable planning CT to cone-beam CT image registration in head-and-neck cancer. Med Phys. 2011;38:2088–2094.
- Hvid CA, Elstrøm UV, Jensen K, et al. Accuracy of software-assisted contour propagation from planning CT to cone beam CT in head and neck radiotherapy. Acta Oncol. 2016;55:1324–1330.
- Broggi S, Scalco E, Belli ML, et al. A comparative evaluation of 3 different free-form deformable image registration and contour propagation methods for head and neck MRI: the case of parotid changes during radiotherapy. Technol Cancer Res Treat. 2017;16:373–381.
- Louie AV, Rodrigues G, Olsthoorn J, et al. Inter-observer and intra-observer reliability for lung cancer target volume delineation in the 4D-CT era. Radiother Oncol. 2010;95:166–171.
- Gaede S, Olsthoorn J, Louie AV, et al. An evaluation of an automated 4D-CT contour propagation tool to define an internal gross tumour volume for lung cancer radiotherapy. Radiother Oncol. 2011;101:322–328.
- Speight R, Sykes J, Lindsay R, et al. The evaluation of a deformable image registration segmentation technique for semi-automating internal target volume (ITV) production from 4DCT images of lung stereotactic body radiotherapy (SBRT) patients. Radiother Oncol. 2011;98:277–283.
- van Dam IE, de Koste J, Hanna GG, et al. Improving target delineation on 4-dimensional CT scans in stage I NSCLC using a deformable registration tool. Radiother Oncol. 2010;96:67–72.
- Stützer K, Haase R, Lohaus F, et al. Evaluation of a deformable registration algorithm for subsequent lung computed tomography imaging during radiochemotherapy. Med Phys. 2016;43:5028–5039.
- Hardcastle N, Hofman MS, Hicks RJ, et al. Accuracy and utility of deformable image registration in 68 Ga 4D PET/CT assessment of pulmonary perfusion changes during and after lung radiation therapy. Int J Radiat Oncol Biol Phys. 2015;93:196–204.
- Al‐Ward SM, Kim A, McCann C, et al. The development of a 4D treatment planning methodology to simulate the tracking of central lung tumors in an MRI‐linac. J Appl Clin Med Phys. 2017;19:145–155.
- de Xivry JO, Janssens G, Bosmans G, et al. Tumour delineation and cumulative dose computation in radiotherapy based on deformable registration of respiratory correlated CT images of lung cancer patients. Radiother Oncol. 2007;85:232–238.
- Mazur TR, Fischer-Valuck BW, Wang Y, et al. SIFT-based dense pixel tracking on 0.35 T cine-MR images acquired during image-guided radiation therapy with application to gating optimization. Med Phys. 2015;43:279–293.
- Fast MF, Eiben B, Menten MJ, et al. Tumour auto-contouring on 2d cine MRI for locally advanced lung cancer: a comparative study. Radiother Oncol. 2017;125:485–491.
- Sharma M, Weiss E, Siebers JV. Dose deformation-invariance in adaptive prostate radiation therapy: implication for treatment simulations. Radiother Oncol. 2012;105:207–213.
- Simon A, Le Maitre A, Nassef M, et al. Is dose deformation-invariance hypothesis verified in prostate IGRT? Int J Radiat Oncol Biol Phys. 2017;97:830–838.
- Moteabbed M, Sharp G, Wang Y, et al. Validation of a deformable image registration technique for cone beam CT-based dose verification. Med Phys. 2015;42:196–205.
- Lou J, Huang P, Ma C, et al. Parotid gland radiation dose‐xerostomia relationships based on actual delivered dose for nasopharyngeal carcinoma. J Appl Clin Med Phys. 2018;19:251–260.
- Arai K, Kadoya N, Kato T, et al. Feasibility of CBCT-based proton dose calculation using a histogram-matching algorithm in proton beam therapy. Phys Med. 2017;33:68–76.
- Kurz C, Kamp F, Park YK, et al. Investigating deformable image registration and scatter correction for CBCT-based dose calculation in adaptive IMPT. Med Phys. 2016;43:5635–5646.
- Landry G, Nijhuis R, Dedes G, et al. Investigating CT to CBCT image registration for head and neck proton therapy as a tool for daily dose recalculation. Med Phys. 2015;42:1354–1366.
- Branchini M, Fiorino C, Dell'Oca I, et al. Validation of a method for “dose of the day” calculation in head-neck tomotherapy by using planning ct-to-MVCT deformable image registration. Phys Med. 2017;39:73–79.
- Cole A, Veiga C, Johnson U, et al. Toward adaptive radiotherapy for lung patients: feasibility study on deforming planning CT to CBCT to assess the impact of anatomical changes on dosimetry. Phys Med Biol. 2018;63:155014.
- Onozato Y, Kadoya N, Fujita Y, et al. Evaluation of on-board kV cone beam computed tomography-based dose calculation with deformable image registration using Hounsfield unit modifications. Int J Radiat Oncol Biol Phys. 2014;89:416–423.
- Marchant TE, Joshi KD, Moore CJ. Accuracy of radiotherapy dose calculations based on cone-beam CT: comparison of deformable registration and image correction based methods. Phys Med Biol. 2018;63:065003.
- Boman E, Kapanen M, Pickup L, et al. Importance of deformable image registration and biological dose summation in planning of radiotherapy retreatments. Med Dosim. 2017;42:296–303.
- Andersen E, Muren L, Sørensen TS, et al. Bladder dose accumulation based on a biomechanical deformable image registration algorithm in volumetric modulated arc therapy for prostate cancer. Phys Med Biol. 2012;57:7089.
- Lee DS, Woo J-Y, Kim JW, et al. Re-irradiation of hepatocellular carcinoma: clinical applicability of deformable image registration. Yonsei Med J. 2016;57:41–49.
- Thor M, Andersen ES, Petersen JB, et al. Evaluation of an application for intensity-based deformable image registration and dose accumulation in radiotherapy. Acta Oncol. 2014;53:1329–1336.
- Kvinnsland Y, Muren LP, Dahl O. Evaluation of a new method for calculation of cumulative doses in the rectum wall using repeat CT scans. Acta Oncol. 2004;43:388–395.
- Rubeaux M, Cazoulat G, Duménil A, et al. Numerical phantom generation to evaluate non-rigid CT/CBCT registration algorithms for prostate cancer radiotherapy. Nice (France): Image-Guidance and Multimodal Dose Planning in Radiation Therapy; 2012. p. 74–81.
- Kobayashi K, Murakami N, Wakita A, et al. Dosimetric variations due to interfraction organ deformation in cervical cancer brachytherapy. Radiother Oncol. 2015;117:555–558.
- Teo B-K, Millar LPB, Ding X, et al. Assessment of cumulative external beam and intracavitary brachytherapy organ doses in gynecologic cancers using deformable dose summation. Radiother Oncol. 2015;115:195–202.
- Jamema SV, Mahantshetty U, Andersen E, et al. Uncertainties of deformable image registration for dose accumulation of high-dose regions in bladder and rectum in locally advanced cervical cancer. Brachytherapy. 2015;14:953–962.
- Andersen ES, Noe KØ, Sørensen TS, et al. Simple DVH parameter addition as compared to deformable registration for bladder dose accumulation in cervix cancer brachytherapy. Radiother Oncol. 2013;107:52–57.
- van Heerden LE, Houweling AC, Koedooder K, et al. Structure-based deformable image registration: added value for dose accumulation of external beam radiotherapy and brachytherapy in cervical cancer. Radiother Oncol. 2017;123:319–324.
- Rosu M, Chetty IJ, Balter JM, et al. Dose reconstruction in deforming lung anatomy: dose grid size effects and clinical implications. Med Phys. 2005;32:2487–2495.
- Zhong H, Chetty IJ. Adaptive radiotherapy for NSCLC patients: utilizing the principle of energy conservation to evaluate dose mapping operations. Phys Med Biol. 2017;62:4333.
- Zhong H, Siebers JV. Monte Carlo dose mapping on deforming anatomy. Phys Med Biol. 2009;54:5815.
- Nassef M, Simon A, Cazoulat G, et al. Quantification of dose uncertainties in cumulated dose estimation compared to planned dose in prostate IMRT. Radiother Oncol. 2016;119:129–136.
- Yu J, Hardcastle N, Jeong K, et al. On voxel-by-voxel accumulated dose for prostate radiation therapy using deformable image registration. Technol Cancer Res Treat. 2015;14:37–47.
- Moulton CR, House MJ, Lye V, et al. Accumulation of rectum dose‐volume metrics for prostate external beam radiotherapy combined with brachytherapy: evaluating deformably registered dose distribution addition using parameter‐based addition. J Med Imaging Radiat Oncol. 2017;61:534–542.
- Vestergaard A, Muren LP, Søndergaard J, et al. Adaptive plan selection vs. re-optimisation in radiotherapy for bladder cancer: a dose accumulation comparison. Radiother Oncol. 2013;109:457–462.
- Lim K, Kelly V, Stewart J, et al. Pelvic radiotherapy for cancer of the cervix: is what you plan actually what you deliver? Int J Radiat Oncol Biol Phys. 2009;74:304–312.
- Stewart J, Lim K, Kelly V, et al. Automated weekly replanning for intensity-modulated radiotherapy of cervix cancer. Int J Radiat Oncol Biol Phys. 2010;78:350–358.
- Oh S, Stewart J, Moseley J, et al. Hybrid adaptive radiotherapy with on-line MRI in cervix cancer IMRT. Radiother Oncol. 2014;110:323–328.
- Rigaud B, Simon A, Gobeli M, et al. CBCT-guided evolutive library for cervical adaptive IMRT. Med Phys. 2018;45:1379–1390.
- Reniers B, Janssens G, de Xivry JO, et al. Dose distribution for gynecological brachytherapy with dose accumulation between insertions: feasibility study. Brachytherapy. 2016;15:504–513.
- Zhen X, Chen H, Yan H, et al. A segmentation and point-matching enhanced efficient deformable image registration method for dose accumulation between HDR CT images. Phys Med Biol. 2015;60:2981.
- Ryckman JM, Shelton JW, Waller AF, et al. Anatomic structure-based deformable image registration of brachytherapy implants in the treatment of locally advanced cervix cancer. Brachytherapy. 2016;15:584–592.
- Kim H, Huq MS, Houser C, et al. Mapping of dose distribution from IMRT onto MRI-guided high dose rate brachytherapy using deformable image registration for cervical cancer treatments: preliminary study with commercially available software. JCB. 2014;6:178.
- Abe T, Tamaki T, Makino S, et al. Assessing cumulative dose distributions in combined radiotherapy for cervical cancer using deformable image registration with pre-imaging preparations. Radiat Oncol. 2014;9:293.
- Kadoya N, Miyasaka Y, Nakajima Y, et al. Evaluation of deformable image registration between external beam radiotherapy and HDR brachytherapy for cervical cancer with a 3D‐printed deformable pelvis phantom. Med Phys. 2017;44:1445–1455.
- Moulton CR, House MJ, Lye V, et al. Registering prostate external beam radiotherapy with a boost from high-dose-rate brachytherapy: a comparative evaluation of deformable registration algorithms. Radiat Oncol. 2015;10:254.
- Castelli J, Simon A, Lafond C, et al. Adaptive radiotherapy for head and neck cancer. Acta Oncol. 2018;57:1284–1292.
- Zhang P, Simon A, Rigaud B, et al. Optimal adaptive IMRT strategy to spare the parotid glands in oropharyngeal cancer. Radiother Oncol. 2016;120:41–47.
- Elstrøm UV, Wysocka BA, Muren LP, et al. Daily kV cone-beam CT and deformable image registration as a method for studying dosimetric consequences of anatomic changes in adaptive IMRT of head and neck cancer. Acta Oncol. 2010;49:1101–1108.
- Nobnop W, Chitapanarux I, Neamin H, et al. Evaluation of deformable image registration (DIR) methods for dose accumulation in nasopharyngeal cancer patients during radiotherapy. Radiol Oncol. 2017;51:438–446.
- Castelli J, Simon A, Rigaud B, et al. A nomogram to predict parotid gland overdose in head and neck IMRT. Radiat Oncol. 2016;11:79.
- Noble DJ, Yeap P-L, Seah SY, et al. Anatomical change during radiotherapy for head and neck cancer, and its effect on delivered dose to the spinal cord. Radiother Oncol. 2019;130:32–38.
- Samavati N, Velec M, Brock KK. Effect of deformable registration uncertainty on lung SBRT dose accumulation. Med Phys. 2016;43:233–240.
- Jung SH, Yoon SM, Park SH, et al. Four-dimensional dose evaluation using deformable image registration in radiotherapy for liver cancer. Med Phys. 2012;40:011706.
- Velec M, Moseley JL, Eccles CL, et al. Effect of breathing motion on radiotherapy dose accumulation in the abdomen using deformable registration. Int J Radiat Oncol Biol Phys. 2011;80:265–272.
- Senthi S, Griffioen GH, de Koste J, et al. Comparing rigid and deformable dose registration for high dose thoracic re-irradiation. Radiother Oncol. 2013;106:323–326.
- Heemsbergen WD, Al-Mamgani A, Witte MG, et al. Urinary obstruction in prostate cancer patients from the Dutch trial (68 Gy vs. 78 Gy): relationships with local dose, acute effects, and baseline characteristics. Int J Radiat Oncol Biol Phys. 2010;78:19–25.
- Acosta O, Drean G, Ospina JD, et al. Voxel-based population analysis for correlating local dose and rectal toxicity in prostate cancer radiotherapy. Phys Med Biol. 2013;58:2581.
- Drean G, Acosta O, Ospina JD, et al. Identification of a rectal subregion highly predictive of rectal bleeding in prostate cancer IMRT. Radiother Oncol. 2016;119:388–397.
- Drean G, Acosta O, Lafond C, et al. Interindividual registration and dose mapping for voxelwise population analysis of rectal toxicity in prostate cancer radiotherapy. Med Phys. 2016;43:2721–2730.
- Monti S, Palma G, D’avino V, et al. Voxel-based analysis unveils regional dose differences associated with radiation-induced morbidity in head and neck cancer patients. Sci Rep. 2017;7:7220.
- Avanzo M, Barbiero S, Trovo M, et al. Voxel-by-voxel correlation between radiologically radiation induced lung injury and dose after image-guided, intensity modulated radiotherapy for lung tumors. Phys Med. 2017;42:150–156.
- Palma G, Monti S, D'Avino V, et al. A voxel-based approach to explore local dose differences associated with radiation-induced lung damage. Int J Radiat Oncol Biol Phys. 2016;96:127–133.
- Jamema SV, Phurailatpam R, Paul SN, et al. Commissioning and validation of commercial deformable image registration software for adaptive contouring. Phys Med. 2018;47:1–8.
- Zhong H, Guttmann D, Huang M, et al. Evaluating the feasibility of applying deformable registration into adaptive therapy for NRG oncology RTOG 1106. Int J Radiat Oncol Biol Phys. 2017;99:E509–E510.
- Veiga C, Lourenço AM, Mouinuddin S, et al. Toward adaptive radiotherapy for head and neck patients: uncertainties in dose warping due to the choice of deformable registration algorithm. Med Phys. 2015;42:760–769.
- Fusella M, Giglioli FR, Fiandra C, et al. Evaluation of dose recalculation vs dose deformation in a commercial platform for deformable image registration with a computational phantom. Med Dosim. 2018;43:82–90.
- Abe Y, Kadoya N, Arai K, et al. Effect of DIR uncertainty on prostate passive-scattering proton therapy dose accumulation. Phys Med. 2017;39:113–120.
- Zhong H, Siddiqui SM, Movsas B, et al. Evaluation of adaptive treatment planning for patients with non-small cell lung cancer. Phys Med Biol. 2017;62:4346.
- Moriya S, Tachibana H, Kitamura N, et al. Dose warping performance in deformable image registration in lung. Phys Med. 2017;37:16–23.
- Zhang L, Wang Z, Shi C, et al. The impact of robustness of deformable image registration on contour propagation and dose accumulation for head and neck adaptive radiotherapy. J Appl Clin Med Phys. 2018;19:185–194.
- Weistrand O, Svensson S. The ANACONDA algorithm for deformable image registration in radiotherapy. Med Phys. 2015;42:40–53.
- Johnson PB, Padgett KR, Chen KL, et al. Evaluation of the tool “Reg Refine” for user-guided deformable image registration. J Appl Clin Med Phys. 2016;17:158–170.
- Velec M, Moseley JL, Svensson S, et al. Validation of biomechanical deformable image registration in the abdomen, thorax and pelvis in a commercial radiotherapy treatment planning system. Med Phys. 2017;44:3407–3417.
- Cazoulat G, Simon A, Dumenil A, et al. Surface-constrained nonrigid registration for dose monitoring in prostate cancer radiotherapy. IEEE Trans Med Imaging. 2014;33:1464–1474.
- Qi XS, Santhanam A, Neylon J, et al. Near real-time assessment of anatomic and dosimetric variations for head and neck radiation therapy via graphics processing unit-based dose deformation framework. Int J Radiat Oncol Biol Phys. 2015;92:415–422.
- Gu X, Dong B, Wang J, et al. A contour-guided deformable image registration algorithm for adaptive radiotherapy. Phys Med Biol. 2013;58:1889.
- Østergaard Noe K, De Senneville BD, Elstrøm UV, et al. Acceleration and validation of optical flow based deformable registration for image-guided radiotherapy. Acta Oncol. 2008;47:1286–1293.
- Brock KK, Dawson LA, Sharpe MB, et al. Feasibility of a novel deformable image registration technique to facilitate classification, targeting, and monitoring of tumor and normal tissue. Int J Radiat Oncol Biol Phys. 2006;64:1245–1254.
- Al-Mayah A, Moseley J, Hunter S, et al. Biomechanical-based image registration for head and neck radiation treatment. Phys Med Biol. 2010;55:6491.
- Polan DF, Feng M, Lawrence TS, et al. Implementing radiation dose-volume liver response in biomechanical deformable image registration. Int J Radiat Oncol Biol Phys. 2017;99:1004–1012.
- Qin A, Liang J, Han X, et al. The impact of deformable image registration methods on dose warping. Med Phys. 2018;45:1287–1294.
- Sharifi H, Zhang H, Bagher-Ebadian H, et al. Utilization of a hybrid finite-element based registration method to quantify heterogeneous tumor response for adaptive treatment for lung cancer patients. Phys Med Biol. 2018;63:065017.
- Castadot P, Lee JA, Parraga A, et al. Comparison of 12 deformable registration strategies in adaptive radiation therapy for the treatment of head and neck tumors. Radiother Oncol. 2008;89:1–12.
- Hardcastle N, Tomé WA, Cannon DM, et al. A multi-institution evaluation of deformable image registration algorithms for automatic organ delineation in adaptive head and neck radiotherapy. Radiat Oncol. 2012;7:90.
- Varadhan R, Karangelis G, Krishnan K, et al. A framework for deformable image registration validation in radiotherapy clinical applications. J Appl Clin Med Phys. 2013;14:192–213.
- Li X, Zhang Y, Shi Y, et al. Comprehensive evaluation of ten deformable image registration algorithms for contour propagation between CT and cone-beam CT images in adaptive head & neck radiotherapy. PLoS One. 2017;12:e0175906.
- Kashani R, Hub M, Balter JM, et al. Objective assessment of deformable image registration in radiotherapy: a multi-institution study. Med Phys. 2008;35:5944–5953.
- Kadoya N, Nakajima Y, Saito M, et al. Multi-institutional validation study of commercially available deformable image registration software for thoracic images. Int J Radiat Oncol Biol Phys. 2016;96:422–431.
- Yeo U, Supple J, Taylor M, et al. Performance of 12 DIR algorithms in low‐contrast regions for mass and density conserving deformation. Med Phys. 2013;40:101701.
- Mogadas N, Sothmann T, Knopp T, et al. Influence of deformable image registration on 4D dose simulation for extracranial SBRT: a multi-registration framework study. Radiother Oncol. 2018;127:225–232.
- Ribeiro CO, Knopf A, Langendijk JA, et al. Assessment of dosimetric errors induced by deformable image registration methods in 4D pencil beam scanned proton treatment planning for liver tumours. Radiother Oncol. 2018;128:174–181.
- Kierkels R, den Otter L, Korevaar E, et al. An automated, quantitative, and case-specific evaluation of deformable image registration in computed tomography images. Phys Med Biol. 2018;63:045026.
- Murphy K, Van Ginneken B, Reinhardt JM, et al. Evaluation of registration methods on thoracic CT: the EMPIRE10 challenge. IEEE Trans Med Imaging. 2011;30:1901–1920.
- Vandemeulebroucke J, Sarrut D, Clarysse P, editors. The POPI-model, a point-validated pixel-based breathing thorax model. XV International Conference on the Use of Computers in Radiation Therapy (ICCR); 2007.
- Castillo R, Castillo E, Fuentes D, et al. A reference dataset for deformable image registration spatial accuracy evaluation using the COPDgene study archive. Phys Med Biol. 2013;58:2861.
- Pukala J, Meeks SL, Staton RJ, et al. A virtual phantom library for the quantification of deformable image registration uncertainties in patients with cancers of the head and neck. Med Phys. 2013;40:111703.
- Brock KK, Consortium D. Results of a multi-institution deformable registration accuracy study (MIDRAS). Int J Radiat Oncol Biol Phys. 2010;76:583–596.
- Neylon J, Min Y, Low DA, et al. A neural network approach for fast, automated quantification of DIR performance. Med Phys. 2017;44:4126–4138.
- Paganelli C, Meschini G, Molinelli S, et al. Patient‐specific validation of deformable image registration in radiation therapy: overview and caveats. Med Phys. 2018;45:e908–e922.