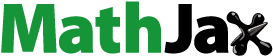
Abstract
Background: Selective avoidance aims at sparing functional lung regions. Here, we preferentially direct radiation to irreversibly nonfunctional lung areas based on planning CT imaging to reduce functional lung damage.
Materials and methods: For 12 stage I-IV NSCLC patients, 5 lung substructures were segmented on the planning CT, combining voxels <–900HU, –900HU to –801HU, –800HU to –701HU, –700HU to –601HU and ≥–600HU (Level 1 to 5). Two VMAT plans were optimized: a reference plan blinded from substructures and a selective avoidance plan (AV) imposing gradually stricter constraints on Level 1–5, based on previously validated associations between lung subvolume baseline density and density increase (ΔHU) after treatment. Characteristics of treatment plans were evaluated, including subvolumes, dose, and predicted ΔHU (with reported 95% CI reflecting prediction model uncertainty).
Results: Segmented substructures were on average 477 cc, 1157 cc, 484 cc, 69 cc, and 123 cc (Level 1–5). AV plans could spare Level 3–5, e.g., mean dose decrease of 3.5 Gy (range 0.6 Gy; 6.0 Gy) for Level 5, p<.001. This significantly reduced the average lung mass with predicted ΔHU>20HU by 12.5 g (95% CI: 5.4–16.9) and 27.1 g (95% CI: 10.2–32.9) for a median and upper 10th percentile patient susceptibility for damage simulation, respectively.
Conclusions: Lung damage avoidance based on CT density is feasible and easy to implement. A biomarker providing a reliable selection of patients with high susceptibility for lung damage will be crucial to show the clinical relevance of this avoidance planning strategy.
Introduction
Historically, the lungs have been considered one homogeneous organ in the radiotherapy treatment planning process. It has become common practice to apply constraints on the mean lung dose (MLD) and/or the relative lung volume receiving 20 Gy (V20) of the total lung volume, in order to limit the rate of severe radiation-induced toxicity, but with the assumption that the lungs are homogeneous and healthy [Citation1,Citation2]. However, both functional and structural differences between areas of the lung can be substantial [Citation3,Citation4]. It was therefore suggested to tailor the lung radiation dose distribution to regional lung characteristics, i.e., avoiding highly functional lung portions as much as possible, in order to reduce pulmonary toxicity. This is especially interesting for lung cancer patients with higher tumor stages where radiation-induced pulmonary toxicity risk is often dose-limiting [Citation2].
The rationale for selective avoidance based on functional imaging characteristics has been described by several authors [Citation5,Citation6]. They focused on sparing the most functional (in terms of ventilation or perfusion) lung regions, while preferentially depositing high dose to dysfunctional regions. Initially, Technetium-99m single positron emission computed tomography (SPECT) was investigated, which suffered from the typical spatial resolution limitations of SPECT imaging [Citation7]. Ventilation positron emission tomography (PET) scans could be obtained by gallium-68 aerosol (Galligas) inhalation and perfusion PET using gallium-68 macroaggregated albumin (MAA) [Citation8]. Recently, MR using hyperpolarized helium-3 was proposed [Citation9]. Finally, different 4DCT-based surrogates of lung ventilation have been validated [Citation10–12].
Planning studies using functional imaging-based avoidance maps for the lung have reported a dosimetric benefit for highly functional regions, which varied according to the functional imaging modality and functionality cutoff value used [Citation13–15]. A major limitation of functional imaging-based lung avoidance is that functional irreversibility is assumed [Citation6]. This assumption has been shown to often be invalid, e.g., due to reperfusion induced by tumor shrinkage or resolving of atelectasis [Citation16,Citation17].
An alternative approach could be to avoid lung portions with high susceptibility for radiation damage. This could be based on our previously published association between the density of lung parenchyma on the baseline planning CT scan and its local density increase in Hounsfield Units (ΔHU) 3 months after treatment, observed for stereotactically fractionated treatments [Citation18]. Lung ΔHU scored on follow-up CT has been used frequently as a surrogate of lung damage [Citation19,Citation20]. It is an objective and reproducible endpoint with a strong dose response [Citation18]. We showed that the regional variability of lung ΔHU for conventionally fractionated treatments as well was dependent on regional baseline CT density [Citation21]. Correlations between radiation-induced CT feature changes and clinical symptoms have only been shown in a small dataset of 20 patients [Citation22]. If such correlation could be confirmed in larger datasets, this could pave the way to a new concept of selective lung avoidance to minimize a clinically relevant risk of lung density increase. Importantly, the low density planning CT regions that will preferentially be irradiated (e.g., emphysematous portions in COPD patients) contain irreversibly damaged alveoli [Citation23]. According to our previous findings, a density increase by more than 20 HU is not to be expected in emphysematous areas, but only in healthy lung tissue. In current work, 20 HU was therefore chosen as a cutoff to represent severe radiation-induced lung density increase.
In this work, the feasibility of lung damage-limiting selective avoidance planning was investigated in a lung cancer dataset from two institutions. A gradually enhanced sparing was implemented for lung substructures with increasing planning CT HU. Simulations of the theoretical gain in terms of damage were reported as the predicted mass of lung tissue spared from a density increase of 20 HU for the avoidance plan compared to an anatomy-based reference plan.
Material and methods
Patient dataset
Twelve stage I-IV non-small–cell lung cancer (NSCLC) patients radically treated with intensity-modulated radiotherapy (IMRT) or volumetric modulated arc therapy (VMAT), equally distributed among upper and lower lobe tumor locations, were randomly picked from a dataset described in detail in previous work [Citation18,Citation22]. For six patients from the University Hospitals Leuven (institution 1) the planning CT was taken in free-breathing, while for six patients from MAASTRO Clinic (institution 2) this was a 50% expiration 4DCT frame. Margins of 5–10 mm from gross tumor volume (GTV) to clinical target volume (CTV) and 5–10 mm from CTV to planning target volume (PTV) were used. Planning CT scans were taken in supine position with the arms raised above the head. CT slice thickness was 3 mm and in-slice pixel size 0.977 to 1.27 mm.
Selective avoidance planning
For every patient, two new VMAT plans were optimized using Eclipse (Varian Medical Systems, Palo Alto, CA): an anatomy-based reference plan and a lung damage avoidance plan. An identical beam setup was used for both plans. The isocenter was located in the center of mass of the PTV and 2 VMAT arcs (6 or 10 MV) with respective collimator rotations of 30° and 330° were initialized. The prescription dose achieved in the clinically accepted plan was used (60 to 66 Gy in fractions of 2 Gy). For a fair comparison between reference and avoidance planning, a fixed set of objectives and weights was used for target and organ at risk (OAR) structures at the start of reference plan optimization, for all patients (Supplementary Appendix 1). During this reference plan optimization, weights were adapted in case OAR or PTV dose constraints were not met. For the avoidance planning, the final set of objectives and weights from the reference plan was used as the starting set, except for the lung. The reference lung objectives on the total lung volume structure were replaced by objectives on five separate ‘lungs minus GTV’ substructures combining lung voxels (the lung was first cropped by 5 mm in order to eliminate boundary effects) with similar CT density (eclipse CT ranger tool): <–900HU, –900HU to –801HU, –800HU to –701HU, –700HU to –601HU and ≥–600HU (‘Level 1’ to ‘Level 5’). Separate three-dimensional (3D) islands smaller than 1 cc were excluded from the structures. The definition of the five lung substructures is outlined in . The Level 1 low-density structure was unconstrained during optimization. Level 2 was assigned lung objectives of the reference plan, while gradually stricter objectives were placed on higher-density Level 3 to 5 (Supplementary Appendix 1). Weights were adapted in order to obtain PTV and OAR (including MLD on the total lung volume) doses reaching the level of the reference plan. Final dose calculation of all plans was performed with an Analytical Anisotropic Algorithm (AAA 10.0.28). A paired t-test evaluated the difference between lung substructure dose (Level 1–5) with both planning strategies.
Figure 1. Example patient (number 8) with on the left the five planning CT density-based substructure segmentations within the ‘lungs minus GTV’ structure (named ‘Level 1’ up to ‘Level 5’). The substructures contain lung voxels <–900 HU (Level 1), between –900 HU and –801 HU (Level 2), between –800 HU and –701 HU (Level 3), between –700 HU and –601 HU (Level 4) and ≥–600 HU (Level 5). In the middle, the anatomy-based VMAT plan naive with respect to CT density heterogeneity in the lung volume is presented. Images on the right present the lung avoidance VMAT plan based on the Level 1 to 5 lung substructures, and applying density-specific dose objectives. Note the drastic isodose shifts (indicated by black arrows on the right axial and coronal slices) to achieve optimal sparing of some high-density components in the lung damage avoidance plan.

Lung density increase (ΔHU) prediction
Sigmoids were previously reported to best fit the observed dose-density increase response curves for lung parenchyma at 3 months after treatment [Citation18]. The sigmoidal description of lung ΔHU was
(1)
(1)
with D the median equivalent dose in 2 Gy fractions (EQD2, α/β = 4 Gy) delivered to a portion of the analyzed lung volume, ΔHU the median absolute density increase of that portion at 3 months, ΔHUmax the saturation level of density increase in the analyzed lung volume, D50 the dose where 50% of ΔHUmax is reached and ɣ fixed at 1.3 [Citation24]. ΔHUmax was found to be dependent on the baseline HU (HU0) of the analyzed lung volume and thus to vary throughout the lung according to [Citation21]
(2)
(2)
This association was significant (p = .011). The uncertainty on the nominal slope value 0.152 could be described by its 95% CI (0.036–0.268). The nominal value of the slope was used in further damage calculations unless otherwise stated.
On the other hand, little variation of D50 was found within the lung [Citation21]. Moreover, as no prognostic factors of D50 were found, it was assumed to reflect the intrinsic lung tissue radiosensitivity of the patient. For the analysis of the lung ΔHU risk of both planning strategies, we further assumed the dose-ΔHU response of a voxel to be identical to that observed for subvolumes. For each plan, the expected ΔHU is calculated using EquationEquations (1)(1)
(1) and Equation(2)
(2)
(2) for every ‘lungs minus GTV’ voxel (with the dose map resampled to the CT resolution), and this for two radiosensitivity scenarios. The value of D50 was set for a median and a top-level susceptibility for lung damage at 28 Gy and 15 Gy (50th and 10th percentile of the distribution between patients [Citation18]), respectively. For every patient, we thus obtain two 3 D maps of expected ΔHU for both planning strategies. The number of lung voxels and the mass (grams of lung) with >20 HU increase as a threshold for severe lung damage were reported. According to EquationEquation (2)
(2)
(2) , ΔHUmax is expected to be 20 HU for HU0 = –935 HU (close to the –950 HU threshold commonly used to radiologically quantify the relative lung area with COPD). Image analysis was performed in MeVisLab 2.6.2 (MeVis Fraunhofer, Bremen, Germany) and Matlab R2015b (The Mathworks Inc., Natick, MA, USA), and statistics in Statistica 13 (Dell Inc., Tulsa, OK, USA).
Results
Patient and treatment characteristics are listed in . Lung substructures used in the selective avoidance planning had mean volumes of (range between brackets) 477 cc (0–2869 cc), 1157 cc (67–2714 cc), 484 cc (32–852 cc), 69 cc (1–307 cc), and 123 cc (52–208 cc) for Level 1 to 5, respectively. The required PTV and OAR dose constraints could be reached in all avoidance plans. shows an example of the substructure segmentations and associated avoidance plan sparing high-density areas close to PTV. The total lung MLD was similar for both planning strategies (average 15.8 Gy and 15.2 Gy for reference and avoidance plans, respectively). Mean heart dose slightly increased in avoidance planning from 13.0 Gy to 13.3 Gy on average (Supplementary Appendix 2). Planned dose of the different lung substructures are summarized in . A significant improvement with avoidance planning was observed for substructures above –800 HU (Level 3 to 5), e.g., average MLD from 16.4 Gy to 12.9 Gy (p < .001) and V20 from 30.6% to 20.3% (p < .001) for Level 5. The impact of avoidance planning on the low dose volume (V5) was limited: average 64.2% to 61.0% (p = .014) for Level 5.
Figure 2. Planned dose for the different lung substructures (Level 1 to 5), containing lung voxels within separate CT density bins as defined in . Mean and standard error of mean dose in the population, weighted by the individual substructure volumes, for the dose metrics mean dose (left graph), volume receiving 20 Gy (V20, middle graph) and volume receiving 5 Gy (V5, right graph). The significance (paired t-test) of the difference between reference and avoidance plan dose was indicated above the data points using asterisks. Highly significant reductions could be obtained with selective avoidance planning for Level 4 and 5 mean dose and V20. V5 of the lung substructures was less influenced by the planning strategy. Planned dose of all individual patients can be found in Supplementary Appendix 2. ***p < .001; **p < .01; * p < .05; NS, not significant (paired t-test).

Table 1. Patient and treatment characteristics of the 12 patients in the dataset (absolute number of patients or median value with range).
The model-based prediction of lung ΔHU showed a growing part of lung with severe damage, from the median to the top-level damage susceptibility scenario (. Only in the top-level damage susceptibility scenario, avoidance planning was seen to substantially reduce the number of damaged ≥–700 HU voxels. The reduction of damaged lung mass by avoidance planning (compared to reference planning) was significant (p = .001): on average (between brackets are results for the 95% CI values of the ΔHUmax prediction model slope) 12.5 (5.4; 16.9) and 27.1 (10.2; 32.9) gram reduction for increasing damage susceptibility (50th and 10th percentile susceptibility, respectively). Assuming a median susceptibility (50th percentile scenario), avoidance planning could not reduce the damaged lung mass in 3 out of 12 patients (one upper lobe and two lower lobe tumors). In the 10th percentile susceptibility scenario, 11 out of 12 patients benefited from avoidance planning, with up to 67 g of lung spared from severe damage in an individual patient (. In relative terms, this accounted for 8.0% of the total lung mass of this patient.
Figure 3. Predicted lung damage for both the reference (REF) and selective avoidance (AV) planning strategies. (a) Percentage of lung voxels predicted with 20 HU density increase or more (arbitrary threshold for severe lung damage), per planning CT voxel density and for 2 damage susceptibility scenarios: a median (50th percentile) and a top-level (10th percentile) susceptibility for lung damage. Note that for voxels below –935 HU, a post-treatment density increase of 20 HU cannot be reached according to our model and predictions are therefore 0%. (b) Predicted lung mass damaged (expressed in gram lung tissue) in a top-level susceptibility (using a 10th percentile value of D50) scenario, for all individual patient plans in the dataset.

Discussion
Conformal avoidance of selected normal lung regions may reduce pulmonary toxicity. Several avoidance planning studies sparing well ventilated and/or perfused lung regions have been conducted, and recently the first patient treatment was delivered [Citation25]. In this work, we present the first simulation study of an alternative approach selectively avoiding lung tissue at risk of post-treatment density increase (as a surrogate of damage). The prognostic model of lung damage used is based on the previously described association between planning CT density of lung volumes and their median absolute increase in density 3 months after treatment [Citation18,Citation21]. VMAT plans sparing lung voxels according to their planning CT density were optimized for a dataset of 12 NSCLC patients. The required lung substructures could be generated using simple HU thresholding and post-processing tools available in commercial treatment planning systems, making our planning strategy easily applicable in clinical practice. We compared our experimental plans to reference plans optimized using the classical MLD constraint.
The lung damage avoidance plans presented a significant dose reduction (mean dose and V20) for substructures with a density ≥–800 HU. This resulted in a lower number of voxels with a relevant density increase of 20 HU. Two damage susceptibility scenarios were simulated by varying D50 (position of the lung tissue dose–ΔHU response curve) in the damage prediction equations. Assuming a median damage susceptibility for every patient, avoidance planning could spare on average 12.5 gram of lung mass from predicted damage (using 95% CI of predictive model coefficients gives 5.4 and 16.9 g). For the lowest 10th percentile D50 (top-level susceptibility), an advantage of avoidance planning could be observed for 11 out of 12 patients in the dataset. A maximum 8% fraction of the total lung mass could be spared from severe damage in an individual patient in that top-level susceptibility scenario. Clinical application of our lung damage avoidance concept might thus be preferable in individuals with higher lung tissue radiosensitivity. An accurate biomarker of radiosensitivity would thus be crucial to be able to successfully translate current avoidance planning to a clinical setting. Moreover, the correlation between lung damage post RT and radiation pneumonitis scores remains to be established. Despite its limited direct implications in terms of clinical outcome measures and quality of life, our study provides a proof of concept of avoidance planning to be tested when a biomarker of radiosensitivity is found.
Avoidance planning could still be improved by an extensive analysis of the ideal number of lung substructures, their patient-individual HU cutoffs and corresponding dosimetric optimization objectives. The success of lung damage avoidance planning was dependent on the respective locations of PTV, high- and low-density lung substructures and other OAR. Bullae (nonfunctional fibrotic air pockets), which could serve the avoidance planning, are most frequently located in the upper lobe. These low-density, often emphysematous, regions are already irreversibly damaged and cannot recover [Citation23], while higher density portions are functionally important [Citation26]. A larger (non-significant) damage reduction was also seen for upper lobe tumor locations in our dataset.
Several studies have described ventilation and perfusion imaging-based regional lung avoidance. Siva et al. have reported that only perfusion-based avoidance planning resulted in significantly lower dose to functional volumes compared to a reference anatomy-based plan [Citation15]. Yamamoto et al. have reported a 2 Gy mean dose reduction to highly ventilated lung with VMAT, which is in the same order of magnitude as our 3.5 Gy reduction for Level 5 [13]. Moreover, Farr et al. have shown the dose volume histogram parameters of highly perfused lung to only slightly improve the correlation with radiation pneumonitis [Citation27]. The planning CT HU-driven reduction of lung density increase (as a surrogate of lung damage) to a relevant lung volume might thus be an interesting new approach. Also, no additional imaging or challenging image registration as in PET/CT and certainly SPECT are needed in our approach. Kimura et al. have obtained a 2% V20 reduction for a CT-based (using one threshold at –860 HU) functional avoidance volume in a COPD population, where we report an average 10% reduction for Level 5 [Citation28]. Mihaylov introduced an energy-minimizing planning concept by using the product of voxel dose, density and volume in inverse optimization instead of the common DVH-based optimization [Citation29]. The resulting plans for lung cancer showed an avoidance of high-density lung tissue similarly to our plans.
This study had limitations. First, it was assumed that the ΔHU prediction model (and its parameters D50 and ΔHUmax) previously derived from lung subvolume data, was applicable to single lung voxels. The similar dose–ΔHU response reported for single voxels, be it with a large standard deviation, underpins the validity of this approach [Citation30]. Also, the highest-density lung voxels of the Level 5 subvolume (voxels above –600 HU on the planning CT), maximally spared during optimization, are outside of the range of our previous HU-ΔHU observations [Citation21].While an extrapolation of the ΔHU prediction model was thus assumed, there is evidence that the airways and vessels included in the Level 5 subvolume are indeed prone to the highest damage. We previously showed in patients treated in the PET-boost dose escalation study that the density increase medially in the lung was significantly higher than in peripheral locations [Citation31]. For ΔHUmax, only a limited variation (R2 = .09) was attributable to baseline lung HU. Other prognostic factors of ΔHU and an individual radiosensitivity assessment could therefore be added to the prognostic model in future studies. Also, our model only predicts local dose-density increase. A possible altered volume effect (following the redistribution of doses within the lung) impacting lung damage cannot be ruled out. However, the low dose difference between planning strategies (V5 from 64% to 61% on average for Level 5) was very small. For D50, it will be crucial to find a reproducible biomarker of lung tissue radiosensitivity [Citation32] (e.g., through the REQUITE observational study [Citation33]) allowing optimal (top-level lung radiosensitivity) patient selection for our selective avoidance planning. It should be noted that assuming another HU increase threshold for severe damage than the current 20 HU, would alter the absolute values of lung mass spared from severe damage (). The presented results in terms of lung mass are thus more of qualitative than quantitative importance. While the impact of density changes on clinical outcomes and quality of life was not yet reliably shown in large datasets, many other risk factors of RP have been reported, e.g., MLD, lower lobe location [Citation34], age and preexisting pulmonary comorbidity [Citation35]. We have previously shown that the lower lobe location is associated with the presence of high-density lung tissue [Citation21]. This risk factor could thus be a reflection of the impact of density change. Furthermore, higher age and preexisting pulmonary comorbidity might have been perceived as risk factors due to their intrinsic association with higher grades of dyspnea and cough already present before the treatment. In order to obtain risk factors related to radiotherapy-induced toxicity outcomes, it was suggested to incorporate baseline symptoms into normal tissue complication probability (NTCP) modeling [Citation36]. Based on these points, we are convinced that lung damage is a strong driver of radiotherapy-induced pulmonary toxicity and that it is thus important to limit the damaged lung volumes. Future NTCP models should thus include the regional dose-dependent risk of lung damage (taking into account an update of the 20 HU increase damage threshold) as a covariate in order to accurately predict the impact of our avoidance planning strategy for relevant clinical endpoints.
Secondly, different planning CT acquisition protocols were used in both institutions. The smaller lung volumes in free-breathing scans of institution 1 (on average 2905 cc versus 5064 cc in 50% expiration in institution 2) did not significantly impede the avoidance planning results: the top-level susceptibility damage reduction was 35.8 g in institution 1 and 22.0 g in institution 2 on average (p = .88, Mann-Whitney U test). An accurate delivery of avoidance dose maps requires an advanced motion management technique (e.g., treatment in deep inspiration breath-hold (DIBH)). As our avoidance strategy shows a significantly lowered damage in both institutions (p = .05 and p = .02 for institution 1 and 2, respectively), it should be feasible for any CT acquisition.
The selective lung damage avoidance approach is based on an objective CT-derived endpoint that could be compared to its prediction in order to continuously refine the model. As the correlation between CT-scored lung damage at 3 months and pulmonary toxicity remains uncertain, a randomized trial should validate the clinical relevance of our concept avoiding lung regions most susceptible for radiation damage. It should be noted that our findings have indicated that a reliable selection of patients with the highest susceptibility for lung damage (e.g., by a reproducible biomarker selecting the 10th percentile lung damage susceptibility patients) is a crucial prerequisite of such randomized trial. This to avoid unnecessary avoidance planning in patients not being at risk of lung damage (in previous work approximately 20% of patients did not show lung density increase at any dose level [Citation21]). A small but significant reduction in total lung MLD was observed in our avoidance plans compared to the reference plans, which would result in 0.9% reduction of severe RP risk, according to QUANTEC [Citation1]. In order to attribute any toxicity outcome difference to the damage avoidance strategy, it would thus be advisable to renormalize avoidance plans to the same MLD as the reference plans. Heart doses could be limited approximately to the original plan’s level. This should be monitored closely as heart dose was shown to be associated to lung density increase as well as to radiation pneumonitis [Citation21,Citation37]. In the absence of reproducible biomarkers of lung radiosensitivity, prospective studies using classical anatomy-based planning and assessing delivered doses to dense lung substructures (and bullae) and the associated outcome in terms of radiation pneumonitis grades, could indirectly provide some indication of the clinical potential of the proposed avoidance planning strategy.
Supplemental Material
Download MS Word (19.3 KB)Disclosure statement
No potential conflict of interest was reported by the authors.
Additional information
Funding
References
- Marks LB, Bentzen SM, Deasy JO. Radiation dose-volume effects in the lung. Int J Radiat Oncol Biol Phys. 2010;76(3 Suppl):S70–S76.
- De Ruysscher D, Faivre-Finn C, Moeller D, et al. European Organization for Research and Training of Cancer (EORTC) recommendations for planning and delivery of high-dose, high precision radiotherapy for lung cancer. Radiother Oncol. 2017;124(1):1–10.
- Hoffman EA, McLennan G. Assessment of the pulmonary structure-function relationship and clinical outcomes measures: quantitative volumetric CT of the lung. Acad Radiol. 1997;4(11):758–776.
- Seppenwoolde Y, Engelsman M, De Jaeger K, et al. Optimizing radiation treatment plans for lung cancer using lung perfusion information. Radiother Oncol. 2002;63(2):165–177.
- Ireland R, Tahir B, Wild J, et al. Functional image-guided radiotherapy planning for normal lung avoidance. Clin Oncol (R Coll Radiol). 2016;28(11):695–707.
- Lambin P, Petit SF, Aerts H, et al. The ESTRO Breur Lecture 2009. From population to voxel-based radiotherapy: exploiting intra-tumour and intra-organ heterogeneity for advanced treatment of non-small cell lung cancer. Radiother Oncol. 2010;96(2):145–152.
- Marks L, Spencer D, Bentel G, et al. The utility of SPECT lung perfusion scans in minimizing and assessing the physiologic consequences of thoracic irradiation. Int J Radiat Oncol Biol Phys. 1993;26(4):659–668.
- Siva S, Devereux T, Ball D, et al. Ga-68 MAA perfusion 4DPET/CT scanning allows for functional lung avoidance using conformal radiation therapy planning. Technol Cancer Res Treat. 2016;15(1):114–121.
- van Beek E, Wild J, Kauczor H, et al. Functional MRI of the lung using hyperpolarized 3-helium gas. J Magn Reson Imaging 2004;20(4):540.
- Reinhardt J, Ding K, Cao K, et al. Registration-based estimates of local lung tissue expansion compared to xenon CT measures of specific ventilation. Med Image Anal. 2008;12(6):752–763.
- Yamamoto T, Kabus S, Lorenz C, et al. Pulmonary ventilation imaging based on 4-dimensional computed tomography: comparison with pulmonary function tests and SPECT ventilation images. Int J Radiat Oncol Biol Phys. 2014;90(2):414–422.
- Castillo R, Castillo E, Martinez J, et al. Ventilation from four-dimensional computed tomography: density versus Jacobian methods. Phys Med Biol. 2010;55(16):4661–4685.
- Yamamoto T, Kabus S, von Berg J, et al. Impact of four-dimensional computed tomography pulmonary ventilation imaging-based functional avoidance for lung cancer radiotherapy. Int J Radiat Oncol Biol Phys. 2011;79(1):279–288.
- St-Hilaire J, Lavoie C, Dagnault A, et al. Functional avoidance of lung in plan optimization with an aperture-based inverse planning system. Radiother Oncol. 2011;100(3):390–395.
- Siva S, Thomas R, Callahan J, et al. High-resolution pulmonary ventilation and perfusion PET/CT allows for functionally adapted intensity-modulated radiotherapy in lung cancer. Radiother Oncol. 2015;115(2):157–162.
- Seppenwoolde Y, Muller SH, Theuws JC, et al. Radiation dose-effect relations and local recovery in perfusion for patients with non-small-cell lung cancer. Int J Radiat Oncol Biol Phys. 2000;47(3):681–690.
- Siva S, Hardcastle N, Kron T, et al. Ventilation/perfusion positron emission tomography-based assessment of radiation injury to lung. Int J Radiat Oncol Biol Phys. 2015;93(2):408–417.
- Defraene G, van Elmpt W, Crijns W, et al. CT characteristics allow identification of patient-specific susceptibility for radiation-induced lung damage. Radiother Oncol. 2015;117(1):29–35.
- Palma DA, van Sörnsen de Koste J, Verbakel W, et al. Lung density changes after stereotactic radiotherapy: a quantitative analysis in 50 patients. Int J Radiat Oncol Biol Phys. 2011;81(4):974–978.
- Diot Q, Kavanagh B, Schefter T, et al. Regional normal lung tissue density changes in patients treated with stereotactic body radiation therapy for lung tumors. Int J Radiat Oncol Biol Phys. 2012;84(4):1024–1030.
- Defraene G, van Elmpt W, Crijns W, et al. Regional variability in radiation-induced lung damage can be predicted by baseline CT numbers. Radiother Oncol. 2017;122(2):300–306.
- Ghobadi G, Wiegman EM, Langendijk JA, et al. A new CT-based method to quantify radiation-induced lung damage in patients. Radiother Oncol. 2015;117(1):4–8.
- Bellomi M, Rampinelli C, Veronesi G, et al. Evolution of emphysema in relation to smoking. Eur Radiol. 2010;20(2):286–292.
- Bentzen SM, Saunders MI, Dische S. From CHART to CHARTWEL in non-small cell lung cancer: clinical radiobiological modeling of the expected change in outcome. Clin Oncol. 2002;14(5):372–381.
- Yamamoto T, Kabus S, Bal M, et al. The first patient treatment of computed tomography functional image-guided radiotherapy for lung cancer. Radiother Oncol. 2016;118(2):227–231.
- Mets OM, Buckens CF, Zanen P, et al. Identification of chronic obstructive pulmonary disease in lung cancer screening computed tomography scans. JAMA. 2011;306(16):1775–1781.
- Farr KP, Kallehauge JF, Moller DS, et al. Inclusion of functional information from perfusion SPECT improves predictive value of dose-volume parameters in lung toxicity outcome after radiotherapy for non-small lung cancer: a prospective study. Radiother Oncol. 2015;117(1):9–16.
- Kimura T, Nishibuchi I, Murakami Y, et al. Functional image-guided radiotherapy planning in respiratory-gated intensity-modulated radiotherapy for lung cancer patients with chronic obstructive pulmonary disease. Int J Radiat Oncol Biol Phys. 2012;82:e633–e670.
- Mihaylov I. New approach in lung cancer radiotherapy offers better normal tissue sparing. Radiother Oncol. 2016;121(2):316–321.
- Bertelsen A, Schytte T, Bentzen SM, et al. Radiation dose response of normal lung assessed by Cone Beam CT – a potential tool for biologically adaptive radiation therapy. Radiother Oncol. 2011;100(3):351–355.
- Defraene G, La Fontaine M, van Kranen S, et al. Radiation-induced lung density changes on CT scan for NSCLC: no impact of dose-escalation level or volume. Int J Radiat Oncol Biol Phys. 2018;102(3):642–650.
- De Ruysscher D, Sharifi H, Defraene G, et al. Quantification of radiation-induced lung damage with CT scans: the possible benefit for radiogenomics. Acta Oncol. 2013;52(7):1405–1410.
- De Ruysscher D, Defraene G, Ramaekers BLT, et al. Optimal design and patient selection for interventional trials using radiogenomic biomarkers: a REQUITE and Radiogenomics consortium statement. Radiother Oncol. 2016;121(3):440–446.
- Bradley J, Hope A, El Naqa I, et al. A nomogram to predict radiation pneumonitis, derived from a combined analysis of RTOG 9311 and institutional data. Int J Radiat Oncol Biol Phys. 2007;69 (4):985–992.
- Vogelius IR, Bentzen SM. A literature-based meta-analysis of clinical risk factors for development of radiation induced pneumonitis. Acta Oncol. 2012;51(8):975–983.
- De Ruysscher D, Dehing C, Yu S, et al. Dyspnea evolution after high-dose radiotherapy in patients with non-small cell lung cancer. Radiother Oncol. 2009;91(3):353–359.
- Huang EX, Hope AJ, Lindsay PE, et al. Heart irradiation as a risk factor for radiation pneumonitis. Acta Oncol. 2011;50(1):51–60.