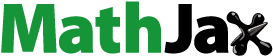
Abstract
Purpose: To examine the feasibility of automatic data extraction from clinical radiation therapy (RT) databases at four hospitals to investigate the impact of mean lung dose (MLD) and age on the risk of early respiratory-related death and early overall death for patients treated with RT for non-small-cell lung cancer (NSCLC).
Material and methods: We included adult patients with NSCLC receiving curatively intended RT between 2002 and 2017 at four hospitals. A script was developed to automatically extract RT-related data. The cause of death for patients deceased within 180 days of the start of RT was retrospectively assessed. Using logistic regression, the risks of respiratory-related death and of overall death within 90 and 180 days were investigated using MLD and age as variables.
Results: Altogether, 1785 patients were included in the analysis of early overall mortality and 1655 of early respiratory-related mortality. The respiratory-related mortalities within 90 and 180 days were 0.9% (15/1655) and 3.6% (60/1655). The overall mortalities within 90 and 180 days were 2.5% (45/1785) and 10.6% (190/1785). Higher MLD and older age were associated with an increased risk of respiratory-related death within 180 days and overall death within 90 and 180 days (all p<.05). For example, the risk of respiratory-related death within 180 days and their 95% confidence interval for patients aged 65 and 75 years with MLDs of 20 Gy was according to our logistic model 3.8% (2.6–5.0%) and 7.7% (5.5–10%), respectively.
Conclusions: Automatic data extraction was successfully used to pool data from four hospitals. MLD and age were associated with the risk of respiratory-related death within 180 days of the start of RT and with overall death within 90 and 180 days. A model quantifying the risk of respiratory-related death within 180 days was formulated.
Introduction
Fatal treatment-induced complications are rare in modern radiation therapy (RT). Yet, incidences of fatal complications are still reported [Citation1,Citation2] and we propose that such mortality may at least in part be mitigated by increased knowledge of individualised tolerance doses to organs at risk. However, estimation of dose–response relationships for rarely occurring toxicities is challenging since both clinical (patient-, disease- and treatment-related) risk factors and follow-up data must be collected for a large patient cohort. For example, assuming 4% incidence and a rule of thumb of at least 20 events per investigated variable, a sample size above 1500 patients would be required to estimate the impact of more than three clinical factors. This sample size is much larger than typical institutional series, cf., QUANTEC [Citation3], and to manually collect such large amount of data would be labour-intensive and time-consuming. Here, we investigate an automatic method of multi-institutional data collection to alleviate these problems.
The median overall survival is about 24 months for patients with locally advanced non-small-cell lung cancer (NSCLC) following the present standard treatment consisting of concurrent chemoradiotherapy with prescribed absorbed doses of 60–70 Gy in 2 Gy fractions [Citation2,Citation4]. Radiation pneumonitis (RP) is a common complication following this treatment that not only depends on the absorbed dose to healthy lung tissue, but also has been reported to depend on other clinical factors such as age, tumour location and chemotherapy regimen [Citation5,Citation6]. The dose–response relationship for developing lower grades of RP is well studied and numerous quantitative models have been presented [Citation5]. The risk of early overall death following RT of lung cancer has also been investigated [Citation2,Citation7,Citation8]. A multivariable model has been reported [Citation2] and individualised prescription strategies have been suggested to improve outcome [Citation9,Citation10]. Early respiratory- and treatment-related mortality, including fatal (grade 5) RP that has been reported for the standard treatment regimen [Citation11–15], has to our knowledge not been quantified in a risk model.
The aim of this study was to examine the feasibility of automatic data extraction from existing clinical databases to investigate the impact of mean lung dose (MLD) and age on early mortality among consecutively treated patients with NSCLC at four Scandinavian hospitals. We introduced the study-specific endpoint ‘respiratory-related death’ and studied the respiratory-related death within 180 days of the start of RT as the primary endpoint, but respiratory-related death within 90 days and the more common endpoints overall death within 90 and 180 days were studied as well.
Material and methods
Patient inclusion
We included adult patients diagnosed with NSCLC receiving standard fractionated (1.7 or 2 Gy fractions) curatively intended RT at the four hospitals, with a delivered total dose of at least 56 Gy. Treatment years included were 2002–2016 at Sahlgrenska University Hospital (Gothenburg, Sweden), 2012–2016 at Skåne University Hospital (Lund, Sweden), 2008–2016 at Herlev and Gentofte Hospital (Herlev, Denmark) and 2010–2017 at Rigshospitalet (Copenhagen, Denmark). The included patients were diagnosed with stages from IA to IV (IASLC staging system, 7th edition [Citation16]), but most of the patients were diagnosed with locally advanced disease, i.e., stage IIIA or IIIB, due to involved regional lymph nodes.
Treatment procedure
The patients were immobilised with a vacuum pillow or prefabricated breast board, preferably with the arms above their head, and a computed tomography (CT) scan was performed in treatment position. From 2009 to 2010 onwards, a positron emission tomography (PET) scan was acquired for most patients. 4DCT was implemented at the four hospitals between 2009 and 2012. The gross tumour volume (GTV) was delineated from the CT image series and guided by the metabolic tumour volume if a PET scan was performed. A clinical target volume (CTV) was created by adding approximately 5–10 mm margin to the GTV and adjusted to avoid, e.g., bones or large vessels. The planning target volume (PTV) was created by adding a 4–15 mm margin to the CTV. The margin included adjustment to tumour motion according to the 4DCT image series when available. The total lung tissue, i.e., both lungs excluding the GTV, was automatically defined using CT numbers according to local clinical protocols.
Three-dimensional conformal radiotherapy (3DCRT), intensity-modulated radiotherapy (IMRT) or volumetric modulated arc therapy (VMAT) treatment technique was used with a photon energy of 6 MV. Treatment planning was done in the Eclipse (Varian Medical Systems, Palo Alto, CA, USA) or Oncentra Masterplan (Elekta AB, Stockholm, Sweden) treatment planning system (TPS), and the analytical anisotropic algorithm (AAA), Acuros XB, or the pencil beam/pencil beam enhanced dose calculation algorithm was used. Prescription doses were 56–84 Gy in 2 Gy fractions or 61.2–64.6 Gy in 1.7 Gy fractions, and the planning aim was to cover the PTV with 95% of the prescribed dose. Treatments were delivered with five or six fractions of 2 Gy per week or with 10 fractions of 1.7 Gy per week (twice daily).
Most patients were given concurrent chemoradiotherapy with a platinum doublet. The pharmaceutical agents and schedules have varied somewhat during the period, but the majority were treated with cisplatin or carboplatin combined with a taxane (docetaxel or paclitaxel) or vinorelbine, usually for three courses where the RT started concurrently with the second cycle.
Outcome measures and cause of death assessment
Dates of death were manually collected from national registries or hospital medical records for all identified patients. Our aim for the respiratory-related endpoints was to study respiratory-related death caused by RT. For all patients deceased within 180 days of the start of RT, the cause of death was retrospectively assessed by one oncologist at each hospital. Effort was put into minimising the uncertainty in the assessment by performing a study-dedicated evaluation based on all available documents such as medical records, radiology reports and post-mortem reports. The evaluation was based on detailed written instructions with examples to minimise inter-oncologist interpretation differences. All causes of death were assigned to one of the following categories:
Disease-related cause of death (lung cancer).
Respiratory-related causes of death.
Other causes of death.
Unknown cause of death.
Respiratory-related causes of death include, besides RP, also other respiratory-related causes of death such as pneumonia, pulmonary embolism or chronic obstructive pulmonary disease (COPD).
The primary endpoint was defined as respiratory-related death (category B) within 180 days of the start of RT. Three secondary endpoints were defined as respiratory-related death (category B) within 90 days of the start of RT, overall death (all categories) within 180 days of the start of RT, and overall death (all categories) within 90 days of the start of RT.
Automatic data extraction
Scripting is a programming-based method for automatic execution of tasks, such as reading from a database and writing information to a file. In this study, the programming language C# was used to write a script that accesses the local databases belonging to the oncology information system (OIS). All four hospitals used the same OIS (ARIA, Version 13.6 or 15.5; Varian Medical Systems, Palo Alto, CA, USA). The script was written in Visual Studio (Version 14.0; Microsoft, Redmond, WA, USA), from where both the Eclipse scripting application programming interface (ESAPI) and structured query language (SQL) could be combined to find and access data in the database. Using these two methods, information which is usually viewed in different modules of ARIA, e.g., information on calculated dose distributions and information on delivered fractions at the treatment machine, could be accessed with the same script. The aim of our script was to automatically extract data for all patients without manually having to open each patient in ARIA.
All patients meeting the inclusion criteria were automatically identified by the script. Age at the start of RT (henceforth referred to as age), fraction dose, number of delivered fractions, treatment length and history of previous RTs were automatically extracted for each identified patient by the script. In this study, we define the MLD as the mean dose to the anatomical region including both lungs in combination but excluding the GTV. For each patient, the delivered MLD was calculated by multiplying the planned MLD per fraction with the number of delivered fractions before automatic extraction. The same script could be used at all hospitals by making small adjustments to different hospital-specific nomenclatures.
For patients treated multiple times with RT for NSCLC, only the first course of treatment was used for the analysis in our study. Patients previously treated with RT to an unspecified anatomical region were manually reviewed in Eclipse to determine if that treatment delivered dose to the thoracic region. For 11 patients with treatment plans created in Oncentra MasterPlan, the dose distribution did not exist in ARIA wherefore the MLDs were manually extracted from Oncentra MasterPlan. Variations between hospitals regarding the use of different dose calculation algorithms [Citation17] and/or different protocols for image guidance were not accounted for.
Errors in the automatically extracted data were minimised by performing both quality controls (QCs) included in the script as well as QCs on the extracted data. The script was iteratively developed as we gained insight on how OIS information had been entered with regards to, for instance, nomenclature, and how clinical practices had changed over time. One example is that automatic QCs were included in the script to verify that manual correction for attenuation in the treatment couch, which was done within a short time period at Sahlgrenska University Hospital, had been correctly applied. At each hospital and for each extracted parameter, we manually confirmed agreement between the extracted value and the value as seen in ARIA for randomly selected patients. We also manually reviewed the patients with the 10 lowest and 10 highest calculated MLDs at each hospital. QCs of the lung delineations included visual examination in Eclipse of all patients identified by the script as having only one delineated lung. This was done to assure that these patients only had one lung with low CT numbers due to for instance pneumonectomy, pneumothorax or atelectasis and not two functioning lungs but only one delineated.
Patient exclusion
Exclusion criteria were previous RT to the thoracic region, treatment longer than 70 days, and missing data. Patients found ineligible in any of the QCs were also excluded. When analysing the risk of respiratory-related death, we excluded patients who died from other causes than respiratory-related (categories A, C and D).
Statistical analysis
Logistic regression was performed using the function fitglm in MATLAB (Version R2017b, MathWorks, Natick, MA, USA). Using this function, the impact of each of the k investigated variables on the risk is assessed by its estimated regression coefficient βj, j=1,2,…,k. fitglm estimates the regression coefficients using maximum likelihood estimation that maximises the log-likelihood function LL calculated according to:
where β0 is the intercept coefficient and the risk for the ith patient is given by:
Here, Xi=xi,1, xi,2,…,xi,k, is the ith row of X, containing the known variable values for the ith patient. Patients i=1,…,M had an endpoint equal to 1 (deceased patients) and patients i=M+1,…,N had an endpoint equal to 0. The odds ratio (OR) of each variable equals eβj.
The analysis was performed according to a predefined statistical analysis plan. For each endpoint with at least 20 events, the OR and the corresponding 95% confidence interval (CI) for the continuous variables MLD and age at the start of RT were estimated by performing uni- and bivariable logistic regression (k=1 and k=2). For reporting purposes only, the Akaike information criterion (AIC) was calculated for each logistic regression according to:
No variable selection was done. Using the likelihood ratio test, we considered a variable with a p-value less than .05 to be statistically significant. For the primary endpoint respiratory-related death within 180 days of the start of RT, the bivariable model with MLD and age as variables was further investigated. The 95% CI of the model was calculated using random sampling with replacement (bootstrapping) with 10,000 bootstrapped data sets. The 2.5th and 97.5th percentiles of the bootstrapped models were used as the limits of the 95% CI of the model. The model was considered statistically significant if the p-value of the model was less than .05 in the likelihood ratio test. The discriminatory power of the model was studied using the area under the receiver operating characteristics (ROC) curve.
Results
Automatic data extraction was successfully used to extract data belonging to the 1851 patients that fulfilled the inclusion criteria. Out of these, 50 patients were removed according to the exclusion criteria, 16 patients removed for reasons detected in one of the QCs, and another 130 patients excluded when studying early respiratory-related mortality (). This resulted in 1785 patients eligible for analysis of early overall mortality, and 1655 patients for analysis of early respiratory-related mortality. Treatment characteristics of the patients included in the analysis are presented in . The respiratory-related mortality within 90 and 180 days of the start of RT was 0.9% (15/1655) and 3.6% (60/1655), respectively. The corresponding numbers for the overall mortality within 90 and 180 days were 2.5% (45/1785) and 10.6% (190/1785). The distribution of estimated causes of death is visualised in for deaths occurring within 90 and 180 days of the start of RT.
Figure 2. Distribution of estimated causes of death for patients deceased within 90 and 180 days of the radiation therapy start.

Table 1. Treatment characteristics of the patients included in the analysis.
The results of the uni- and bivariable analyses of MLD and age are presented in . Respiratory-related death within 90 days was not analysed as the number of events was below 20. Higher MLD and older age were associated with an increased risk of overall death within 90 and 180 days of the start of RT, and respiratory-related death within 180 days of the start of RT.
Table 2. Odds ratios, confidence intervals, p-values and the Akaike information criteria of the mean lung dose and age based on uni- and bivariable logistic regressions.
A statistically significant logistic model (p<.001) quantifying the risk of respiratory-related death within 180 days of the start of RT as a function of MLD and age was formulated as:
where β0= −10.613, βMLD=0.129 and βAge=0.074.
As an example of the risks of respiratory-related death within 180 days of the start of RT as estimated by the model, combinations of MLD and age associated with a risk of 3% and 10% are illustrated in with iso-probability curves. The agreement between modelled and observed respiratory-related mortality within 180 days is visualised in . The area under the ROC curve was 0.72.
Figure 3. (A) Iso-probability curves representing 3% and 10% risk of respiratory-related death within 180 days of the start of radiation therapy as a function of mean lung dose and age. The shaded areas correspond to the 95% confidence intervals. (B) Calibration plot comparing modelled and observed respiratory-related mortality within 180 days. Patients are stratified into seven equally large bins according to their modelled mortality. The positions of the bins on the horizontal axis are at the mean modelled mortality in each bin. The vertical error bars are the 95% binomial confidence intervals, the numbers above are the observed data in each bin (events/total) and the blue line is the identity line.

Discussion
Automatic data extraction was successfully developed and implemented, and resulted in a large study population of 1851 consecutively treated patients from four hospitals. The collected data were used to investigate the impact of MLD and age on early mortality. Both MLD and age were found to impact early respiratory-related mortality as well as early overall mortality.
The scripting capability of ARIA was used to automatically extract data at the four hospitals. Advantages of using scripting to extract data compared to manual extraction are the time efficiency and that human errors in interpretation and handling of OIS and TPS data are mitigated. However, the data in a database with a well-defined structure are not necessarily consistent. If the data are consistent or not depends to a large extent on how the database content has been created by the users and how this has changed over time. Data extraction by automatic methods therefore requires a thorough understanding of this. Furthermore, any clinical database will also contain inconsistencies and mistakes. This might result in failure to identify eligible patients or that the extracted data are incorrect. While it is difficult to certify that all patients are identified, the correctness of the extracted data can be substantiated by performing QCs. In addition to QCs evaluating the reasonableness of the extracted data and the manual verification of extreme parameter values and randomly selected patients, we handled some deviating clinical practices during the studied time period within the script. We identified 16 patients in different QCs that were ineligible for analysis: 10 patients with incorrect lung delineations and six patients that did not meet the inclusion criteria. This might seem as a very low number for a study population of 1851 patients but can partly be explained by the iteratively developed script where issues were addressed early in the process.
In this study, we found that the risk of the previously unstudied endpoint early respiratory-related death within 180 days of the start of RT is associated with the MLD. The decision to use the mean dose to both lungs in combination as a parameter in the analysis instead of using dose parameters related to, for example, the ipsilateral or contralateral lung separately was guided by our clinical practice and previous reports in the literature [Citation5,Citation7,Citation18–20]. The MLD has been found to impact both various grades of RP as well as early overall death following RT of lung cancer [Citation5,Citation7,Citation18–21]. The risk of overall death within 90 and 180 days was associated with the MLD in our study as well. The volume of the target is associated with the MLD, which could mean that disease-related death also is associated with MLD. We found that one of the most common causes of death, even in this early period after RT, was due to the disease. Therefore, early overall mortality might not be suitable to use as a substitute for early treatment-related mortality.
In our study cohort, older patients had a higher risk of all considered endpoints compared to younger patients. In two previous studies, age was not found to be associated with fatal RP [Citation12] or with overall death within 90 days from treatment completion [Citation8]. Both studies also included other clinical factors in their analysis. Ambiguous reports of the relationship between age and early mortality might be an effect of age being correlated to for instance comorbidities or performance status. Such correlations make it challenging to distinguish the impact of age itself, even in multivariable analyses.
The classification of early respiratory-related death caused by RT includes uncertainties that to some extent will be caused by the difficulty of interpreting the actual cause of death. We made an effort to classify all disease-related causes of death as belonging to category A. The respiratory-related deaths may include, besides RP, also other respiratory-related causes of death, such as pneumonia, pulmonary embolism or COPD. It is difficult to know whether if, or to what extent, the RT contributed to make those respiratory-related comorbidities fatal. The way we defined these endpoints, we believe that it is more likely that we have included causes of death that were not treatment-related than it is that we have missed to include respiratory-related causes of death.
The fact that we consider a long period of time, in our case 16 years, may introduce uncertainties in the results. There are variations in treatment procedures over the years which we have not considered in the modelling, for example, delivering VMAT instead of 3DCRT, and changes in imaging procedures such as the introduction of 4DCT for treatment planning.
There are multiple clinical risk factors whose relationship to early respiratory-related mortality and early overall mortality would be of interest to quantify, such as pulmonary function, comorbidity, performance status, BMI, as well as dose parameters besides the MLD, e.g., the mean heart dose. Unfortunately, there are currently limitations in using automatic methods to extract some parameters. All clinical systems do not allow for automatic extraction of data and in those cases time consuming manual data collection is required. Furthermore, for inclusion of dose parameters, it is required that the organ of interest has been delineated in a systematic way for all patients. In our case, for example, the heart was not delineated routinely in the early treatment years. Including the dose to the heart in the analysis would therefore require substantial delineation effort. However, the inclusion of age in our model results in further individualised risk stratification beyond using a strictly MLD-based model [Citation5].
Conclusions
Automatic data extraction was successfully used to pool data from four hospitals, and a model quantifying the risk of early respiratory-related death after RT of NSCLC based on MLD and age could be created. In our study population, the MLD and age were associated with the risk of respiratory-related death within 180 days of the start of RT as well as with the risk of overall death within 90 and 180 days.
Disclosure statement
Dr. Claus F. Behrens and Dr. Ivan R. Vogelius report grants and non-financial support from Varian Medical Systems during the conduct of the study and grants from ViewRay outside the submitted work.
Additional information
Funding
References
- Spencer K, Ellis R, Birch R, et al. Caution is required in the implementation of 90-day mortality indicators for radiotherapy in a curative setting: a retrospective population-based analysis of over 16,000 episodes. Radiother Oncol. 2017;125(1):140–146.
- Warner A, Dahele M, Hu B, et al. Factors associated with early mortality in patients treated with concurrent chemoradiation therapy for locally advanced non-small cell lung cancer. Int J Radiat Oncol Biol Phys. 2016;94(3):612–620.
- Deasy JO, Bentzen SM, Jackson A, et al. Improving normal tissue complication probability models: the need to adopt a “data-pooling” culture. Int J Radiat Oncol Biol Phys. 2010;76(3):S151–S154.
- Bradley JD, Paulus R, Komaki R, et al. Standard-dose versus high-dose conformal radiotherapy with concurrent and consolidation carboplatin plus paclitaxel with or without cetuximab for patients with stage IIIA or IIIB non-small-cell lung cancer (RTOG 0617): a randomised, two-by-two factorial phase 3 study. Lancet Oncol. 2015;16(2):187–199.
- Marks LB, Bentzen SM, Deasy JO, et al. Radiation dose–volume effects in the lung. Int J Radiat Oncol Biol Phys. 2010;76(3):70–76.
- Vogelius IR, Bentzen SM. A literature-based meta-analysis of clinical risk factors for development of radiation induced pneumonitis. Acta Oncol. 2012;51(8):975–983.
- Tucker SL, Liu A, Gomez D, et al. Impact of heart and lung dose on early survival in patients with non-small cell lung cancer treated with chemoradiation. Radiother Oncol. 2016;119(3):495–500.
- Bowden JCS, Williams LJ, Simms A, et al. Prediction of 90 day and overall survival after chemoradiotherapy for lung cancer: role of performance status and body composition. Clin Oncol. 2017;29(9):576–584.
- Appelt AL, Vogelius IR, Farr KP, et al. Towards individualized dose constraints: adjusting the QUANTEC radiation pneumonitis model for clinical risk factors. Acta Oncol. 2014;53(5):605–612.
- Thor M, Deasy J, Iyer A, et al. Toward personalized dose-prescription in locally advanced non-small cell lung cancer: validation of published normal tissue complication probability models. Radiother Oncol. 2019;138:45–51.
- Jin H, Tucker SL, Liu HH, et al. Dose–volume thresholds and smoking status for the risk of treatment-related pneumonitis in inoperable non-small cell lung cancer treated with definitive radiotherapy. Radiother Oncol. 2009;91(3):427–432.
- Palma DA, Senan S, Tsujino K, et al. Predicting radiation pneumonitis after chemoradiation therapy for lung cancer: an international individual patient data meta-analysis. Int J Radiat Oncol Biol Phys. 2013;85(2):444–450.
- Shi A, Zhu G, Wu H, et al. Analysis of clinical and dosimetric factors associated with severe acute radiation pneumonitis in patients with locally advanced non-small cell lung cancer treated with concurrent chemotherapy and intensity-modulated radiotherapy. Radiat Oncol. 2010;5(1):35.
- Khalil AA, Hoffmann L, Moeller DS, et al. New dose constraint reduces radiation-induced fatal pneumonitis in locally advanced non-small cell lung cancer patients treated with intensity-modulated radiotherapy. Acta Oncol. 2015;54(9):1343–1349.
- Keffer S, Guy CL, Weiss E. Fatal radiation pneumonitis: literature review and case series. Adv Radiat Oncol. (in press).
- Sobin LH, Gospodarowicz MK, Wittekind C, editors. 2011. TNM classification of malignant tumours. Chichester, UK: John Wiley & Sons.
- Hedin E, Bäck A. Influence of different dose calculation algorithms on the estimate of NTCP for lung complications. J Appl Clin Med Phys. 2013;14(5):127–139.
- Seppenwoolde Y, Lebesque JV, De Jaeger K, et al. Comparing different NTCP models that predict the incidence of radiation pneumonitis. Int J Radiat Oncol Biol Phys. 2003;55(3):724–735.
- Wijsman R, Dankers FJ, Troost EG, et al. Inclusion of incidental radiation dose to the cardiac atria and ventricles does not improve the prediction of radiation pneumonitis in advanced-stage non-small cell lung cancer patients treated with intensity modulated radiation therapy. Int J Radiat Oncol Biol Phys. 2017;99(2):434–441.
- Kwa SL, Lebesque JV, Theuws JC, et al. Radiation pneumonitis as a function of mean lung dose: an analysis of pooled data of 540 patients. Int J Radiat Oncol Biol Phys. 1998;42(1):1–9.
- Tang X, Li Y, Tian X, et al. Predicting severe acute radiation pneumonitis in patients with non-small cell lung cancer receiving postoperative radiotherapy: development and internal validation of a nomogram based on the clinical and dose–volume histogram parameters. Radiother Oncol. 2019;132:197–203.