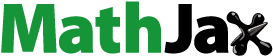
Abstract
Objective
A growing literature on patient preferences informs decisions in research, regulatory science, and value assessment, but few studies have explored how preferences vary across patients with differing treatment experience. We sought to quantify patient preferences for the benefits and risks of lung cancer treatment and test how preferences differed by line of therapy (LOT).
Methods
Preferences were elicited using a discrete choice experiment (DCE) following rigorous patient and stakeholder engagement. The DCE spanned five attributes (each with three levels): progression-free survival (PFS), short-term side effects, long-term side effects, risk of developing late-onset side effects, and mode of administration (MOA) – each defined across 3 relevant levels. A D-efficient design was used to generate 3 survey blocks of 9 paired-profile choice tasks each and respondents were asked which profile they preferred and then if they preferred to have no treatment (opt-out). A mixed logit model, controlling for opt-out, was used to estimate preferences. Preferences and trade-offs between PFS and other attributes were compared across two groups: those receiving ≤1 LOT and those receiving ≥2 LOT.
Results
Of the 466 participants, 42% received ≤1 LOT and 58% received ≥2 LOT. Stated preferences differed between the groups overall (p<.001) and specifically for 18 months of PFS (p<.001), moderate short-term side effects (p<.001), no long-term side effects (p=.03), and 30% chance of late-onset side effects (p=.02). Those receiving differing amounts of LOT were willing to trade different amounts of PFS to change from moderate to mild short-term side effects (p<.001), moderate to no (p<.001) and mild to no (p<.001) long-term side effects. There were also differing amounts of tradeoff acceptable between the groups for a 10% decrease in risk of late-onset side effects (p=.016), a decrease in MOA from infusion every 3 weeks to pills taken daily at any time (p=.005) and from pills taken daily without food to pills taken daily at any time (p<.001).
Conclusion
We demonstrate differences in preferences based on experience with LOT, suggesting that patient treatment experience may have an impact on their preferences. As patient preference data become an important component of treatment decision making, preference differences should be considered when recommending therapies at different stages in the treatment journey. Understanding patient preferences regarding treatment decisions is essential to informing shared decision-making and ensuring treatment plans are consistent with patients’ goals.
Introduction
Lung cancer is the leading cause of cancer deaths in the United StatesCitation1 and the most common cancer diagnosisCitation1. Globally, lung cancer is the most common type of cancer for both diagnoses and deathsCitation2. The burden of lung cancer is largely due to its high mortality rate – it has a 19% five-year survival rate, which is among the lowest of all cancersCitation3. Almost 80% of lung cancer cases are diagnosed at a late stage (stage III or IV) due to the asymptomatic nature of the disease in early stages and often the malignant tumor has already spread to other parts of the bodyCitation4. Line of therapy (LOT) is defined as the number of therapies a patient receives to treat their lung cancer (excluding maintenance therapy) in their lifetime. Patients often receive multiple LOT in order to slow disease progression and prolong survivalCitation5. LOT is an important parameter both from a drug development and a clinical practice point of view. LOT is often an eligibility criterion for lung cancer clinical trials. Some clinical trials, especially phase 1 clinical trials, may be exclusively available to patients who have been on multiple LOTs. More LOTs are associated with more side effects (higher accumulation) the more treatment a patient receives and therefore will impact how clinical decisions can be made.
New treatment options have become available for non-small cell lung cancer (NSCLC) patients in recent years with the development of targeted therapies and immunotherapy. Since 2015, the US Food and Drug Administration (FDA) approved several new drugs for the treatment of NSCLC, the most frequent histological subtype of lung cancer. However, the side effects and toxicities of these treatments can be significant. With the appearance of new treatment options for lung cancer, the complexity of treatment decisions for people living with lung cancer has increased. Preference research finds that patients with lung cancer are willing to endure prolonged treatment in exchange for longer survivalCitation6,Citation7 and that experiences concerning physical, psychological and organizational areas can affect preferencesCitation8. Lung cancer is at the forefront of precision medicine with biomarker-driven care becoming the mainstay for treatment of patients with advanced-stage disease. Patients with lung cancer with targetable driver mutations such as Epidermal Growth Factor Receptor (EGFR)Citation9 or Anaplastic lymphoma kinase (ALK)Citation10 have multiple treatment options available and are living longer with targeted oral treatments.
While research on patient preferences in lung cancer has increased over timeCitation11–14, patient preference heterogeneity is still not well understood. Prior to the advent of biomarker-driven care, willingness to pay for a new treatment for advanced NSCLC has been shown to be higher among those with a history of chemotherapy compared with those who had not received chemotherapyCitation15. A more recent study showed a statistically stronger preference for “clearly longer survival” in a latent class analysis of patients with all cancer types who had received surgery, but patients with either colon or lung cancer were included in that analysisCitation6. There is a paucity of evidence on how clinical factors such as stage, histology at diagnosis, and presence of targetable driver mutations interact with LOT to affect patient preference.
Diverse stakeholders, including regulatory organizations such as the FDA, understand the importance of incorporating the voice of patients into drug development and regulatory benefit-risk analysis. Patient testimony has been a mainstay in patient advocacy and is the primary emphasis of patient-focused drug development (PFDD). In light of the recent twenty first Century Cures Act, the FDA now supports the collection of patient experience data including quantitative patient preference informationCitation16. Guidelines for lung cancer treatment written by the National Comprehensive Cancer Network (NCCN) recognize patient heterogeneity in recommendations, with some treatments only recommended in certain patient populationsCitation17. Preferences vary across patient groups, and decision makers have stressed the importance of studying this heterogeneityCitation18.
Cancer treatment decisions are complex and patient preferences for treatments can vary widely. It is critical that treatment decisions for NSCLC take into account the risks patients are willing to accept in order to attain or avoid benefits and side effects. We sought to quantify the preferences of people with NSCLC and explore variation of preferences by LOT. Understanding preference heterogeneity is a key requirement of preference studies as outlined by the FDA guidance and current good research practices. It can allow decision makers to better understand decision populations and to customize decision rule to meet the needs of specific populations. While it was not our primary intention, evidence on variation in treatment preference by LOT can demonstrate to practicing clinicians how treatment experience can affect patient preferences.
Materials and methods
Survey development
The project was guided through rigorous engagement with patients with lung cancer, modeled after previous patient-engagement exercisesCitation19. A 27-member patient action committee (PAC) was formed through the survivors’ board of a patient advocacy organization, LUNGevity Foundation, which guided the development of the survey (LUNGevity website). PAC members represented a range of demographic and clinical backgrounds. The engagement period spanned multiple years and consisted of PAC meetings, individual interviews, and email communication. Engagement activities were first geared towards acquainting the study team and PAC membersCitation20 and defining project goalsCitation21, and then shifted towards developing a pilot surveyCitation22, and the final DCE survey.
The survey was developed through an iterative mixed-methods processCitation23,Citation24 and followed good-research practices for attribute identification and survey developmentCitation25,Citation26. Attributes were identified from the literatureCitation12 and interviews with PAC membersCitation20,Citation21. Levels were selected based on efficacy and risks associated with existing lung cancer medications. The survey was emailed to all PAC members for comment, with pre-test interviews conducted with four PAC members to identify usability issues. The survey was then pilot tested at three in-person lung cancer survivorship summits. Appropriate and iterative adaptations were made after the pretest interviews and each pilot test round.
The DCE consisted of five attributes that could be presented at three levels each: progression free survival (PFS; 12, 18, 24 months), severity of short-term side effects (mild, moderate, severe), severity of long-term side effects (none, mild, moderate), risk of late-onset side effects (10, 20, 30%), and mode of administration (pill taken daily at any time, pills taken daily that you need to take 1 h before eating or 2 h after eating, infusion in the hospital or clinic every 3 weeks). PFS was chosen over overall survival (OS) as previous studies demonstrate that patients are often not willing to trade between OS and other attributesCitation27. In addition, pilot testing of the survey also revealed that lung cancer patients valued PFS over PS as an attribute for the DCE.
From the five attributes at three levels, 243 separate profiles or more than 50,000 profile pairs could be generated. To narrow the number of choice options and balance response burden and statistical efficiencyCitation28, the DCE employed a blocked D-efficient main-effects experimental design using Ngene software (ChoiceMetrics). Participants were randomly assigned to one of the three blocks of 9 paired comparisons each (27 in total). Priors were not incorporated in the experimental design, as correlation introduced by including priors reduced our ability to estimate independent preference coefficients in previous studiesCitation29. A fixed choice task, which allowed for testing of version consistency, was added to each survey blockCitation30. Therefore, participants completed 10 paired-comparison choice tasks each.
Participants were shown a series of paired hypothetical treatment profiles and asked to select the profile they preferred. As participants were at varying stages of disease, profiles were presented as fixed health profiles and asked participants to select a profile while considering that they are newly diagnosed with lung cancer. The use of a fixed profile has been successfully used to reduce measurement biasCitation11,Citation31. An opt-out option was added after each task to evaluate the potential selection of no treatmentCitation32,Citation33. This dual response design was chosen to include the possible choice of no treatment, while also ensuring efficiencyCitation34. Full scenarios were shown to maximize information on acceptable tradeoffs in each choiceCitation35. An example choice task is presented in .
We examined rates of nonresponse and task nonattendance of the choice tasks. Acceptability of the DCE was assessed to ensure patient-centeredness, relevance, and response burden in accordance with stated-preference method guidanceCitation18,Citation36. Participants were asked to indicate their level of agreement on a 5-point Likert scale ranging from strongly disagree to strongly agree on four statements that assessed response burden (ease of understanding and ease of answering), response consistency, and relevance of the DCECitation37,Citation38. The survey also included self-report questions about demographic information and disease/treatment history, personality traits, patients’ experiences with side effects, and their concerns about lung cancer treatment.
Survey distribution and study sample
The survey was programmed in Qualtrics (Provo, Utah) and distributed online. Multiple recruitment channels were chosen to ensure a widespread distribution of participants. This included recruitment through the patient advocacy and research organizations LUNGevity Foundation, Cancer Support Community, Patient Advocate Foundation, and Edge Research. Participants were required to be at least 18 years of age, live in the US, and have been diagnosed with NSCLC. Underrepresented minorities were oversampled based on race and ethnicity.
Statistical analysis
Responses from patients with NSCLC who completed at least once choice task were included in the analysis. Analyses were conducted separately for participants that had received ≤1 LOT and ≥2 LOT. Demographic and clinical characteristics, concerns regarding lung cancer treatment, and side effect experience were analyzed descriptively. Differences between groups with varying number of LOT were assessed using Fisher’s exact test or a t-test, as appropriate. All tests of significance were two-sided and statistical significance was established at p<.05. Statistical analyses were performed using Stata version 15 (StatCorp, College Station, TX, USA) and R, version 3.6.1 (R Core Team, 2019).
Subjective evaluation
We explored the acceptability of the DCE instrument by evaluating the Likert responses for the DCE choice task evaluation questions and comparing to an agreement threshold of 75%. This threshold was based on previous research reporting agreement with these itemsCitation37,Citation39. The questions were summarized as the percentage of participants that chose either “Agree” or “Strongly Agree” to each of the statements. Each of these percentages were compared to a 75% agreement benchmark using a one-proportion, two-sided z-test wherein a p-value of >.05 indicated that item met the benchmark, and a p-value <.05 indicated that the item either was statistically significantly above or below the benchmarkCitation37. Differences in the endorsement of debriefing items across the two LOT groups were compared using two-sample t-tests.
Preference model
The DCE results were analyzed using a mixed logit model, which allows for the examination of preference heterogeneityCitation40. This model assumes that preferences vary across individuals according to a continuous distribution. The model provides mean coefficients as well as a measure of their distributions in the form of a standard deviation (SD). A statistically significant SD indicates that there is significant preference heterogeneity for that attribute. Aggregate models were used to evaluate overall preferences and to explore heterogeneity identified from the mixed logit model. Stratified analyses were conducted to determine if preferences differed between participants that had received different numbers of LOT (≤1 LOT and ≥2 LOT).
The dependent variable was the participants’ preferred treatment in each choice task and the independent variables were the levels of each of the attributes in each profile. Effects coding was utilized for each attribute to provide parameter estimates for each of the three levels. An alternative specific constant (ASC) was used to control for the opt-out option and provided a “no choice” option. After comparing the model fits (based on Akaike Information Criteria and Chi-square) of different mixed logit models including different (sets of) random parameters, all parameters were set at random with a normal distribution for the final analysis, including the ASC. Overall models and individual parameter estimates between those receiving ≤1 LOT and those receiving ≥2 LOT were assessed via Wald testsCitation41. We also looked at the additional stratifications of age, race, gender, and education. Raw parameter estimates were shifted so that a chosen level of each attribute was set to 0.
Healthy month equivalence model
The random parameters from the mixed logit model were estimated in the “preference space”, which means that each estimate represents the marginal utility of that attribute levelCitation42–44. Parameters in the “willingness to pay” (WTP) space are easier to interpret and they can be estimated directly or can be obtained from the marginal utility by dividing it by the coefficient of a covariate expressed in monetary value (a price for example), taken as a non-random parameter. The ratio can then be interpreted as a monetary value (or willingness to pay). Since we have a time attribute as opposed to a price attribute, we were able to calculate time equivalents in this same fashion. That is, the utility (U) participant n acquires from choosing treatment j for task t can be expressed by:
(1)
(1)
Where ɛ is participant-specific random error and and
incorporate both preference estimates and variance-scale for X, a vector of treatment characteristics (short-term side effects, long-term side effects, and mode of administration) and p, the time benefit of PFS, respectively. Trade-offs between changes in attributes and timeCitation45,Citation46 were estimated consistent with healthy-months equivalents (HME) approachCitation22,Citation47,Citation48 so that if we let
EquationEquation (1)
(1)
(1) can be rewritten as:
(2)
(2)
These time equivalents represent the number of additional months of PFS a patient would be willing to give up for a treatment that provides a decrease in side effects or a more desirable mode of administration. Positive HME values indicate that the attribute is desirable and negative HME values indicate that an attribute is undesirable.
PFS and chance of late-onset side effects were recoded as continuous variables to estimate HME values, while short-term side effects, long-term side effects, and mode of administration was recoded using dummy coding. The reference level for each of the dummy coded variable was the level that was hypothesized to be least desirable; severe for short-term side effects, moderate for long-term side effects, and infusion every 3 weeks for mode of administration. This specification allows us to examine how much PFS participants are willing to trade to move from a worse attribute level to a better attribute, i.e. for short-term side effects we evaluated how much PFS participants were willing to trade to go from experiencing severe short-term side effects to experiencing either moderate or mild short-term side effects. A mixed logit was used as before, but PFS was set as fixedCitation49 while short-term side effects, long-term side effects, risk of late onset-side effects, and mode of administration were modeled as random parameters with normal distributions. Difference in time equivalents between those receiving one or less LOT and those receiving two or more LOT were assessed via t-tests.
Results
Descriptive statistics
A total of 466 of the 520 eligible patient participants (90%) completed as least one DCE task. 1% had not yet received a therapy, 41% had received only one LOT, and 58% had two or more LOT. A total of 82 choice tasks were not completed among 17 participants (2.0%). Twelve participants (2.6%) always selected “Drug A” or “Drug B” across all choice tasks indicating task nonattendance. The majority of patients were female (74.4%) and the average age of participants was 58.1 years old (standard deviations [SD] = 13.0). Participants were primarily white (83.8%), with 68.5% being married and 81.1% having children. Clinical factors significantly associated with the number of LOTs received were years since diagnosis (p=.04), stage at diagnosis (p<.001), current stage (p<.001), biomarker presence (p<.001), clinical trial participation (p<.001), and doctor-led decision-making style (p=.004). Patient characteristics can be seen in .
Table 1. Patient characteristics by number of lines of therapy.
Treatment preferences
Aggregate mixed-logit results are presented in . Participants valued all treatment attributes (p < 0.05). Results met face validity as preference estimates moved in the hypothesized and intuitive directionCitation50,Citation51; participants favored PFS and wanted to avoid short-term side effects, long-term side effects, risk of late-onset side effects and more restrictive modes of administration. The coefficient for the ASC was −5.7 (SD = 4.1), participants preferred some treatment to no treatment at all. The large SD associated with the ASC indicates that there was a lot of heterogeneity across participants in the decision between no treatment and the hypothetical treatments presented.
Figure 2. Aggregate preferences: mean preference weights with 95% confidence interval and standard deviation of preference distribution.
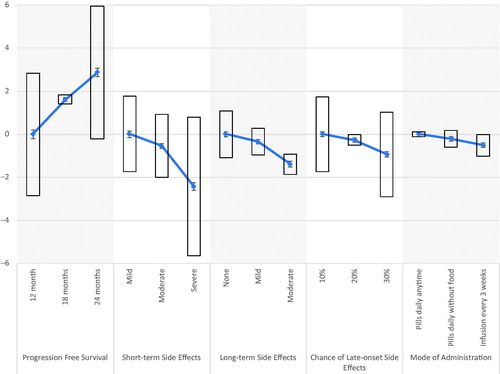
The direction of the preference estimates remained similar to that of the aggregated mixed logit model when stratified by LOT (≤1 or ≥2), but the two models were statistically different as assessed by a Wald test (p<.001) [Citation41]. Stated preferences were statistically different on the following treatment attributes: 18 months (p=.017) and 24 months (p=.004) of PFS, moderate short-term side effects (p<.001), moderate long-term side effects (p=.012), and 30% chance of late-onset side effects (p= <.001). Preferences did not significantly differ across 12 months of PFS, mild or severe short-term side effects, long-term side effects, or mode of administration. The two models also did not differ in the ASC. Re-scaled coefficients are displayed in and graphed in . Additional stratifications of age, gender, race, and education were not found to be statistically significant (p>.05) when assessed by a Wald test.
Table 2. Mixed logit results stratified by LOT.
Healthy-month equivalents
presents the healthy-month equivalents results stratified by LOT. Participants were willing to trade between 1.3 and 11.4 months of PFS in order to decrease side effects or change between modes of administration. Participants that had received ≤1 LOT and ≥2 LOT were willing to trade a different amount of PFS to go from moderate to mild short-term side effects (p<.001), moderate to no (p<.001) and mild to no (p<.001) long-term side effects. There were also differencing amounts of tradeoff acceptable between the groups for a 10% decrease in risk of late-onset side effects (p=.016), a decrease in mode of administration from an infusion every 3 weeks to pills taken daily at any time (p=.005) and from pills taken daily without food to pills taken daily at any time (p<.001). The two groups did not differ on amount of PFS willing to trade to go from severe to mild short-term side effects, but biggest trade-off occurred in both groups here with ≤1 LOT willing to trade 11.4 months of PFS and ≥2 LOT willing to trade 10.8 months. Those with ≤1 LOT were willing to trade more for decreases in long-term side effects and chance of late-onset side effects, while those with ≥2 LOT were willing to trade more for a decrease in moderate to mild short-term side effects, and less restrictive modes of administration.
Debriefing items
The majority of patients found the choice tasks easy to understand (84.8%), easy to answer (66.1%), consistent with their preferences (86.6%), and relevant to them (66.5%). shows the agreement rate with each of these statements by LOT grouping. Acceptability measures met or exceeded the 75% threshold for easy to understand (p<.001) and consistent with preferences (p<.001) for both groups. However, they failed to meet the a priori benchmark for easy to answer and relevant to participants. The groups differed on agreement for finding the choice tasks relevant to them, with the ≤1 LOT group finding the DCE less relevant to them than the ≥2 LOT group (53.7 vs 75.7%, p<.001). The groups did not differ on easy to understand (81 vs 87.5%, p=.06), easy to answer (64.7 vs 67.0%, p=.61), and consistent with their preferences (85.3 vs 87.5%, p=.49). We also explored factors related to finding the choice tasks relevant within the ≤1 LOT group, as the degree of acceptance was so low. We found that current stage was related to relevance with 79.7% of those finding the tasks irrelevant to them to have no evidence of disease.
Discussion
A DCE was used to show that patients with NSCLC have varying preferences for lung cancer treatment. The study sample was composed of a clinically heterogeneous group of patients with lung cancer. Participants had different histologies of NSCLC, were at different disease stages, and had undergone different treatments. We showed that patients with different numbers of LOT displayed different preferences for lung cancer treatment. Significant preference heterogeneity was present. Patients with different number of LOT were willing to trade different amounts of PFS for varying levels of treatment attributes. Thus, efforts to deliver more desirable lung cancer treatments may be more effective if tailored to individuals’ preferences and treatment history.
We found that years since diagnosis, stage at diagnosis, current stage, biomarker presence, lung cancer type (adenocarcinoma vs not), clinical trial participation, and doctor led decision-making style were all significantly associated with LOT. Biomarker-driven lung cancer, specifically adenocarcinoma, tends to have more treatment optionsCitation9,Citation10 and patients having ≤1 LOT are more typically early-stage patients who have received surgery and neoadjuvant/adjuvant chemotherapy with curative intent, while those having ≥2 LOT include more advanced-stage patients with more aggressive tumors. Those patients having ≤1 LOT may also be more willing to try more toxic treatments or treatments with less benefit, since this is a scenario they have not yet experienced. There also may be less patient choice in first line therapy, as indicated by a higher percentage of those in the ≤1 LOT group adopting a doctor-led decision making style about treatment. There are more clinical trials for advanced-stage patientsCitation52 and therefore such patients might be more attuned to a longer PFS for a hypothetical drug. It is therefore not surprising that patients with ≤1 LOT in our cohort were more likely be to early-stage patients with no evidence of disease (NED) and whose tumors lack an actionable biomarker. Conversely, almost 50% of the patients in our cohort who had received ≥2 LOT were more likely to have biomarker-driven advanced-stage lung cancer which tends to be aggressive, have more treatment options, and more clinical trial options available. These factors and their role in preference heterogeneity are not well understood. Further research is warranted to understand the relationships between these factors and number of LOT and how that relates to treatment preferences.
We were able to explore whether participants prefer a treatment with both risks and benefits to no treatment at all by including a treatment opt-out choice. In our models, there was significant variability around the ASC representing the opt-out, but overall treatment was preferred to no treatment. The large negative coefficient of the ASC displays that there is significant disutility association with the no treatment option. This was the case in both LOT sub-groups.
Modeling the short-term side effects and long-term treatment effects attributes as continuous would have resulted in a more parsimonious model. By allowing these variables to be modeled as categorical, we were able to explore whether patients assigned different time equivalence estimates to a change from a severe to a moderate treatment effect or a change from a moderate to a mild treatment effect. Using this specification, the model did not have to assume that patients assigned the same importance to both changes.
Healthy-month equivalents varied over LOT, indicating that those receiving different amounts of therapies place value on different treatment attributes. We found that those with more LOT were willing to trade more PFS for less severe short-term side effects, indicating that those patients may place a greater emphasis on the present. Patients with a greater number of LOT were also willing to trade more PFS for a less intensive mode of treatment administration than those with fewer LOT. These differences may be due to the build-up of treatment-related side effects over time in those patients receiving multiple LOT (i.e. neuropathy). Those with fewer LOT were willing to trade more PFS for less severe long-term side effects and less risk of late-onset side effects, demonstrating that those patients may place more emphasis on the future and may be less worried about the impact of short-term side effects. As LOT is related to disease progression, this may offer some understanding as to where the groups were willing to make differing amounts of trade-off.
Participants were recruited in the US through several methods, including patient advocacy and research organizations. This method of recruitment may result in larger preference heterogeneity, but by using mixed logit models, we were able to capture this variation. While other sample strategies were examined, this strategy was chosen in order to provide the largest sample size, geographic variation, and a better representation of patients. We also excluded family members and caregivers from our analyses. Previous research shows that patient and caregiver preferences may differCitation37,Citation53 and thus we chose to focus on the patient perspective. This allowed us to gain insight about patients’ preferences and trade-offs they were willing to make. This recruitment method also resulted in a sample that was majority female which was consistent with other advocacy based studiesCitation53,Citation54. Greater involvement by women is explained by many factors, including volunteerism and humanistic tendencies. We do not believe that this is presents a bias in our work, but reflects reality in advocacy groups. We made many efforts to be inclusionary in our study, including having diverse patient engagement through the development of our study.
Difficulty in answering DCEs is consistent with previous literatureCitation55. Other methods of preference elicitation, including simple choice or ranking techniques, have been shown to be easier to answer, but a DCE was used here in order to evaluate explicit trade-offs patients were willing to make between PFS and other treatment attributes. DCEs have also been shown to be more consistent with preferences, as was illustrated in our study as well. Recent guidelines for the application of a DCE analysis in health were applied to help direct the analyses and minimize biasCitation36.
This study does not explore reasons why preferences differ over participants with differing amounts of therapies and as number of LOT is associated with other clinical factors, there may be other factors influencing the preference differences we have seen here. This limits implications for the tailoring of lung cancer treatment to patients based on lines of therapy received. Further research is needed including methods such as prospective studies and qualitative interviews that explore this observed difference in preferences between patients with different quantities of therapies. This necessary research will ultimately help clinicians individualize lung treatment.
Conclusion
The study results suggest that lung cancer may be preference-sensitive and that preferences might differ by clinical and demographic characteristics. We showed that LOT is related to differences in preferences and that patients with NSCLC consider both benefits (PFS) and risks in their treatment decision making. Short-term, long-term, and late-onset side effects each factored into patient’s preferences and treatment decisions should consider each of these different types of side effects. Therefore, it is important that patients have access to relevant information and tools to help inform the shared decision-making process regarding their treatment options to ensure patient-centric care.
Transparency
Declaration of funding
This work was supported by LUNGevity (with funding from Celgene, Merck, and Boehringer Ingelheim). Celgene, Merck, and Boehringer Ingelheim had no influence on the design of the project, data collection, analysis, interpretation, or writing of the report.
Declaration of financial/other relationships
The authors report no potential conflicts of interest. Peer reviewers on this manuscript have no relevant financial or other relationships to disclose.
Compliance with ethical standards
This research was conducted in accordance with the Declaration of Helsinki, and the study protocol was reviewed by the Institutional Review Board. Dr. Presley is a Paul Calabresi Scholars supported by the OSU K12 Training Grant for Clinical Faculty Investigators (5K12 CA133250-09)
Acknowledgements
The authors sincerely thank LUNGevity and the members of the Patient Action Committee (PAC) for their participation, engagement, and valuable contributions to Project Transform.
References
- Siegel RL, Miller KD, Jemal A. Cancer statistics, 2020. CA Cancer J Clin. 2020;70(1):7–30.
- Bray F, Ferlay J, Soerjomataram I, et al. Global cancer statistics 2018: GLOBOCAN estimates of incidence and mortality worldwide for 36 cancers in 185 countries. CA Cancer J Clin. 2018;68(6):394–424.
- Siegel RL, Miller KD, Jemal A. Cancer statistics, 2019. CA Cancer J Clin. 2019;69(1):7–34.
- National Institutes of Health, National Cancer Institute. Surveillance, Epidemiology and End Results (SEER) Program. Cancer stat facts: lung and bronchus cancer; 2018 [cited 2019 Sep 29]. Available from: https://seer.cancer.gov/statfacts/html/lungb.html.
- Molina JR, Yang P, Cassivi SD, et al. Non-small cell lung cancer: epidemiology, risk factors, treatment, and survivorship, Mayo Clinic Proceedings. Philadelphia (PA): Elsevier; 2008, pp. 584–594.
- Schmidt K, Damm K, Vogel A, et al. Therapy preferences of patients with lung and colon cancer: a discrete choice experiment. PPA. 2017;11:1647–1656.
- Pacchiana MV, Capelletto E, Carnio S, et al. Patients' attitudes and physicians' perceptions toward maintenance therapy for advanced non-small-cell lung cancer: a multicenter Italian survey . Clin Lung Cancer. 2017;18(4):381–387.
- Aumann I, Kreis K, Damm K, et al. Treatment-related experiences and preferences of patients with lung cancer: a qualitative analysis. Health Expect. 2016;19(6):1226–1236.
- Soria J-C, Ohe Y, Vansteenkiste J, et al. Osimertinib in untreated EGFR-mutated advanced non–small-cell lung cancer. N Engl J Med. 2018;378(2):113–125.
- Peters S, Camidge DR, Shaw AT, et al. Alectinib versus crizotinib in untreated ALK-positive non–small-cell lung cancer. N Engl J Med. 2017;377(9):829–838.
- Bridges JF, Mohamed AF, Finnern HW, et al. Patients' preferences for treatment outcomes for advanced non-small cell lung cancer: a conjoint analysis . Lung Cancer. 2012;77(1):224–231.
- Schmidt K, Damm K, Prenzler A, et al. Preferences of lung cancer patients for treatment and decision-making: a systematic literature review. Eur J Cancer Care. 2016;25(4):580–591.
- Gironés R, Torregrosa D, Gómez-Codina J, et al. Lung cancer chemotherapy decisions in older patients: the role of patient preference and interactions with physicians. Clin Transl Oncol. 2012;14(3):183–189.
- Mühlbacher AC, Bethge S. Patients' preferences: a discrete-choice experiment for treatment of non-small-cell lung cancer. Eur J Health Econ. 2015;16(6):657–670.
- Leighl NB, Tsao WS, Zawisza DL, et al. A willingness-to-pay study of oral epidermal growth factor tyrosine kinase inhibitors in advanced non-small cell lung cancer. Lung Cancer. 2006;51(1):115–121.
- FDA. 21st Century Cures Act; 2016 [cited 2019 Sep 29]. Available from: https://www.congress.gov/114/plaws/publ255/PLAW-114publ255.pdf.
- National Comprehensive Cancer Network. NCCN categories of preference; 2017 [cited 2019 Sep 29]. Available from: https://www.nccn.org/professionals/physician_gls/categories_of_consensus.aspx.
- FDA, patient preference information – voluntary submission, review in premarket approval applications, humanitarian device exemption applications, and de Novo requests, and inclusion in decision summaries and device labeling: guidance for industry, Food and Drug Administration staff, and other stakeholders; 2016 [cited 2019 Jan 14]. Available from: https://www.fda.gov/regulatory-information/search-fda-guidance-documents/patient-preference-information-voluntary-submission-review-premarket-approval-applications.
- Seo J, Smith BD, Estey EH, et al. Assessing patient preferences for the benefits and risks of treating for acute myeloid leukemia (AML): a pilot study. Washington, DC: American Society of Hematology; 2016.
- Dy SM, Janssen EM, Ferris A, et al. Live, learn, pass it on: a patient advocacy engagement project on the lived experience of lung cancer survivors. J Patient Exp. 2017;4(4):162–168.
- Bridges JF, Janssen EM, Ferris A, et al. Project Transform: engaging patient advocates to share their perspectives on improving research, treatment and policy. Curr Med Res Opin. 2018;34(10):1755–1762.
- Janssen EM, Dy SM, Meara AS, et al. Analysis of patient preferences in lung cancer - estimating acceptable tradeoffs between treatment benefit and side effects. Patient Prefer Adherence. 2020;14:927–937.
- Janssen EM, Segal JB, Bridges JF. A framework for instrument development of a choice experiment: an application to type 2 diabetes. Patient. 2016;9(5):465–479.
- Janssen EM, Benz HL, Tsai J-H, et al. Identifying and prioritizing concerns associated with prosthetic devices for use in a benefit-risk assessment: a mixed-methods approach. Expert Rev Med Devices. 2018;15(5):385–398.
- Coast J, Al‐Janabi H, Sutton EJ, et al. Using qualitative methods for attribute development for discrete choice experiments: issues and recommendations. Health Econ. 2012;21(6):730–741.
- Janssen EM, Bridges JF. Art and science of instrument development for stated-preference methods. Patient. 2017;10:377–379.
- Bridges JF, la Cruz Md, Pavilack M, et al. Patient preferences for attributes of tyrosine kinase inhibitor treatments for EGFR mutation-positive non-small-cell lung cancer. Future Oncol. 2019;15(34):3895–3907.
- Johnson FR, Lancsar E, Marshall D, et al. Constructing experimental designs for discrete-choice experiments: report of the ISPOR conjoint analysis experimental design good research practices task force. Value Health. 2013;16(1):3–13.
- Richardson DR, Seo J, Smith BD, et al. Quantifying patient preferences for treatment outcomes in AML: a discrete-choice experiment. Blood. 2018;132(Supplement 1):718–718.
- Janssen EM, Marshall DA, Hauber AB, et al. Improving the quality of discrete-choice experiments in health: how can we assess validity and reliability? Expert Rev Pharmacoecon Outcomes Res. 2017;17(6):531–542.
- Bridges JF, Kinter ET, Schmeding A, et al. Can patients diagnosed with schizophrenia complete choice-based conjoint analysis tasks? Patient. 2011;4(4):267–275.
- Lancsar E, Louviere J. Conducting discrete choice experiments to inform healthcare decision making: a user's guide. Pharmacoeconomics. 2008;26(8):661–677.
- de Bekker‐Grob EW, Ryan M, Gerard K. Discrete choice experiments in health economics: a review of the literature. Health Econ. 2012;21(2):145–172.
- Veldwijk J, Lambooij MS, de Bekker-Grob EW, et al. The effect of including an opt-out option in discrete choice experiments. PLoS One. 2014;9(11):e111805
- Mühlbacher A, Johnson FR. Choice experiments to quantify preferences for health and healthcare: state of the practice. Appl Health Econ Health Policy. 2016;14(3):253–266.
- Bridges JF, Hauber AB, Marshall D, et al. Conjoint analysis applications in health-a checklist: a report of the ISPOR good research practices for Conjoint Analysis Task Force. Value Health. 2011;14(4):403–413.
- Bridges JF, Tsai J-H, Janssen E, et al. How do members of the Duchenne and Becker muscular dystrophy community perceive a discrete-choice experiment incorporating uncertain treatment benefit? An application of research as an event. Patient. 2019;12(2):247–257.
- Janssen EM, Longo DR, Bardsley JK, et al. Education and patient preferences for treating type 2 diabetes: a stratified discrete-choice experiment. Patient Prefer Adherence. 2017;11:1729–1736.
- Janssen EM, Hauber AB, Bridges JF. Conducting a discrete-choice experiment study following recommendations for good research practices: an application for eliciting patient preferences for diabetes treatments. Value Health. 2018;21(1):59–68.
- Hauber AB, González JM, Groothuis-Oudshoorn CG, et al. Statistical methods for the analysis of discrete choice experiments: a report of the ISPOR Conjoint Analysis Good Research Practices Task Force. Value Health. 2016;19(4):300–315.
- Hausman J, McFadden D. Specification tests for the multinomial logit model. Econometrica. 1984;52(5):1219–1240.
- Scarpa R, Thiene M, Train K. Utility in willingness to pay space: a tool to address confounding random scale effects in destination choice to the Alps. Am J Agric Econ. 2008;90(4):994–1010.
- Train K, Weeks M. Discrete choice models in preference space and willingness-to-pay space. Applications of simulation methods in environmental and resource economics. Dordrecht: Springer; 2005. p.1–16.
- Hole AR, Kolstad JR. Mixed logit estimation of willingness to pay distributions: a comparison of models in preference and WTP space using data from a health-related choice experiment. Empir Econ. 2012;42(2):445–469.
- Hauber AB, Itzler R, Johnson FR, et al. Healthy-days time equivalents for outcomes of acute rotavirus infections. Vaccine. 2011;29(45):8086–8093.
- Johnson FR, Hauber AB, Özdemir S. Using conjoint analysis to estimate healthy-year equivalents for acute conditions: an application to vasomotor symptoms. Value Health. 2009;12(1):146–152.
- Mehrez A, Gafni A. Quality-adjusted life years, utility theory, and healthy-years equivalents. Med Decis Making. 1989;9(2):142–149.
- Hauber AB. Healthy-years equivalent: wounded but not yet dead. Expert Rev Pharmacoecon Outcomes Res. 2009;9(3):265–269.
- Train KE. Discrete choice methods with simulation. Cambridge (UK): Cambridge University Press; 2009.
- De Bekker‐Grob EW, Hol L, Donkers B, et al. Labeled versus unlabeled discrete choice experiments in health economics: an application to colorectal cancer screening. Value Health. 2010;13(2):315–323.
- Ryan M, Bate A, Eastmond CJ, et al. Use of discrete choice experiments to elicit preferences. BMJ Quality & Safety. 2001;10(Supplement 1):i55–i60.
- Subramanian J, Regenbogen T, Nagaraj G, et al. Review of ongoing clinical trials in non–small-cell lung cancer: a status report for 2012 from the ClinicalTrials. gov website. J Thorac Oncol. 2013;8(7):860–865.
- Janse SA, Dumanis SB, Huwig T, et al. Patient and caregiver preferences for the potential benefits and risks of a seizure forecasting device: a best–worst scaling. Epilepsy Behav. 2019;96:183–191.
- Peay HL, Hollin I, Fischer R, et al. A community-engaged approach to quantifying caregiver preferences for the benefits and risks of emerging therapies for Duchenne muscular dystrophy. Clin Ther. 2014;36(5):624–637.
- Richardson DR, Crossnohere NL, Seo J, et al. Age at diagnosis and patient preferences for treatment outcomes in AML: a discrete choice experiment to explore meaningful benefits. Cancer Epidemiol Biomarkers Prev. 2020;29(5):942–948.