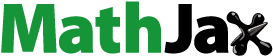
Abstract
Background
Although adolescent basketballers differ in body size, shape, and composition, less is known about how these factors interact during physical development.
Aim
We used ontogenetic allometry to identify the optimal body size and shape characteristics associated with physical performance in adolescent basketball players, and investigated the effects of training experience, training volume, maturity status, and club characteristics on physical performance development.
Subjects and methods
Two hundred and sixty-four male basketballers, from five age-cohorts (11–15 years of age), were followed consecutively over three years. Three physical performance components, anthropometrics, training information, and biological maturation were assessed bi-annually. Longitudinal multiplicative allometric models were developed.
Results
Players with a physique that had a dominant ectomorphic component performed better in all physical performance components. When adjusting for confounders other than size, the development of running speed was independent of body size. Players advanced in maturation were physically fitter. Training data had no significant effect on developmental trajectories of running speed or lower body explosive strength. Club characteristics had no significant association with any physical performance trajectories.
Conclusion
Leaner players have advantages in physical performance and individual characteristics play an important role, over and beyond club structure, in developing physical performance.
Introduction
It is acknowledged that adolescent basketball players of the same competitive age-category differ in body size, shape, and composition, resulting from variations in the timing and tempo of growth (Guimarães, Baxter-Jones, et al. Citation2019; Ramos, Massuça, et al. Citation2021). However, less is known as to how body factors confound the interpretation of physical performance development, since the effects of normal growth may mask or be greater than the developmental changes associated with systematic training (Baxter-Jones et al. Citation1993; Nevill et al. Citation1998).
Allometric scaling provides an insightful approach to interpret differences in physical performance developmental trends that are interrelated with changes in body size, shape, and composition (Beunen et al. Citation2002; Nevill et al. Citation2009). For example, allometric scaling has been used previously to examine inter-individual variation in anaerobic power (Carvalho et al. Citation2011), aerobic fitness (Carvalho et al. Citation2013), and agility (Carvalho et al. Citation2017) in youth basketball players. The findings suggest that the scaling factors are apparently exclusive for each physical performance component. However, these are cross-sectional reports that relied on separate allometric models considering height, or weight, or fat-free mass, as independent and mutually exclusive scaling factors. As such, they were not able, for example, to estimate any stature-to-body mass ratio to compare with the Reciprocal Ponderal Index (RPI) to determine an overall description of body shape or physique (in somatotype terminology this suggests an ectomorphic dominant physique) that may be best linked with physical performance unfolding during puberty. Furthermore, it has been systematically reported that somatotype is linked to physical performance (Carter and Heath Citation1990; Suchomel Citation2002; Kubo et al. Citation2022).
Along with inter-individual differences in body size, shape, and proportions, biological maturation and training history play important roles in explaining physical performance trajectories, especially during periods of rapid growth (Baxter-Jones Citation2017; Ramos, Volossovitch et al. Citation2021). For example, young basketballers who are advanced in biological maturation and/or have more accumulated training experience tend to be physically fitter and outperform their peers in several physical performance tasks (Guimarães, Ramos, et al. Citation2019). Also, somatotype, particularly its ectomorphic component (linearity in physique), is positively related with performance in basketball (Gryko et al. Citation2018). In fact, guards and forwards from top placed teams were shown to be more ectomorphic, that is, to possess a more linear physique than those from less basketball successful teams (Ackland et al. Citation1997; Carter et al. Citation2005). Additionally, the influence of the sporting environment on performance and development of youth athletes is well-known (Rees et al. Citation2016). Players spend so much time with their coaches within the club facilities that there is a strong call for studies linking individual characteristics with environmental factors (Bergeron et al. Citation2015).
Although there is a growing interest in the long-term development of young athletes (Pichardo et al. Citation2018), longitudinal data on the physical development of young basketball players is limited (te Wierike et al. Citation2014; Carvalho et al. Citation2019). Therefore, we use an allometric approach, embedded in a longitudinal multilevel (hierarchical) statistical model, to address two key aims. First, we identified the optimal body size and shape characteristics associated with the physical performance of adolescent basketball players. Second, we investigated the effects of training experience, training volume, maturity status, and club characteristics on physical performance development, using ontogenetic allometry. The following questions were examined: (i) is a more ectomorphic body shape positively associated with physical performance development? (ii) does increased training experience, training volume, and biological maturation enhance physical performance levels? (iii) are club characteristics significantly linked with physical performance development over time?
Subjects and methods
Sample and design
The data for this paper come from the In Search of Excellence - a Mixed-longitudinal Study in Young Athletes (INEX) study, carried out in Porto, Portugal, from 2017 through to 2019. The main purpose of the INEX study was to investigate the interactions among individual characteristics and environmental factors affecting growth, physical and specific-skills performance, game proficiency and psychological development in a variety of sports. The INEX study design is described in detail elsewhere (Guimarães et al. Citation2021a). The basketball sample of the INEX study used a 3-year mixed-longitudinal design with five entry age-cohorts (11, 12, 13, 14, and 15 years) which had 2-year overlaps between age-cohorts, enabling the generation of 7-year developmental trajectories.
A total of 293 adolescent male basketball players coming from 20 out of the 25 clubs in the Porto Basketball Association were randomly selected to participate in the INEX study by their coaches and/or club team managers. Baseline measurements were initiated in June 2017 and repeated bi-annually until December 2019. All assessments occurred during the same time-periods (June and December) within a time window of 15–20 days. The proportion of dropouts across the study was 6%. To be included in the present analysis, complete data were required from a minimum of two, out of the six, time points. Altogether, 264 players fulfilled this condition and 1261 measurements were obtained (). Any information from the clubs was obtained from presidents or directors of the 20 clubs (Guimarães et al. Citation2021a). Written informed consent was obtained from parents or legal guardians as well as individual assent from each participant. The study was approved by the Ethics Committee of the lead institution (CEFADE 13.2017), while the Porto Basketball Association gave formal permission for data collection.
Table 1. Number of measurements per time point for each age category.
Physical performance
Physical performance was assessed using standardised tests: (1) the Yo-Yo Intermittent Recovery Test – Level 1 (Yo-Yo IR1) – aerobic capacity – players performed repeated 40 m (2 × 20 m) runs, with a 10 s active recovery period between runs (Bangsbo et al. Citation2008); (2) 20 m sprint – running speed – players ran in a straight line at full speed (Kirkendall et al. Citation1987); (3) countermovement jump (CMJ) – lower body explosive strength – players performed the vertical jump as advocated by Bosco et al. (Citation1983). A detailed description of the protocol of each test is presented in Guimarães et al. (Citation2021a).
Anthropometry
Body mass was measured using a bio-impedance scale (Tanita®BC-418MA, Tanita Corp., Tokyo, Japan) with a precision of 100 g. Stature (cm) and sitting height (cm) were measured without shoes and with the participant’s head positioned in the Frankfurt plane using a Harpenden stadiometer (Holtain Ltd., Crymych, UK) with a precision of 0.1 cm. Measurements were taken following the International Working Group on Kinanthropometry protocols (Ross and Marfell-Jones Citation1995).
Training information
The players’ training experience, expressed as accumulated years of formal basketball training, was obtained from self-report questionnaires and validated against registration histories available from the official website of the Portuguese Basketball Federation (FPB): http://www.fpb.pt. Training volume data, expressed as training hours per week, were obtained from self-report questionnaires and confirmed by coaches and/or club team managers.
Biological maturation
Biological maturation was assessed by predicting years from attainment of peak height velocity (PHV), a maturational milestone presented as a biological age. A prediction equation from anthropometric data was used to predict age from PHV (Mirwald et al. Citation2002). The equation uses sex-specific formula of measures of chronological age, height, sitting height and body mass to predict years from or after the occurrence of PHV, termed as “maturity offset (years from PHV).” A positive (+) maturity offset represents the predicted number of years the participant is beyond their age of PHV, whereas a negative (–) value represents the predicted number of years before the attainment of their PHV.
Data quality control
Data quality control was ensured using a five-step procedure: (1) anthropometric measurements were performed by trained personnel from the Kinanthropometry Lab of the lead institution; (2) an in-field reliability approach was used such that a random sample of three-to-five participants was re-measured every day; (3) reliability estimates were computed using the technical error of measurement (TEM) as well as ANOVA-based intraclass correlations (R), the TEM was 0.1 kg for body mass, 0.2 cm for stature, and 0.1 cm for sitting height and the R-values for physical performance tests ranged from 0.93 (CMJ) to 0.99 (20 m sprint); (4) data cleaning was undertaken to control for punching errors in data entry as well as the putative presence of outliers; (5) normality checks in the distributions of all variables were undertaken.
Statistical analysis
The descriptive statistics are presented as means and standard deviations (Mean ± SD) and were calculated using IBM SPSS 27.0 (IBM Corp., Armonk, NY, USA). Since data of repeated observations (level-1) were nested within players (level-2) who were nested within clubs (level-3) a multilevel (hierarchical) model (Snijders and Bosker Citation2012) with fixed and random effects was used in Stata 17.0 software (StataCorp, College Station, TX, USA). Fixed effects comprised a quadratic term of age (age2 to account for non-linearity), as well as time-varying covariates (training experience, weekly hours of training, and maturity offset), whereas random effects accounted for variation between players at baseline and their longitudinal trends, plus a club component and a residual term. We first used a linear model with height and weight, and then compared it to the ontogenetic multiplicative allometric model (Nevill et al. Citation1998) and tested the fit using loglikelihood ratio statistics (results not presented). It was found that the allometric model fitted the data better. We then used a sequential approach with two steps. In step 1, each physical performance component was modelled as: y = body massk1 · staturek2 · exp (ai + bi·age + ci·age2) εij, which is a log-linear multilevel regression model that can be expressed this way for the 20 m sprint, for example (for the other physical performance components the equation is the same),
Where k1 and k2 are the allometric ontogenetic coefficients, ai and bi are allowed to vary randomly from player to player (level-2), and εij is assumed to have a constant error variance between repeated observations (level-1). The polynomial of age (age + age2) accounts for the curvilinear trend in all physical performance tests (Guimarães et al. Citation2021b).
In step 2, the previous model was expanded with the inclusion of three time-varying covariates namely, training experience (texp), weekly hours of training (wht) and maturity offset (moff). The log-linear model is now expressed this way (again for 20 m sprint),
Although we have previously shown that level-3 (club) does not significantly relate to differences in players' intraindividual changes in physical performance tests, we maintained it in the random part of the model as advocated by Hedeker and Gibbons (Citation2006). All parameters were simultaneously estimated using full maximum likelihood procedures implemented in the multilevel mixed effects module of the Stata 17 software (StataCorp Citation2021). Since model 1 is nested within model 2, we used the likelihood ratio test to compare them as is generally advocated (Hedeker and Gibbons Citation2006). In all analyses the significance level was set at 5%.
Results
The descriptive statistics for physical performance components, anthropometry, training information, and biological maturation are shown in . In general, players became fitter with increasing age. That is, the performance on the Yo-Yo IR1, 20 m sprint time, and CMJ test improved with age. In addition, body mass, stature, sitting height, training experience, and maturity offset averages increased with age, whereas mean training volume showed a distinct pattern, with the number of training hours per week being the same until 16.0 years of age and then increasing.
Table 2. Descriptive statistics (mean ± SD) for basketball players across the study years.
The allometric scaling coefficients from Model 1 are shown in . All physical performance tests unfolded in a linear fashion (only age centred is statistically significant, p < 0.05) and all parameter estimates for fixed effects were statistically significant (p < 0.01), with the only exception being the Yo-Yo IR1. In the Yo-Yo IR1, the multiplicative allometric scaling model showed that aerobic capacity (distance covered in m) = mass−1.353 · stature4.498 = (stature · mass−0.300)4.498. This stature-to-body mass ratio is similar to the RPI (i.e. stature · mass−0.333), suggesting that young basketballers with a tendency for a more linear physique (in somatotype terms with an ectomorphic dominance) have advantages in aerobic capacity across age. The models for the 20 m sprint (m·s−1) = mass−0.130 · stature0.660 = (stature · mass−0.197)0.660 and the lower body explosive strength (cm) = mass−0.440 · stature1.856 = (stature · mass−0.237)1.856 reveal a relatively similar trend, i.e. less heavy and taller players have advantages in explosive strength relative to their peers with no such physical configuration. A non-significant random component is evident for the club level, whereas there is a significant indication of random intercepts and random slopes for the three tests, but no significant covariances were observed for the Yo-Yo IR1 and 20 m sprint tests. As expected, the residual random component for all tests was statistically significant.
Table 3. Multilevel regression models 1 for physical performance development: parameter estimates ± standard-errors for fixed and random effects.
Model 2 (with the inclusion of training experience, training volume and maturity offset) fitted the data better than Model 1 (). The new model showed that the performances in all tests now have curvilinear trends (age and age2 are statistically significant, p < 0.05). In the Yo-Yo IR1, the multiplicative allometric scaling model in aerobic capacity (distance covered in m) = mass−1.493 · stature3.426 = (stature · mass−0.436)3.426 after adjusting for all covariates in the model (all significant but training experience). This finding confirms that young basketballers with a more linear physique are more prone to excel in aerobic capacity across age even though the scaling is slightly greater than the expected for the RPI. The model for the 20 m sprint (m·s−1) = mass−0.219 · stature0 and showed that running speed is independent of size when adjusted for the covariates in the model (only the maturity offset is statistically significant). For the lower body explosive strength (cm) = mass−0.489 · stature1.379 = (stature · mass−0.355)1.379 when adjusted for the covariates in the model (again only the maturity offset is statistically significant). The scaling coefficients are now like those of the RPI, that is, less heavy and taller players have clear advantages in explosive strength performance across age. As per Model 1, a non-significant random component is evident for the club level, however random intercepts and random slopes are significant for the three tests, and a non-significant covariance was evident for the Yo-Yo IR1 test. The residual random component for each test was statistically significant.
Table 4. Multilevel regression models 2 for physical performance development: parameter estimates ± standard-errors for fixed and random effects.
Discussion
Our study reports a novel attempt to use ontogenetic allometry embedded in a longitudinal multilevel modelling with three levels to (i) identify an optimal body size, shape, and composition associated with successful physical performance developmental trajectories, (ii) investigate the effects of training experience, training volume, and maturity status, and (iii) test the impact of club characteristics on players’ physical performance development. Our findings consistently showed that an overall physique with a dominant ectomorphic component, that is, taller and less heavy, positively favours physical performance developmental trends. However, when adjusting for training experience, training volume, and maturity offset above and beyond the scaling factors, the development of running speed becomes independent of body size. When considering a polynomial of age (age and age2) a curvilinear physical performance developing trend was observed.
Allometric scaling has a long history (Gould Citation1966) and has been frequently used to partition out differences in body size, shape, and composition in different physical performance components in non-athletic (Corlett Citation1984; Nevill et al. Citation1998, Citation2009; Beunen et al. Citation2002; Silva et al. Citation2016), as well as in athletic populations, namely adolescent basketball players (Carvalho et al. Citation2011, Citation2013, Citation2017). However, the above-mentioned reports are cross-sectional in nature and used a single scaling factor such as height, weight, or lean body mass. Few researchers have employed longitudinal designs coupled with ontogenetic allometry to examine growth-related changes of physical performance in young athletes (Baxter-Jones et al. Citation1993; Beunen et al. Citation1997; Eisenmann et al. Citation2001; Valente-dos-Santos et al. Citation2014). In fact, longitudinal data using allometric models related to the unfolding of physical performance in youth basketballers are non-existent, which poses problems when comparing our findings.
In the Yo-Yo IR1 test it was found that stature-to-body mass ratio (stature · mass−0.436)3.426 best characterises the optimal relationship between aerobic capacity and shape. That is, young basketball players who are relatively taller displaying a linear physique, mostly ectomorphic, have advantages in aerobic capacity across age, even after adjusting for all covariates. Consistent with previous studies (Nevill et al. Citation2009; Bustamante et al. Citation2015; Silva et al. Citation2016), our findings support the notion that stature-to-body mass ratio (known as the RPI = height · mass−0.333) is a suitable predictor of aerobic capacity development. A different picture emerges when the allometric approach is not employed. For example, Carvalho et al. (Citation2019) reported no significant influence of body size (stature and body mass) on the longitudinal changes of the Yo-Yo IR1 in female adolescent basketball players. Training experience did not independently explain differences in the developmental trends of the Yo-Yo IR1. Although not using allometric scaling, Carvalho et al. (Citation2019) reported similar findings in young female basketballers followed longitudinally. In contrast, training volume data showed that basketball players who train more hours per week tend to have less aerobic capacity across time. However, the minor variation of training volume across the study years (players always trained 4.5 h·week−1 from 11.0 to 16.0 years) may explain this unexpected result. These findings suggest that aerobic capacity is perhaps mostly linked with growth changes and not training, as biological maturation was positively related to changes in aerobic capacity. These are expected results since maturation is accompanied by morphological and physiological development leading to increases in fat-free mass, heart volume, lung function, aerobic power, and muscular strength (Baxter-Jones Citation1995). Consequently, during adolescence, basketball players advanced in maturity status tend to outperform their late maturing peers in, at least two different Yo-Yo tests (Carvalho et al. Citation2018; Arede et al. Citation2019; Guimarães, Baxter Jones, et al. Citation2019; Guimarães, Ramos, et al. Citation2019; Guimarães et al. Citation2021a).
The allometric model used to predict running speed (20 m sprint) identified the optimal stature-to-body mass ratio mostly linked to the performance in this test to be (stature · mass−0.197)0.660. In line with previous data (Nevill et al. Citation2009; Bustamante et al. Citation2015; Silva et al. Citation2016), sprint performance of young basketball players appears to benefit from being taller and leaner. However, after adjusting for covariates above and beyond the scaling factors, the development of running speed became size-independent. This confirms the assumption that running speed is theoretically size-independent, that is, the theoretical exponent = 0 (Asmussen and Heeboll-Nielsen Citation1955; Corlett Citation1984). These are surprising findings because such assumptions are based on the geometric similarity theory, which suggests that human physiques are geometrically like each other, which is not correct (Nevill et al. Citation2009). Furthermore, training experience and training volume were not significantly independently associated with the developmental trajectories of running speed, when other confounders were in the model. It would be expected that both markers of training were significantly linked with running speed trajectories over time since more time engaged in training and competition usually represents more opportunities for players to improve their overall physical performance. However, training data collected retrospectively, as the amount of training expressed in hours, is probably not a sufficiently sensitive indicator (Valente-dos-Santos et al. Citation2014). On the other hand, biological maturation was positively linked with running speed development. These results corroborate our previous cross-sectional data from Portuguese adolescent basketball players suggesting that maturity status, rather than years of training, was the primary contributor to several physical performance components (Guimarães, Ramos, et al. Citation2019). This last finding is most certainly linked to the fact that youngsters undergo greater morphological and physiological changes during maturation responsible for major improvements in physical performance (Baxter-Jones Citation1995; Malina et al. Citation2004).
In the CMJ test (marker of lower body explosive strength), the allometric model showed negative body mass and positive stature exponents, confirming that an appropriate proportion of stature/body mass is related to greater performance (Nevill et al. Citation2009; Bustamante et al. Citation2015; Silva et al. Citation2016). As defined by our results on stature-to-body mass ratio (stature · mass−0.355)1.379, basketball players with linear physiques (i.e. with a more ectomorphic body shape) have advantages in the CMJ since the body needs to be vertically propelled. Once again, our findings do not align with those from Carvalho et al. (Citation2019) who showed that stature and body mass did not affect the development of CMJ over time in young female basketball players. We, therefore, highlight the importance of using allometric scaling to better partition differences in body size, shape, and composition of youth athletes. Like running speed, none of the training history predictors significantly influenced the longitudinal changes in the CMJ. Biological maturation independently explained differences in developmental trajectory trends of CMJ. This confirms our previous cross-sectional data reporting that biological maturation positively impacts on vertical jump performance (Guimarães, Baxter Jones, et al. Citation2019; Guimarães, Ramos, et al. Citation2019; Guimarães, et al. Citation2021a). As young players mature, their performance is enhanced by significant gains in the characteristics of muscular strength and power (Baxter-Jones Citation1995).
The three physical performance tests followed a curvilinear developmental trend, which corroborates previous longitudinal data in youth basketball. For example, apart from the Yo-Yo IR1 test, Carvalho et al. (Citation2019) reported improvements in vertical jump and agility in young female basketballers between the ages 12–14, followed by a decline in the rate of improvement until age 16. Also, te Wierike et al. (Citation2014) showed that repeated sprint ability in young male basketballers develops most rapidly from 14 to 17 years and tends to reach a plateau from 17 to 19 years. Similarly, curvilinear trajectories have been found in young soccer players in aerobic performance (Carvalho et al. Citation2014), running speed (Leyhr et al. Citation2018), as well as vertical jumping (Deprez et al. Citation2015). These findings can be explained by, at least, four factors: (1) a putative “adolescent awkwardness,” a phenomenon of disruption of motor coordination causing an apparent plateau in performance in late adolescence; (2) changes in body size, shape and composition during puberty affecting physical performance development; (3) peak spurts in several physical performance components occurring coincidently with PHV (i.e. around 14 years of age in boys) or within 6 months of its attainment (Guimarães, Maia, et al. Citation2021); and (4) ceiling effect of the testing protocols limiting the progression from a certain high-level of proficiency.
To the best of our knowledge, this is the first time that a three-level multilevel modelling approach was used to investigate the development of physical performance in youth athletes adjusted for repeated observations within (level-1) and between (level-2) players and between clubs (level-3). As shown in the variance components results, no significant relationships were found in physical performance development across clubs. This novel finding reveals that clubs did not significantly influence the development of physical performance over time, which, in other words, suggests that belonging to different clubs of the Porto Basketball Association do not impact on physical performance developmental trajectories. The limited number of clubs considered in our study may explain this result. However, it is more likely that the substantial differences in terms of characteristics, infrastructures, and human resources among the clubs (data not shown) are surpassed by similar training regimes designed by basketball coaches and strength and conditioning trainers, as well as internal policies across the twenty clubs. For example, most clubs (60%) had a gym where coaches and trainers can implement appropriate long-term athletic developmental programs aiming to enhance and improve the physical performance of young basketballers. In turn, since significant inter-individual differences were identified, physical performance development followed distinct paths, that is, their trajectories were not parallel. So, receiving the same opportunities by the clubs does not guarantee that all players respond equally to training and competition over time.
Although our findings extend the available literature in this area, this study is not without limitations. First, we acknowledge that our sample is not widely representative. Although young basketball players from the Porto Basketball Association are expected to be like those from other regions or countries, we recommend caution when generalising our results. Second, we recognise that collecting retrospective training information only based on accumulated years of formal basketball training or number of training hours per week may limit our knowledge of the athlete's sporting career. Yet, by consulting official records, we strongly believe that we were able to obtain more reliable data rather than using written or oral questioning. Third, the reduced number of clubs considered may have been responsible for the non-significant variance component. However, twenty is a number generally accepted to be included in a three-level modelling and, in our case, represented 80% of the clubs from distinct regions of the Porto city.
Conclusions
In conclusion, this longitudinal study using ontogenetic allometric models improves current understanding of physical performance development in youth basketball by showing that players with linear body size, shape, and composition, that is, with an ectomorphic dominance, tend to perform better in aerobic capacity, running speed, and lower body explosive strength. Furthermore, players advanced in biological maturation tend to be physically fitter. In contrast, training experience and training volume had no significant links with developmental trajectories in 20 m sprint and CMJ tests. In this cohort, all three physical performance components developed in a curvilinear fashion suggesting a leveling-off in late adolescence. Club characteristics had no apparent link with differences in physical performance improvements. Since high levels of physical performance are determinants to success in basketball, coaches must be aware that their rate of development is not constant when implementing training regimes. We, therefore, suggest that coaches use well-known testing procedures to measure physical performance as frequently as possible. This approach will allow them to track the developmental trajectories of players throughout their sporting careers and, consequently, better control training and competition effects. Furthermore, we recommend basketball coaches and strength and conditioning trainers recognise that leaner players have advantages in physical performance and that biological maturation positively impacts, and confounds, its development in youth. So, coaches of youth teams should complement the assessment of performance with the estimation of biological age using, for example, a simple and non-invasive tool as the maturity offset. The collection of such data will help coaches to reduce bias during selection or recruitment processes, and club directors to design and implement appropriate and sustainable long-term developmental programs. In future, researchers should consider more often the use of the allometric scaling to control for differences in body size, shape, and composition. Also, they should rely on joint allometric models with two scaling factors to estimate stature-to-body mass ratio and compare it with the RPI in order to determine an overall description of body shape or physique. Moreover, in future, researchers should include more detailed and precise data on previous and current sport engagement, as well as data from parents and coaches as active participants in the sporting environment of young athletes.
Acknowledgments
The authors thank all INEX participants – athletes, families, coaches and clubs – as well as all assessment team members: Aarón Civit, Adriano Cerdeira, Alexandra Guimarães, Américo Santos, Ana Araújo, Ana Carolina Reyes, Anemari Azevedo, António Cardoso, Átila Paiva, Bebiana Sabino, Bruno Gomes, Bruno Pinto, Carina Caneppele, Carla Santos, Carlos Ladislau, Clara Silva, Cláudia Dias, Cláudio Santos, Diana Carrito, Diogo Barbosa, Eduardo Santos, Emanuel Chagas, Estella Guedes, Esthefani Moya, Fernando Cardoso, Fernando Garbeloto, Filipa Sousa, Filipe Ferrão, Francisco Albergaria, Gabriela Noivo, Gonçalo Gonçalves, Helder Zimmermann, Ivanna Lima, Joana Cerqueira, Joana Ribeiro Soares, João Araújo, João Ribeiro, Joaquim Pires, José Filipe Santos, Luís Almeida, Maickel Padilha, Marcos André Moura, Maria do Céu Baptista, Mário Moreira, Maryam Abarghouei Nejad, Mayra Paula, Melissa Pereira, Michel Bertani, Norberto Pinto, Nuno Bompastor, Patrícia Ferreira, Pedro da Mota, Pedro Grenha, Pedro Leite, Rafael Bagatin, Rafael Silva, Ricardo Cerqueira, Rojapon Buranarugsa, Sara Ferreira, Sara Pereira, Simão Madureira, Sofia Girão, Sofia Monteiro, Teresa Dias, Teresa Ribeiro, Thayse Gomes, Tiago Fernandes, Tomás Pinto, Tomas Scheiber, and Vicenzo Rago.
Disclosure statement
No potential conflict of interest was reported by the author(s).
Additional information
Funding
References
- Ackland TR, Schreiner AB, Kerr DA. 1997. Absolute size and proportionality characteristics of World Championship female basketball players. J Sports Sci. 15(5):485–490.
- Arede J, Ferreira AP, Gonzalo-Skok O, Leite N. 2019. Maturational development as a key aspect in physiological performance and national-team selection in elite male basketball players. Int J Sports Physiol Perform. 14(7):902–910.
- Asmussen E, Heeboll-Nielsen K. 1955. A dimensional analysis of physical performance and growth in boys. J Appl Physiol. 7(6):593–603.
- Bangsbo J, Iaia FM, Krustrup P. 2008. The Yo-Yo Intermittent Recovery Test: a useful tool for evaluation of physical performance in intermittent sports. Sports Med. 38(1):37–51.
- Baxter-Jones A, Goldstein H, Helms P. 1993. The development of aerobic power in young athletes. J Appl Physiol. 75(3):1160–1167.
- Baxter-Jones ADG. 1995. Growth and development of young athletes. Sports Med. 20(2):59–64.
- Baxter-Jones ADG. 2017. Growth and maturation. In: Armstrong N, van Mechelen W, editors. Oxford textbook of children’s sport and exercise medicine. 3rd ed. Oxford: Oxford University Press; p. 13–24.
- Bergeron MF, Mountjoy M, Armstrong N, Chia M, Côté J, Emery CA, Faigenbaum A, et al. 2015. International Olympic Committee consensus statement on youth athletic development. Br J Sports Med. 49(13):843–851.
- Beunen G, Baxter-Jones AD, Mirwald RL, Thomis M, Lefevre J, Malina RM, Bailey DA. 2002. Intraindividual allometric development of aerobic power in 8- to 16-year-old boys. Med Sci Sports Exerc. 34(3):503–510.
- Beunen GP, Rogers DM, Woynarowska B, Malina RM. 1997. Longitudinal study of ontogenetic allometry of oxygen uptake in boys and girls grouped by maturity status. Ann Hum Biol. 24(1):33–43.
- Bosco C, Luhtanen P, Komi PV. 1983. A simple method for measurement of mechanical power in jumping. Eur J Appl Physiol Occup Physiol. 50(2):273–282.
- Bustamante A, Maia J, Nevill A. 2015. Identifying the ideal body size and shape characteristics associated with children’s physical performance tests in Peru. Scand J Med Sci Sports. 25(2):e155–e165.
- Carter JEL, Ackland TR, Kerr DA, Stapff AB. 2005. Somatotype and size of elite female basketball players. J Sports Sci. 23(10):1057–1063.
- Carter JEL, Heath BH. 1990. Somatotyping. Development and applications. Cambridge: Cambridge University Press.
- Carvalho HM, Bidaurrazaga-Letona I, Lekue JA, Amado M, Figueiredo AJ, Gil SM. 2014. Physical growth and changes in intermittent endurance run performance in young male Basque soccer players. Res Sports Med. 22(4):408–424.
- Carvalho HM, Coelho-e-Silva MJ, Eisenmann JC, Malina RM. 2013. Aerobic fitness, maturation, and training experience in youth basketball. Int J Sports Physiol Perform. 8(4):428–434.
- Carvalho HM, Coelho-e-Silva MJ, Gonçalves CE, Philippaerts RM, Castagna C, Malina RM. 2011. Age-related variation of anaerobic power after controlling for size and maturation in adolescent basketball players. Ann Hum Biol. 38(6):721–727.
- Carvalho HM, Gonçalves CE, Collins D, Paes RR. 2018. Growth, functional capacities and motivation for achievement and competitiveness in youth basketball: an interdisciplinary approach. J Sports Sci. 36(7):742–748.
- Carvalho HM, Gonçalves CE, Grosgeorge B, Paes RR. 2017. Validity and usefulness of the Line Drill Test for adolescent basketball players: a Bayesian multilevel analysis. Res Sports Med. 25(3):333–344.
- Carvalho HM, Leonardi TJ, Soares ALA, Paes RR, Foster C, Gonçalves CE. 2019. Longitudinal changes of functional capacities among adolescent female basketball players. Front Physiol. 10:339.
- Corlett JT. 1984. Power function analysis of physical performance by Tswana children. J Sports Sci. 2(2):131–137.
- Deprez D, Valente-Dos-Santos J, Coelho-E-Silva MJ, Lenoir M, Philippaerts R, Vaeyens R. 2015. Multilevel development models of explosive leg power in high-level soccer players. Med Sci Sports Exerc. 47(7):1408–1415.
- Eisenmann JC, Pivarnik JM, Malina RM. 2001. Scaling peak VO2 to body mass in young male and female distance runners. J Appl Physiol . 90(6):2172–2180.
- Gould SJ. 1966. Allometry and size in ontogeny and phylogeny. Biol Rev Camb Philos Soc. 41(4):587–640.
- Gryko K, Kopiczko A, Mikołajec K, Stasny P, Musalek M. 2018. Anthropometric variables and somatotype of young and professional male basketball players. Sports. 6(1):9.
- Guimarães E, Baxter-Jones A, Maia J, Fonseca P, Santos A, Santos E, Tavares F, Janeira MA. 2019. The roles of growth, maturation, physical fitness, and technical skills on selection for a portuguese under-14 years basketball team. Sports. 7(3):61.
- Guimarães E, Baxter-Jones ADG, Williams AM, Tavares F, Janeira MA, Maia J. 2021a. The role of growth, maturation and sporting environment on the development of performance and technical and tactical skills in youth basketball players: the INEX study. J Sports Sci. 39(9):979–991.
- Guimarães E, Baxter-Jones ADG, Williams AM, Tavares F, Janeira MA, Maia J. 2021b. Modelling the dynamics of change in the technical skills of young basketball players: the INEX study. PLOS One. 16(9):e0257767.
- Guimarães E, Maia JAR, Williams M, Sousa F, Santos E, Tavares F, Janeira MA, Baxter-Jones ADG. 2021. Muscular strength spurts in adolescent male basketball players: the INEX Study. IJERPH. 18(2):776.
- Guimarães E, Ramos A, Janeira MA, Baxter-Jones ADG, Maia J. 2019. How does biological maturation and training experience impact the physical and technical performance of 11–14-year-old male basketball players? Sports. 7(12):243.
- Hedeker D, Gibbons R. 2006. Longitudinal data analysis. New York (NY): Wiley.
- Kirkendall D, Gruber J, Johnson R. 1987. Measurement and evaluation for physical educators. Champaign (IL): Human Kinetics.
- Kubo A, Murata S, Abiko T, Tanaka S. 2022. The relationship between children’s somatotypes, motor examination results, and motor skills: assessing 6- to 10-year-olds. J Phys Ther Sci. 34(7):492–496.
- Leyhr D, Kelava A, Raabe J, Höner O. 2018. Longitudinal motor performance development in early adolescence and its relationship to adult success: an 8-year prospective study of highly talented soccer players. PLOS One. 13(5):e0196324.
- Malina RM, Bouchard C, Bar-Or O. 2004. Growth, maturation and physical activity. 2nd ed. Champaign (IL): Human Kinetics.
- Mirwald RL, Baxter-Jones ADG, Bailey DA, Beunen GP. 2002. An assessment of maturity from anthropometric measurements. Med Sci Sports Exerc. 34(4):689–694.
- Nevill A, Tsiotra G, Tsimeas P, Koutedakis Y. 2009. Allometric associations between body size, shape, and physical performance of Greek children. Pediatr Exerc Sci. 21(2):220–232.
- Nevill AM, Holder RL, Baxter-Jones A, Round JM, Jones DA. 1998. Modeling developmental changes in strength and aerobic power in children. J Appl Physiol. 84(3):963–970.
- Pichardo AW, Oliver JL, Harrison CB, Maulder PS, Lloyd RS. 2018. Integrating models of long-term athletic development to maximize the physical development of youth. Int J Sports Sci Coach. 13(6):1189–1199.
- Ramos S, Volossovitch A, Ferreira AP, Fragoso I, Massuça LM. 2021. Training experience and maturational, morphological, and fitness attributes as individual performance predictors in male and female under-14 Portuguese elite basketball players. J Strength Cond Res. 35(7):2025–2032.
- Ramos SA, Massuça LM, Volossovitch A, Ferreira AP, Fragoso I. 2021. Morphological and fitness attributes of young male Portuguese basketball players: normative values according to chronological age and years from peak height velocity. Front Sports Act Living. 3:629453.
- Rees T, Hardy L, Güllich A, Abernethy B, Côté J, Woodman T, Montgomery H, et al. 2016. The Great British medalists project: a review of current knowledge on the development of the world’s best sporting talent. Sports Med. 46(8):1041–1058.
- Ross WD, Marfell-Jones RJ. 1995. Cineantropometria. In: Duncan J, MacDougall H, Wenger A, Green HJ, editors. Evaluación fisiológica del deportista. Barcelona: Paidotribo.
- Silva S, Bustamante A, Nevill A, Katzmarzyk PT, Freitas D, Prista A, Maia J. 2016. An allometric modelling approach to identify the optimal body shape associated with, and differences between Brazilian and Peruvian youth motor performance. PLOS One. 11(3):e0149493.
- Snijders TAB, Bosker RJ. 2012. Multilevel analysis: an introduction to basic and advanced multilevel modeling. 2nd ed. Thousand Oaks (CA): Sage.
- StataCorp. 2021. Stata: release 17. Statistical Software. College Station (TX): StataCorp LLC.
- Suchomel A. 2002. Relation between anthropometric somatotype and motor performance in prepubescent and pubescent Czech children. Anthropologie. 40(2):193–201.
- Te Wierike SC, de Jong MC, Tromp EJ, Vuijk PJ, Lemmink KA, Malina RM, Elferink-Gemser MT, Visscher C. 2014. Development of repeated sprint ability in talented youth basketball players. J Strength Cond Res. 28(4):928–934.
- Valente-dos-Santos J, Coelho-e-Silva MJ, Duarte J, Pereira J, Rebelo-Gonçalves R, Figueiredo A, Mazzuco MA, et al. 2014. Allometric multilevel modelling of agility and dribbling speed by skeletal age and playing position in youth soccer players. Int J Sports Med. 35(9):762–771.