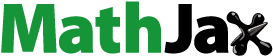
Abstract
This study addresses the group-based nature of financial vulnerability in the low-income environment. Adopting a highly flexible stochastic dissemination model, we assess the impact of insurance on the resilience of a low-income group to wealth shocks. For this purpose, the transient wealth of a group of interacting uninsured and insured agents is considered. The model is extended to capture four types of transaction events: external arrivals, internal redistributions, wealth losses and premium payments. Risk-sharing mechanisms, mitigating the impact of financial losses that are otherwise uninsured, are widespread in low-income communities. Our modelling of redistribution events captures the wealth transactions associated with these mechanisms, alongside the purchase of commodities and services from within the group. Through this set-up, we present a method for incorporating the high level of wealth interaction characteristic of the low-income setting in the assessment of the effectiveness of insurance. The model is underlined by an exogenously evolving Markov background process that represents the state of the economy. To analyse the distribution of wealth jointly with the state of the background process, a system of coupled differential equations for the joint transient distribution of agent wealth is derived, and is reduced to a linear system of differential equations through consideration of the moments of agent wealth. Sensitivity analysis is performed to establish the impact of the system's structure and stochastic dynamics on the wealth of the group. The probability of falling below the poverty line is then determined through application of a normal approximation and the impact of insurance in reducing this probability considered under varying levels of subsidisation.
1. Introduction
Given the widespread exclusion of the low-income sector from traditional financial and insurance services (Demirgüç-Kunt et al. Citation2022), low-income populations rely on alternative risk mitigation strategies. Risk-sharing is one such strategy for smoothing consumption fluctuations and mitigating the high-risk nature of the low-income environment. Gift exchange, informal credit and rotating savings associations, mutual aid and burial societies are examples of informal risk-sharing mechanisms (see, for example, Bardhan & Udry Citation1999) used to lessen exposure to the idiosyncratic risks faced by low-income households. These risks include death, accident, illness and asset loss, such as livestock and crop loss, due to, for example, fire, insect and disease, and loss of business. Support is typically provided in the form of monetary payments, labour services and food provisions, with the informal nature of these agreements characterised by the absence of a formal contract or rules and regulations.
A large development economics literature exists on the study of risk-sharing mechanisms, with many studies highlighting the prevalence of risk-sharing within small social groups rather than across entire communities. Townsend (Citation1994) and de Weerdt & Dercon (Citation2006), among others, discuss the presence of subgroups within villages or communities, such as burial societies, women's organisations, families, religious and ethnic groups, and their influence on the structure of risk-sharing in a heterogeneous society. Dependent on the risk, groups with similar (or dissimilar, when diversification of the risk is desired) features will be sought as participants in the risk-sharing agreement, where the socioeconomic characteristics often determining partnership preferences are shaped by membership of these typically overlapping subgroups. The prevalence of risk-sharing groups and their uniqueness in comparison to the well-studied high-income environment means that consideration of their financial behaviours is crucial to understanding the financial structures of low-income communities. Grimard (Citation1997), Ligon (Citation2002), Murgai et al. (Citation2002), Fafchamps & Lund (Citation2003), Dercon et al. (Citation2006), Fafchamps & La Ferrara (Citation2012), Attanasio et al. (Citation2012) and Mobarak & Rosenzweig (Citation2013) are further examples of empirical work considering risk-sharing at this more granular level.
It is often the case that agents within informal risk-sharing agreements have interchangeable roles, with recipients of capital also acting as capital providers at varying points in time (Coate & Ravallion Citation1993). However, some indigenous associations provide insurance in a more formalised manner. Focusing specifically on funeral associations, explicit rules on membership, insurance schedules and payouts are observed in a study of group-based insurance in rural areas of Ethiopia and Tanzania (Dercon et al. Citation2006). Additional coverage for risks other than those relating to funeral costs were offered by a typically low number of schemes. Consumers therefore increased their level of coverage by participating in more than one scheme. Applications for short-term credit payments (or loans) were also permitted by some groups in the event of a shock. To observe the role of social networks in the lack of insurer trust prevalent in the microinsurance environment, Chemin (Citation2018) compares health insurance uptake among members of randomly selected and pre-existing informal groups. An increased one-year retention rate is observed following promotion of insurance within existing informal groups, where no renewals occur under random selection.
In this paper, we focus on the dynamics of wealth within a low-income group such as those discussed in the aforementioned studies. Considering a system of wealth in which both transactions and losses occur, an adjustment of the stochastic dissemination model proposed by Chan & Mandjes (Citation2023) is adopted to analyse the behaviour of group wealth over time. This model is a highly general model describing the spread of wealth over a population of agents and accounts for the occurrence of two types of wealth transactions: there is external inflow of wealth, reflecting, for example, the payment of salaries from agents outside the population or government cash-transfers, as well as internal redistribution of wealth within the population, reflecting transactions such as the purchase of commodities or services from other agents within the population or risk-sharing arrangements such as gift exchange and informal credit provisions. Transaction rates are affected by an exogenously evolving Markov background process that represents the state of the economy. In the numerical analysis presented in Section 6, a two-state background process is chosen to mimic the fluctuation of the system between ‘good’ and ‘bad’ states, where a good state may represent a period of economic growth and a bad state the occurrence of a pandemic or economic recession. The background process is of particular significance in the low-income setting due to the instability of the associated markets.
Our goal in adopting this model is to study the financial vulnerability of low-income groups. For this purpose, we calculate the probability of an agent falling below the poverty line, given they are a member of such a group. To the best of our knowledge, all existing theoretical studies consider this probability on an individual agent basis. Our main contribution is that we mathematically assess the impact of group membership and the associated group interactions on this probability. Risk-sharing arrangements are useful in mitigating idiosyncratic risks, but correlated risks, such as those resulting from natural disasters, often affect agents simultaneously. Formal insurance provides more robust protection against such correlated risks. The impact of insurance on the probability of an agent falling below the poverty line is therefore also considered. To do this, we extend the model of Chan & Mandjes (Citation2023) to further encompass the susceptibility of agents to wealth losses, due to, for example, catastrophic natural disasters, severe illness and the loss of a household member or breadwinner. As in Kovacevic & Pflug (Citation2011), losses are modelled proportionally to agent wealth. This reflects both the level of exposure in the low-income environment and our definition of ‘wealth’ as the ability to produce. Climate-related events could, for example, immediately eliminate a high proportion of even a wealthy agent's productivity through loss of land and livestock. Premium payments are captured by high frequency, low severity loss events.
Previous research on the impact of microinsurance mechanisms on the probability of falling below the poverty line has largely been undertaken through application of multi-equilibrium models and dynamic stochastic programming (Ikegami et al. Citation2017, Chantarat et al. Citation2017, Carter & Janzen Citation2018, Liao et al. Citation2020, Janzen et al. Citation2021) or from a ruin-theoretic perspective (Kovacevic & Pflug Citation2011, Flores-Contró et al. Citation2022), where the probability mimics an insurer's ruin probability. Notably, these studies suggest that purchase of insurance and the associated need for premium payment increases the risk of falling below the poverty line for the most vulnerable. As such, public-private partnerships between government and private microinsurers are proposed to minimise the severity of premium payments. Government support may be provided in the form of cash transfers or, more cost-effectively, through carefully designed premium subsidies, where subsidies can be targeted such that only the most vulnerable receive support.
In a simulation-based study of a similar nature to the analysis presented in this paper, Will et al. (Citation2006) assess the impact of the availability of microinsurance on participation in risk-sharing agreements, combining an agent-based simulation model and the Watts–Strogatz small-world networks model (Watts & Strogatz Citation1998). Here, the influence on the survival of households, particularly those who cannot afford insurance, above a critical level is observed. For households that fall below the critical level, transfers are received from one randomly selected household with which they have an established connection. Due to premium payments, insured households have less capacity to provide risk-sharing support to the uninsured, reducing the resilience of the uninsured to income shocks as a consequence. Formal insurance is however found to be complementary to informal insurance in the event of covariate shocks, aligning with previous findings in the literature (Mobarak & Rosenzweig Citation2012, Dercon et al. Citation2014). In contrast to the model proposed in this paper, interactions associated with transfer of wealth within the local economy are not captured in the model of Will et al. (Citation2006). Risk-sharing refers only to the provision of monetary support in the event of a wealth shock.
To analyse the transfer of wealth in the system, as in Chan & Mandjes (Citation2023), we derive a system of coupled differential equations for the transient distribution of agent wealth, jointly with the state of the Markov background process. Here, the concept of wealth reflects the ability of an agent to produce and so encompasses land, property, livestock, physical and human capital, with health a form of capital in extreme cases where sufficient health services and food accessibility are not guaranteed (Dasgupta Citation1997). Derivation of the moments of the wealth process enables numerical analysis through reduction of the system to a system of linear differential equations. Sensitivity analysis is undertaken to provide insight into the impact of the structure of the system and its stochastic dynamics on the wealth of the group. More specifically, a system with one leader and two internally homogeneous groups of uninsured and insured agents is considered, where the leader may be the facilitator of the insurance scheme, an informal employer or the leader of a societal group. Mean and variance wealth processes are presented to compare the vulnerability of those with and without insurance coverage, and in line with Flores-Contró et al. (Citation2022), the impact of subsidies provided equally to all insured agents and targeted subsidies provided to only a subset of insured agents are considered. By the central limit theorem, we use a normal approximation to determine the probability of falling below the poverty line and the distribution of the number of agents that fall, where the parameters of the distribution are combinations of the stationary means and reduced second moments of the wealth process.
The fundamental concepts underlying this stochastic dissemination model follow from a large literature on queuing network and population models. Fiems et al. (Citation2018) implement a similar approach in the queuing context, with randomly occurring linear transformations of the population vector of the network aligning with the internal redistribution events of this model. Transformations are multiplicative as in the case presented here. For thorough discussion of stochastic networks with application to queuing and population models, see Kelly (Citation2011) and Serfozo (Citation2012). Markov modulated models are also found in the risk theory literature. Examples of Markov modulated risk models can be seen in Asmussen (Citation1989) and Schmidli (Citation2017), among others.
The proposed model can easily be adjusted to account for large groups or economic entities, if, for example, risk-sharing among cities or countries is of interest. Risk-sharing studies at the international level include those by Devereux & Smith (Citation1994), Sørensen & Yosha (Citation2017) and Gardberg (Citation2019) and largely appear in the economics literature. Considering risk-sharing in the form of risk pooling, Ni et al. (Citation2020) discuss the pooling of flood risk exposure at the continental and global levels. Although interesting, in this analysis, the focus remains on the subgroups prevalent in low-income communities.
The peer-to-peer (P2P) risk-sharing structures studied in Abdikerimova & Feng (Citation2021) in the context of insurance and mutual aid, and in Feng et al. (Citation2022) with specific application to flood risk, provide a formal representation of the informal risk-sharing arrangements prevalent in low-income communities. Formal P2P insurance requires specification of risk-sharing rules that determine how each member of the pool contributes to payment of a loss. Denuit & Dhaene (Citation2012) propose a conditional mean risk-sharing rule that is suitable in the P2P setting (Denuit Citation2019, Citation2020). This rule has been applied by Denuit & Robert (Citation2020) for large losses, Denuit & Robert (Citation2021a, Citation2021b, Citation2021c) for large groups and Denuit & Robert (Citation2022) and Denuit et al. (Citation2022) for correlated losses. The latter provides detailed discussion of desirable properties of risk-sharing rules. P2P models should be considered when designing formal insurance products for the interacting low-income groups central to this paper.
The remainder of the paper is structured as follows. Section 2 presents the stochastic dissemination model and derivation of the system of coupled differential equations for the joint distribution of agent wealth. Time-dependent first and second moments are derived in Sections 4 and 5, respectively. Sensitivity analysis is presented in Section 6 and the normal approximation of the probability of falling below the poverty line in Section 7. Concluding remarks are provided in Section 8.
2. Wealth dissemination model
In this paper, the stochastic behaviour of is studied under varying levels of insurance coverage, where
is the wealth of an agent i at time t for
. Agent wealth takes only discrete values and is regarded as being composed of
wealth units. As in Chan & Mandjes (Citation2023), the dynamics of the wealth model are affected by an autonomously evolving continuous-time Markov background process
, which is irreducible and has state space
for
.
Over time, the background process moves between states according to the transition rate matrix , where
(1)
(1) See, for example, Norris (Citation1997) for a thorough presentation of continuous-time Markov chains.
Changes in an agent's wealth are triggered by the occurrence of four types of transaction events. Namely, external arrivals, internal redistributions, catastrophic losses and premium payments. Given the background process is in state , the joint probability generating function must therefore capture the following components, where events (1) and (2) are defined as in Chan & Mandjes (Citation2023):
All agents in receipt of an external arrival of wealth of type j, i.e. all agents
, experience an increase of one wealth unit, where
for
. External arrivals occur at exponentially distributed times with rate
At internal shock times, transfers of wealth occur such that every wealth unit of agent i transfers
wealth units to agent j, where
is randomly distributed. As such, the number of wealth units at agent j after an internal shock at time t>0 is
(2)
(2) where
is the wealth vector immediately before the shock and
a sequence of independent and identically distributed random variables. Note that, redistribution events do not pose a risk of negative wealth as proportional wealth transfers ensure that an agent can only give away a maximum of their current number of wealth units.
Each
shares the same distribution as
. The random variables
for
and
are independent, however, dependence in j is permitted. Agents therefore redistribute their wealth independently of one another but with dependence between the transactions made by a single agent. Wealth is redistributed within the population at exponentially distributed times with rate
, such that transaction events affect the entire system simultaneously. Randomness in the occurrence of transaction events and in the size of redistributions reflects the uncertainties associated with informal work, on both the supplier and consumer sides, and with the experience of idiosyncratic losses and the ensuing risk transfers provided by community members.
For
where
, the associated probability generating functions are defined by
(3)
(3)
Remark 2.1
The number of wealth units transferred by each wealth unit is assumed to be Bernoulli distributed. Under this specification, each wealth unit can transfer at most one wealth unit to any other agent. If transfers were instead assumed to be binomially distributed with parameter n>1, the possibility of wealth creation would be captured, where wealth may be created through, for example, investment or the adoption of productive technologies.
Definition of redistribution events that affect only a subset of agents is also possible through specification of multiple transaction rate parameters. This would enable inclusion of differing transaction behaviours, allowing, for example, informal work-based wealth interactions and risk-sharing mechanisms to be modelled separately.
(3) | A loss forces a proportion of an affected agent's wealth to leave the system. For a loss of type By construction, the model permits the occurrence of losses with varying frequency and severity through specifcation of the binomial probability and exponential rate parameters. This is particularly useful in the microinsurance setting, where coverage for commonly occurring events, including hospital admissions and household deaths, is needed in addition to low frequency, high severity events such as natural disasters. For | ||||
(4) | In an analogous manner to loss events, for a premium payment of type Note that, For |
3. Derivation of the system of differential equations for the joint probability generating function
To analyse the transient wealth of the system and its behaviour as the Markov background process
evolves, the multivariate time-dependent joint probability generating function (PGF) of
is used. This PGF defines the joint distribution of
and is given by
(8)
(8) for
with
.
In the remainder of this section, a system of coupled differential equations for the time-dependent joint PGF in (Equation8(8)
(8) ) is established. Considering an interval of length
, the system is defined through observation of the change in the wealth vector
or the background state, and the associated change in
induced by each event that could occur between times t and
. The contribution of each event to the system is as follows, where the background process, external arrival and internal redistribution components are as in Chan & Mandjes (Citation2023):
Background process change. The background process transitions from state l to state
between times t and
, where the process is in state l at time t:
(9)
(9)
External arrival. An external arrival of type j occurs, such that the wealth of all agents
increases by one wealth unit:
(10)
(10)
Internal redistribution. An internal shock triggers the redistribution of the wealth of all agents within the system. Let
denote the occurrence of an internal redistribution shock between times t and
when the background process is in state k. Then, by the law of total expectation:
(11)
(11)
(12)
(12)
(13)
(13)
(14)
(14) where
.
Loss. A loss causes each wealth unit of all agents
to decrease by
(0 or 1), independently of one another. In a similar manner to that of the internal shock case, by the law of total expectation:
(15)
(15)
(16)
(16)
(17)
(17) where
denotes the occurrence of a loss event in the interval
.
Premium payment. A premium payment causes each wealth unit of all agents
to decrease by
(0 or 1), independently of one another. Since premium payments are modelled analogously to uninsured losses, the associated term is derived in the same way:
(18)
(18)
No event. There are no transitions of the background process, external arrivals, internal shocks, losses or premium payments between times t and
.
As such,
(19)
(19) The system of differential equations for the joint PGF is then obtained by subtracting
from both sides of (Equation19
(19)
(19) ), dividing by
and taking the limit as
, such that
(20)
(20) using the fact that the row sums of Q are 0. The following proposition therefore holds:
Proposition 3.1
For ,
satisfies the system of differential equations in (Equation20
(20)
(20) ), with initial condition
(21)
(21) where
and
.
The internal redistribution component (Equation14(14)
(14) ) causes the system to be non-linear due to the
argument. While it is possible to solve (Equation20
(20)
(20) ) numerically, to capture the full distribution of
, for the remainder of the paper, our focus is on the time-dependent first, reduced and mixed second moments of transient wealth. By reducing the system to a system of linear differential equations, this enables tractable analysis of the evolution of wealth over time and for analytical expressions for the moments of transient wealth to be obtained.
4. Derivation of first moments of transient wealth
In this section, the proof of Proposition 4.1 derives the first moments of transient wealth for the highly generalised system of Section 3. One special case relating to the study of insurance as a means for reducing the financial vulnerability of a group is then presented in Section 4.1, where full insurance coverage is considered.
Proposition 4.1
Let and
. For
, the transient mean wealth of the system
satisfies a system of dI coupled, non-homogeneous linear differential equations of the following form:
(22)
(22) with initial condition
(23)
(23) where
and
.
Proof.
Taking the derivative of (Equation20(20)
(20) ) with respect to
and evaluating at
yields
(24)
(24) where
and use of the chain rule permits computation of the derivative of
:
(25)
(25) for
, where
(26)
(26) Define vectors
and
such that
and
for each
. Let
(27)
(27) and
, where
and
is analogously defined. Let
and
where
and
. Then, in matrix-vector form,
satisfies (Equation22
(22)
(22) ), as required. The initial condition in (Equation23
(23)
(23) ) follows trivially by definition of
.
The vector of transient state probabilities of the background process satisfies
(28)
(28) where ⊗ is the Kronecker product and
the I-dimensional identity matrix, such that for
, it holds that
(29)
(29) Solving for
in (Equation22
(22)
(22) ) for
then gives
(30)
(30) as in Proposition 4.2 of Chan & Mandjes (Citation2023).
The probability of falling below the poverty line in Section 7 is considered in infinite time. As such, the normal approximation relies upon the steady-state mean and variance of agent wealth. Let be the unique solution of
, such that it is the stationary distribution of
. Then, if the Markov chain
is stable,
and so the steady-state mean vector
satisfies
(31)
(31) The formal stability condition is stated and proved in Fiems et al. (Citation2018) and Chan & Mandjes (Citation2023) as follows:
Proposition 4.2
Let ω be the eigenvalue of A in (Equation31(31)
(31) ) with the largest real part, defining the spectral abscissa of A. Then, the Markov chain
is ergodic if
.
Proof.
The ergodicity proofs appearing in Fiems et al. (Citation2018) and Chan & Mandjes (Citation2023), for related models, can be adapted in a straightforward way to our model.
The dissemination model can be adjusted to fit many constructions of a population simply through varying the selection of parameters. In the following subsection, we present a special case that addresses the wealth behaviours of populations aligning with the type of low-income group that we are interested in. Section 4.1 presents the interacting transient mean wealth dynamics for an uninsured group and a group with full insurance. This case assumes the presence of a ‘leader’, who only receives wealth from outside of the system.
4.1. Special case: full or no coverage
Consider a population that consists of a single distinct agent (‘leader’) and two internally homogeneous interacting subpopulations (‘followers’), one insured and one uninsured. Focusing on the impact of insurance on the financial vulnerability of the group, with the exception of their insurance coverage, the two follower groups are assumed to be homogeneous. The insured subpopulation consists of agents and the uninsured subpopulation
agents. The indices u and p are used throughout the remainder of the paper to reflect the uninsured group and those making premium payments in return for full insurance coverage, respectively.
Let ,
and
denote the mean wealth of the leader, an arbitrary uninsured agent and an arbitrary insured agent, respectively, at time t, with background process in state k. Due to the homogeneity within the follower groups, mean wealth is assumed to be equal across all agents in each group. Let J = I,
and
such that there are I,
and
types of external arrivals, losses and premium payments, respectively, and let
,
and
, where
and
, such that any event type affects a single agent and that the sets of agents affected by losses and premium payments are mutually exclusive. For the purpose of this example, agents affected by each type of loss are assumed to be independent of the background state. As such, we denote
and
. Since only the insurance coverage status differs between the two groups of followers, let
denote the rate of external arrival experienced by the uninsured and insured subpopulations with background process in state k. Equivalently, let the leader's external arrival rate be denoted
.
Within subpopulations, the random variable describing the internal redistribution of wealth now only depends on the state of the background process k. Therefore, let
,
,
,
,
,
,
,
and
denote the expected values of all possible internal transactions (per wealth unit) within the population, when the background process is in state k.
Since the two follower groups are internally homogeneous, all agents within each group must experience loss and premium payment events in the same way. As such, the rate and probability of loss parameters corresponding to the event experience of each agent are fixed within the two subpopulations. Fixing the sets of affected agents S such that , where all agents are affected by each event, would also facilitate the homogeneous assumption. Let
and
denote the loss rate and probability of loss (per wealth unit) associated with all types of loss
for uninsured followers with background process in state k. Similarly, let
and
denote the premium payment rate and probability of payment (per wealth unit) associated with all premiums
for insured followers with background process in state k.
Under this special case, the system in Proposition 4.1 reduces to the following:
(32)
(32)
(33)
(33)
(34)
(34) such that
for
(35)
(35) where each
and
for
is a d-dimensional vector,
(36)
(36) where
(37)
(37) and
for
, and
(38)
(38)
Remark 4.1
Expressions required to analyse an agent's mean wealth can also be obtained when increasing the heterogeneity in the population in an analogous manner. The resulting differential equations simply become more complex due to an increased number of terms.
For heterogeneous event experience, multiple agents could still be affected by the same loss (or premium payment) through the specification of and
assumed in the special case considered. This would encompass the potential for covariate losses due to, for example, natural disasters, in addition to individually experienced losses. However,
and
(respectively
and
) would need to coincide for all affected.
Now, consider the system presented in this special case with background process reflecting the state of the economy. As such, let k = 1, 2, where the process fluctuates been good (k = 1) and bad (k = 2) economic states, representing economic growth and recession, respectively. In this setting, the leader could, for example, be the facilitator of the insurance scheme, an informal employer or the leader of a societal group. The leader's wealth is obtained from outside of the system, while followers obtain their wealth from the leader. External arrival parameters are therefore defined as follows:
(39)
(39) Here,
, such that the leader receives additional income at a higher rate during periods of economic growth.
Due to the binomial specification of wealth unit transfer at redistribution events, for each agent, transfer of wealth to the system is multinomially distributed with parameters corresponding to the vectors , for
and k = 1, 2. Let the redistribution of leader wealth be distributed with parameters 1 and
, and redistribution of follower wealth be distributed with parameters 1 and
, where
and
. Here, follower wealth is distributed evenly throughout the follower group. The multinomial distribution could also be specified such that it captures variation in the proportion of wealth saved by a follower and the proportion consumed. This is considered in the sensitivity analysis of Section 6.
Remark 4.2
Specifying or
would enable the possibility that wealth leaves the system at transaction events, with multinomial probabilities
and
, respectively.
Although, in reality, it is likely that insurance covers only a proportion of experienced losses, in this example, the uninsured and insured groups are assumed to be mutually exclusive. As such, the uninsured group experiences losses in full with no premium payments and the insured group makes premium payments with no loss experience. The associated net profit condition is therefore
(40)
(40) One application of a wealth system of this structure would be to a pension or funeral insurance scheme, where each agent represents a household. In such a case, the insured group could be considered as a group of households containing at least one individual who makes contributions to the scheme, who therefore do not experience financial loss on the death of a household member. Retired workers without a pension, non-workers or households without a funeral insurance contract would then constitute the uninsured group. Although in this paper, external transactions other than those to the leader are not considered, pension payments or insurance payouts could be captured by the receipt of external arrivals of wealth by uninsured agents. Alternatively, the system could represent insurance provided through employment benefits, where the working are covered and the non-working are not, or simply the case where the uninsured choose not to insure.
The system of differential equations under this two-state Markov background process is as follows:
(41)
(41)
(42)
(42)
(43)
(43) Then, where
is stable and the stationary distribution of
exists, the steady-state mean wealth of the leader and the uninsured and insured followers in background states 1 and 2 are given by
(44)
(44) and
(45)
(45) where
(46)
(46) for
and
.
5. Derivation of reduced and mixed second moments of transient wealth
The reduced and mixed second moments of transient wealth are now derived under the special case of Section 4.1. This result extends to that of the whole system.
Denote the reduced second moments of and the mixed second moments of
and
by
(47)
(47) and
(48)
(48) respectively. Then, Proposition 5.1 holds.
Proposition 5.1
Let and
. For
, the transient reduced and mixed second moments of the wealth of the system
satisfies a system of
coupled, non-homogeneous linear differential equations of the following form:
(49)
(49) with initial conditions
(50)
(50) and
(51)
(51) where
and
.
Proof.
Taking the derivative of (Equation20(20)
(20) ) with respect to
and
and evaluating at
yields
(52)
(52) where
and the second derivative of
is obtained by:
(53)
(53)
(54)
(54) for
. For
,
(55)
(55) and for
(56)
(56) However, since the binomial parameter n = 1 for all
in the case considered here,
for all j, i and
.
For ease of presentation, consider a population consisting of leaders,
uninsured followers and
insured followers, as in Section 4.1. Define the matrix
(57)
(57) where
(58)
(58) for n = 1, 2, 3, and
(59)
(59)
(60)
(60)
(61)
(61) Let
(62)
(62) and
(63)
(63) such that
(64)
(64) where
denotes the 8-dimensional identity matrix. Finally, for
(65)
(65) and
(66)
(66) define
(67)
(67) Then, in matrix-vector form,
satisfies (Equation49
(49)
(49) ), as required, where
(68)
(68) and each
is a d-dimensional vector. The initial conditions (Equation50
(50)
(50) ) and (Equation51
(51)
(51) ) follow by definition of
and
.
An equivalent system for a general population of ungrouped agents can be obtained. In this case, matrices and
are of much higher dimension and so are not presented here. Also note that the definitions in (62) and (Equation66
(66)
(66) ) allow for consideration of an additional interacting group of proportionally insured agents, experiencing both losses and premium payments, through an adjustment of the indicator functions. In this case, the severity of losses and premium payments would change, however their frequencies would remain the same.
Numerical solutions for the first and second moments of transient wealth, derived from the systems of differential equations in Sections 4 and 5, are presented in Figure alongside the 95% confidence intervals (CIs) of estimates obtained via 500 simulations of the wealth process. The special case selected for the comparison corresponds to that of Figure (a).
Figure 1. (a) Mean and (b) variance of uninsured and insured agent wealth for with the corresponding 95% CIs. Process simulated for calculation of CIs for N = 500 realisations, with time-step dt = 0.01 and terminal time T = 30.

In the following section, sensitivity analysis on the mean and variance of agent wealth in a system aligning with the special case of Section 4.1 is presented.
6. Sensitivity analysis
In this section, we perform sensitivity analysis to assess the impact of changes in the system's stochastic dynamics on the wealth distribution. Experiments are performed on the impact of the rate of external arrivals, losses and premium payments. We additionally consider how changes in the characteristics of the interacting subpopulations influence the wealth dynamics, specifically focusing on initial wealth, group size and retainment levels. Since the leader is assumed to be an agent that distributes external wealth to follower agents and thus may not be a member of a low-income group, we present only the wealth dynamics of the follower subpopulations.
The aim in carrying out these experiments is to provide insight into how insurance affects the wealth of agents in a low-income group such as those discussed in Section 1. We further aim to highlight the flexibility of the model, and thus its usefulness in undertaking such analysis, where simple parameter respecifications allow for the testing of varying scenarios.
Throughout the analysis, the loss proportion is fixed at
, the expected value of a beta distribution with parameters
and
. The premium proportion
required to cover losses of this size in full, such that the insured group does not experience any loss of wealth at loss events, is specified under the expected value principle. As such,
(69)
(69) where θ is the loading factor set by the insurer and
is the proportion of losses that are covered.
In Figures , initial state (), transition rate (
, redistribution rate (
), loss frequency (
), loss proportion (
), premium frequency (
), loading factor (
), insurance coverage (
) and leader retention rate (
) parameters remain fixed, unless otherwise specified. Loss and premium rate parameters are also fixed across good (state 1) and bad (state 2) economic states. Discussion of the experiments is split into three sections:
Figure 2. Mean and variance of uninsured and insured agent wealth for with (a) 50, (b) 25 and (c) 2 agents in each follower group.

Group size and initial wealth
Given the level of interaction between the follower groups, wealth levels are likely to be dependent on group size. Observing the wealth behaviours of different sized groups is therefore useful to determine the group size that increases the resilience of follower wealth to income shocks and to gain insight on how wealth is redistributed within the population. This information could help to inform the design of group insurance products and, if shared at the local level, informal risk-sharing mechanisms.
Figure presents the mean and variance of transient wealth for three sizes of follower group. Assessing initial wealth as a determinant of wealth behaviours, Figure compares three wealth levels, where the impact of increased leader wealth is observed in Figure (c).
Figure 3. Mean and variance of uninsured and insured agent wealth for with (a)
, (b)
, (c)
and
, where
.

Figures and consider homogeneous preference for wealth retention at redistribution events across uninsured and insured agents. In each case, differences in the mean and variance of wealth between the two groups are negligible. Rates of loss and premium payment are approximately 0.091 and 0.14, respectively. This therefore suggests that for equal wealth retention levels, the excess cost of premium payment is distributed throughout the group at transaction events, such that its impact on the wealth of insured followers is unobservable. Differences between the mean wealth of the two groups become slightly more apparent once a retention rate of 0.9 is exceeded, with both groups still retaining the same proportion of wealth. As we increase from 0.9 through the highest levels of retention, the wealth of the uninsured marginally increases above that of the insured. The increase in vulnerability associated with premium payment requirements observed in the existing literature (Kovacevic & Pflug Citation2011, Janzen et al. Citation2021, Flores-Contró et al. Citation2022) is therefore only apparent for extremely high levels of retention in this particular group setting.
Interestingly, mean wealth increases over time in small interacting groups (Figure (c)) but decreases over time in larger groups (Figure (a,b)). This finding aligns with the high rate of wealth redistribution per agent, with each agent distributing approximately 90% of their wealth, evenly across all other agents, at transaction events. Thus, the greater the number of agents, the lower the contribution from each agent to every other agent. Such a high level of dissemination reflects the limited facility for savings in the low-income setting. Variability of wealth in the system is however much greater when the sample size is small.
As expected, increasing the initial wealth of agents increases the mean wealth in the system, with the impact more significant at the beginning of the observation (Figure (a,b)). However, while previous studies observe decreasing vulnerability with increasing wealth, for high levels of wealth redistribution, increasing initial wealth has little impact on long-term wealth behaviours. Increased variation appears among the wealth of agents with greater initial wealth, but as in Figure (a,b), this variation decreases over time. This infers that the even redistribution of wealth by each follower agent to all other followers prevents the long-term presence of any agents of particularly high or low wealth in the system.
Subsidisation and wealth retainment
As discussed in Section 1, existing research suggests that purchase of insurance without subsidies by agents closest to the poverty line increases their probability of falling below this critical level. Reducing the cost of insurance premiums through subsidisation is one way in which governments provide support. We therefore consider the impact of premium subsidisation on the wealth of a group-based system. Figures , , and present the mean and variance of uninsured and insured agent wealth for (a) unsubsidised insurance, (b) insurance with a 50% subsidy and (c) insurance with a 100% subsidy, where subsidies are provided homogeneously across all insured agents.
Figure 4. Mean and variance of uninsured and insured agent wealth for with subsidisation of (a) 0%, (b) 50% and (c) 100% of the premium for all insured agents.

Figure 5. Mean and variance of uninsured and insured agent wealth for with subsidisation of (a) 0%, (b) 50% and (c) 100% of the premium for all insured agents.

Figure 6. Mean and variance of uninsured and insured agent wealth for and subsidisation barrier (a) B = 2, (b) B = 5 and (c) B = 10. Process simulated for N = 500 realisations, with time-step dt = 0.01 and terminal time T = 30.

Figure 7. Mean and variance of uninsured and insured agent wealth for with subsidisation of (a) 0%, (b) 50% and (c) 100% of the premium for all insured agents.

Figure 8. Mean and variance of uninsured and insured agent wealth for with subsidisation of (a) 0%, (b) 50% and (c) 100% of the premium for all insured agents.

Computations for derivation of the first, reduced and mixed second moments of transient wealth in Sections 4 and 5 are analogous when accounting for premium subsidies provided uniformly to all agents in this manner, with only a change in parameter required. Insurance premiums are specified as for Figures and , with subsidies applied through a reduction in the premium payment probability by either 50% (case (b)) or 100% (case (c)).
In this way, subsidies may be considered to be random and are proportional to agent wealth. Structuring subsidy provision in this way captures the uncertainty typically associated with provision of financial support and social security in the low-income economies relevant to the microinsurance and risk-sharing context. Random subsidies could also reflect the prevalence of informal, pay-as-you-go coverage in the low-income setting, due to the variability in income levels associated with high rates of informal sector work. Intuitively, premium subsidies increase agent wealth in all cases considered.
If financially feasible, uninsured agents may choose to save a proportion of their wealth to protect against future losses rather than purchasing insurance, see, for example, Gollier (Citation2003) and Miranda & Farrin (Citation2012), while agents with limited capacity for savings likely consume all of their wealth. In the subsequent experiments, we assume insured agents do not retain a more significant portion of wealth for themselves at redistribution events, given they are fully covered by insurance. In Figures and , the impact of the proportion of wealth retained by uninsured agents at transaction events is tested, with all other parameters fixed, through observation of the change in population wealth under two scenarios: with and without uninsured savings.
A wealth retainment parameter of causes an agent to distribute their wealth evenly across all agents. Although in reality, wealth redistribution may not be uniform throughout a population, when considering a group of low-income agents with similar socioeconomic backgrounds, such an assumption aligns with the prevalence of risk-sharing, high levels of consumption and limited capacity for savings and is therefore not so out of place. Note that, altering the probability of an agent retaining wealth at transaction events requires an adjustment of the transient first, reduced and mixed second moment differential equations corresponding to the special case of Section 4.1. This adjustment is not presented here. Mean and variance are again equivalent for uninsured and insured agents with the same retainment preference in all subsidisation cases (Figure ). For an increased uninsured retainment parameter (Figure ), mean uninsured wealth lies above that of the insured, with variance increasing with increasing wealth.
Since uninsured and insured agent wealth coincide under homogeneous retainment parameters, the increased wealth observed in Figure (a) among the uninsured, where the premium is paid by the insured in full, is accountable to the increased level of savings. The reduction in the redistribution of uninsured wealth lessens the mitigation of excess premium payment costs at redistribution events. Insured wealth therefore decreases with increasing uninsured retention, such that their vulnerability to premium payment events increases. The greater the disparity in the proportion of retained wealth, the greater the difference in the mean wealth of the two follower groups. An equivalent finding is observed, in reverse, if the retention rate of the insured is assumed to be higher than that of the uninsured. This case could be used to reflect findings such as those in Will et al. (Citation2006), where, due to the need for premium payments, the insured contribute less to the uninsured. In such an experiment, the insured may use their retained wealth to make their premium payments.
The sharing nature of group-based wealth systems is further exemplified by the increase in mean wealth among uninsured agents when subsidies are provided to the insured. In an interacting group, insurance subsidies therefore also increase the resilience of uninsured agents to wealth shocks.
Consideration of the cost of social protection is important when designing sustainable government subsidisation schemes. Figure presents the mean and variance of transient wealth within the two follower groups under a ‘smart’ subsidy scheme (Hill et al. Citation2014) aligning with the barrier strategy of Flores-Contró et al. (Citation2022). Premium subsidies are provided only to those with wealth below a critical level B. Case (a), for barrier B = 2, coincides with the optimal barrier derived in Flores-Contró et al. (Citation2022). Note that the decrease in wealth observed in Figures – appears with less severity under the barrier scheme, while the variance has little deviation, decreasing gradually throughout the observation period. This suggests that the severity of catastrophic loss and premium payment events is mitigated by the subsidisation barrier. The high probability of falling below the poverty line for those closest to its critical level is eliminated since agents experience neither losses or premium payments when below the barrier under full insurance coverage. This may also account for the increased variance in the wealth distribution under the barrier scheme in comparison to fixed subsidisation. Adjusting the retainment level as in Figures and yields analogous results in regard to the disparities between uninsured and insured wealth, with uninsured wealth increasing with increasing retention.
External arrivals and loss of wealth
The decrease in wealth observed in the previous experiments aligns with the low level of external arrivals of wealth to the system. Wealth is added to the system only through external transactions experienced by the leader. These transactions induce an increase in leader wealth by a single wealth unit, negligible when spread evenly across the follower groups. As such, mean wealth decreases in line with greater rates of loss and premium payment experienced by all other agents in the system.
The rate of external arrivals may vary depending on the type of entity the leader represents. Increasing the rate of external arrivals helps to identify how increased external support, whether from governmental organisations or employers, influences the wealth behaviours of the group. Comparing the results with those of Figure , we see that increasing the rate of external arrivals (Figure ) significantly decreases the rate of wealth decay in the case of unsubsidised insurance. This finding supports exploration of capacity for increasing external inputs of wealth to a low-income system. For fully subsidised insurance, despite the continued experience of uninsured losses and the largely even redistribution of wealth, the system's wealth increases.
It is also important to consider the dependence of wealth behaviours on the rate of loss. Loss experience may vary between low-income communities. Performing sensitivity analysis on this parameter therefore helps to highlight how agents respond to different types of losses. For , an uninsured agent loses, on average, 50% of their wealth at each loss event. At such a high rate, the increase in wealth arrivals experienced by the leader in Figure is ineffective in enabling growth in the wealth of follower groups (Figure ). Even with such high rates of loss, insurance premiums providing full insurance coverage decrease the wealth of the insured below that of the uninsured group when retainment parameters are inhomogeneous.
7. The trapping probability
As discussed in Section 1, the aim of this paper is to determine the impact of insurance on the financial vulnerability of low-income individuals through analysis of the trapping probability, where the term ‘trapping’ refers to the event at which an agent falls into an area of poverty from which it is difficult to escape without external help. In order to assess this measure with the stochastic dissemination model presented here, as in Chan & Mandjes (Citation2023), a normal approximation is used to estimate the probability of agent wealth falling below a given critical level. Aligning with the poverty trapping context, see, for example, Azariadis & Stachurski (Citation2005) and Bowles et al. (Citation2006), this critical level represents the critical level of wealth below which an agent would struggle to meet their basic needs, i.e. the poverty line.
Let ψ denote the probability that the stationary wealth of an arbitrary follower lies below the critical wealth level . Given the group-based nature of the dissemination model, under consideration of a sufficiently large number of agents, a standard normal approximation may be used to estimate this probability. As such, let the trapping probability of an arbitrary uninsured follower be given by
(70)
(70) where
denotes the stationary wealth of follower j for
. The mean and reduced second moments of stationary wealth are given by
(71)
(71) where
.
Although the trapping probabilities of a homogeneous group of agents are identically distributed, due to the reliance of agent wealth on the shared background process, they are not independent. As such, in order to analyse trapping within the full uninsured follower group, define the following random variable:
(72)
(72) where
. Then, for
and
, let
(73)
(73) and let
(74)
(74) be its normal approximation, for bivariate normal
with mean
and covariance matrix
(75)
(75) where
.
In this analysis, the approximation presented by Cox & Wermuth (Citation1991) for the distribution of bivariate normal random variables , with zero means, unit variances and correlation coefficient ρ, is adopted for estimation of the joint trapping probability in (Equation74
(74)
(74) ). This approximation is applicable since
in all examples considered.
The mean and variance of the number of trapped agents are derived by definition of B, where and
(76)
(76)
(77)
(77) Then,
(78)
(78) Applying a normal approximation with
and
, it holds that for
,
(79)
(79)
Remark 7.1
The trapping probability for an insured follower is defined analogously for , with subscripts p replacing u. The condition for application of the approximation of the distribution of bivariate normal random variables is again satisfied.
Figures and present density plots for the number of trapped uninsured and insured agents for three levels of subsidisation, with wealth systems as in Figures and . A large sample of size 2001 is selected to increase the accuracy of the normal approximation. The sample constitutes 1 leader, and 1000 followers in each of the two groups. Initial wealth () and the rate of external arrivals (
) remain fixed, with all other parameters as stated at the beginning of Section 6. Trapping probabilities calculated by (Equation70
(70)
(70) ) are presented at the top of each plot.
Figure 9. Distribution of number of trapped for and
, with subsidisation of (a) 0%, (b) 50% and (c) 100% of the premium for all insured agents.

Figure 10. Distribution of number of trapped for and
, with subsidisation of (a) 0%, (b) 50% and (c) 100% of the premium for all insured agents.

In line with Flores-Contró et al. (Citation2022), trapping probabilities consistently decrease with increasing subsidy. However, we additionally observe a decrease in the trapping probabilities of the uninsured. This again suggests that subsidisation positively impacts uninsured wealth and thus their resilience to wealth shocks. Trapping probabilities and the corresponding count distributions are identical when retention rates are equal (Figure ). When the uninsured agent retains a greater proportion of wealth, the trapping probability of the insured group is consistently higher. As such, if agents can afford to save and thus limit their level of wealth redistribution, insurance increases the vulnerability of the insured, as observed in Section 6. However, in the long-term, the distribution of the number of uninsured agents that become trapped remains unchanged.
In the experiments of this paper, wealth behaviours do not change with the insured status of an agent. Under the alternative assumption that insured agents reduce their redistribution rate due to liquidity constraints associated with the need for premium payment, the increase in trapping probability may be counteracted. When uninsured and insured agents have the same retention preferences, both groups have equivalent vulnerability.
Based on the steady-state mean and variance of agent wealth, trapping probabilities are high in comparison to the infinite-time trapping probabilities of Kovacevic & Pflug (Citation2011) and Flores-Contró et al. (Citation2022), for equivalent initial wealth. However, this could be accountable to our consideration of exclusively full, or no insurance coverage, rather than due to the impact of the group structure. Previous research on this measure largely considers a single agent (household) with proportional insurance coverage. The impact of wealth losses is therefore reduced in comparison to when experienced collectively by the uninsured, and thus, through redistribution events, all agents, in this group setting.
Full insurance coverage is also considered in Will et al. (Citation2006). In this study, two insured agent behaviours are compared. One in which insured agents continue to provide risk-sharing support to the uninsured, aligning with the experiments undertaken in this paper, and one in which they do not. In contrast to the findings of the sensitivity analysis presented here, relative to idiosyncratic shocks, formal insurance is observed to negatively impact the probability of the wealth of an uninsured agent falling below a given critical level. However, in this alternative study, risk-sharing support is provided by only a small number (4) of interacting agents and thus is limited in its capacity. In addition, due to the homogeneous assumption of initial wealth across the two groups, our analysis assumes that insured agents can, in fact, afford insurance, with their purchase of coverage drawing their wealth down to that of the uninsured. Therefore, while insured wealth falls below that of the uninsured as a result of premium payments, as also noted in Will et al. (Citation2006), provision of risk-sharing support remains affordable in our case. For covariate shocks, insurance benefits the uninsured as observed in the analysis of this paper.
8. Concluding remarks
This paper extends a highly generalised stochastic dissemination model and presents application of the model to wealth dissemination in a low-income group. The extended model captures the effectiveness of insurance in reducing the financial vulnerability of low-income agents and allows for analysis of their associated resilience to wealth shocks. Existing research largely considers this problem on an individual agent basis. The model additionally addresses the effect of risk-sharing behaviours, which are of particular prevalence in low-income communities, on the impact of insurance. Apart from the simulation study of Will et al. (Citation2006), to the best of our knowledge, this feature of the low-income environment and its influence on the impact of insurance is otherwise unstudied.
Participation in risk-sharing mechanisms and their prevalence across the societal groups of low-income communities influences many components of the agent decision to insure. These components, which include insurer trust and financial education levels, must be understood in order to increase microinsurance penetration and thus financial inclusion in the low-income market. Our proposition of a highly flexible modelling framework is therefore an important contribution to the literature on this topic.
Observations in the sensitivity analysis presented in Section 6 bring to light the possibility that within the group structure, the financial impact of losses and premium payments are, in fact, largely shared among agents, thus reducing their vulnerability to the associated shocks. This aligns with the findings of Will et al. (Citation2006). Although this observation is dependent on the level of sharing within a group, when considering a group of agents of the same socioeconomic background in the low-income setting, the assumption of even (or close to even) redistribution of wealth is not such a strict assumption. The limited capacity for savings also increases the likelihood of agents retaining only a small proportion of their wealth.
Subsidisation of insurance premiums benefits both insured and uninsured agents. This provides further evidence for the benefit of government-insurer partnerships and a key takeaway, in that insurance mechanisms have the potential to benefit an entire community even when only part of that community is insured. Insured agents in receipt of premium subsidies have greater access to wealth at redistribution event times, thus increasing the wealth of all agents. However, a reduction in uninsured sharing due to increased wealth retention drives insured wealth below that of the uninsured, with less support available at redistribution events to mitigate the risk of premium payments, even when subsidised. It is noted in the literature that receipt of government cash-transfers and purchase of insurance can change the supporting behaviours of low-income individuals. As such, future research will involve testing the impact of alternative behaviours determined by the insurance and social protection status of agents.
In addition to studying transient agent wealth behaviours, we calculate the probability of an agent falling below the poverty line and mathematically assess the impact of group membership and the associated group interactions on this probability. Although differing for idiosyncratic shocks, our findings align with those of Will et al. (Citation2006) for covariate losses. Differences in the size of risk-sharing groups and in the level of risk-sharing contribute to the disparity in the idiosyncratic results. Only wealth interactions that model the provision of support in the event of a loss are considered in Will et al. (Citation2006). For insured agents, trapping probabilities decrease with increasing subsidisation as expected, but are high in comparison to the results of Flores-Contró et al. (Citation2022) and Kovacevic & Pflug (Citation2011). The assumption of full or no coverage likely contributes to this observation. Future work will assess the impact of considering proportionally insured agents, susceptible to losses and premium payments, on the observations of this paper. Notably however, the trapping probabilities of uninsured agents also decrease with increasing subsidisation.
While the selected model yields intuitive results, it is important to highlight its limitations. Premium payments are required to be proportional to agent wealth. In reality, this is an unlikely feature of insurance, requiring premiums to be consistently reviewed over the course of the insured period. However, premium payments that increase with increasing wealth align with the proportional structure of losses, with those of greater wealth susceptible to higher losses and thus encountering higher premium payments. On the other hand, adjusting classical risk theory models, such as those considered in Kovacevic & Pflug (Citation2011) and Flores-Contró et al. (Citation2022), to capture randomly occurring interactions between multiple capital processes is non-trivial. The flexibility of the stochastic dissemination model of this paper facilitates re-specification of the population structure and wealth transaction events through simple parameter adjustments, enabling analysis of an unbounded number of scenarios.
Disclosure statement
No potential conflict of interest was reported by the author(s).
Additional information
Funding
References
- Abdikerimova, S. & Feng, R. (2021). Peer-to-peer multi-risk insurance and mutual aid. European Journal of Operational Research 299(2), 735–749.
- Asmussen, S. (1989). Risk theory in a Markovian environment. Scandinavian Actuarial Journal 1989(2), 69–100.
- Attanasio, O., Barr, A., Cardenas, J. C., Genicot, G. & Meghir, C. (2012). Risk pooling, risk preferences, and social networks. American Economic Journal: Applied Economics 4(2), 134–167.
- Azariadis, C. & Stachurski, J. (2005). Poverty traps. In Handbook of economic growth. Elsevier. P. 295–384. North Holland.
- Bardhan, P. & Udry, C. (1999). Development microeconomics. Oxford University Press.
- Bowles, S., Durlauf, S. & Hoff, K. (2006). Poverty traps. Russell Sage Foundation New York.
- Carter, M. R. & Janzen, S. A. (2018). Social protection in the face of climate change: targeting principles and financing mechanisms. Environment and Development Economics 23(3), 369–389.
- Chan, K. & Mandjes, M. (2023). A versatile stochastic dissemination model. Methodology and Computing in Applied Probability 25(3), 1–25.
- Chantarat, S., Mude, A., Barrett, C. & Turvey, C. (2017). Welfare impacts of index insurance in the presence of a poverty trap. World Development 94, 119–138.
- Chemin, M. (2018). Informal groups and health insurance take-up evidence from a field experiment. World Development 101, 54–72.
- Coate, S. & Ravallion, M. (1993). Reciprocity without commitment: characterization and performance of informal insurance arrangements. Journal of Development Economics 40(1), 1–24.
- Cox, D. R. & Wermuth, N. (1991). A simple approximation for bivariate and trivariate normal integrals. International Statistical Review 59, 263–269.
- Dasgupta, P. (1997). Nutritional status, the capacity for work, and poverty traps. Journal of Econometrics 77(1), 5–37.
- Demirgüç-Kunt, A., Klapper, L., Singer, D. & Ansart, S. (2022). The global findex database 2021: financial inclusion, digital payments, and resilience in the age of COVID-19. World Bank: Washington, DC.
- Denuit, M. (2019). Size-biased transform and conditional mean risk sharing, with application to P2P insurance and tontines. ASTIN Bulletin: The Journal of the IAA 49(3), 591–617.
- Denuit, M. (2020). Investing in your own and peers' risks: the simple analytics of P2P insurance. European Actuarial Journal 10(2), 335–359.
- Denuit, M. & Dhaene, J. (2012). Convex order and comonotonic conditional mean risk sharing. Insurance: Mathematics and Economics 51(2), 265–270.
- Denuit, M., Dhaene, J. & Robert, C. Y. (2022). Risk-sharing rules and their properties, with applications to peer-to-peer insurance. Journal of Risk and Insurance 89(3), 615–667.
- Denuit, M. & Robert, C. Y. (2020). Large-loss behavior of conditional mean risk sharing. ASTIN Bulletin: The Journal of the IAA 50(3), 1093–1122.
- Denuit, M. & Robert, C. Y. (2021a). From risk sharing to pure premium for a large number of heterogeneous losses. Insurance: Mathematics and Economics 96, 116–126.
- Denuit, M. & Robert, C. Y. (2021b). Stop-loss protection for a large P2P insurance pool. Insurance: Mathematics and Economics 100, 210–233.
- Denuit, M. & Robert, C. Y. (2021c). Corrigendum and addendum to “From risk sharing to pure premium for a large number of heterogeneous losses”. Insurance: Mathematics and Economics 101, 640–644.
- Denuit, M. & Robert, C. Y. (2022). Conditional tail expectation decomposition and conditional mean risk sharing for dependent and conditionally independent losses. Methodology and Computing in Applied Probability 24, 1953–1985.
- Dercon, S., de Weerdt, J., Bold, T. & Pankhurst, A. (2006). Group-based funeral insurance in Ethiopia and Tanzania. World Development 34(4), 685–703.
- Dercon, S., Hill, R. V., Clarke, D., Outes-Leon, I. & Taffesse, A. S. (2014). Offering rainfall insurance to informal insurance groups: evidence from a field experiment in Ethiopia. Journal of Development Economics 106, 132–143.
- Devereux, M. B. & Smith, G. W. (1994). International risk sharing and economic growth. International Economic Review 35(3), 535–550.
- de Weerdt, J. & Dercon, S. (2006). Risk-sharing networks and insurance against illness. Journal of Development Economics 81(2), 337–356.
- Fafchamps, M. & La Ferrara, E. (2012). Self-help groups and mutual assistance: evidence from urban Kenya. Economic Development and Cultural Change 60(4), 707–733.
- Fafchamps, M. & Lund, S. (2003). Risk-sharing networks in rural Philippines. Journal of Development Economics 71(2), 261–287.
- Feng, R., Liu, C. & Taylor, S. (2022). Peer-to-peer risk sharing with an application to flood risk pooling. Annals of Operations Research 321(1-2), 813–842.
- Fiems, D., Mandjes, M. & Patch, B. (2018). Networks of infinite-server queues with multiplicative transitions. Performance Evaluation 123-124, 35–49.
- Flores-Contró, J. M., Henshaw, K., Loke, S. H., Arnold, S. & Constantinescu, C. (2022). Subsidising inclusive insurance to reduce poverty. arXiv preprint arXiv:2103.17255.
- Gardberg, M. (2019). Determinants of international consumption risk sharing in developing countries. IFN Working Paper No. 1261.
- Gollier, C. (2003). To insure or not to insure?: an insurance puzzle. The Geneva Papers on Risk and Insurance Theory 28, 5–24.
- Grimard, F. (1997). Household consumption smoothing through ethnic ties: evidence from Cote d'Ivoire. Journal of Development Economics 53(2), 391–422.
- Hill, R. V., Gajate-Garrido, G., Phily, C. & Dalal, A. (2014). Using subsidies for inclusive insurance: lessons from agriculture and health. Microinsurance Paper No.29. Geneva, Switzerland: International Labour Organization (ILO).
- Ikegami, M., Carter, M. R., Barrett, C. B. & Janzen, S. (2017). Poverty traps and the social protection paradox. In The economics of poverty traps. University of Chicago Press. P. 223–256. Chicago.
- Janzen, S., Carter, M. R. & Ikegami, M. (2021). Can insurance alter poverty dynamics and reduce the cost of social protection in developing countries? Journal of Risk and Insurance 88(2), 293–324.
- Kelly, F. P. (2011). Reversibility and stochastic networks. Cambridge University Press.
- Kovacevic, R. M. & Pflug, G. (2011). Does insurance help to escape the poverty trap? - a ruin theoretic approach. Journal of Risk and Insurance 78(4), 1003–1028.
- Liao, P., Zhou, X. & Fan, Q. (2020). Does agricultural insurance help farmers escape the poverty trap? research based on multiple equilibrium models. The Geneva Papers on Risk and Insurance - Issues and Practice 45(1), 203–223.
- Ligon, E. (2002). Targeting and informal insurance. Discussion Paper 2002/008. The United Nations University World Institute for Development Economics Research (UNU-WIDER).
- Miranda, A. M. & Farrin, K. (2012). Index insurance for developing countries. Applied Economic Perspectives and Policy 34(3), 391–427.
- Mobarak, A. M. & Rosenzweig, M. R. (2012). Selling formal insurance to the informally insured. Yale Economics Department Working Paper No. 97, Yale University Economic Growth Center Discussion Paper No. 1007.
- Mobarak, A. M. & Rosenzweig, M. R. (2013). Informal risk sharing, index insurance, and risk taking in developing countries. American Economic Review 103(3), 375–380.
- Murgai, R., Winters, P., Sadoulet, E. & de Janvry, A. (2002). Localized and incomplete mutual insurance. Journal of Development Economics 67(2), 245–274.
- Ni, W., Henshaw, K., Zhu, W., Wang, J., Hu, M. & Constantinescu, C. (2020). On flood risk management across socio-economic environments. Revista Anales del Instituto de Actuarios Españoles 4a(26).
- Norris, J. R. (1997). Markov chains. Cambridge University Press.
- Schmidli, H. (2017). The Markov modulated risk model. In Risk theory. Springer. P. 183–195. Switzerland.
- Serfozo, R. (2012). Introduction to stochastic networks 44. Springer.
- Sørensen, B. E. & Yosha, O. (2017). International risk sharing and European monetary unification. Journal of International Economics 45(2), 211–238.
- Townsend, R. M. (1994). Risk and insurance in village India. Econometrica: Journal of the Econometric Society 62, 539–591.
- Watts, D. J. & Strogatz, S. H. (1998). Collective dynamics of ‘small-world’ networks. Nature 393(6684), 440–442.
- Will, M., Groeneveld, J., Frank, K. & Müller, B. (2006). Informal risk-sharing between smallholders may be threatened by formal insurance: lessons from a stylized agent-based model. PLoS ONE 16(3), e0248757.