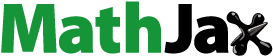
Abstract
Airborne light detection and ranging (LiDAR) data are increasingly used to inform sustainable forest management practices. Information about species composition is needed for a range of applications; however, commonly used area-based summaries of LiDAR data are limited to accurately differentiate tree species. The objective of this study was to map dominant species groups across a large (>580,000 ha) boreal forest by combining area-based and individual tree metrics derived from single photon LiDAR data with multispectral information derived from Sentinel-2 imagery. Classification of the forest into jack pine (Pinus banksiana), black spruce (Picea mariana), mixed conifer, mixedwood, and hardwood species groups resulted in an overall accuracy of 67.8% compared with field data. A more generalized classification into softwood, hardwood, and mixedwood had an overall accuracy of 83.2%. The reflectance in the red edge region of the electromagnetic spectrum (λ = 740 nm), the area and volume of tree crowns, and the cumulative distribution of LiDAR returns in the canopy were particularly important variables to discriminate between species groups. Wall-to-wall predictions of species groups based on remotely sensed data—as derived herein—could provide a spatially-detailed alternative to stand-level species composition information traditionally derived from photo-interpreted aerial imagery.
RÉSUMÉ
Les données de balayage laser aéroporté (LiDAR) sont de plus en plus utilisées pour soutenir la gestion durable des forêts. Connaître la composition des espèces d’arbres est nécessaire pour de multiples applications; cependant, des limites existent concernant l’utilisation de mesures régionales de données LiDAR pour différencier les espèces de manière précise. L’objectif de cette étude est de cartographier les espèces d’arbres dominantes à travers une grande (>580,000 ha) forêt boréale en combinant à la fois des mesures LiDAR régionales et à l’échelle de l’arbre avec des images satellites multispectrales prises par Sentinel-2. La classification de la forêt en pin gris (Pinus banksiana), épinette noire (Picea mariana), conifères mixtes, forêt mixte, et feuillus mixtes a résulté en une précision de 67.8% en comparaison avec des données de terrain. Une précision de 83.2% a également été obtenue avec une classification plus générale soit conifères, forêt mixte et feuillus. La réflectance dans la région du spectre électromagnétique correspondant au point d’inflexion du rouge (λ = 740 nm), la superficie et le volume des couronnes d’arbres et la distribution cumulative des points LiDAR dans les parties supérieures de la canopée étaient des variables particulièrement importantes pour différencier les espèces. La cartographie des espèces dominantes à partir de données de télédétection—telles qu’elles sont dérivées ici—pourrait être une alternative aux estimations à l’échelle du peuplement issues traditionnellement de la photo-interprétation d’imagerie aérienne.
Introduction
Sustainable operational forest management requires detailed spatial information about forest composition and structure (Wulder et al. Citation2008). In particular, information related to species composition is needed to assess species-specific growing stocks and yield (e.g., Maltamo and Packalen Citation2014; Tompalski et al. Citation2018) and monitor tree species richness (Gamfeldt et al. Citation2013). Traditionally, assessment of species composition involved manual photo-interpretation of aerial imagery, from which stands of homogeneous forest type can be delineated, and field information obtained from plots sampled across the landscape (Leckie and Gillis Citation1995). However, the accuracy of these photo-interpreted species compositions is rarely assessed (Tompalski et al. Citation2021), and when they are, have been found to have a relatively low level of accuracy (54%–56% in Pinto et al. Citation2007, ∼36% in Thompson et al. Citation2007). Moreover, the accuracy of photo-interpretation depends on the complexity of the forest environment, as well as the experience of the photo-interpreter, the latter of which are increasingly rare. For these reasons, there has been a growing interest in assessing species composition using remotely sensed data. However, to date, remotely sensed applications have been unable to replicate the detailed species compositions associated with photo-interpreted forest inventories, and scaling up species mapping approaches across large-areas remains a significant challenge (Fassnacht et al. Citation2016).
Technologies, such as light detection and ranging (LiDAR) data acquired from airborne platforms, also referred to as airborne laser scanning (ALS), provide a 3-dimensional point cloud representation of the landscape from which information about forest structure can be extracted. As a result, the past decade has seen the widespread adoption of ALS information into the forestry inventory cycle to improve the precision and accuracy of forest resources inventory (White et al. Citation2016; Coops et al. Citation2021). To date, the most common approach to utilize ALS in a forest inventory is with an area-based approach (ABA), which consists of developing predictive models of forest attributes obtained from ground plot information using plot-level LiDAR summary metrics derived from the vertical distribution of the point cloud as predictor variables (Næsset Citation2002; White et al. Citation2013; Wulder et al. Citation2014). While ALS provides robust area-based estimates of forest attributes, such as Lorey’s height, basal area, stem volume, and above-ground biomass, which are strongly related to the structural information provided by the point cloud, other attributes, such as species composition are more challenging to derive accurately using summary metrics of LiDAR point cloud heights (Fassnacht et al. Citation2016).
Species-related traits, such as the pigments, water content and internal structure of leaves, branching, foliage density, and crown shape influence the distribution and intensity of returns in ALS point clouds and the spectral response measured by optical sensors, particularly in parts of the visible (λ = 400 nm–700 nm), near-infrared (λ = 700 nm–1300 nm) and shortwave infrared (λ = 1300 nm–2500 nm) regions of the electromagnetic spectrum (Fassnacht et al. Citation2016). Research examining the prediction of tree species with ALS data has largely focused on the individual tree level by using individual tree detection (ITD) and individual tree crown (ITC) segmentation algorithms from which tree-specific crown shape and density metrics can be derived (Holmgren and Persson Citation2004; Michałowska and Rapiński Citation2021). Nevertheless, structural information alone generally has a lower potential than spectral information to classify tree species (Fassnacht et al. Citation2016). Spectral information is often integrated by combining ALS data and high-resolution multispectral or hyperspectral imagery (Holmgren and Persson Citation2004; Dalponte et al. Citation2014; Holmgren et al. Citation2008; Coops et al. Citation2004) or by using novel multispectral ALS sensors which emit laser pulses at different wavelengths (Axelsson et al. Citation2018; Budei et al. Citation2018; Mikko Kukkonen et al. Citation2019; Prieur et al. Citation2021). As tree-level approaches are prone to bias due to issues with segmentation (Kaartinen et al. Citation2012), approaches that combine ABA and ITC, known as semi-ITC, have also been explored (Breidenbach et al. Citation2010). In areas where species-specific attribute estimates are required for the inventory (e.g., Finland), non-parametric supervised learning methods, such as k-nearest neighbors algorithms (k-NN) are commonly used for area-based estimates (e.g., Lindberg et al. Citation2013; Maltamo et al. Citation2021).
Area-based or stand-level predictions of species information have been achieved using spectral data from medium resolution multispectral satellite imagery, such as Sentinel-2 or Landsat exclusively (Astola et al. Citation2019; Grabska et al. Citation2019; Persson et al. Citation2018; Breidenbach et al. Citation2021; Hemmerling et al. Citation2021) but the integration of these data with ALS in an area-based context has been less explored (Fassnacht et al. Citation2016). Therefore, the objectives of this research were: (i) to produce area-based predictions of dominant species groups using a combination of standard LiDAR height metrics, aggregated metrics derived from ABA and ITC methods, and Sentinel-2 imagery across a very large (>580,000 ha) boreal forest; (ii) to explore and assess the ability of these three sets of metrics to differentiate between species groups; and (iii) to discuss implications of using this approach for large-area operational forest management at the stand level.
Data and methods
Study area
The study area was the Romeo Malette Forest, located in Ontario, Canada (48.48° N, 81.33° W). The Romeo Malette Forest consists of 630,000 ha, of which 582,430 ha is forested land. It is part of the Boreal Shield Ecozone of Canada (). The area is characterized by long cold winters and short warm summers with monthly average daily temperature ranging between −16.8 °C and 17.5 °C and monthly precipitation ranging between 41.3 mm and 90.9 mm (1981–2010 normals). The terrain is dominated by relatively flat to moderately rolling topography (280 m–450 m above sea level) and dominant tree species include black spruce (Picea mariana), jack pine (Pinus banksiana), white spruce (Picea glauca), trembling aspen (Populus tremuloides) and paper birch (Betula papyrifera).
Figure 1. Map of the study area (A) and location within the province of Ontario and the Canadian boreal shield ecozone (B).

The Romeo Malette Forest is actively managed for timber production and fiber procurement. Management activities are guided by Ontario’s Crown Forest Sustainability Act and related policies, and are based on a 10-year forest management plan, which includes a detailed description and mapping of logging and renewal operations and the development of planned road access. The current plan covers the years 2019–2029 and management activities are reported or projected based on forest units defined from tree species composition groupings (Parton et al. Citation2006). Annual work schedules identify the location of operations scheduled for implementation during each year and provide information on the areas scheduled for harvest, road construction/maintenance, renewal, tending, and protection operations. The Romeo Malette Forest has been certified under the National Boreal Standard of the Forest Stewardship Council (FSC) since 2006.
Field data
A set of 244 circular sample plots (400 m2; radius = 11.28 m), selected following a structurally guided sampling approach driven by ALS metrics were sampled in 2018 (Queinnec et al. Citation2022). Plot center coordinates were obtained with the EOS Arrow 100 GPS receiver by acquiring a minimum of 600 points per plot center and the use of differential post-processing to achieve sub-meter position accuracy. The height, species, and diameter at the breast height (DBH) of each tree with DBH ≥ 7.1 cm located within the plot boundary were recorded at each plot. The vertical stratification of tree crowns was also assessed by the field crews to identify suppressed trees or trees located within or above the dominant canopy layer. Additionally, 53 of the plots were stem mapped and the distance and azimuth from the plot center to each stem (DBH ≥ 7.1 cm) located in the plot boundary were recorded using an azimuth and range finder instrument (TruPulse® 360). For leaning trees, the distance and azimuth between the stem and the vertical projection of the crown top on the ground were also measured. Trees with a crown located inside the plot boundary but with a stem outside were also mapped. The cartesian coordinates of tree tops were determined from the polar coordinates (distance and azimuth of tree tops) measured from the plot center.
For each ground plot, the trees located within—or above—the dominant canopy layer were used to derive dominant species groups as described in . Two functional groupings were established. The first was more general and grouped plots according to the dominance of forest types: softwood, mixedwood, and hardwood dominated groups. The second functional grouping distinguished the dominance of the two most common commercial tree species: black spruce and jack pine. Plots were assigned to five functional groups: black spruce-dominated, jack pine-dominated, mixed conifer, mixedwood, and hardwood. Similar species groupings were used in previous forest inventories at the Romeo Malette Forest (Murray Woods et al. Citation2011; Queinnec et al. Citation2022). The capacity of the developed approach using Sentinel-2 and ALS data to accurately predict each of these groupings was assessed.
Table 1. Stratification of ground plots into 3 or 5 functional groups based on the species of the trees positioned in—or above—the dominant canopy layer.
LiDAR data and pre-processing
Wall-to-wall ALS data were collected across the Romeo Malette Forest in June and July 2018 with the Leica SPL100 as part of an acquisition across the forest management units of Ontario (Bilyk et al. Citation2021). The SPL100 is a single-photon LiDAR instrument that emits laser pulses in the green part of the electromagnetic spectrum (λ = 532 nm). Each beam transmitted by the laser is split into a 10 × 10 array of beamlets and the energy returned from each beamlet is recorded with an array of cells that are activated by the arrival of single photons. This differs from conventional discrete-return ALS systems where detector cells are activated when the amount of energy is above a detection threshold (Harding Citation2018). The SPL100 can be operated at high altitude (typically 2000 m–4000 m above ground level) with a high frequency of low-energy pulses, which gives them an operational advantage over conventional ALS systems when high-density point clouds (typically 30 points/m2–50 points/m2) are required for large ALS acquisition programs (Yu et al. Citation2020). Previous studies conducted in boreal or mixedwood forests have demonstrated that the SPL100 provided area-based forest attribute estimates with an accuracy comparable to what can be expected from conventional linear-mode LiDAR systems (Queinnec et al. Citation2022; White et al. Citation2021; Yu et al. Citation2020; Wästlund et al. Citation2018).
For the Romeo Malette Forest acquisition, the SPL100 was flown onboard an aircraft at an average altitude of 3800 m and a flying speed of 90 m/s for an average swath width of 2000 m on the ground. The point cloud was geolocated, denoised, and classified into ground and vegetation returns by the data provider according to the approach outlined in Gluckman (Citation2016). This resulted in an average point cloud density of 40.8 points/m2 across the study area.
The point cloud was normalized to heights above ground using a triangular irregular network of the ground returns. A 25-cm resolution canopy height model (CHM) was generated from the normalized point cloud using the highest return within the grid of each cell. To densify the point cloud and reduce the number of pits, each return was considered as a disk of 30 cm diameter, which corresponds to the actual footprint of each beamlet on the ground (beam divergence of 0.08 mrad and altitude of 3800 m above ground). The CHM was then smoothed using a Gaussian filter (σ = 1) on a 5 × 5 pixel moving window.
LiDAR metrics
Two sets of LiDAR summary metrics were calculated from the normalized ALS point cloud and derived CHM for each of the 244 ground plots and across the entire study area at a resolution of 20 × 20 m matching the ground plot area of 400 m2 (). The lidR package (Roussel et al. Citation2020) implemented in R (R Core Team Citation2022) was used to generate the ALS metrics. The first set of metrics included standard area-based metrics calculated from the height distribution of the LiDAR returns, as well as the rumple index, which characterizes the surface roughness of the canopy. We also calculated the signal attenuation depth (SAD) of each return (Véga et al. Citation2016; Irwin et al. Citation2021) which indicates how far a return travels within the canopy. SAD is calculated for each return as the difference between the CHM and the return height and normalized to the CHM. SAD = 0 indicates a return on the outer canopy layer while SAD = 1 indicates a return on the ground. SAD was calculated only for returns where CHM > 2 m.
Table 2. Description of the predictor variables derived from the ALS data and Sentinel-2 imagery.
The second set of metrics was derived by summarizing the distribution of ITD and ITC metrics at the plot-level or 20 × 20 m pixel-level using the sum, median, and standard deviation of values (). The ITD was performed using the local maximum filter algorithm implemented in lidR (Popescu and Wynne Citation2004; Roussel et al. Citation2020). The algorithm uses a circular moving window to detect local maxima in the CHM and a size parameter controls the diameter of the window. We varied the size parameter between 0.5 m and 5 m at 0.5 m intervals to assess the influence of the window on the ITD performance window shapes. The individual tree tops detected by the ITD algorithm were then used as seed points in the marker-controlled watershed segmentation algorithm implemented in the R package imager to detect ITCs. The marker-controlled watershed algorithm was selected as it is parameter-free, not designed for particular crown shapes, and computationally efficient, which are important considerations for large areas with varying species composition, such as the Romeo Malette Forest. The algorithm inverts the CHM and sets the detected tree tops as local minima. A region is then propagated around each tree top by filling the inverted CHM. To avoid over-propagation, we limited the region-growing algorithm to the pixels of the CHM that were not below 1 meter of the detected tree top height. That way, we expected trees with a conical crown shape to grow in smaller regions than trees with a flatter and broader crown.
Sentinel-2 imagery
We obtained 44 Sentinel-2A and Sentinel-2B level-1C (top-of-atmosphere reflectance) scenes acquired across the RMF between June 1 and September 30, 2018 and processed them to level-2A (bottom-of-atmosphere reflectance) using the R package sen2r (Ranghetti et al. Citation2020). This R package streamlines the downloading and pre-processing of Sentinel-2 imagery using the atmospheric correction algorithm Sen2Cor (Main-Knorn et al. Citation2017). All pixels classified as missing values, shadows, clouds, or cirrus by the level-2A scene classification map were masked out and scenes were projected and resampled to align with the 20 × 20 m SPL100 metrics grid using nearest neighbor interpolation. The Sentinel-2 bands used in this study had a spatial resolution of either 10 × 10 m or 20 × 20 m so downscaling to the resolution of the gridded ALS metrics was not required. A cloud-free composite product was then produced by averaging the pixel values across all available cloud-free observations for each pixel. Spectral bands listed in were selected as predictor variables for species group classification.
Classification of dominant species groups
Random forest models were used to classify the dominant species groups () using the area-based, ITC and Sentinel-2 predictors (). The conditional random forest algorithm implemented in the function cforest of the party package in R (Strobl et al. Citation2007; Citation2008; Hothorn et al. Citation2006) was selected due to its ability to compute an unbiased measure of variable importance (see below). Predictor variables with a pairwise Pearson correlation coefficient >0.9 were identified and from each pair of variables, only the one with the lowest average correlation among the other predictor variables was retained. We also performed different scenario runs, as described in , to assess the influence of different sets of predictor variables on the accuracy of the species group classification.
Table 3. Description of the predictor variables included in the different scenario runs.
Accuracy assessment of the ITD and area-based species classification
The performance of the ITD was assessed using the 53 stem-mapped plots. Detected trees were automatically matched to their nearest field-mapped trees based on the 3-dimensional distance between their tree tops. Only pairs of detected and mapped trees with a horizontal distance ≤5 m and height difference ≤3 m were considered as valid matches, or true positives (TP). Since the ITD algorithm only detects trees in the upper canopy layer, only field trees that were in or above the dominant canopy layer and that were not matched to any detected tree top were considered as false negatives (FN). All remaining detected tree tops not matched to any field tree were considered as false positives (FP). The accuracy of the ITD algorithm was assessed for each set of parameters by calculating the recall (EquationEquation 1(1)
(1) ), precision (EquationEquation 2
(2)
(2) ), and F-score (EquationEquation 3
(3)
(3) ). Recall, or producer’s accuracy, indicates the proportion of correctly detected trees out of all trees mapped on the field, whereas precision (user’s accuracy) indicates the proportion of correctly detected trees out of all trees detected by the ITD algorithm. The F-score combines recall and precision measures by calculating their harmonic mean, which penalizes more low or unbalanced recall and precision values than the arithmetic or geometric means.
(1)
(1)
(2)
(2)
(3)
(3)
The accuracy of the random forest classification models was assessed using repeated k-fold cross-validation (k = 10; repeated 5 times). Due to the imbalance of species groups in the observations, the training folds were randomly sampled so that all species groups had the same number of observations as the least frequent species group. The testing folds remained unchanged. The accuracy of the models was assessed using overall accuracy (OA; EquationEquation 4(4)
(4) ), recall (EquationEquation 1
(1)
(1) ), precision (EquationEquation 2
(2)
(2) ), and F-score (EquationEquation 3
(3)
(3) ) from the predictions of the repeated folds. The OA is a global measure of accuracy that indicates the proportion of observations that were correctly classified across all species groups whereas recall, precision, and F-score are calculated for each species group.
(4)
(4)
The cross-validation was also used to optimize the parameter mtry that controls the number of predictor variables that are randomly selected to split the data in the nodes of each tree. The mtry value of the model with the lowest overall accuracy across the folds was used in the final model for both the 3-group and 5-group classification.
Assessing the importance of predictor variables
The importance of the predictor variables to predict the species groups was assessed using the conditional variable importance measure from the conditional random forest model (Strobl et al. Citation2008). Variable importance in the random forest is assessed by examining the effect of breaking the relationship between a predictor variable and the response, by permuting the predictor variable values, on the prediction accuracy (measured as OA). The difference between prediction OA before and after permutation of a predictor variable values is used as a measure of variable importance. Strobl et al. (Citation2007) showed that when predictor variables are of different nature or vary in their scale of measurement, which is the case herein, the permutation scheme used in random forest tends to be biased toward certain variables. The conditional variable importance used herein is derived from a novel conditional permutation scheme, which enables to derive unbiased variable importance (Strobl et al. Citation2008). Conditional importance measures were linearly scaled between 0 (least important variable) and 100 (most important variable).
Random forest variable importance provides a measure of the overall contribution of each predictor variable to the species groups classification model but does not assess if some variables are more useful to discriminate between specific species groups than others. Therefore, we performed a pairwise comparison of the predictor variable values between species groups to gain insight into why some pairs of species groups were more or less separable. For each of the 15 most important predictor variables, we used the Kruskal-Wallis test, a non-parametric equivalent to a one-way analysis of variance, to test the null hypothesis that the mean rank of the variable is equal across the species groups. When a significant difference (p-value < 0.05) was observed across the species group, we used the post-hoc Dunn’s test with Holm’s p-value adjustment method to examine pairwise differences. That way, we were able to examine if some variables had more predictive power for some species groups compared to others.
Comparing predicted species group to existing photo-interpreted inventory
We compared the estimates of species groups derived herein to stand-level species information available from the most up-to-date photo-interpreted forest resources inventory information available at the Romeo Malette Forest which was initially established with manual photo-interpretation of multispectral aerial imagery acquired in 2005. About 20% of the stands initially established were updated using new aerial photographs or field-based surveys regularly until 2014 to account for harvesting, natural depletion, silvicultural treatments, regeneration, or changes in stand development stages. The objective of this comparison was to explore the classification results across a broader geographic extent than the cross-validation at the plot-level provided, and at the stand-level which is the management unit used for decision making. However, we did not consider the photo-interpreted data as a reference to assess the accuracy of our classification since their accuracy is poorly defined (Pinto et al. Citation2007; Thompson et al. Citation2007).
We selected 43,806 productive forest stands (median area of 5.6 ha) with SPL100 estimates of canopy height over 5 m (zq90 > 5 m) and over 50% canopy cover (pzabove2 > 50%) to remove stands recently harvested or disturbed. In each stand, an estimate of overstory species composition as well as the percentage of crown covers each tree species occupies was available from the photo-interpreted inventory, and this information was used to classify each stand into the five functional species groups listed in . In each stand, we calculated the most frequently predicted species group and compared it to the species group derived from the photo-interpreted inventory.
Results
Individual tree detection and crown segmentation
The accuracy of the ITD varied depending on the diameter of the local maxima filter window and species groups. The highest median F-score was observed for a circular window diameter of 1.5 m for black spruce dominated (Fmedian = 0.82) and mixed conifer (Fmedian = 0.82), 2 m for mixedwood (Fmedian = 0.72), 2.5 m for jack pine dominated (Fmedian = 0.85), and 3 m for hardwood (Fmedian = 0.75) species groups (). In all species groups combined, the highest median F-score of 0.72 was obtained for window diameters of 2 m, 2.5 m, and 3 m, and the window diameter of 2 m was retained to apply the ITD algorithm across the Romeo Malette Forest. Median values of recall, precision, and F-score for each species group and window diameter are presented in Supplementary Table 1.
Classification of species groups
The classification of ground plots into the three functional species groups softwood, mixedwood, and hardwood resulted in an overall accuracy ranging between 65.98% for the model including Sentinel-2 bands only, and 83.20% for the model including all predictor variables (). When including all predictor variables, the softwood species groups had a precision and recall of 92.13% and 91.75% (F-score = 91.94%), and the hardwood species group had a precision and recall of 90.58% and 82.63% (F-score = 86.42%), respectively. The accuracy of the classification was poorer for the mixedwood species group with a precision of 45.62% and a recall of 60.00% (F-score = 51.83%). The confusion matrix is presented in and illustrated in and recall, precision, and F-score for each species group and model scenario are included in Supplementary Figures 1 and 2.
Figure 3. Comparison of the overall accuracy of the random forest classification model of the ground plots into softwood, mixedwood, and hardwood species groups (3 Species Groups) or jack pine dominated, black spruce dominated, mixed conifer, mixedwood, and hardwood species groups (5 Species Groups).

Figure 4. Chord diagram visualizing the confusion matrix of the random forest species classification model into (A) softwood, mixedwood, and hardwood species group; or (B) jack pine dominated, black spruce dominated, mixed conifer, mixedwood, and hardwood species groups for the scenario run that included all predictor variables. Predictions from all cross-validation folds were aggregated and are included in this diagram. The predicted species groups are shown in the bottom half and the reference species groups are shown in the upper half. The predicted species groups are shown in the bottom half and the reference species groups are shown in the upper half. Links illustrate the number of observations that were correctly classified (link between same predicted and reference species groups) and the number of classification errors (link originating from a predicted species group going to a different reference species group).

Table 4. Confusion matrix showing the results of the broader, 3-group classification model using all predictor sets. Diagonal bold values indicate the number of observations for which the predicted species group correspond to the reference species group.
When dividing the softwood species group into jack pine dominated, black spruce dominated, and mixed conifer groups, the overall accuracy of the model ranged between 46.22% for the model including Sentinel-2 bands only and 67.89% for the model including all the predictor variables (). The hardwood species group remained the most accurate with a precision of 87.34% and a recall of 87.19% (F-score = 87.27%). Jack pine and black spruce species groups had a similar precision of 62.50% and 61.76%, respectively. The recall was, however, higher for black spruce (58.60%) than for jack pine (47.5%) dominated groups, which indicates that jack pine was misclassified as another species group more often than black spruce. Most classification errors in the jack pine and black spruce species groups occurred from the confusion of one with the other or with the mixed conifers species group. Similar to the findings of the classification into softwood, mixedwood, and hardwood, low confusion was observed between the coniferous species and the mixedwood and hardwood species. The confusion matrix is presented in and illustrated in and recall, precision, and F-score for each species group and model scenario are included in Supplementary Figures 1 and 2.
Table 5. Confusion matrix for the five species groups classification model using all predictor sets. Diagonal bold values indicate the number of observations for which the predicted species group correspond to the reference species group.
Variable importance for species groups classification
The variable importance of the 3-groups and 5-group species classification models including all predictor variables are presented in . For the most detailed 5 species groups classification model, the second red edge band of Sentinel-2 (s2_red_edge_2; λ = 740 nm), the cumulative percentile of height returns zpcum8 and the sum of segmented crown volumes (cr_vol_sum) showed markedly higher variable importance than other predictor variables for classifying species groups (). Six other variables, including four crown metrics, the 25th percentile of signal attenuation depth and zpcum9, had similarly scaled variable importance values ranging between 23% and 32%. The scaled variable importance values of the remaining variables were markedly lower (0%–14%). The most important metrics for the 5-group species classification model also generally had high variable importance in the 3-group model (). Out of the 9 variables highlighted above that had markedly higher importance in the 5-group model (23%–100%), all except zpcum9 were also ranked in the nine most important variables in the 3-group model.
Comparison of predictor variables across species groups
Not all species groups were highly distinct across the predictor variables considered (). Hardwood was generally the species group that was most distinct from others, especially when compared to jack pine and black spruce dominated groups. Importance of metrics related to crown area and volume were significantly different across all species groups except when comparing black spruce and jack pine dominated groups with the mixed conifer group as well as when comparing jack pine and black spruce dominated groups. The reflectance values of Sentinel-2 spectral bands were generally significantly different when comparing jack pine and black spruce dominated groups with the other species groups, but not—or less—significantly, different when comparing mixedwood, mixed conifer, and hardwood groups. The SWIR2 band (λ = 2190 nm) reflectance value of Sentinel-2 was significantly different (p < 0.001) when comparing mixed conifer, mixedwood, and hardwood groups with the black spruce dominated group, but not significantly different when these groups were compared to the jack pine dominated group. The only predictor variables displaying significantly different values between jack pine and black spruce dominated groups were zpcum9 (p < 0.05), zpcum8 (p < 0.01), and the Sentinel-2 SWIR2 reflectance (p < 0.05).
Figure 6. Pairwise comparison of significant differences in predictor variable values across species groups. The blue and red color gradients indicate if the pairwise difference between the median values is negative or positive, respectively. JP: jack pine dominated; BS: black spruce dominated; MC: mixed conifers; MW: mixedwood; HW: hardwood.

Comparing predicted species group to existing photo-interpreted inventory
shows the distribution of the most frequently predicted species group in the stands of the photo-interpreted forest resource inventory. The black spruce dominated species group was the most frequently predicted species group in 86.2% of the stands dominated by black spruce according to the photo-interpreted forest resource inventory. In stands classified as jack pine-dominated, jack pine was the most frequently predicted species group in 45.7% of the stands, and black spruce was the most frequently predicted species group in 29.7% of the stands. The most frequently predicted species groups in stands classified as hardwood in the photo-interpreted inventory were hardwood (53.8%) and mixedwood (40.7%). Overall, we observed a negligible number of stands where mixed conifer was the most frequently predicted species group. Black spruce dominated (68.1%) and mixedwoods (28.7%) were the most frequently predicted species groups in stands classified as mixed conifer by the photo-interpreted inventory. show some examples of stands and their species group predictions. A comparison against aerial imagery is also available in Supplementary Figure 3.
Figure 7. Chord diagram showing the distribution of the most frequently predicted species groups in the stands of the photo-interpreted inventory. The most frequently predicted species groups in each stand are shown in the bottom half and the stand-level species groups derived from photo-interpreted species composition are shown in the upper half.

Figure 8. Wall-to-wall predictions of species groups overlaid with forest stands labeled by their inventory (photo-interpreted) species groups. Predictions were only made in pixels where zp90 > 5 m, pzabove2 > 50%, and in productive forest stands. JP: jack pine dominated; BS: black spruce dominated; MC: mixed conifers; MW: mixedwood; HW: hardwood.

Discussion
In this study, we combined area-based and individual tree metrics obtained from ALS data with Sentinel-2 data to predict dominant species groups across a large (>580,000 ha) boreal forest located in Ontario, Canada. Below, we discuss the accuracy of the species group predictions, as well as the main variables that helped discriminate between species groups in the context of findings from previous research. We also discuss the implications of using the such approach in an operational context, where information needs vary depending on the forest management approaches.
Accuracy of species group predictions
Our results indicated that the prediction of species groups was the most accurate (OA = 83.20% for 3-group classification; OA = 67.79% for 5-group classification) when models combined ALS and Sentinel-2 predictors relative to models that included only subsets of these predictors. Kukkonen et al. (Citation2018) also observed that the accuracy of Scots pine (Pinus sylvestris), Norway spruce (Picea abies), and broadleaved species volume improved when using both Sentinel-2 and ALS data (RMSE = 49.8%–69.0%) compared to using ALS data only (RMSE = 53.9%–77.1%). In a 14,000 ha temperate forest, Plakman et al. (Citation2020) also found that the combination of Sentinel-2 data and crown structural metrics derived from ALS data yielded the most accurate classification of eight species (OA = 78.5%) at the individual tree level. However, their results show that models based on Sentinel-2 alone performed better (OA = 73.7%) than models only based on crown structural metrics (OA = 58.4%), which was not the case in our study.
Accuracies comparable to the one obtained in this study with the best performing model combining ALS and Sentinel-2 have been observed in studies using Sentinel-2 data alone. For example, Persson et al. (Citation2018) mapped five species in a 1500 ha boreal forest with an OA of 88.2% and Breidenbach et al. (Citation2021) mapped Scots pine (Pinus sylvestris), Norway spruce (Picea abies) and deciduous forests across Norway with an OA of 90.5%. In these two studies, models using multitemporal Sentinel-2 data, that can capture different phenological dynamics between species, outperformed models based on single-date Sentinel-2 acquisition. This could explain the fact that we observed markedly lower accuracy (OA = 65.98% for 3-group classification; OA = 46.23% for 5-group classification) in our study when using a single composite of Sentinel-2 data acquired between June and September. Moreover, our study considered mixed conifers and mixedwood species groups, which comprise a diverse range of species and are not as clearly distinguishable compared to other forest types dominated by a single species.
The accuracy of the models using ALS data only (OA = 76.2%–82.6% for 3-group classification; OA = 61.0%–63.8% for 5-group classification) was within the range of accuracy values that Fassnacht et al. (Citation2016) compiled from 17 studies classifying species with ALS data (OA = ∼55%–90%). It is important to note however that these predictions ranged from the individual tree species to species groups, in various forest types and areas as well as with different ALS sensors and acquisition parameters. To our knowledge, only one study by Prieur et al. (Citation2021) has examined tree species classification with the SPL100. Their study area was also located in Ontario, Canada but in a more complex species-environment than the Romeo Malette Forest studied herein. Prieur et al. (Citation2021) obtained accuracies comparable to the ones observed herein, with an OA of 82.9% if grouping predictions into softwood and hardwood species groups and 68.3% if grouping predictions into pine, spruce, other softwood hardwood, and intolerant hardwood species groups.
Variables driving species groups classification
Our study highlighted that the three sets of predictors provided valuable information to discriminate the modeled species groups. As discussed by Fassnacht et al. (Citation2016), species influence the distribution of ALS returns within the canopy as a function of differences in crown shape or foliage density. Two metrics representing the cumulative distribution of returns in the upper layers of the canopy (zpcum8, zpcum9) had a markedly larger conditional variable importance than the other plot-level summary ALS metrics. The signal attenuation depth metrics, which are influenced by foliage density and canopy closure, were also important variables in the random forest classification model, especially to discriminate between coniferous species groups and mixedwood or hardwood species groups. This also confirms results from Irwin et al. (Citation2021) who observed that the signal attenuation depth of the SPL100 was generally lower in closed-canopy deciduous forests, compared to coniferous forest types. It is also important to note here that that point clouds of the SPL100 tend to be dominated by first returns in the upper canopy because of the low energy of the laser beams and the filtering of noise returns that can decrease the amount of returns in the intermediate and lower layers of the canopy (Yu et al. Citation2020; Brown et al. Citation2020).
The red edge region of the electromagnetic spectrum is generally found to be of high importance in vegetation mapping because it results from a low reflectance in the red, due to top chlorophyll absorption, and a high reflectance in the near-infrared (NIR), due to the internal structure of leaves (Horler et al. Citation1983). This high relevance is also reflected in our findings, with the red edge 2 band of Sentinel-2 (λ = 740 nm) being the important spectral band to discriminate species groups, a finding that is supported by other studies carried out in forests with both coniferous and deciduous trees (Astola et al. Citation2019; Persson et al. Citation2018). The red edge 2 band was also the most significantly different (p-value < 0.001) when comparing the jack pine and black spruce dominated species groups with the mixedwood or hardwood species groups. Among the blue (λ = 490 nm), green (λ = 560 nm), red (λ = 665 nm), red edge 2 (λ = 740 nm), and SWIR 2 (λ = 2190 nm) Sentinel-2 bands included in the models, the SWIR 2 band was the only one where significantly different reflectance values were observed between the jack pine and black spruce dominated groups in our study. Sentinel-2 red edge 2 band (λ = 740 nm) and narrow-NIR band (λ = 860 nm), which were not included in our models due to its high correlation (r > 0.99) with the red edge 2 band, have also been shown to help discriminate Scots pine from Norway spruce (Mikael Kukkonen et al. Citation2018). These results are in line with previous research that has observed that the spectral reflectance of black spruce needles was lower than jack pine needles in the NIR (λ = 700 nm–1000 nm) region of the electromagnetic spectrum (Middleton et al. Citation1997). In a more recent study also examined the shortwave infrared (SWIR) region of the electromagnetic spectrum, Hovi et al. (Citation2017) observed that spectral differences between coniferous boreal tree species needles were larger in the SWIR (λ = 1300 nm–2500 nm) region of the electromagnetic spectrum compared to the visible (λ = 400 nm–700 nm) or NIR (λ = 700 nm–1300 nm) regions. In addition to the reflectance of needles, the site on which tree species occur can also influence the of each species group. For example, black spruce often occurs on wetter sites than jack pine, and the SWIR region of the electromagnetic spectrum is known to be sensitive to moisture (Zhu et al. Citation2014).
Individual tree crown features derived from ALS can be effective to separate species groups, in particular coniferous from broadleaf species (Fassnacht et al. Citation2016). On the other hand, Duncanson et al. (Citation2015) found that the accuracy of area-based estimates of above-ground biomass modeled from ITC metrics aggregated at the plot-level, as done in this research, did not outperform the accuracy of models based on plot-level height summary metrics. In our study, including ITC metrics marginally improved the accuracy of our random forest classifications compared to models with area-based metrics alone. ITC metrics were significantly different (p-value < 0.05) when comparing the hardwood species group to the conifer groups, but not among conifer groups. The metrics related to the crown area or proxy of crown volume were more important if they were summed rather than averaged within a plot or a pixel. Summed metrics could possibly capture more information, e.g., in relation to canopy cover, than averaged metrics and explain why they were more important predictors. The fact that we limited the marker-controlled watershed segmentation to the top of each tree was important. Without this limit, delineated crowns tended to occupy the entire plot or pixel area, and summed crown area metrics had limited variability across the species groups, especially when segmenting closed canopies. This approach was suitable to improve the explanatory power of the crown metrics but would not have been as effective if the objective was to isolate individual tree and their entire crowns as best as possible. More complex individual tree crown metrics, such as 3-dimensional alpha shapes that represent the envelope of a tree crown (Korhonen et al. Citation2013), could have more explanatory power than the ones that were used herein to classify tree species. However, the generation of detailed individual tree metrics across large areas, such as the Romeo Malette Forest is challenging due to the computational requirements and the necessity of tuning of individual tree segmentation algorithm parameters to different forest types.
The measure of the intensity of linear-mode LiDAR instruments, which is related to the amount of energy in the reflected pulse, can be more useful than the structural information of the point cloud to discriminate tree species (Fassnacht et al. Citation2016; Shi et al. Citation2018). Equivalent intensity measures cannot be derived from LiDAR instruments based on single photon LiDAR detection since the returned signal is binary instead of being a pulse of energy. A pseudo-intensity measure can be generated by aggregating the number of binary returns detected for each transmitted pulse but its properties and characteristics compared to the intensity of other ALS sensors are not well known (Hartzell et al. Citation2018). We did not include the SPL100 pseudo-intensity features as predictor variables in this study, and other studies have shown that it provides a limited amount of information for species-specific volume predictions or species classification. However, further research characterizing their properties across different surfaces and suitable standardization methods would be valuable.
Implication for operational forest management
Whether to use tree-level, area-based or stand-level forest inventory ultimately depends on the information needs End users have come to expect detailed species composition estimates, such as the ones provided by manually photo-interpreted inventories. However, in some contexts, knowledge of dominant species may be sufficient to support information needs, such as estimating timber volume (Tompalski et al. Citation2014). Area-based species group predictions, such as the ones derived herein can inform extensive forest management practices, which prevail in Canada where forests cover large extents, are mainly publicly owned and managed for multiple objectives (Wulder et al. Citation2007). In nations with smaller forest landbase and larger share of private ownership, intensive forest management practices are more common and require more detailed and precise species composition information than the ones derived herein. Changes in silvicultural approaches and practices, associated with changing climate broader sustainable use of forest resources (Achim et al. Citation2022), could also require a more precise inventory of forest resources across large scales.
The operational uptake of forest inventories based on individual tree attributes has partly been limited by the bias induced by over-segmentation or under-segmentation errors associated to the ITC delineation process (Vastaranta et al. Citation2012; Breidenbach et al. Citation2010; White et al. Citation2016). Semi-ITC approaches, in which detected segments can be matched to one or multiple trees have been used to reduce this bias (Breidenbach et al. Citation2010; Breidenbach and Astrup Citation2014). Although the range of forest types on which the development and accuracy of ITC algorithms are being assessed is increasing (Kaartinen et al. Citation2012), complex forest types in which a range of species and conditions coexist are limiting the application of ITC methods across large areas (Zhen et al. Citation2016; Fassnacht et al. Citation2016). Regardless, in the context of species mapping, there is no demonstrated gain in the accuracy of forest attribute prediction when using ITC or semi-ITC approaches compared to an ABA. For example, Ørka et al. (Citation2013) used ALS and high-resolution multispectral and hyperspectral imagery to estimate the proportion of spruce, pine and deciduous tree species in a boreal forest of Norway with an R2 = 0.66–0.96 when using an ITC or semi-ITC approach and an R2 = 0.83–0.89 when using an ABA. The three approaches provided estimates with relatively similar accuracy, but the ITC particularly outperformed the ABA to estimate the proportion of hardwood species that were not as frequent as pine and spruce in their study area. There are few studies however that have compared the relative performance of species predictions generated with area-based and ITC approaches and additional research quantifying differences in a range of forest environments would be informative.
Our area-based predictions of species groups had more spatial detail than the stand-level estimates from the photo-interpreted inventory. The overall stand-level patterns of species distributions were also relatively well captured, except in stands of the mixed conifers species groups where misclassifications of black spruce or mixedwood dominated. It is important to consider that the photo-interpreted data should not be necessarily considered as reference data. Differences between the predicted species groups and the ones available in the forest inventory polygons could be explained by errors in the area-based predictions, photo-interpretation errors, or time span between the photo-interpretation and the SPL100 acquisition. By using consistent data sources and methods, species predictions with remotely sensed data also avoid issues associated with subjective interpretation, particularly when many photo-interpreters are involved as is common when inventories span large forest management units.
The derivation of the aggregated crown metrics developed herein is more computationally intensive than the standard area-based summary metrics, which is an important consideration for operational uptake. However, the ITD and ITC algorithms that we used were applied on the raster-based CHM, which is faster than applying these algorithms directly on the point cloud. Moreover, no predictions were made at the individual tree level since ITC metrics were aggregated, which is an important implication for processing time in large areas, such as the Romeo Malette Forest. Area-based species information, together with area-based forest inventory attributes could be used to automatically delineate homogeneous forest stands similar to photo-interpreted stands as part of the forest inventory. In addition, the wall-to-wall species group predictions could be used to select which optimal set of ITC methods and parameters should be used for a stand of interest.
In this study, we observed a relatively marginal gain in species group classification OA when combining ALS and Sentinel-2 predictor variables compared to using ALS metrics only, particularly for the 3-groups species classification (). The Romeo Malette forest is actively managed and some of the structural differences captured by the ALS summary metrics could be related to past silvicultural treatments targeting specific stands or species groups. In unmanaged forests, ALS metrics, in particular those related to height, could be less important and the gain in prediction accuracy obtained by combining ALS and Sentinel-2 data could be larger than the one we observed herein. Sentinel-2 data are global, free, and publicly available and their integration into the generation of enhanced forest inventory products is relatively straightforward. As discussed above, few studies have examined the integration of ALS and Sentinel-2 imagery for forest inventory products, and further research, particularly in areas with more diverse species composition and different management regimes, would be interesting to better understand the benefits of integrating such data into forest inventory frameworks.
Conclusion
ALS data are being increasingly integrated into forest inventory workflows to provide detailed and spatially extensive area-based estimates of key forest attributes for forest managers. However, information related to species composition is often obtained from other data sources, such as photo-interpreted imagery, which does not match the spatial resolution of ALS-based inventories and can have relatively poor accuracy. Previous research has examined individual tree species classification with ALS data, often in combination with spectral data from high-resolution imagery, but scaling this information up to large extents remains a challenge. In this study, we demonstrated across a large forest area (>580,000 ha) that some of the species composition information provided by photo-interpreted inventories can be derived with relatively high accuracy using a combination of area-based and individual tree crown summary metrics of forest structure from ALS data as well as moderate resolution multispectral data from Sentinel-2. We found that the spectral information of Sentinel-2, particularly in the near-infrared region of the electromagnetic spectrum (λ = 740 nm), the area and volume of upper tree crowns, and the cumulative distribution of returns in the upper sections of the canopy were the most important metrics in the classification models. Area-based species grouping products, such as the ones derived herein could be integrated operationally to inform regional forest management and monitoring as well as used to guide the derivation of more precise information (i.e., tree-level) where needed. Future research improving the amount of detail in species composition from remotely sensed products in an operationally viable manner would be particularly useful for forest management.
Supplemental Material
Download MS Word (3.5 MB)Acknowledgments
We thank the Ontario Ministry of Natural Resources and Forestry for providing us with the LiDAR and field data used in this research.
Disclosure statement
No potential conflict of interest was reported by the author(s).
Additional information
Funding
References
- Achim, A., Moreau, G., Coops, N.C., Axelson, J.N., Barrette, J., Bédard, S., Byrne, K.E., et al. 2022. “The changing culture of silviculture.” Forestry: An International Journal of Forest Research, Vol. 95(No. 2): pp. 143–152. doi:10.1093/forestry/cpab047.
- Astola, H., Häme, T., Sirro, L., Molinier, M., and Kilpi, J. 2019. “Comparison of Sentinel-2 and Landsat 8 imagery for forest variable prediction in Boreal region.” Remote Sensing of Environment, Vol. 223: pp. 257–273. doi:10.1016/j.rse.2019.01.019.
- Axelsson, A., Lindberg, E., and Olsson, H. 2018. “Exploring multispectral ALS data for tree species classification.” Remote Sensing, Vol. 10(No. 3): pp. 183. doi:10.3390/rs10020183.
- Bilyk, A., Pulkki, R., Shahi, C., and Larocque, G.R. 2021. “Development of the Ontario Forest Resources Inventory: A historical review.” Canadian Journal of Forest Research, Vol. 51(No. 2): pp. 198–209. doi:10.1139/cjfr-2020-0234.
- Breidenbach, J., and Astrup, R. 2014. “The semi-individual tree crown approach.” In Forestry Applications of Airborne Laser Scanning. Managing Forest Ecosystems, 113–133. Dordrecht: Springer. doi:10.1007/978-94-017-8663-8_6.
- Breidenbach, J., Naesset, E., Lien, V., Gobakken, T., and Solberg, S. 2010. “Prediction of Species Specific Forest Inventory attributes using a nonparametric semi-individual tree crown approach based on fused airborne laser scanning and multispectral data.” Remote Sensing of Environment, Vol. 114(No. 4): pp. 911–924. doi:10.1016/j.rse.2009.12.004.
- Breidenbach, J., Waser, L.T., Debella-Gilo, M., Schumacher, J., Rahlf, J., Hauglin, M., Puliti, S., and Astrup, R. 2021. “National mapping and estimation of forest area by dominant tree species using Sentinel-2 data.” Canadian Journal of Forest Research, Vol. 51(No. 3): pp. 365–379. doi:10.1139/cjfr-2020-0170.
- Brown, R., Hartzell, P., and Glennie, C. 2020. “Evaluation of SPL100 single photon LiDAR data.” Remote Sensing, Vol. 12(No. 4): pp. 722. doi:10.3390/rs12040722.
- Budei, B.C., St-Onge, B., Hopkinson, C., and Audet, F.A. 2018. “Identifying the genus or species of individual trees using a three-wavelength airborne LiDAR system.” Remote Sensing of Environment, Vol. 204: pp. 632–647. doi:10.1016/j.rse.2017.09.037.
- Coops, N.C., Tompalski, P., Goodbody, T.R.H., Queinnec, M., Luther, J.E., Bolton, D.K., White, J.C., et al. 2021. “Modelling LiDAR-derived estimates of forest attributes over space and time: A review of approaches and future trends.” Remote Sensing of Environment, Vol. 260: pp. 112477. doi:10.1016/j.rse.2021.112477.
- Coops, N.C., Wulder, M.A., Culvenor, D.S., and St-Onge, B. 2004. “Comparison of forest attributes extracted from fine spatial resolution multispectral and LiDAR data.” Canadian Journal of Remote Sensing, Vol. 30(No. 6): pp. 855–866. doi:10.5589/m04-045.
- Dalponte, M., Ørka, H.O., Ene, L.T., Gobakken, T., and Naesset, E. 2014. “Tree crown delineation and tree species classification in Boreal forests using hyperspectral and ALS data.” Remote Sensing of Environment, Vol. 140: pp. 306–317. doi:10.1016/j.rse.2013.09.006.
- Duncanson, L.I., Dubayah, R.O., Cook, B.D., Rosette, J., and Parker, G. 2015. “The importance of spatial detail: Assessing the utility of individual crown information and scaling approaches for LiDAR-based biomass density estimation.” Remote Sensing of Environment, Vol. 168: pp. 102–112. doi:10.1016/j.rse.2015.06.021.
- Fassnacht, F.E., Latifi, H., Stereńczak, K., Modzelewska, A., Lefsky, M., Waser, L.T., Straub, C., and Ghosh, A. 2016. “Review of studies on tree species classification from remotely sensed data.” Remote Sensing of Environment, Vol. 186: pp. 64–87. doi:10.1016/j.rse.2016.08.013.
- Gamfeldt, L., Snäll, T., Bagchi, R., Jonsson, M., Gustafsson, L., Kjellander, P., Ruiz-Jaen, M.C., et al. 2013. “Higher levels of multiple ecosystem services are found in forests with more tree species.” Nature Communications, Vol. 4(No. 1): pp. 1–8. doi:10.1038/ncomms2328.
- Gluckman, J. 2016. “Design of the processing chain for a high-altitude, airborne, single photon LiDAR mapping instrument.” Laser Radar Technology and Applications XXI (Vol. 9832), 20–28. Bellingham, Washington USA: SPIE. doi:10.1117/12.2219760.
- Grabska, E., Hostert, P., Pflugmacher, D., and Ostapowicz, K. 2019. “Forest stand species mapping using the Sentinel-2 time series.” Remote Sensing, Vol. 11(No. 10): pp. 1197. doi:10.3390/rs11101197.
- Harding, D. 2018. “Pulsed laser altimeter ranging techniques and implications for terrain mapping.” In Topographic Laser Ranging and Scanning, 2nd ed., 201–220. Boca Raton, FL: Taylor & Francis; CRC Press. doi:10.1201/9781315154381-5.
- Hartzell, P., Dang, Z., Pan, Z., and Glennie, C. 2018. “Radiometric evaluation of an airborne single photon LiDAR sensor.” IEEE Geoscience and Remote Sensing Letters, Vol. 15(No. 9): pp. 1466–1470. doi:10.1109/LGRS.2018.2841811.
- Hemmerling, J., Pflugmacher, D., and Hostert, P. 2021. “Mapping temperate forest tree species using dense Sentinel-2 time series.” Remote Sensing of Environment, Vol. 267: pp. 112743. doi:10.1016/j.rse.2021.112743.
- Holmgren, J., and Persson, Å. 2004. “Identifying species of individual trees using airborne laser scanner.” Remote Sensing of Environment, Vol. 90(No. 4): pp. 415–423. doi:10.1016/S0034-4257(03)00140-8.
- Holmgren, J., Persson, Å., and Söderman, U. 2008. “Species identification of individual trees by combining high resolution LiDAR data with multi-spectral images.” International Journal of Remote Sensing, Vol. 29(No. 5): pp. 1537–1552. doi:10.1080/01431160701736471.
- Horler, D.N.H., Dockray, M., and Barber, J. 1983. “The red edge of plant leaf reflectance.” International Journal of Remote Sensing, Vol. 4(No. 2): pp. 273–288. doi:10.1080/01431168308948546.
- Hothorn, T., Bühlmann, P., Dudoit, S., Molinaro, A., and Laan, M.J. and Van Der, 2006. “Survival ensembles.” Biostatistics, Vol. 7(No. 3): pp. 355–373. doi:10.1093/biostatistics/kxj011.
- Hovi, A., Raitio, P., and Rautiainen, M. 2017. “A spectral analysis of 25 Boreal tree species.” Silva Fennica, Vol. 51(No. 4): 7753. doi:10.14214/sf.7753.
- Irwin, L., Coops, N.C., Queinnec, M., McCartney, G., and White, J.C. 2021. “Single photon LiDAR signal attenuation under Boreal forest conditions.” Remote Sensing Letters, Vol. 12(No. 10): pp. 1049–1060. doi:10.1080/2150704X.2021.1962575.
- Kaartinen, H., Hyyppä, J., Yu, X., Vastaranta, M., Hyyppä, H., Kukko, A., Holopainen, M., et al. 2012. “An international comparison of individual tree detection and extraction using airborne laser scanning.” Remote Sensing, Vol. 4(No. 4): pp. 950–974. doi:10.3390/rs4040950.
- Korhonen, L., Vauhkonen, J., Virolainen, A., Hovi, A., and Korpela, I. 2013. “Estimation of tree crown volume from airborne LiDAR data using computational geometry.” International Journal of Remote Sensing, Vol. 34(No. 20): pp. 7236–7248. doi:10.1080/01431161.2013.817715.
- Kukkonen, M., Korhonen, L., Maltamo, M., Suvanto, A., and Packalen, P. 2018. “How much can airborne laser scanning based forest inventory by tree species benefit from auxiliary optical data?” International Journal of Applied Earth Observation and Geoinformation, Vol. 72: pp. 91–98. doi:10.1016/j.jag.2018.06.017.
- Kukkonen, M., Maltamo, M., Korhonen, L., and Packalen, P. 2019. “Multispectral airborne LiDAR data in the prediction of Boreal tree species composition.” IEEE Transactions on Geoscience and Remote Sensing, Vol. 57(No. 6): pp. 3462–3471. doi:10.1109/TGRS.2018.2885057.
- Leckie, D.G., and Gillis, M.D. 1995. “Forest Inventory in Canada with emphasis on map production.” The Forestry Chronicle, Vol. 71(No. 1): pp. 74–88. doi:10.5558/tfc71074-1.
- Lindberg, E., Holmgren, J., Olofsson, K., Wallerman, J., and Olsson, H. 2013. “Estimation of tree lists from airborne laser scanning using tree model clustering and K-MSN imputation.” Remote Sensing, Vol. 5(No. 4): pp. 1932–1955. doi:10.3390/rs5041932.
- Main-Knorn, M., Pflug, B., Louis, J., Debaecker, V., Müller-Wilm, U., and Gascon, F. 2017. “Sen2Cor for Sentinel-2.” October 3. doi:10.1117/12.2278218.
- Maltamo, M., Packalen, P., and Kangas, A. 2021. “From comprehensive field inventories to remotely sensed wall-to-wall stand attribute data-a brief history of management inventories in the Nordic countries.” Canadian Journal of Forest Research, Vol. 51(No. 2): pp. 257–266. doi:10.1139/cjfr-2020-0322.
- Maltamo, M., and Packalen, P. 2014. “Species-specific management inventory in Finland.” In Forestry Applications of Airborne Laser Scanning. Managing Forest Ecosystems, 241–252. Dordrecht: Springer. doi:10.1007/978-94-017-8663-8_12.
- Michałowska, M., and Rapiński, J. 2021. “A review of tree species classification based on airborne LiDAR data and applied classifiers.” Remote Sensing, Vol. 13(No. 3): pp. 353–27. doi:10.3390/rs13030353.
- Middleton, E.M., Chan, S.S., Rusin, R.I., and Mitchell, S.K. 1997. “Optical properties of black spruce and jack pine needles at BOREAS sites in Saskatchewan, Canada.” Canadian Journal of Remote Sensing, Vol. 23(No. 2): pp. 108–119. doi:10.1080/07038992.1997.10855193.
- Næsset, E. 2002. “Predicting forest stand characteristics with airborne scanning laser using a practical two-stage procedure and field data.” Remote Sensing of Environment, Vol. 80(No. 1): pp. 88–99. doi:10.1016/S0034-4257(01)00290-5.
- Ørka, H.O., Dalponte, M., Gobakken, T., Naesset, E., and Ene, L.T. 2013. “Characterizing forest species composition using multiple remote sensing data sources and inventory approaches.” Scandinavian Journal of Forest Research, Vol. 28(No. 7): pp. 677–688. doi:10.1080/02827581.2013.793386.
- Parton, J., Vasiliauskas, S., Lucking, G., and Watt, W. 2006. Standard forest units for Northeastern Ontario Boreal Forests. Northeast Science & Information, NESI Technical Note TN021. Science & Information Branch, Northeast Science & Information, Ministry of Natural Resources.
- Persson, M., Lindberg, E., and Reese, H. 2018. “Tree species classification with multi-temporal Sentinel-2 data.” Remote Sensing, Vol. 10(No. 11): pp. 1794. doi:10.3390/rs10111794.
- Pinto, F., Rouillard, D., Sobze, J.M., and Ter-Mikaelian, M. 2007. “Validating tree species composition in forest resource inventory for Nipissing Forest, Ontario, Canada.” The Forestry Chronicle, Vol. 83(No. 2): pp. 247–251. doi:10.5558/tfc83247-2.
- Plakman, V., Janssen, T., Brouwer, N., and Veraverbeke, S. 2020. “Mapping species at an individual-tree scale in a temperate forest, using Sentinel-2 images, airborne laser scanning data, and random forest classification.” Remote Sensing, Vol. 12(No. 22): pp. 3710. doi:10.3390/rs12223710.
- Popescu, S.C., and Wynne, R.H. 2004. “Seeing the trees in the forest: Using LiDAR and multispectral data fusion with local filtering and variable window size for estimating tree height.” Photogrammetric Engineering & Remote Sensing, Vol. 70(No. 5): pp. 589–604. doi:10.14358/PERS.70.5.589.
- Prieur, J.F., St-Onge, B., Fournier, R.A., Woods, M.E., Rana, P., and Kneeshaw, D. 2021. “A comparison of three airborne laser scanner types for species identification of individual trees.” Sensors, Vol. 22(No. 1): pp. 35. doi:10.3390/s22010035.
- Queinnec, M., Coops, N.C., White, J.C., McCartney, G., and Sinclair, I. 2022. “Developing a forest inventory approach using airborne single photon LiDAR data: From ground plot selection to forest attribute prediction.” Forestry: An International Journal of Forest Research, Vol. 95(No. 3): pp. 347–362. doi:10.1093/forestry/cpab051.
- R Core Team. 2022. R: A Language and Environment for Statistical Computing. Vienna. https://www.r-project.org/.
- Ranghetti, L., Boschetti, M., Nutini, F., and Busetto, L. 2020. “‘Sen2r’: An R toolbox for automatically downloading and preprocessing Sentinel-2 satellite data.” Computers & Geosciences, Vol. 139: pp. 104473. doi:10.1016/j.cageo.2020.104473.
- Roussel, J.-R., Auty, D., Coops, N.C., Tompalski, P., Goodbody, T.R.H., Meador, A.S., Bourdon, J.-F., Boissieu, F. de, and Achim, A. 2020. “LidR: An R package for analysis of airborne laser scanning (ALS) data.” Remote Sensing of Environment, Vol. 251: pp. 112061. doi:10.1016/j.rse.2020.112061.
- Shi, Y., Wang, T., Skidmore, A.K., and Heurich, M. 2018. “Important LiDAR metrics for discriminating forest tree species in Central Europe.” ISPRS Journal of Photogrammetry and Remote Sensing, Vol. 137: pp. 163–174. doi:10.1016/j.isprsjprs.2018.02.002.
- Strobl, C., Boulesteix, A.-L., Zeileis, A., and Hothorn, T. 2007. “Bias in random forest variable importance measures: Illustrations, sources and a solution.” BMC Bioinformatics, Vol. 8(No. 1): pp. 25–21. doi:10.1186/1471-2105-8-25.
- Strobl, C., Boulesteix, A.L., Kneib, T., Augustin, T., and Zeileis, A. 2008. “Conditional variable importance for random forests.” BMC Bioinformatics, Vol. 9(No. 1): pp. 1–11. doi:10.1186/1471-2105-9-307.
- Thompson, I.D., Maher, S.C., Rouillard, D.P., Fryxell, J.M., and Baker, J.A. 2007. “Accuracy of forest inventory mapping: Some implications for Boreal forest management.” Forest Ecology and Management, Vol. 252(No. 1–3): pp. 208–221. doi:10.1016/j.foreco.2007.06.033.
- Tompalski, P., Coops, N.C., White, J.C., and Wulder, M.A. 2014. “Simulating the Impacts of Error in Species and Height upon Tree Volume Derived from Airborne Laser Scanning Data.” Forest Ecology and Management, Vol. 327: pp. 167–177. doi:10.1016/j.foreco.2014.05.011.
- Tompalski, P., Coops, N.C., Marshall, P.L., White, J.C., Wulder, M.A., and Bailey, T. 2018. “Combining multi-date airborne laser scanning and digital aerial photogrammetric data for forest growth and yield modelling.” Remote Sensing, Vol. 10(No. 3): pp. 347. doi:10.3390/rs10020347.
- Tompalski, P., White, J.C., Coops, N.C., Wulder, M.A., Leboeuf, A., Sinclair, I., Butson, C.R., and Lemonde, M.O. 2021. “Quantifying the precision of forest stand height and canopy cover estimates derived from air photo interpretation.” Forestry: An International Journal of Forest Research, Vol. 94(No. 5): pp. 611–629. doi:10.1093/forestry/cpab022.
- Vastaranta, M., Kankare, V., Holopainen, M., Yu, X., Hyyppä, J., and Hyyppä, H. 2012. “Combination of individual tree detection and area-based approach in imputation of forest variables using airborne laser data.” ISPRS Journal of Photogrammetry and Remote Sensing, Vol. 67: pp. 73–79. doi:10.1016/j.isprsjprs.2011.10.006.
- Véga, C., Renaud, J.P., Durrieu, S., and Bouvier, M. 2016. “On the interest of penetration depth, canopy area and volume metrics to improve LiDAR-based models of forest parameters.” Remote Sensing of Environment, Vol. 175: pp. 32–42. doi:10.1016/j.rse.2015.12.039.
- Wästlund, A., Holmgren, J., Lindberg, E., and Olsson, H. 2018. “Forest variable estimation using a high altitude single photon LiDAR system.” Remote Sensing, Vol. 10(No. 9): pp. 1422. doi:10.3390/rs10091422.
- White, J.C., Coops, N.C., Wulder, M.A., Vastaranta, M., Hilker, T., and Tompalski, P. 2016. “Remote sensing technologies for enhancing forest inventories: A review.” Canadian Journal of Remote Sensing, Vol. 42(No. 5): pp. 619–641. doi:10.1080/07038992.2016.1207484.
- White, J.C., Penner, M., and Woods, M. 2021. “Assessing single photon LiDAR for operational implementation of an enhanced forest inventory in diverse mixedwood forests.” The Forestry Chronicle, Vol. 97(No. 1): pp. 78–96. doi:10.5558/tfc2021-009.
- White, J.C., Wulder, M.A., Varhola, A., Vastaranta, M., Coops, N.C., Cook, B.D., Pitt, D., and Woods, M. 2013. “A best practices guide for generating forest inventory attributes from airborne laser scanning data using an area-based approach.” Forestry Chronicle, Vol. 89(No. 6): pp. 722–723. doi:10.5558/tfc2013-132.
- Woods, M., Lim, K., and Treitz, P. 2008. “Predicting forest stand variables from LiDAR data in the Great Lakes – St. Lawrence Forest of Ontario.” Forestry Chronicle, Vol. 84(No. 6): pp. 827–839. doi:10.5558/tfc84827-6.
- Woods, M., Pitt, D., Penner, M., Lim, K., Nesbitt, D., Etheridge, D., and Treitz, P. 2011. “Operational Implementation of a LiDAR Inventory in Boreal Ontario.” The Forestry Chronicle, Vol. 87(No. 4): pp. 512–528. doi:10.5558/tfc2011-050.
- Wulder, M.A., Bater, C.W., Coops, N.C., Hilker, T., and White, J.C. 2008. “The role of LiDAR in sustainable forest management.” The Forestry Chronicle, Vol. 84(No. 6): pp. 807–826. doi:10.5558/tfc84807-6.
- Wulder, M.A., Campbell, C., White, J.C., Flannigan, M., and Campbell, I.D. 2007. “National circumstances in the international circumboreal community.” The Forestry Chronicle, Vol. 83(No. 4): pp. 539–556. doi:10.5558/tfc83539-4.
- Wulder, M.A., Coops, N.C., Hudak, A.T., Morsdorf, F., Nelson, R., Newnham, G., and Vastaranta, M. 2014. “Status and prospects for LiDAR remote sensing of forested ecosystems.” Canadian Journal of Remote Sensing, Vol. 39(No. S1): pp. S1–S5. doi:10.5589/M13-051.
- Yu, X., Kukko, A., Kaartinen, H., Wang, Y., Liang, X., Matikainen, L., and Hyyppä, J. 2020. “Comparing features of single and multi-photon LiDAR in Boreal forests.” ISPRS Journal of Photogrammetry and Remote Sensing, Vol. 168: pp. 268–276. doi:10.1016/j.isprsjprs.2020.08.013.
- Zhen, Z., Quackenbush, L.J., and Zhang, L. 2016. “Trends in automatic individual tree crown detection and delineation—Evolution of LiDAR data.” Remote Sensing, Vol. 8(No. 4): pp. 333. doi:10.3390/rs8040333.
- Zhu, G., Ju, W., Chen, J.M., and Liu, Y. 2014. “A novel moisture adjusted vegetation index (MAVI) to reduce background reflectance and topographical effects on LAI retrieval.” PLOS One, Vol. 9(No. 7): pp. e102560. doi:10.1371/journal.pone.0102560.