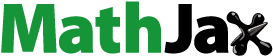
ABSTRACT
Later chronotypes may be associated with lower diet quality and later timing of energy intake in paediatric age. Period of data collection of these variables may affect these parameters and inter-relationship. We aimed to assess the cross-sectional association of chronotype with a diet quality score (HEI) and caloric midpoint (time of achieving 50% of total daily energy intake), considering summer holidays, using data from the National Food, Nutrition and Physical Activity Survey 2015–2016 for 578 participants (6–14 years of age). Chronotype was estimated by the midpoint of sleep and categorized as Early, Intermediate and Late, using physical activity diaries, while outcomes using two food diaries/24 h recalls. Associations of chronotype with outcomes were assessed by linear regressions adjusted for sex, age, parental education (model 1), BMI, sports practice (model 2), and summer holidays (no/yes) (model 3). In model 2, a Late vs. Early chronotype was negatively associated with HEI ( = −0.74; 95% CI: −1.47, −0.07) and positively with caloric midpoint (
= 0.35; 95% CI: 0.02, 0.69). However, associations lost significance in model 3. The association between chronotype and dietary habits may be benefit from being studied considering school terms and summer holidays. Future larger prospective studies are needed to clarify the role of summer holidays on this inter-relationship.
Introduction
Humans are adapted to the 24-h day through an internal circadian clock system, composed by a central clock, the suprachiasmatic nucleus, and peripheral clocks in various tissues (Cagampang and Bruce Citation2012). The endogenous clocks govern cyclic processes including metabolism and sleep-wake and fasting-feeding cycles, being also synchronized by the light-dark cycle and energy intake (Cagampang and Bruce Citation2012).
Chronotype reflects the individual differences in the entrainment of the circadian clock, being influenced by environmental, biological, and social factors such as work or school schedules (Roenneberg et al. Citation2019). The Munich ChronoType Questionnaire (MCTQ) (Roenneberg et al. Citation2003) assesses sleep onset and sleep end timings and estimates chronotype by the clock time of the midpoint of sleep on free days (MSF).
Chrononutrition focuses on the study of the fasting-feeding cycle, encompassing the timing, frequency, and regularity of food intake which are also influenced by sleep and social schedules (Almoosawi et al. Citation2019).
A later/evening chronotype, compared to an earlier/morning type, has been positively associated with a poorer diet quality (Rodríguez-Cortés et al. Citation2022) and a later timing of food intake in adults (Phoi et al. Citation2022) and children (Roßbach et al. Citation2018). In turn, these may be positively associated with overweight in children (Eng et al. Citation2009; Vilela et al. Citation2019), and in adults, when timing is measured by the caloric midpoint, which is the time at which 50% of total daily energy intake is achieved. (Teixeira et al. Citation2019).
School-aged youth is particular susceptible to exhibit a mismatch between the dark-light cycle, sleep and eating cycles, since their chronotype becomes later with age, and they experience different constraints during summer holidays, compared to school terms, which may promote later sleep times, poorer and later eating habits (Brazendale et al. Citation2017; Moreno et al. Citation2019). Evidence is still scarce and heterogenous on the relationship between chronotype and eating habits in paediatric age, especially during school terms/holidays (Almoosawi et al. Citation2019). Hence, this study aims to assess the association of chronotype, as the MSF, with diet quality and timing of energy intake, considering the period of data collection in summer holidays and school terms, in Portuguese children and adolescents (6–14 years (y)).
Materials and methods
IAN-AF 2015–2016 survey
This study used data from the National Food, Nutrition and Physical Activity Survey of the Portuguese population, 2015–2016 (IAN-AF 2015–2016) (Lopes et al. Citation2017, Citation2018). In brief, a representative sample of the general Portuguese population (3 months–84 y) was selected, using the National Health Registry as the sampling frame and a multi-stage random sampling approach. In the firs sampling stage, within each of the seven geographical regions of the Nomenclature of Territorial Units for Statistics (NUTS II), Health Units were randomly selected, weighed by the number of registered health users. In the second, within each Unit, individuals were randomly selected, by sex and age groups. From October 2015 to September 2016, comprising both school terms and holidays, two personal computer-assisted interviews (8–15 d apart) were conducted by trained staff, using the “You eAT&Move” software developed for the survey (Goios et al. Citation2020).
Study location and time zones
Portugal is constituted by Portugal mainland and the Archipelagos of Madeira and Azores. Winter’15 occurred from December 22nd to March 20th and Summer’16 from June 20th to September 22nd. Daylight Saving Time (DST) is adopted between the end of March and end of October. Standard time of Portugal mainland and Madeira is UTC + 0 and UTC + 1 during DST, whilst Azores standard time is UTC-1 and UTC + 0 during DST. Hereafter, clock times are presented in local time 24-h format (h:min) and were converted to decimal hours for statistical analysis.
Participant selection
A total of 5811 individuals completed the dietary assessments, including 1327 children under 10 y and 632 adolescents aged 10–17 y, in the IAN-AF 2015–2016 (Lopes et al. Citation2017, Citation2018). Physical activity was assessed by physical activity (PA) diaries only for participants aged 6 to 14 y, which allowed chronotype estimation, and by the International Physical Activity Questionnaire for those above 14 y. For the present study, inclusion criteria were school-aged children and adolescents (5–14 y) with two valid 24-h recalls or food diaries (n = 716) and three or more PA diaries (≥12 h of reported activities), including one weekday and at least one weekend night (Friday to Saturday or Saturday to Sunday) (n = 593). Exclusion criteria were having reported health conditions that could affect either circadian rhythms [autism spectrum disorders (n = 2), attention deficit and/or hyperactivity disorder (n = 4), epilepsy (n = 2) and cancer of the central nervous system (n = 1)] or diet quality due to restriction of certain food groups [diabetes (n = 1), food allergies (n = 2) and intolerances (n = 3)]. The final sample (n = 578) was composed of 237 children (6–9 y) and 341 adolescents (10–14 y).
Data collection
Dietary intake
Dietary intake was assessed following the European Food Safety Authority (EFSA) guidelines (European Food Safety Authority Citation2014) by two non-consecutive 24-h recalls responded by adolescents plus their main caregiver; or by two non-consecutive food diaries filled by parents of children under 10 y of age. The timing, location, types and quantities of consumed food and beverages were collected per eating occasion with the validated “eAT” platform (Goios et al. Citation2020), using the FoodEX2 classification system (European Food Safety Authority Citation2011), food pictures (Torres et al. Citation2017), and an extended version of the Portuguese Food Composition Table for energy and nutrient intake estimation (Instituto Nacional de Saúde Ricardo Jorge Citation2006).
Diet quality
Diet quality was assessed by a healthy eating index (HEI) previously developed and validated for Portuguese children (Pinto da Costa et al. Citation2019) and adapted to adolescents (Vilela et al. Citation2020). Briefly, this index included four food groups considered healthier (Fruit and vegetables; Cereals and potatoes; Dairy; White meat, fish and eggs) and five considered less healthy (Red meat and processed meat; Salty snacks; Sugar-sweetened beverages; Sugar and honey; Sweets), based on the paediatric dietary recommendations of the World Health Organization (WHO) (World Health Organization Citation2006). From the average daily consumption of the 2 d (g/d), quartiles were calculated for each food group, separately for children (3–9 y) and adolescents (10–14 y). From the lowest to the highest quartile, a score between 1 and 4 points was assigned, in ascending order for healthier food groups, and in descending order for less healthy ones. Since Salty snacks and Sugar and honey had a higher prevalence of non-consumers, consumption of each of these food groups were re-categorised as no (0 g/d)) or as yes (>0 g/d), which were assigned 2 and 1 points, respectively.
The sum of the scores of the nine food groups resulted in the final HEI score which could range from 9 points to 32 points. The higher the score, the greater adherence to a healthier eating pattern and therefore, a higher diet quality.
For the present sample, separately for children and adolescents, the mean daily consumption of HEI’s food groups (g/d) by each quartile and the proportions of consumption of Salty snacks and Sugar and honey (no/yes) are in Table S1 in supplementary materials.
Timing of energy intake
Timing of energy intake was assessed as the distribution of proportion of total daily energy intake (TEI) throughout the 24-h day. Using two food diaries or 24-h recalls, TEI (kcal) and respective quartiles were calculated for each weekday and weekend day. Then, it was determined the clock times (h:min) at which the following parameters occurred: energy intake onset (defined by the first eating occasion reported) 25%, 50% and 75% of TEI and energy intake offset, given by the last eating occasion reported. The average times of the 2 d were then obtained. The caloric midpoint was defined as the clock time at which 50% of daily TEI was achieved (McHill et al. Citation2017). It was selected as the summary measure of temporal distribution of energy intake. Since individuals can exhibit variations of the timing of food intake on free days and on school/workdays the caloric midpoint lag was calculated for individuals who had dietary reports on both types of days (n = 150) as the difference between the mean caloric midpoint on weekends and on weekdays.
Physical activity diaries
Adolescents and children’s parents completed PA diaries adapted from Bouchard’s 3-d-activity record (Bouchard et al. Citation1983), on two consecutive weekdays and two weekend days. Individuals were given a grid, in paper, for each day to report their primary activity every 15-min interval over 24 h, from 00:00 h to 24:00 h (Lopes et al. Citation2018). In the computerized PA diaries, only two activities were related to sleep-wake behaviour: “sleeping” and “getting ready to sleep.”
Chronotype
In this study, chronotype estimation was performed by adapting Roenneberg’s work and parts of the MCTQ (Roenneberg et al. Citation2019). The MCTQ for children and adolescents includes questions regarding sleep onset and end times on school days and school-free days, and alarm clock use (Roennenberg et al. Citation2015). Using that information, the MSF and the midpoint of sleep on workdays (MSW) are determined as the halfway point between sleep onset and sleep end times. If sleep duration is longer on free days and no alarm is used, MSF is corrected for sleep debt accumulated during the workweek (MSFsc) (Roenneberg et al. Citation2019). The proposed formulas were adapted in the present study, as done by other authors who used specific questions about sleep times (Phoi et al. Citation2022). All formulas can be consulted as supplementary material in Table S2. Here, weeknights were considered as workdays, and weekend nights as free days, with no alarm or imposed wake up time. The activity described as “sleeping” was screened, and naps during the day were excluded as preconized by MCTQ (Roennenberg et al. Citation2015). For each weekend night and weeknight, the reported first and last clock times of “sleeping” on PA diaries were, respectively, used as proxies of sleep onset and sleep end, to calculate MSF, MSW and respective averages. The same process was performed for sleep duration. If sleep duration on weekend nights was longer than on weeknights, the average MSF was adjusted for the calculated sleep debt during the school week, generating a sleep debt adjusted MSF (MSFsa) (h:min). Chronotype was then categorised according to MSFsa tertiles as Early, Intermediate and Late.
Missing data handling
For this study, there were around 40% missing first clock times of “sleeping” which were necessary to compute MSW and 34% to compute MSF. In this sub-heading, the process of missing definition and handling will be explained. For simplification, the first clock times of “sleeping” will be described as “bedtimes.”
Each day of the PA diaries started at 00:00 h and ended at 24:00 h. On a given day, when a participant went to bed after 00:00 h, the activity “sleeping” would be first mentioned at the applicable time of the same day. When a participant went to bed at exactly 00:00 h or earlier, the diary would start at 00:00 h with “sleeping,” and the last activity of the previous day had to be checked to ascertain the bedtime. If the participant had not filled a diary in the previous day, this bedtime was unknown and considered to be missing. In this sample, of the total bedtimes on weekdays (n = 1212) and on weekends (n = 1169), there were 474 and 402 missing bedtimes on a previous day, respectively
Missing bedtimes were due to a lack of consecutiveness of days of the PA diaries, either because it was not required between a weekday and weekend day or Saturday and Sunday, or the instruction of consecutiveness between two weekdays was not followed. To the best of our knowledge, the cause for missingness was not related with our exposure nor outcomes. Thus, we assumed that missing bedtimes on weekdays and weekends were missing at random (Little and Rubin Citation2014). A two-step single imputation approach was applied for free days and weekdays separately. For each day of each participant, we performed a mean imputation with the reported first clock time of “sleeping” on other free days (n = 352) and weekdays (n = 471). If the participant did not have other free days (n = 50) or weekdays (n = 3), missing bedtimes were imputed using a linear regression model with significant covariates (participant’s sex, age, and last clock time of “sleeping” on the respective day).
Summer school holidays
In the 2015/16 schoolyear, summer holidays began on June 9th, for grades 1–8 (6–14 y of age), and on June 3rd , for grade 9 (14 y). The end of summer holidays was given by the start of the 2016/17 schoolyear, which occurred between 9th to 15th of September. Thus, the period of summer holidays was considered to be from June 9th to September 15, 2016. Summer holidays was categorized as no, if no free days of the PA diaries occurred during summer holidays, and as yes, if the free days of PA diaries did occur within this period.
Other covariates
Participants’ sex and age at first interview (y) were self-reported. Sports practice (no/yes) was self-reported by the question: “Do you practice extra-curricular regular and programmed sports physical activity?.” Participants’ height (to the nearest 0.1 cm) and weight (nearest 0.1 Kg) were objectively measured according to procedure standards (Stewart and Marfell-Jones Citation2001). Body mass index (BMI) (Kg/m2) was calculated, and four categories were defined using the WHO’s sex-specific BMI-for-age z-scores cut-offs (de Onis et al. Citation2006). In this sample, proportions of underweight (< −2 standard deviations (SD)), normal weight (−2 ≤ SD < +1), pre-obesity (+1 ≤ SD < +2), and obesity (≥+2 SD) were 1.2%, 62.8%, 23.4% and 12.6%, respectively. Since underweight had a low prevalence in this sample and most literature does not analyse pre-obesity and obesity separately (Chaput et al. Citation2015; Eng et al. Citation2009), BMI category was re-categorised as underweight or normal weight, if BMI-for-age z-score <+1 SD, and as pre-obesity or obesity, if it was ≥+1 SD.
Parental education level was defined according to the parent that reported the highest level of education completed. The education levels ranged from none, 1st cycle of primary school (4th grade), 2nd cycle of primary school (6th grade), 3rd cycle of primary school (9th grade), secondary school (12th grade), post-secondary level (professional courses excluding higher education degrees), and tertiary level (higher education degrees, such as bachelor, master, and doctorate). Lastly, parental education level was re-categorised as post-secondary or lower level and tertiary level.
For each participant, the local clock times of sunrise and sunset in the first free day of the PA diaries were obtained using the function sunriseset of the R package maptools (Bivand and Lewin-Koh Citation2017) and the central coordinates of their NUTS II region. Daylight duration (h) was also calculated as the time interval between sunset and sunrise clock times.
Ethics approval and consent to participate
The IAN-AF 2015–2016 survey was conducted according to the guidelines of the Declaration of Helsinki and was approved by Ethical Committee of the Institute of Public Health, University of Porto, the Ethical Commissions of the Regional Administrations of Health involved and the National Commission for Data Protection.
All participants were asked to provide their written informed consent according to the Ethical Principles for Medical Research involving human subjects expressed in the Declaration of Helsinki and the national legislation. For children and adolescents (<18 y), the consent form was provided by the parents/legal representatives, which was also signed by adolescents (10–17 y). Documents with identification data were treated separately and stored in a different dataset.
Statistical analysis
Chronotype was categorised according to MSFsa tertiles (h:min) as Early (≤3:10 h), Intermediate (>3:10 h and < 3:56 h) and Late (≥3:56 h). Sample’s characteristics were analysed by chronotype. Continuous variables were considered to have a normal distribution by visual inspection of histograms and Q-Q plots. Frequency and proportion [n (%)] of categorical variables, or mean and SD of continuous variables, were compared between chronotype categories, using Pearson’s chi-squared test and one-way analysis of variance (ANOVA), respectively. Post-hoc tests were performed, namely Bonferroni adjusted Z-test for column proportions, and Tukey’s multiple comparisons test with Bonferroni correction for column means (or Games-Howell, if homogeneity of variances was not assumed).
Two separate linear regression analyses were performed between the categories of chronotype and each outcome: the HEI score (points) and the caloric midpoint (h). Summer holidays and daylight duration were tested as potential confounders in preliminary analyses, by assessing the association of each of them with chronotype categories (ordinal logistic regressions) and with the HEI score and caloric midpoint (linear regressions). When both were included in the models, only summer holidays remained significantly associated and thus, daylight duration was excluded.
In the ordinal logistic regression model, chronotype was considered a categorical variable with an associated order, since the three categories of chronotype are based on the MSFsa tertiles of the sample. An ascending order of chronotype categories was selected from Early, Intermediate and, lastly, Late category. For the independent variable summer holidays (no/yes), the reference category was no.
The presented models were adjusted for the following potential confounders: participants’ sex, age, parental education (model 1), model 1 plus BMI category, sports practice (model 2) and, model 2 plus summer holidays (model 3). Interaction terms between chronotype and BMI, age, sex and summer holidays were tested but lacked statistical significance. Multicollinearity was assessed by calculating the Variance Inflation Factor (VIF) values.
As a sensitivity analysis, the sample was stratified into the two strata of summer holidays (no/yes).
Since chronotype was based on tertiles of MSFsa of the sample, new tertiles for each stratum of summer holidays, were calculated and the respective chronotype categories were defined. For the stratum no (n = 412), Chronotype during school terms was defined as Early (≤3:04 h), Intermediate (>3:04 h and < 3:47 h) and Late (≥3:47 h). For the stratum yes (n = 166), Chronotype during summer holidays was defined as Early (≤3:34 h), Intermediate (>3:34 h and <4:33 h) and Late (≥4:33 h). For the stratum no, models 1 and 2 were performed, whereas for the stratum yes, only model 1 was conducted due to the smaller sample size.
For the linear regression models, the estimated unstandardized coefficients () and respective 95% confidence intervals (CI) are presented. For the ordinal logistic models, odds-ratio (OR) and 95% CI are presented.
A significance level of 0.05 was assumed for all analyses. R statistical computing program, version 3.4.1 (R Foundation for Statistic Computing, Austria, 2010) was used to calculate time parameters of sleep and energy intake. Statistical Package for the Social Sciences, version 27.0 (IBM SPSS Statistics for Windows, IBM Corp., Armonk, NY) was used for descriptive statistics and regression models, using Generalized Linear Models.
Results
Of the 578 included participants whose mean age was 10 y, most were girls (52%), had parents with tertiary education level (63%) and were underweight or had normal weight (64%). Participants with a Late chronotype were older, had a higher HEI score although differences were marginally significant (p < 0.068), and had a later daily energy intake onset, offset, 25% and 75% of TEI, than those with Early chronotypes (all p < 0.001). The same group had a later caloric midpoint (15:00 h ± 1:43 h) compared to those with Early (14:30 h ± 1:33 h) and Intermediate chronotypes (14:42 h ± 1:41 h) (p = 0.048). For participants with dietary assessments both on weekends and weekdays, there were no statistically significant differences of the mean caloric midpoint lag between chronotype categories (p = 0.450) ().
Table 1. Characteristics of participants, by categories of chronotype.
After adjustment for sociodemographic variables, BMI, and sports practice, a Late chronotype was negatively associated with the HEI (model 2: = −0.74; 95% CI: −1.44, −0.33) compared to an Early chronotype. However, after further adjusting for summer holidays, the association was no longer statistically significant (model 3:
= −0.47; 95% CI: −1.19, 0.25). Similarly, a Late chronotype vs. Early was positively associated with the caloric midpoint (model 2:
= 0.35; 95% CI: 0.02, 0.69). Nevertheless, after further adjusting for summer holidays, the direction of association was maintained but this association was no longer statistically significant (model 3:
= 0.25; 95% CI: −0.09, 0.60) ().
Table 2. Associations of chronotype with the healthy eating index score (points) and the caloric midpoint (h).
For the association between summer holidays (no/yes) and chronotype, the ordinal logistic equation model, adjusted for sociodemographic variables, revealed that participants in the group of summer holidays = yes had a higher odds ratio of being in a later chronotype category, compared to participants who were in the group of summer holidays = no (model 1: OR = 2.70; 95% CI: 1.90, 3.83) (Table S3).
The group summer holidays=yes was also negatively associated with the HEI (model 1: = −1.09; 95% CI: −1.70, −0.49) and positively associated with the caloric midpoint (model 1:
= 0.44; 95% CI: 0.15, 0.74) after adjusting for sociodemographic variables (Table S4).
The sensitivity analysis showed that within both stratum of summer holidays, the associations of chronotype during school terms and chronotype during summer holidays (for stratum no and for stratum yes, respectively) with both outcomes maintained the directions seen in the models before stratification but were not statistically significant (Table S5).
Discussion
Chronotype and diet quality
In the present study, a Late chronotype, compared to Early, was negatively associated with diet quality measured by the HEI score, after adjusting for sociodemographic, anthropometric and sport covariates.
Outcome measures related to diet quality vary from study to study, with some analysing food groups, and others using different dietary indexes, which hinders comparison of results. Also, studies did not account for summer holidays, and thus, models 1 and 2 will also be discussed.
Present findings agree with previous studies focused on the effect of bedtimes and rise times on healthy dietary indexes with food groups similar to those contained in the HEI. A study among Australian youngsters with mean age of 12 y, described that, compared to being “early-to-bed and early-to-rise,” being “late-to-bed and late-to-rise” was negatively associated with the score of the Dietary Guideline Index for Children and Adolescents (Golley et al. Citation2013). Likewise, a multinational study, comprising individuals aged 9 to 11 y, found that bedtime was inversely associated with a healthy dietary pattern (Chaput et al. Citation2015). Nonetheless, Fleig and Randler (Citation2009) were not able to find an association between chronotype and consumption of sweets or vegetables, in adolescents (Fleig and Randler Citation2009).
When further adjustment for summer holidays was added, the associations between late chronotype and HEI were no longer significant, thus suggesting summer holidays was a confounder.
Concerning the sensitivity analysis, in both strata of summer holidays (yes and no), the respective chronotype categories were not significantly associated with the HEI score. Nevertheless, it is possible that the smaller sample size obtained after stratification contributed to the lack of statistical significance. Amongst young populations, a prior study with a collection period only on summer holidays, found associations between a later midpoint of sleep and some food groups but not all, namely with a higher consumption of high-energy-dense and nutrient-poor foods (Thellman et al. Citation2017). Another work, during school terms, found an association between evening types and a lower intake of vegetables and fruits (Yu et al. Citation2020).
Chronotype and timing of energy intake
A recent scoping review in adults concluded that late/evening chronotypes were linked to consuming a greater proportion of daily TEI towards “later” in the day, frequently defined as after 20:00 (Phoi et al. Citation2022). In this sample, in comparison to Early chronotypes, Late chronotypes were associated with a later caloric midpoint, after adjusting for sociodemographic parameters, sports practice, and BMI. To the best of our knowledge, no study has yet performed such analysis in children and adolescents. Comparison with existing evidence is hindered by heterogeneity in the outcome definition and data collection period. Nonetheless, a later chronotype, defined by the highest MSFsc tertile, was associated with having a higher proportion of TEI after 18:00, in adolescents (10–18 y) (Roßbach et al. Citation2018).
Similar to what was observed for the HEI score, analyses suggest that summer holidays might also be confounding the association between chronotype and caloric midpoint.
Divergent results discussed in the literature regarding the association of chronotype with both outcomes might also be partially explained by heterogeneity in methodology and study’s population, as age and cultural factors can affect sleep and dietary habits.
The potential role of summer holidays
In the present study, being on summer holidays was positively associated with having a Late chronotype and a later caloric midpoint, whereas it was negatively associated with diet quality. When the main regression analyses where further adjusted for summer holidays, the previous associations of chronotype with diet quality and timing of energy intake lost statistical significance, albeit the direction of associations was maintained. Although this suggests that summer holidays had a confounding role, the limited sample size of participants in summer holidays and in school terms could have contributed for such results.
In this sample, summer holidays could have acted as a proxy of a variety of environmental and social factors that are associated with chronotype, diet quality and timing of energy intake (Zerbini et al. Citation2022). While the exact factors related to summer holidays cannot be disentangled in this study, possible factors explaining the described associations are explored.
Our findings are in line with the “Structured Days Hypothesis.” This hypothesis has been supported by subsequent studies (Weaver et al. Citation2020; Zosel et al. Citation2022) and advocates that, compared to school terms, summer holidays are a less formal setting that promotes obesogenic behaviours, such as later and irregular sleep and eating habits, increased screen times and greater food availability, including of caloric-rich foods, leading to an excessive weight gain (Brazendale et al. Citation2017). High caloric foods and poor-nutrient foods may be included in the Sweets, Salty snacks, and Sugar-sweetened beverages groups of the HEI used in our study. An increase in their consumption during summer holidays, would lead to a lower quality score during this period, contributing to the negative association between summer holidays and HEI score.
Although mechanisms are still unclear, another potential contributing factor for the association of summer holidays with a lower diet quality and a later timing of energy intake, is the circadian misalignment that could occur during this period (Moreno et al. Citation2019). Due to fewer social demands and an increased artificial light exposure later in the day, the sleep-wake cycle can become desynchronized with the light-dark cycle, which in turn can promote later eating habits (Moreno et al. Citation2019). Biologically, central and peripheral biological clocks may favour an earlier temporal energy distribution pattern (Flanagan et al. Citation2021). Chronodisruption of this pattern may lead to altered hormones levels and changes in the reward-motivational response, thus affecting food choice by favouring high palatable foods (Flanagan et al. Citation2021). Moreover, the DST and a longer photoperiod present during summer may also play a role in the delay of the sleep-wake cycle and a circadian misalignment, in ways that are still unclear, especially in children and adolescents (Zerbini et al. Citation2022). Additionally, as observed in this sample, Late chronotype-individuals compared to Early types, may have a later energy intake offset and a shorter sleep duration. This could contribute to having less appetite when waking up, postponement of meals, and therefore, a later caloric midpoint. Despite of this, the average sleep duration on weekends was not included as a covariate in the regression models since it was within the advocated sleep needs (Paruthi et al. Citation2016).
The interplay of chronotype, eating habits, social schedules and environmental factors during different periods of data collection, should be further investigated in large-scale studies in pediatric age, due to specifities in their circadian system (Zerbini et al. Citation2022).
Strengths and limitations
Strengths of this work include data from a harmonized dietary intake assessment method that captured seasonal variability (Hu Citation2002), and a healthy eating index adapted and validated for Portuguese children and adolescents. Additionally, the caloric midpoint might be a more comprehensive measure of the overall timing of energy intake, compared to meal timings or absolute energy intake after arbitrary time cut-offs. As limitations, the caloric midpoint might have not been representative of habitual behaviour, due to day-to-day instability. Despite this, there were no differences of the caloric midpoint lag between chronotype categories. Although the dietary intake assessment might have not occurred on the same days as the PA diaries, their time-gap is of 15 days or less.
In IAN-AF no validated instruments to estimate chronotype were applied, as it was beyond its scope. Here, chronotype was estimated by the MSFsa, which was calculated with the self-reported clock times of “sleeping,” on at least one weeknight plus one or two weekend nights of the PA diaries, which was the only type of available data. This is a subjective method which has yet to be used to estimate chronotype, similar to studies that have used isolated questions or sleep diaries (Fleig and Randler Citation2009; Silva et al. Citation2016), and thus, the validity cannot be assured (Phoi et al. Citation2022). As performed by other authors (Fleig and Randler Citation2009; Silva et al. Citation2016), the MSFsa was calculated assuming that, on weekend nights, sleep was not interfered by alarm clocks, parents or schedules, which might have not been true for all participants. While the cut-offs for MSFsa tertiles might have been underestimated, it is not expected this could have affected the results. The first and last “sleeping” times might have been better proxies of sleep onset and sleep end times, compared to bedtime and getting up time used in other works (Fleig and Randler Citation2009).
We performed data imputation methods to deal with missing first clock times of “sleeping”. Although this may have decreased data variability, we made efforts to minimize this effect, and we do not expect it has biased our results for the following reasons: (1) the cause of missingness was lack of consecutiveness between PA diaries, which was not related with chronotype nor dietary intake (missing at random); (2) frequency was similar on weekdays and weekends; (3) for each participant, we performed imputation separately for weekdays and weekends, ensuring that imputed values were based on the same type of weekday; (4) for each type of weekday, we do not expect a high intra-individual variability in bedtimes amongst 6–14-y-olds; (5) values imputed by mean prediction were all unique, ensuring variability of values.
Moreover, it cannot be excluded the possible effect of confounding variables not assessed in this study, including light exposure, consumption of stimulants and undiagnosed disorders. Lastly, although theoretical pathways linking summer holidays to chronotype and eating habits were discussed, the cross-sectional study design does not allow to establish causality between these variables. Generalization of results should consider aforementioned limitations and participants characteristics.
In conclusion, the present study showed that in Portuguese children and adolescents aged between 6 and 14 y, a later chronotype was negatively associated with diet quality and positively associated with caloric midpoint, although associations lost statistical significance after considering summer holidays. Albeit this may suggest that summer holidays might have confounded these associations, the sample size could have affected the results, which should be interpreted with caution. Therefore, to clarify the role of summer holidays on the association between chronotype and overweighted-related behaviours such as diet quality and timing of enery intake, further prospective and larger sample-sized studies are needed, that consider period of data collection. Moreover, summer holidays may represent a proxy for a combination of environmental and social factors, that can negatively influence eating habits and chronotype, higlighting the relevance to elucidate the underlying factors and mechanisms, specifically in peadiatric age.
Author contributions
SC conceptualized and designed the present study, acquired data from physical activity diaries, performed statistical analysis in SPSS, interpreted data, and wrote the manuscript with inputs from all authors. SV participated and supervised all the aforementioned steps. DC conducted data management in R and participated in the design of the methodology of the study, statistical analysis, and interpretation of data. All authors reviewed and approved the submitted manuscript.
Supplemental Material
Download PDF (487.7 KB)Acknowledgments
The IAN-AF 2015–2016 had institutional support from the General Directorate of Health (DGS), the Regional Health Administration Departments, the Central Administration of the Health System (ACSS) and the European Food Safety Authority (CFT/EFSA/DCM/2012/01-C03). The researchers acknowledge all these institutions and all people involved in all phases of the survey, as well as the participants.
We gratefully acknowledge Inês Sanches for the technical assistance in preliminary steps of this research, Professor Marta Garaulet (Ph.D.) and Professor Carla Lopes (Ph.D.) for the discussion about the theme of this manuscript.
Disclosure statement
No potential conflict of interest was reported by the author(s).
Data availability statement
The authors confirm that the data supporting the findings of this study are available within the article [Tables 1 and 2 present below] its supplementary materials [Separate word file with Tables S1–S5].
Supplementary material
Supplemental data for this article can be accessed online at https://doi.org/10.1080/07420528.2024.2339970
Additional information
Funding
References
- Almoosawi S, Vingeliene S, Gachon F, Voortman T, Palla L, Johnston JD, Van Dam RM, Darimont C, Karagounis LG. 2019. Chronotype: implications for epidemiologic studies on chrono-nutrition and cardiometabolic health. Adv Nutr. 10:30–42. doi: 10.1093/advances/nmy070.
- Bivand R, Lewin-Koh N 2017. Maptools: tools for reading and handling spatial objects. R package version 0.9-2. https://cran.r-project.org/package=maptools.
- Bouchard C, Tremblay A, Leblanc C, Lortie G, Savard R, Thériault G. 1983. A method to assess energy expenditure in children and adults. Am J Clin Nutr. 37:461–467. doi: 10.1093/ajcn/37.3.461.
- Brazendale K, Beets MW, Weaver RG, Pate RR, Turner-McGrievy GM, Kaczynski AT, Chandler JL, Bohnert A, von Hippel PT. 2017. Understanding differences between summer vs. school obesogenic behaviors of children: the structured days hypothesis. Int J Behav Nutr Phys Act. 14:1–14. doi: 10.1186/s12966-017-0555-2.
- Cagampang FR, Bruce KD. 2012. The role of the circadian clock system in nutrition and metabolism. Br J Nutr. 108:381–392. doi: 10.1017/S0007114512002139.
- Chaput J-P, Katzmarzyk PT, LeBlanc AG, Tremblay MS, Barreira TV, Broyles ST, Fogelholm M, Hu G, Kuriyan R, Kurpad A, et al. 2015. Associations between sleep patterns and lifestyle behaviors in children: An international comparison. Int J Obes Suppl. 5:S59–S65.
- de Onis M, Onyango A, Borghi E, Siyam A, Pinol A. 2006. WHO child growth standards: length/height-for-age, weight-for-age, weight-for-length, weight-for-height and body mass index-for-age. Methods and development. Geneva: World Health Organization; [accessed 2022 Oct 12]. https://www.who.int/publications/i/item/924154693X.
- Eng S, Wagstaff DA, Kranz S. 2009. Eating late in the evening is associated with childhood obesity in some age groups but not in all children: the relationship between time of consumption and body weight status in U.S. children. Int J Behav Nutr Phys Act. 6:27. doi: 10.1186/1479-5868-6-27.
- European Food Safety Authority. 2011. Report on the development of a food classification and description system for exposure assessment and guidance on its implementation and use. EFSA J. 9:1–84. doi: 10.2903/j.efsa.2011.2489.
- European Food Safety Authority. 2014. Guidance on the EU menu methodology. EFSA J. 12:1–80. doi: 10.2903/j.efsa.2014.3944.
- Flanagan A, Bechtold DA, Pot GK, Johnston JD. 2021. Chrono-nutrition: from molecular and neuronal mechanisms to human epidemiology and timed feeding patterns. J Neurochem. 157:53–72. doi: 10.1111/jnc.15246.
- Fleig D, Randler C. 2009. Association between chronotype and diet in adolescents based on food logs. Eat Behav. 10:115–118. doi: 10.1016/j.eatbeh.2009.03.002.
- Goios ACL, Severo M, Lloyd AJ, Magalhães VPL, Lopes C, Torres DPM. 2020. Validation of a new software eAT24 used to assess dietary intake in the adult Portuguese population. Public Health Nutr. 23:3093–3103. doi: 10.1017/S1368980020001044.
- Golley RK, Maher CA, Matricciani L, Olds TS. 2013. Sleep duration or bedtime? Exploring the association between sleep timing behaviour, diet and BMI in children and adolescents. Int J Obes. 37:546–551. doi: 10.1038/ijo.2012.212.
- Hu FB. 2002. Dietary pattern analysis: a new direction in nutritional epidemiology. Curr Opin Lipidol. 13:3–9. doi: 10.1097/00041433-200202000-00002.
- Instituto Nacional de Saúde Ricardo Jorge. 2006. Tabela da Composição dos Alimentos [Food Composition Table]. Lisboa: Instituto Nacional de Saúde Dr. Ricardo Jorge.
- Little RJA, Rubin DB. 2014. Statistical analysis with missing data. 2nd ed. New Jersey: John Wiley & Sons, Inc.
- Lopes C, Torres D, Oliveira A, Severo M, Guiomar S, Alarcão V, Ramos E, Rodrigues S, Vilela S, Oliveira L, et al. 2018. National food, nutrition, and physical activity survey of the Portuguese general population (2015–2016): protocol for design and development. JMIR Res Protoc. 7:e42. doi: 10.2196/resprot.8990.
- Lopes C, Torres D, Oliveira A, Severo M, Guiomar S, Alarcão V, Vilela S, Ramos E, Rodrigues S, Oliveira L, et al. 2017. National food, nutrition and physical activity survey of the Portuguese general population. EFSA support publ. 14. doi: 10.2903/sp.efsa.2017.en-1341.
- McHill AW, Phillips AJK, Czeisler CA, Keating L, Yee K, Barger LK, Garaulet M, Scheer FAJL, Klerman EB. 2017. Later circadian timing of food intake is associated with increased body fat. Am J Clin Nutr. 106:1213–1219. doi: 10.3945/ajcn.117.161588.
- Moreno JP, Crowley SJ, Alfano CA, Hannay KM, Thompson D, Baranowski T. 2019. Potential circadian and circannual rhythm contributions to the obesity epidemic in elementary school age children. Int J Behav Nutr Phys Act. 16:1–10. doi: 10.1186/s12966-019-0784-7.
- Paruthi S, Brooks LJ, D’Ambrosio C, Hall WA, Kotagal S, Lloyd RM, Malow BA, Maski K, Nichols C, Quan SF, et al. 2016. Consensus statement of the american academy of sleep medicine on the recommended amount of sleep for healthy children: methodology and discussion. J Clin Sleep Med. 12:1549–1561.
- Phoi Y, Rogers M, Bonham M, Dorrian J, Coates A. 2022. A scoping review of chronotype and temporal patterns of eating of adults: Tools used, findings, and future directions. Nutr Res Rev. 35:112–135. doi: 10.1017/S0954422421000123.
- Pinto da Costa M, Durão C, Lopes C, Vilela S. 2019. Adherence to a healthy eating index from pre-school to school age and its associations with sociodemographic and early life factors. Br J Nutr. 122:220–230. doi: 10.1017/S0007114519001028.
- Rodríguez-Cortés FJ, Morales-Cané I, Rodríguez-Muñoz PM, Cappadona R, De Giorgi A, Manfredini R, Rodríguez-Borrego MA, Fabbian F, López-Soto PJ. 2022. Individual circadian preference, eating disorders and obesity in children and adolescents: a dangerous liaison? A systematic review and a meta-analysis. Children. 9:167. doi: 10.3390/children9020167.
- Roenneberg T, Pilz LK, Zerbini G, Winnebeck EC. 2019. Chronotype and social jetlag: a (self-) critical review. Biology (Basel). 8:1–19. doi: 10.3390/biology8030054.
- Roenneberg T, Wirz-Justice A, Merrow M. 2003. Life between clocks: daily temporal patterns of human chronotypes. J Biol Rhythms. 18:80–90. doi: 10.1177/0748730402239679.
- Roennenberg et al. 2015. Munich Chronotype Questionnaire (MCTQ) for children and adolescents – MCTQ, english, version 2015-01. 1–4. [accessed 2022 Oct 4]. https://www.thewep.org/documentations/mctq/item/english-mctq-full-children.
- Roßbach S, Diederichs T, Nöthlings U, Buyken AE, Alexy U. 2018. Relevance of chronotype for eating patterns in adolescents. Chronobiol Int. 35:336–347. doi: 10.1080/07420528.2017.1406493.
- Silva CM, Mota MC, Miranda MT, Paim SL, Waterhouse J, Crispim CA, Mendes C, Mota MC, Miranda MT, Paim SL, et al. 2016. Chronotype, social jetlag and sleep debt are associated with dietary intake among Brazilian undergraduate students. Chronobiol Int. 33:740–748.
- Stewart AD, Marfell-Jones MJ. 2001. International standards for anthropometric assessment. Potchefstroom: International Society for the Advancement of Kinanthropometry.
- Teixeira GP, Barreto ACF, Mota MC, Crispim CA. 2019. Caloric midpoint is associated with total calorie and macronutrient intake and body mass index in undergraduate students. Chronobiol Int. 36:1418–1428. doi: 10.1080/07420528.2019.1652830.
- Thellman K, Dmitrieva J, Miller A, Harsh J, LeBourgeois M. 2017. Sleep timing is associated with self-reported dietary patterns in 9- to 15-year-olds. Sleep Heal. 3:269–275. doi: 10.1016/j.sleh.2017.05.005.
- Torres D, Faria N, Sousa N, Teixeira S, Soares R, Amorim H, Guiomar S, Lobato L, Oliveira C, Correia D, et al. 2017. Inquérito Alimentar Nacional e de Atividade Física, IAN-AF 2015–2016: Manual Fotográfico de Quantificação de Alimentos [National Food, Nutrition and Physical Activity Survey of the Portuguese population, 2015–2016: Photographic Manual for Food Quantifica]. Universidade do Porto. editor. p. 241. [accessed 2022 Oct 10]. www.ian-af.up.pt.
- Vilela S, Muresan I, Correia D, Severo M, Lopes C. 2020. The role of socio-economic factors in food consumption of Portuguese children and adolescents: results from the national food, nutrition and physical activity survey 2015-2016. Br J Nutr. 124:591–601. doi: 10.1017/S0007114520001373.
- Vilela S, Oliveira A, Severo M, Lopes C. 2019. Chrono-nutrition: the relationship between time-of- day energy and macronutrient intake and children’s body weight status. J Biol Rhythm. 34:332–342. doi: 10.1177/0748730419838908.
- Weaver RG, Armstrong B, Hunt E, Beets MW, Brazendale K, Dugger R, Turner-McGrievy G, Pate RR, Maydeu-Olivares A, Saelens B, et al. 2020. The impact of summer vacation on children’s obesogenic behaviors and body mass index: a natural experiment. Int J Behav Nutr Phys Act. 17:1–14. doi: 10.1186/s12966-020-01052-0.
- World Health Organization. 2006. Food and nutrition policy for schools: a tool for the development of school nutrition programmes in the European Region. Copenhagen: World Health Organization Regional Office for Europe. https://apps.who.int/iris/handle/10665/107797.
- Yu B-M, Yeung WF, Ho YS, Ho FYY, Chung KF, Lee RLT, Lam MY, Chen S. 2020. Associations between the chronotypes and eating habits of Hong Kong school-aged children. Int J Environ Res Public Health. 17:2583. doi: 10.3390/ijerph17072583.
- Zerbini G, Merrow M, Winnebeck EC. 2022. Weekly and seasonal variation in the circadian melatonin rhythm in humans: a response. J Pineal Res. 72:e12777. doi: 10.1111/jpi.12777.
- Zosel K, Monroe C, Hunt E, Laflamme C, Brazendale K, Weaver RG. 2022. Examining adolescents’ obesogenic behaviors on structured days: a systematic review and meta-analysis. Int J Obes (Lond). 46:466–475. doi: 10.1038/s41366-021-01040-9.