Abstract
Little is known about what features of AAC systems are regarded by AAC professionals as more suitable for children with different characteristics. A survey was conducted in which participants rated the suitability of hypothetical AAC systems on a Likert scale from 1 (very unsuitable) to 7 (very suitable) alongside a discrete choice experiment. The survey was administered online to 155 AAC professionals in the United Kingdom of Great Britain and Northern Ireland. Statistical modeling was used to estimate how suitable 274 hypothetical AAC systems were for each of 36 child vignettes. The proportion of AAC systems rated at least 5 out of 7 for suitability varied from 51.1% to 98.5% for different child vignettes. Only 12 out of 36 child vignettes had any AAC systems rated at least 6 out of 7 for suitability. The features of the most suitable AAC system depended on the characteristics of the child vignette. The results show that, while every child vignette had several systems that had a good suitability rating, there were variations, that could potentially lead to inequalities in provision.
Augmentative and alternative communication (AAC) can improve the lives of many people with communication difficulties (Hajjar et al., Citation2016; Ryan et al., Citation2015; Schlosser & Wendt, Citation2008). For children, provision of AAC is especially important, as it can affect their social participation as well as their development and learning, having an impact on the rest of their lives (Lund & Light, Citation2006; Ryan et al., Citation2015). In recent years, the expectations of people who use AAC to participate in all aspects of society has increased (Hemsley & Murray, Citation2015; Hynan et al. Citation2015; Light, McNaughton et al. Citation2019; Sundqvist & Rönnberg, Citation2010; Williams et al., Citation2008, Citation2012).
Many different AAC systems exist, with very different features. Children may benefit from AAC due to a wide range of reasons and may have a variety of conditions such as cerebral palsy and autism spectrum condition. Children with the same diagnosis each have disparate needs, abilities, and personal circumstances. Selecting a suitable AAC system for a child is thus a highly complex task, requiring the balancing of many different competing concerns, and the process is unique to each child (Dietz et al., Citation2012; Lund et al., Citation2017; Lynch et al., Citation2019). For example, matching might involve assessing whether a child is more motivated by vocabulary represented by photos or text. This consideration would have to be balanced against which mode of graphical representation would better suit the child’s future needs given their progress. For more details, see Beukelman and Light (Citation2020).
Research has revealed some important factors in AAC professionals’ decision-making such as a child’s preferences and family circumstances (Enderby et al., Citation2013; Geytenbeek et al., Citation2015; Thistle & Wilkinson, Citation2015). Assessing the cognitive demands of a given AAC system places on a child is another important factor (Fried-Oken et al., Citation2019). There are also guidelines for how AAC services should be organized (Choi & Pak, Citation2006; National Health Service (NHS) England, 2016; Royal College of Speech and Language Therapists, Citation2009). Some research has been conducted on AAC professionals’ decision-making. For example, it has been shown that professionals with different backgrounds (Dietz et al., Citation2012) and levels of experience (Sauerwein & Wegner, Citation2020) differ in their consideration of factors. Still, little is known about the details of how AAC professionals make decisions, or what features lead AAC professionals to judge AAC systems as a suitable match for children with different characteristics (Dietz et al., Citation2012; McFadd & Wilkinson, Citation2010; Ryan et al., Citation2015).
The current study examined AAC professionals’ judgment and decision-making for children, specifically how suitable they believe different AAC systems are for different children. (By AAC system we refer in this manuscript to aided systems which may be either high-tech or low-tech.) Examining AAC professionals’ judgment and decision-making addresses the previously noted knowledge gap and allows an examination of whether a gap exists between research and practice. The study also makes it possible to reflect on how improvements may be made to current practice and service structures.
The current study is part of three linked studies that used different methods to address the topic of AAC professionals’ judgment and decision-making from different angles. The principal research question for the first study (Webb et al. Citation2019b) was: What is the relative importance of AAC system attributes and child characteristics in AAC professionals’ decision-making in daily practice? The study revealed how important different factors were in AAC professionals’ daily practice; in other words how important specific factors were averaged over the case-mix AAC professionals see. For example, it revealed that interface-related AAC system attributes were relatively more important than hardware attributes in professionals’ daily practice. Averaged over the case-mix that they saw, participants also judged children’s cognitive and learning abilities to be more important than their physical features. The second study (Webb et al., Citation2019a) had the principal research question: When choosing an AAC system, what tradeoffs do AAC professionals make between system attributes, and how do such tradeoffs change depending on the characteristics of the child? This study revealed how AAC professionals make decisions when choosing for an individual child. For example, it showed that children’s motivation to communicate using AAC and predicted future abilities had a greater influence on how participants traded-off different AAC system attributes than their language ability and previous experience with AAC.
Finally, the current study has the principal research question: How do AAC professionals judge the suitability of different AAC systems to be for a child, and how do their judgements change depending on child characteristics? This study adds information about strength of preference, revealing not only what AAC system an AAC professional would choose for a child, but also how suitable they believe the system to be for that child.
The context for the current study is the UK, where it is estimated that 1 in 200 children could benefit from AAC (Enderby et al., Citation2013; Gross, Citation2010; Judge et al., Citation2017). There is some variation across the UK in how children are allocated AAC systems, but in general children’s needs, abilities and circumstances are assessed by a multidisciplinary team of AAC professionals. Final recommendations are made with input from children and their support network about their preferences and opinions about the potential options. The composition of the multidisciplinary team varies, and can include speech and language therapists, occupational therapists, and teachers (Lynch et al., Citation2019; NHS England, Citation2016).
The current study was part of a wider research project entitled Identifying Appropriate Symbol Communication aids for children who are non-speaking: enhancing clinical decision-making (I-ASC) (Murray et al., Citation2020). The project used a variety of research methods (Judge et al., Citation2020; Lynch et al., Citation2019; Murray et al., Citation2019) to study AAC provision for children in the UK. The evidence produced by the project has been used in the creation of a suite of resources for AAC professionals and other stakeholders to support best practice, which is available for free at https://iasc.mmu.ac.uk/. See for a schematic overview of the relations between the current study and the different components of the I-ASC project.
Figure 1. Schematic representation of the relationship between the current study and the wider I-ASC project.
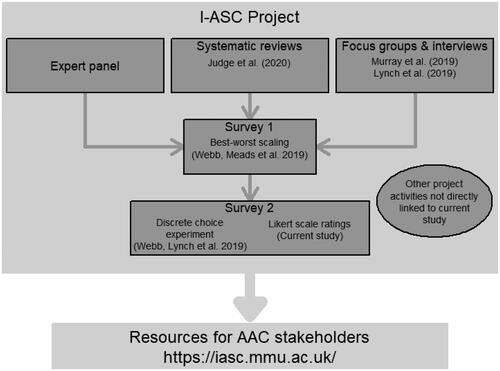
The current study contributed to the wider project by showing how suitable AAC professionals judged different AAC systems to be for children with different characteristics. It examined whether, in the opinion of survey participants, there was an AAC system which suited the needs of every individual child. In addition, it complemented investigations as to what systems AAC professionals would choose, as they may not necessarily choose what they believe to be the most suitable AAC system for a child. For example, the most suitable AAC system may not be chosen due to resource constraints such as cost or instruction time.
Method
This study used data collected from the same participants as Webb et al. (Citation2019a), as well as the same procedures.
Research design
The study used a survey design that combined a discrete choice experiment (DCE) and Likert scale ratings. The current study analyzed data from the Likert scale tasks which were asked after each DCE task in which participants rated how good a match an AAC system was for a child vignette on a scale from 1 (very unsuitable) to 7 (very suitable). The current study is concerned with cardinal measures of an AAC system’s suitability for a child.
Likert scale ratings allowed the research question of how suitable a match different AAC systems are for different children to be addressed. The innovative method of combining a Likert scale with a DCE enabled the estimation of suitability ratings for many more AAC system-child combinations than would be feasible for survey participants to rate individually.
The study received ethical approval from an NHS Research Ethics Committee (REC reference 6/NW/0165). Participants gave informed consent.
Participants
The target population was AAC professionals working in the UK who contributed to AAC decision-making for children. Participants were recruited using the email lists of the I-ASC project and Communication Matters (a UK-wide AAC charity and chapter of the International Society for Augmentative and Alternative Communication), as well as project members’ personal contacts. In addition, the survey was advertised on the I-ASC project’s website and social media. The survey was open for responses from 20 October 2017 until 4 March 2018.
A total of 172 people submitted complete responses, of which 155 completed the DCE and Likert scale tasks. Participant demographics are summarized in . The demographics of participants who did not complete the DCE or Likert scale tasks were largely similar to those who did; however, they were slightly older on average, at 46 years, and were more likely to have a professional background as an occupational therapist (n = 5, 29.4% compared to n = 11, 7%) than a background in speech and language therapy (n = 8, 47.1% compared to n = 117, 75.5%).
Materials and measures
The study used a discrete choice experiment survey with additional Likert scale questions. The survey development and administration are described in the section that follows.
Procedures
In Webb et al. (Citation2019b), lists of 18 AAC system attributes and 19 child characteristics were constructed using information from systematic reviews of the literature (Judge et al., Citation2020), input from an expert panel as well as focus groups and interviews with AAC professionals, people who use AAC, their families and other stakeholders (Lynch et al., Citation2019; Murray et al., Citation2019). These lists were then included in a survey that used a method called best-worst scaling Case 1 (BWS) (Cheung et al., Citation2016) and that was administered to 93 AAC professionals.
From the BWS attributes, five AAC system attributes and four child attributes were selected for inclusion in a DCE/Likert scale survey based on the following criteria: (a) attributes formed coherent descriptions of AAC systems/children, (b) attributes reflected the specific aims of the I-ASC project, (c) most attributes were of high relative importance according to the BWS survey results, and (d) the number of attributes was not so large as to overburden DCE survey respondents. The final list of attributes and levels for the DCE is given in .
Table 1. Attributes and levels for discrete choice experiment and likert scale survey.
Table 2. Participant demographics (N = 172).
The statistical design of the DCE was constructed using Ngene (ChoiceMetrics). This software package selected a design that maximized D-efficiency, which may be thought of as a measure of how much information it is possible to extract from survey responses (Kuhfeld et al., Citation1994). The design had 60 tasks which were divided into five blocks of 12. Each participant was randomly allocated to answer a block of 12 questions, with random allocations of blocks and child vignettes independent of each other. It was possible to form 54 child vignettes and 432 AAC systems from the sets of attributes. A total of 18 child vignettes and 158 AAC systems were identified as representing unrealistic combinations and excluded from being used in the survey according to the judgements of authors with AAC expertise (an example is that it would be unrealistic to have a vocabulary set with staged progression with fewer than 50 vocabulary items).
The survey was piloted with five AAC professionals. In response to feedback, small changes were made to wording and visual presentation to improve clarity. Piloting revealed that some AAC professionals did not have enough input into decision-making in their daily practice to meaningfully engage with the DCE/Likert scale tasks. To address this, at the beginning of the survey, participants answered the question “I confirm my work involves assessing children for aided AAC systems and I contribute to the decision-making in relation to the language and vocabulary organization within AAC systems.” Those who responded no were directed to answer only demographic questions and were not shown the DCE or Likert scale tasks.
The survey was administered online by a market research company. Before starting the survey, participants were given instructions, including attributes/characteristics and levels descriptions. In each DCE task, participants were shown a child vignette formed from the set of child attributes. For example:
Child A has receptive language exceeding expressive language. Child A is able to use AAC for a few communicative functions. Child A is motivated to communicate through symbol communication systems. Child A is predicted to plateau in skills and abilities.
Statistical Analysis
Analysis of responses used a random utility theory framework (Louviere et al., Citation2000) that assumed individuals assigned a utility to each option. The utility of each option was modeled as depending partly on the attributes of AAC systems as well as having a random component, representing all aspects of decision-making not explicitly captured by the model. Individuals were then assumed to choose the AAC system with the highest utility, and rated AAC systems higher if they had a higher utility.
Ratings and choices were analyzed jointly using choice-ordered logit models (Webb & Hess, Citation2021) that had a set of parameters representing how individuals made their decisions. Statistical techniques were used to find the parameters that maximized the probability of observing the choice and ratings participants made. The full model with parameters for every interaction between AAC system and child attributes had too many parameters to estimate robustly. Therefore, an iterative process was used in which a series of models with only one parameter were estimated. The parameter that contributed most to explaining how participants made their decision was selected for inclusion. A further series of models with two parameters were then estimated, and again the parameter that contributed most to explaining participants’ decision-making was selected. This continued until all parameters were included. The final model was then selected using the Akaike information criterion (Akaike, Citation1974), a measure of how well a model fits a dataset (see , Supplemental file, for technical details of the model estimation).
The final model was used to predict participants’ ratings for every AAC system for every child vignette. It was then calculated for each child vignette what percentage of AAC systems had a rating of at least 5 out of 7, and what percentage had a rating of at least 6 out of 7. All model estimation was carried out using the Apollo choice modeling package for R (Hess & Palma, Citation2019).
Results
The raw results for model estimation are given in , Supplemental file. gives for each child vignette the percentage of all 274 AAC systems included in the survey that were rated above 5 and above 6. All child vignettes had at least 51.1% of AAC systems rated above 5, and for 19 out of 36 this percentage was above 90%. For 24 out of 36 child vignettes, no AAC system was rated at 6 or above. However, some child vignettes had a range of AAC systems rated at least 6, for example; five vignettes had over 10% of AAC systems rated at least 6; and one vignette had over 20%.
Table 3. For each child vignette, the proportions of systems rated at least 5 and at least 6.
Of the 24 child vignettes without an AAC system, 11 rated 6 or higher were predicted to regress in skills and abilities, whereas eight were predicted to plateau and four were predicted to progress. In contrast, out of the 12 vignettes with an AAC system rated at least 6, seven were predicted to progress in skills and abilities, four to plateau and one was predicted to regress. All but one of the 12 child vignettes with at least one AAC system rated 6 or above for suitability were motivated to communicate using AAC.
shows how the most suitable AAC systems for each child vignette were rated. The vignette “delayed receptive and expressive language, no AAC experience, not motivated to communicate by any means, expected to regress in skills and abilities” had the lowest rated most suitable AAC system, at 5.62. The vignette “receptive language exceeding expressive language, experience of using AAC for a range of functions, motivated to communicate using AAC, expected to progress in skills and abilities” had the highest rated most suitable system, at 6.62. The difference of 1 between the ratings of the most suitable AAC system represents 14.3% of the available scale from 1 to 7.
Descriptions of what the most suitable AAC systems were for each vignette are given in and , Supplemental file. The results are summarized in , which illustrates how often a given AAC system feature was part of a child vignette’s most suitable system. Vocabulary sets with staged progression were a feature for 21 out of 36 child vignettes. Only a single child vignette had no pre-provided vocabulary set as a feature of a most suitable AAC system. Having fewer than 50 items was only seen as a feature of the most suitable AAC systems for two child vignettes.
Figure 4. Number of times each AAC system level was part of a child vignette’s most preferred system.
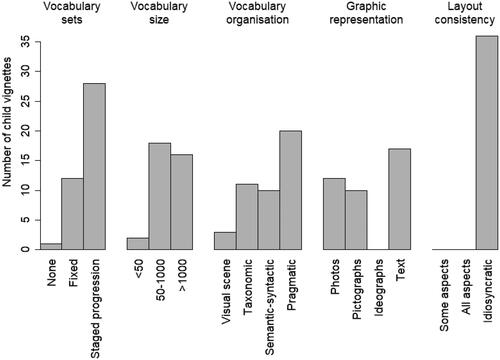
For most child vignettes (20), the highest rated AAC system had pragmatic vocabulary organization, with the most suitable system having visual scene organization for only two child vignettes. When photos were a feature of a most suitable AAC system, this was associated with lower ratings for those systems, in contrast to text, which was associated with higher rated most suitable AAC systems. Ideographs were not a feature of the most suitable AAC system for any child vignette. An idiosyncratic layout was a feature of the most suitable AAC system for all child vignettes.
Discussion
The results show that participants rated the suitability of AAC systems differently depending on the characteristics of the child vignette they were presented with. There was considerable variation in the fraction of AAC systems that were highly rated, and the features of the most suitable AAC systems varied for different child vignettes. This is not surprising, as it is in line with the analysis of participants’ choices (Webb et al., Citation2019a) and with previous findings in the literature (Johnson et al., Citation2006; Light & McNaughton, Citation2014); however, it is an encouraging sign of the face validity of the current study’s approach.
Methods used in the current study allowed for the calculation of how participants rated the suitability of 274 AAC systems for each of 36 child vignettes; this, in turn, allowed a comparison between child vignettes in terms of what fraction of AAC systems were rated above 5 and above 6; however, the set of AAC systems used in this survey was not intended to be representative of the characteristics of AAC systems currently available on the market. There may be no available system matching a given description, or there may be several different models all having features matching the description; thus, for example, if participants rated 50% of AAC systems in this survey at least 5 out of 7 for suitability, it does not mean they would give 50% of currently available AAC systems a similar rating. It follows that the proportion of AAC systems suitable for a given child vignette reported here may not reflect the range of suitable systems that AAC professionals would consider choosing between in daily practice. Yet, despite this caveat, the AAC systems presented were considered to be feasible, whether or not they were available “off the shelf”, so the results of the current study do give an indication of the relative numbers of possible AAC systems that were regarded as acceptable or good for different children.
If an average rating of 5 of more out of 7 is taken as good in terms of suitability, and 6 out of 7 taken as excellent, then for all child vignettes at least half of AAC systems were a good fit (the reader may instead choose to interpret 5 as an acceptable rating and 6 as good, for example, but the meaning of our discussion is unchanged); however, there was still much variation in the number of AAC systems that were a good fit, from a low of 51.1% to a high of 98.5%. In addition, more variation is revealed in terms of excellent systems. Most child vignettes had no AAC systems that were an excellent fit, yet for one child vignette (which could in some ways be considered to have the strongest prognosis for improvement) 20.4% of AAC systems were considered excellent for suitability. For many child vignettes almost all AAC systems were a good fit, yet only a small fraction were an excellent fit.
One possible interpretation of most systems being rated good for most child vignettes is that there was a weak underlying decision-making rationale. This interpretation would also be consistent with only a small number of vignettes having AAC systems rated over 6: in most cases no stand-out system (and thus decision rationale) emerged. An alternative explanation for these observations is that participants rated the suitability of a given AAC system in the context of the alternative being no system. If they believed “some communication is better than none”, then this may have led to most AAC systems being rated as good. A further interpretation is that the AAC system attributes used in this study are relatively unimportant compared to other attributes not included, although the extensive attribute development process weighs against this possibility. One additional reason behind the finding that many AAC systems were rated good, but relatively few were rated excellent, is participants’ interpretation of a “suitable match” for a child vignette. It may be that participants interpreted suitable, especially on the upper half of the Likert scale, as meaning adequate. This could have led to participants rating many AAC systems similarly, rather than making distinctions between an adequate and optimal match. If participants did approach the Likert scale tasks in that way, it would lead to problems in interpreting the study’s results as giving cardinal information about strength of preference. However, examining participants’ responses reveals that they are consistent with them distinguishing between, say, 5 being an adequate match and 7 being an optimal match of AAC system to a child vignette. For child vignettes which may broadly be described as more motivated to communicate via AAC, and with a strong prognosis for improvement, participants tended to rate most AAC systems as at least good, but clearly distinguished some systems as being a better match for their needs, indicating participants saw a difference between adequate and optimal matching. In addition, suitability ratings of AAC systems with greater learning demands (large vocabulary, semantic-syntactic organization, etc.) for more challenging child vignettes were generally lower than the suitability ratings of basic AAC systems for children motivated to use AAC and with strong prognoses for improvement. This later observation is consistent with participants distinguishing between poor and adequate matches for child vignettes.
Motivation to communicate using AAC and prognosis
The vignettes where there were AAC systems ranked over 6 could broadly be described as those where the child was described as more motivated to communicate via AAC and with stronger prognoses for improvement, that implies that there is at least the potential for inequalities in AAC provision to arise. For some children, fewer AAC systems are well suited to them, so that barriers to accessing some systems, such as cost or requiring a large amount of AAC practitioner input to set up, may disproportionately affect them, compared to children for whom many AAC systems are suitable. In light of this finding, it is encouraging that some dedicated funding for AAC systems is available, and Webb et al. (Citation2019b) found that UK AAC professionals ascribed low importance to cost in their decision-making. However, other evidence suggests cost can play a significant role in AAC professionals’ decision-making in other countries (Van Niekerk et al., Citation2018), and future research could usefully address the extent to which this leads to inequalities in AAC provision.
Previous evidence has shown a need for lower learning demands of AAC systems for some children (Light et al., Citation2019; Light, Wilkinson, et al., Citation2019). This need may have led participants to rate only AAC systems with low learning demands highly for some children, explaining some of the observed variation in the number of systems with high suitability ratings. In particular, it is a plausible explanation as to why child vignettes predicted to regress in skills and abilities had fewer AAC systems with high suitability ratings.
In line with the previous observation, graphic representation using photos, considered to have lower learning demands, were commonly a feature of the most suitable AAC system for child vignettes predicted to regress in skills and abilities and without motivation to communicate using AAC. Photos were associated with having a lower rated most suitable AAC system, and text, with greater learning demands, was associated with having a higher rated most suitable AAC system. The implication is that for children who require an AAC system with low learning demands, not only were there fewer systems that were a good match, even the most suitable systems were not an ideal match.
Another factor in how many AAC systems were given high suitability ratings was whether a child vignette included “was motivated to communicate using AAC” or not. For vignettes in which the child was motivated to communicate using AAC, many more AAC systems tended to be rated as good or excellent for suitability. Such motivation was also an important factor in the DCE results, where it led participants to make what could be regarded as more ambitious choices, for example a large vocabulary, or graphic representation using ideographic symbols rather than photos. The current study has given context to this finding by suggesting that, although motivation to communicate using AAC was an important determinant of participants’ choices, the consequences of such choices were not necessarily large, as participants regarded many less preferred AAC systems as well suited for motivated children. These findings are in line with previous evidence that attitudes toward AAC, and valuing an AAC system are important factors in successfully adopting AAC (Johnson et al., Citation2006; Light & McNaughton, Citation2014), so that children motivated to use AAC are still likely to succeed, even with an AAC system that is not a perfect match.
Most suitable AAC systems
The analysis also reveals which AAC systems participants regarded as most suitable for each child vignette. A caveat is that, although combinations of attributes which were regarded as unrealistic were excluded by the research team, there is no guarantee that for each AAC system included in the current study, an AAC system exists in the real world that has similar characteristics. There were significant differences between child vignettes in terms of how highly rated the most suitable AAC systems were, up to 14.3% of the rating scale’s available range. This may reflect that, for some children the best available AAC system was not as suitable to their needs and abilities as for other children. However, previous findings have shown that personalizing an off-the-shelf AAC system is an important factor in whether a child successfully adopts it (Dietz et al., Citation2012; King et al., Citation2008; Light & McNaughton, Citation2013). Thus it may be that participants would have given similar ratings to the most suitable AAC system for all child vignettes if it was clear that they would be personalized to the individual child.
shows the number of times each AAC system level was part of a child vignette’s most preferred system, and certain characteristics appeared much more often than others. For example, very few child vignettes had a highest rated AAC system with fewer than 50 vocabulary items. This is in line with findings from the DCE, which showed that participants were always more likely to choose AAC systems with more than 50 vocabulary items than systems with fewer than 50, regardless of the child vignette they were choosing for. The result is also consistent with few child vignettes having no pre-provided vocabulary sets and many having staged progression as a feature of their most suitable AAC system. A roughly even number of child vignettes had 50–1000 and over 1000 words as part of their most suitable AAC system, so it was not the case that participants believed that more vocabulary items were always better.
Visual scene was the most preferred mode of vocabulary organization for few child vignettes, yet visual scene displays are increasingly common (Beukelman et al., Citation2021; Wilkinson et al., Citation2012). This is not necessarily a contradiction, as the child vignettes used in this study are intentionally not representative of the population of children who would benefit from AAC. Rather, they represent a wide range of characteristics. Thus, it may be that AAC professionals encounter many children with characteristics similar to the vignettes where visual scene is most suitable, and fewer children similar to other vignettes.
All most preferred systems featured an idiosyncratic layout. This uniformity of opinion is surprising. One possible explanation is a form of measurement error. The statistical model had a limited number of parameters to avoid overfitting, and it may be that this resulted in some child vignettes appearing to have idiosyncratic as their most suitable layout, whereas in fact participants would have rated an alternative more highly. An alternative explanation is that in the context of the survey, any degree of layout customization was classed as idiosyncratic, whereas in practice most AAC professionals wouldn’t necessarily think of them that way. In any case, this unusual finding should be investigated further.
Comparison with other findings
Although DCEs are common in healthcare (Soekhai et al., Citation2019), this is the first study we are aware of that combines choices with ratings. An advantage of this approach is that it gives more information and makes it possible to answer other research questions than with a standard DCE with low extra participant burden and minimal additional resources to gather the data. The current study’s novel methods gave quantitative insight into current practice around UK AAC professionals’ decision-making. Particularly, it and the two linked survey studies address the concept of feature matching, i.e., matching the characteristics of the child with the most relevant AAC system attributes. Their findings can be linked to evidence from the wider project: Participants included in other elements of the I-ASC study (e.g., Lynch et al., Citation2019; Murray et al., Citation2019) described how they considered and made tradeoffs across the decision-making process. At a feature matching level this included consideration of particular child characteristics, for example the child’s motivation to communicate, their abilities to learn or their likely decline in learning capacity. Regarding AAC system attributes, we found consideration of the child’s physical and cognitive characteristics. This included communication aid size and weight, which were important for very small children or for children who were ambulatory. Communication aid appearance, voice quality, and reliability were also salient features. The software attributes prioritized reflected both the needs of the child and those providing support.
Implications
The different components of research resulted in a general explanatory model of decision-making in AAC for children. Full details are given in Murray et al. (Citation2020), and a schematic representation is given in . The I-ASC explanatory model will aid and inform practice by providing a conceptual overview of the linked aspects of decision-making and the different components of AAC provision for children. It will also stimulate future research by highlighting areas where we currently lack understanding and empirical evidence.
Figure 5. The I-ASC explanatory model of decision making. From Murray et al. (Citation2019).
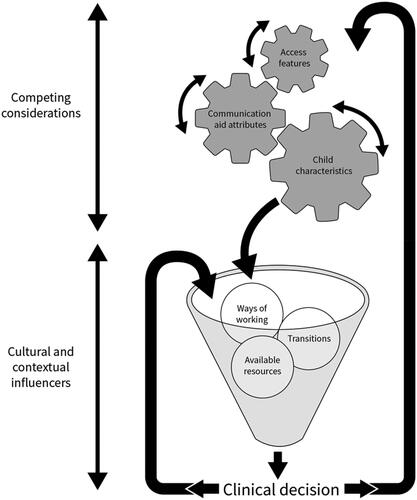
In summary, the I-ASC exploration concluded that those charged with the responsibility for proposing specific communication aids face a complex task that includes identifying the particular child characteristics, access features, and communication aid attributes. A key lesson for practice is that these must be considered in the recommendations for each child. The challenge is that these are not separate, fixed components of the decision-making process, but are constantly moving, with some being more fluid and others more stable depending on context as teams reach their decisions.
Limitations and future directions
It is possible to calculate a numerical rating for each AAC system and to test whether any differences are statistically significant. It is not possible, however, to know how meaningful participants considered to be the difference between, for example, an AAC system rated 5 out of 7 and one rated 6 out of 7. It may be that they considered two such AAC systems to be very similar, or they could have believed that the higher rated system would have a significantly positive effect on a child’s future for many years. Future studies using a similar method may wish to investigate giving participants guidance as to how to interpret a unit difference in the rating scale.
The ratings for AAC systems are derived from statistical modeling of a limited number of choices for each individual, and so participants may have given different responses if the context was changed to rating an AAC system directly; however, with 36 child vignettes and 274 AAC systems, rating every system for every vignette would have required participants to complete 864 rating tasks, which is unfeasible. In addition, DCE choices and ratings were gathered at the same time and participants’ ratings may have been influenced by the previous choice task; however, incorporating the Likert scale as part of a DCE had many practical advantages, as discussed above. In addition, recruiting participants to both the BWS and DCE surveys was difficult given the low numbers of AAC professionals in the UK, estimated at around 800 (Communication Matters, private correspondence). Thus it was uncertain whether recruiting participants for a third survey would be practical.
In common with all stated preference studies, there is concern about the ecological validity of the survey instrument and whether it captured the relevant aspects of the decision-making situation. However, the survey went through an extensive development process (Webb et al., Citation2021) to help create as realistic a scenario as possible, and which captured the most important aspects of decision-making. A related limitation is that we could include only a subset of the many characteristics that influence how suitable a match an AAC system is for a child. Thus, for example, while we provide information on systematic organization and representation, we did not include features related to access. In addition, the survey measures what AAC system attributes participants believed would match well with children. It does not necessarily capture the effectiveness of the sort of AAC systems suggested here to be a suitable match for children, and empirical research into whether AAC professionals’ beliefs match up with real-world effectiveness would be useful to investigate in future.
It was previously mentioned that the set of AAC systems used in the survey was not necessarily reflective of AAC systems available on the market. Although all systems were feasible, many would not be available off the shelf, and in practice would require practitioners to adapt an existing AAC vocabulary set. The skills, willingness and culture of doing this is likely to vary across practice settings. Additionally the availability of AAC systems will vary from place to place (particularly across countries) and the AAC systems and vocabularies placed on the market will change over time.
This study had a relatively low sample size compared to many similar studies in healthcare (Soekhai et al., Citation2019). As noted previously, however, the number of AAC professionals in the UK is small, so that the sample size represents a sizeable fraction of the target population.
There was probably some heterogeneity in participants’ opinions and preferences depending on their individual experiences and familiarity with different AAC systems and children. It is a limitation of our study that we did not collect data on what AAC system participants’ were familiar with, and only broad information about what diagnoses they commonly encountered; however, it is difficult to collect such detailed information in a short web survey where the focus was on preference elicitation tasks. There may also be decision-making differences between more experienced AAC professionals, as in Sauerwein and Wegner (Citation2020) (though note that in that study, novices were defined as first-year masters students, some of which had not taken an AAC course, whereas here 93% of participants had at least one year of professional AAC experience). Future work could examine heterogeneity in AAC professionals’ decision-making more closely. This includes how decision-making varies among different specialties, which is not possible to explore in the current study due to 75% of participants having a speech and language therapy background. For example, speech and language therapists may have interpreted regression as a loss of language skills, whereas occupational therapists interpreted it as a loss of physical abilities or vision, and made different decision accordingly.
There is much scope for future research to build on the current study. For example, it would be useful to examine whether the findings about the suitability of hypothetical AAC systems concur with AAC professionals’ opinions about the suitability of real life systems. In addition, the current study highlighted areas in which inequalities in provision could occur, and in future, it could be examined whether such inequalities are found. Finally, it would be fruitful to explore whether AAC professionals’ opinions about the suitability of AAC systems are in agreement with other stakeholders such as people who use AAC and their families. This latter issue is of particular importance given the likely impact on how motivated a child is to use an AAC system, and on how motivated a family is to provide support.
Conclusion
This study complements the earlier BWS and DCE studies, with all three studies examining the decision-making of AAC professionals choosing AAC systems for children from a different perspective. There have also been synergies from performing the studies together in a single research project. The results, together with the findings of the wider research project have been used to help create practical resources to help AAC professionals working with children in their everyday practice. The suite of resources is freely available at https://iasc.mmu.ac.uk/.
Supplemental Material
Download MS Word (50.3 KB)Disclosure statement
No potential conflict of interest was reported by the authors.
Additional information
Funding
References
- Akaike, H. (1974). A new look at the statistical model identification. IEEE Transactions on Automatic Control, 19(6), 716–723. https://doi.org/10.1109/TAC.1974.1100705
- Beukelman, D., & Light, J. (2020). Augmentative and alternative communication for children and adults. (5th ed.). Paul H. Brookes Publishing Co.
- Beukelman, D. R., Thiessen, A., & Fager, S. K. (2021). Personalization of visual scene displays: Preliminary investigations of adults with aphasia, typical females across the age-span, and young adult males and females. Topics in Language Disorders, 41(3), e1–E11. https://doi.org/10.1097/TLD.0000000000000256
- Cheung, K. L., Wijnen, B. F., Hollin, I. L., Janssen, E. M., Bridges, J. F., Evers, S. M., & Hiligsmann, M. (2016). Using best–worst scaling to investigate preferences in health care. PharmacoEconomics, 34(12), 1195–1209. https://doi.org/10.1007/s40273-016-0429-5
- Choi, B. C., & Pak, A. W. (2006). Multidisciplinarity, interdisciplinarity and transdisciplinarity in health research, services, education and policy: 1. Definitions, objectives, and evidence of effectiveness. Clinical and Investigative Medicine. Medecine Clinique et Experimentale, 29(6), 351–364.
- Dietz, A., Quach, W., Lund, S. K., & Mckelvey, M. (2012). AAC assessment and clinical-decision making: The impact of experience. Augmentative and Alternative Communication (Baltimore, Md. : 1985), 28(3), 148–159. https://doi.org/10.3109/07434618.2012.704521
- Enderby, P., Judge, S., Creer, S., & John, A. (2013). Examining the need for and provision of AAC methods in the UK. Advances in Clinical Neuroscience & Rehabilitation, 13, 20–23.
- Fried-Oken, M., Mooney, A., & Kinsella, M. (2019). Cognitive Demands Checklist for Augmentative and Alternative Communication (CDC4AAC). Presentation at the Assistive Technology Industry Association (ATIA) Annual Conference, Orlando, FL.
- Geytenbeek, J. J., Vermeulen, R. J., Becher, J. G., & Oostrom, K. J. (2015). Comprehension of spoken language in non‐speaking children with severe cerebral palsy: An explorative study on associations with motor type and disabilities. Developmental Medicine and Child Neurology, 57(3), 294–300. https://doi.org/10.1111/dmcn.12619
- Gross, J. (2010). Augmentative and alternative communication: A report on provision for children and young people in England. Office of the Communication Champion.
- Hajjar, D. J., Mccarthy, J. W., Benigno, J. P., & Chabot, J. (2016). You get more than you give”: Experiences of community partners in facilitating active recreation with individuals who have complex communication needs. Augmentative and Alternative Communication (Baltimore, Md. : 1985), 32(2), 131–142. https://doi.org/10.3109/07434618.2015.1136686
- Hemsley, B., & Murray, J. (2015). Distance and proximity: Research on social media connections in the field of communication disability. Disability and Rehabilitation, 37(17), 1509–1510. https://doi.org/10.3109/09638288.2015.1057031
- Hess, S., & Palma, D. (2019). Apollo: A flexible, powerful and customisable freeware package for choice model estimation and application. Journal of Choice Modelling, 32, 100170. https://doi.org/10.1016/j.jocm.2019.100170
- Hynan, A., Goldbart, J., & Murray, J. (2015). A grounded theory of internet and social media use by young people who use augmentative and alternative communication (AAC). Disability and Rehabilitation, 37(17), 1559–1575. https://doi.org/10.3109/09638288.2015.1056387
- Johnson, J. M., Inglebret, E., Jones, C., & Ray, J. (2006). Perspectives of speech language pathologists regarding success versus abandonment of AAC. Augmentative and Alternative Communication, 22(2), 85–99. https://doi.org/10.1080/07434610500483588
- Judge, S., Enderby, P., Creer, S., & John, A. (2017). Provision of powered communication aids in the United Kingdom. Augmentative and Alternative Communication (Baltimore, Md. : 1985), 33(3), 181–187. https://doi.org/10.1080/07434618.2017.1347960
- Judge, S., Randall, N., Goldbart, J., Lynch, Y., Moulam, L., Meredith, S., & Murray, J. (2020). The language and communication attributes of graphic symbol communication aids–a systematic review and narrative synthesis. Disability and Rehabilitation. Assistive Technology, 15(6), 652–662. https://doi.org/10.1080/17483107.2019.1604828
- King, G., Batorowicz, B., & Shepherd, T. A. (2008). Expertise in research-informed clinical decision making: Working effectively with families of children with little or no functional speech. Evidence-Based Communication Assessment and Intervention, 2(2), 106–116. https://doi.org/10.1080/17489530802296897
- Kuhfeld, W. F., Tobias, R. D., & Garratt, M. J. (1994). Efficient experimental design with marketing research applications. Journal of Marketing Research, 31(4), 545–557. https://doi.org/10.1177/002224379403100408
- Light, J., & McNaughton, D. (2013). Putting people first: Re-thinking the role of technology in augmentative and alternative communication intervention. Augmentative and Alternative Communication (Baltimore, Md. : 1985), 29(4), 299–309. https://doi.org/10.3109/07434618.2013.848935
- Light, J., & McNaughton, D. (2014). Communicative competence for individuals who require augmentative and alternative communication: A new definition for a new era of communication? Augmentative and Alternative Communication (Baltimore, Md. : 1985), 30(1), 1–18. https://doi.org/10.3109/07434618.2014.885080
- Light, J., McNaughton, D., Beukelman, D., Fager, S. K., Fried-Oken, M., Jakobs, T., & Jakobs, E. (2019). Challenges and opportunities in augmentative and alternative communication: Research and technology development to enhance communication and participation for individuals with complex communication needs. Augmentative and Alternative Communication (Baltimore, Md. : 1985), 35(1), 1–12. https://doi.org/10.1080/07434618.2018.1556732
- Light, J., McNaughton, D., & Caron, J. (2019). New and emerging AAC technology supports for children with complex communication needs and their communication partners: State of the science and future research directions. Augmentative and Alternative Communication (Baltimore, Md. : 1985), 35(1), 26–41. https://doi.org/10.1080/07434618.2018.1557251
- Light, J., Wilkinson, K. M., Thiessen, A., Beukelman, D. R., & Fager, S. K. (2019). Designing effective AAC displays for individuals with developmental or acquired disabilities: State of the science and future research directions. Augmentative and Alternative Communication (Baltimore, Md. : 1985), 35(1), 42–55. https://doi.org/10.1080/07434618.2018.1558283
- Louviere, J. J., Hensher, D. A., & Swait, J. D. (2000). Stated choice methods: Analysis and applications., Cambridge University Press.
- Lund, S. K., & Light, J. (2006). Long-term outcomes for individuals who use augmentative and alternative communication: Part I–What is a “good. Augmentative and Alternative Communication (Baltimore, Md. : 1985), 22(4), 284–299. https://doi.org/10.1080/07434610600718693
- Lund, S. K., Quach, W., Weissling, K., Mckelvey, M., & Dietz, A. J. (2017). Assessment with children who need augmentative and alternative communication (AAC): Clinical decisions of AAC specialists. Language, Speech, and Hearing Services in Schools, 48(1), 56–68. https://doi.org/10.1044/2016_LSHSS-15-0086
- Lynch, Y., Murray, J., Moulam, L., Meredith, S., Goldbart, J., Smith, M., Batorowicz, B., Randall, N., & Judge, S. (2019). Decision-making in communication aid recommendations in the UK: Cultural and contextual influencers. Augmentative and Alternative Communication (Baltimore, Md. : 1985), 35(3), 180–192. https://doi.org/10.1080/07434618.2019.1599066
- McFadd, E., & Wilkinson, K. (2010). Qualitative analysis of decision making by speech-language pathologists in the design of aided visual displays. Augmentative and Alternative Communication, 26(2), 136–147. https://doi.org/10.3109/07434618.2010.481089
- Murray, J., Lynch, Y., Goldbart, J., Moulam, L., Judge, S., Webb, E., Jayes, M., Meredith, S., Whittle, H., Randall, N., Meads, D., & Hess, S. (2020). The decision-making process in recommending electronic communication aids for children and young people who are non-speaking: The I-ASC mixed-methods study. Health Services and Delivery Research, 8(45), 1–158. https://doi.org/10.3310/hsdr08450
- Murray, J., Lynch, Y., Meredith, S., Moulam, L., Goldbart, J., Smith, M., Randall, N., & Judge, S. (2019). Professionals’ decision-making in recommending communication aids in the UK: Competing considerations. Augmentative and Alternative Communication, 35(3), 167–179. https://doi.org/10.1080/07434618.2019.1597384
- NHS England. (2016). Guidance for commissioning AAC services and equipment. https://www.england.nhs.uk/commissioning/wp-content/uploads/sites/12/2016/03/guid-comms-aac.pdf
- Royal College of Speech and Language Therapists (2009). Resource manual for commissioning and planning services for SLCN. Royal College of Speech and Language Therapists.
- Ryan, S. E., Shepherd, T., Renzoni, A. M., Anderson, C., Barber, M., Kingsnorth, S., & Ward, K. (2015). Towards advancing knowledge translation of AAC outcomes research for children and youth with complex communication needs. Augmentative and Alternative Communication, 31(2), 137–147. https://doi.org/10.3109/07434618.2015.1030038
- Sauerwein, A. M., & Wegner, J. R. (2020). Clinical reasoning skills in AAC intervention planning: Investigating the expert-novice gap. Teaching and Learning in Communication Sciences & Disorders, 4(2), 7. https://doi.org/10.30707/TLCSD4.2/XNDO8764
- Schlosser, R. W., & Wendt, O. (2008). Effects of augmentative and alternative communication intervention on speech production in children with autism: A systematic review. American Journal of Speech-Language Pathology, 17(3), 212–230. https://doi.org/10.1044/1058-0360(2008/021)
- Soekhai, V., De Bekker-Grob, E. W., Ellis, A. R., & Vass, C. M. (2019). Discrete choice experiments in health economics: Past, present and future. PharmacoEconomics, 37(2), 201–226. https://doi.org/10.1007/s40273-018-0734-2
- Sundqvist, A., & Rönnberg, J. (2010). A qualitative analysis of email interactions of children who use augmentative and alternative communication. Augmentative and Alternative Communication (Baltimore, Md. : 1985), 26(4), 255–266. https://doi.org/10.3109/07434618.2010.528796
- Thistle, J. J., & Wilkinson, K. M. (2015). Building evidence-based practice in AAC display design for young children: Current practices and future directions. Augmentative and Alternative Communication (Baltimore, Md. : 1985), 31(2), 124–136. https://doi.org/10.3109/07434618.2015.1035798
- Van Niekerk, K., Dada, S., Tönsing, K., & Boshoff, K. (2018). Factors perceived by rehabilitation professionals to influence the provision of assistive technology to children: A systematic review. Physical & Occupational Therapy in Pediatrics, 38(2), 168–189. https://doi.org/10.1080/01942638.2017.1337661
- Webb, E. J., & Hess, S. (2021). Joint modelling of choice and rating data: Theory and examples. Journal of Choice Modelling, 40, 100304. https://doi.org/10.1016/j.jocm.2021.100304
- Webb, E. J., Lynch, Y., Meads, D., Judge, S., Randall, N., Goldbart, J., Meredith, S., Moulam, L., Hess, S., & Murray, J. (2019a). Finding the best fit: Examining the decision-making of augmentative and alternative communication professionals in the UK using a discrete choice experiment. BMJ Open, 9(11), e030274. https://doi.org/10.1136/bmjopen-2019-030274
- Webb, E. J., Meads, D., Lynch, Y., Judge, S., Randall, N., Goldbart, J., Meredith, S., Moulam, L., Hess, S., & Murray, J. (2021). Attribute selection for a discrete choice experiment incorporating a best-worst scaling survey. Value in Health : The Journal of the International Society for Pharmacoeconomics and Outcomes Research, 24(4), 575–584. https://doi.org/10.1016/j.jval.2020.10.025
- Webb, E. J., Meads, D., Lynch, Y., Randall, N., Judge, S., Goldbart, J., Meredith, S., Moulam, L., Hess, S., & Murray, J. (2019b). What’s important in AAC decision making for children? Evidence from a best–worst scaling survey. Augmentative and Alternative Communication (Baltimore, Md. : 1985), 35(2), 80–94. https://doi.org/10.1080/07434618.2018.1561750
- Wilkinson, K. M., Light, J., & Drager, K. (2012). Considerations for the composition of visual scene displays: Potential contributions of information from visual and cognitive sciences. Augmentative and Alternative Communication (Baltimore, Md. : 1985), 28(3), 137–147. https://doi.org/10.3109/07434618.2012.704522
- Williams, M., Beukelman, D., & Ullman, C. (2012). AAC text messaging. Perspectives on Augmentative and Alternative Communication, 21(2), 56–59. https://doi.org/10.1044/aac21.2.56
- Williams, M. B., Krezman, C., & McNaughton, D. J. (2008). Reach for the stars”: Five principles for the next 25 years of AAC. Augmentative and Alternative Communication, 24(3), 194–206. https://doi.org/10.1080/08990220802387851