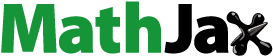
Abstract
Objective: Over the 2018–2019 flu season we conducted a randomized controlled trial examining the efficacy of a Twitter campaign on vaccination rates. Concurrently we investigated potential interactions between digital social network structure and vaccination status. Participants: Undergratuates at a large midwestern public university were randomly assigned to an intervention (n = 353) or control (n = 349) group. Methods: Vaccination data were collected via monthly surveys. Participant Twitter data were collected through the public-facing Twitter API. Intervention impact was assessed with logistic regression. Standard network science tools examined vaccination coverage over online social networks. Results: The campaign had no effect on vaccination outcome. Receiving a flu shot the prior year had a positive impact on participant vaccination. Evidence of an interaction between digital social network structure and vaccination status was detected. Conclusions: Social media campaigns may not be sufficient for increasing vaccination rates. There may be potential for social media campaigns that leverage network structure.
1. Introduction
Seasonal influenza is a major annual health burden that causes significant mortality and morbidity in the United States each year.Citation1 While the flu does not typically cause significant mortality in college-aged (18–24) individuals, this group can suffer high morbidity.Citation2–4 Contracting the flu negatively impacts a college student’s academic performance, social life, and ability to work. Outbreaks of seasonal influenza on a college campus also have the potential to rapidly spread given that many students inhabit densely populated shared living spaces, and have close proximity to others in classrooms and dining areas.Citation5–7 Undergraduates can serve as disease conduits to at-risk populations as the flu spreads from October to May,Citation6 a period including winter break when many students come into contact with groups that suffer high mortality from the illness.
There has been great interest in understanding the vaccination behaviors of the US undergraduate population,Citation8–19 likely due to their reportedly low vaccination rate (found to be anywhere from 8–30%Citation15,Citation20–22 of the student body). Cross-sectional survey techniques have illuminated social, psychological, and physical barriers to increased undergraduate vaccination.Citation8,Citation10,Citation13,Citation15,Citation17,Citation23,Citation24 However, few published studies have probed the effectiveness of various interventions on collegiate vaccination rates,Citation9,Citation11,Citation12,Citation18,Citation19,Citation25 and of those even fewer have used participant vaccination outcome as the variable of interest.Citation9,Citation11,Citation18,Citation19
Concurrently, there has been a surge in the presence of social networking services (SNS), with utilization increasing from 36% of US adults in 2009 to 72% in 2019 (70% to 90% utilization in college-aged Americans).Citation26 Researchers have leveraged this digital facet of life to fight influenza by developing flu surveillance methods using TwitterCitation27–31 or Google TrendsCitation30,Citation32–35 data, as well as demonstrating market segmentation possibilities through tweet sentiment analysis.Citation36–38 Only a handful of studies have examined SNS as platforms for public health intervention,Citation11,Citation19 and in these studies SNS were often combined with offline interventions, making isolating the impact of the SNS difficult if not impossible. In the current literature there is little to no work directly examining the effectiveness of interventions utilizing SNS to raise vaccination rates, despite the presence of SNS in existing (CDC) campaigns.Citation39,Citation40
The network aspect of SNS adds a wrinkle to this form of public health intervention not present in promotional interventions on traditional media sources like television and radio. The effect of offline social networks on flu vaccination choice has been previously demonstrated,Citation14,Citation41–43 and digital social networks have been shown to be assortative according to vaccination sentiment.Citation36 Whether these digital networks are heterogeneous with respect to user vaccination status has not yet been explored. Assortative mixing of vaccination status on such networks has the potential to impact the effectiveness of a social media campaign.
In the 2018–2019 flu season we conducted a randomized controlled trial to investigate the efficacy of a social media campaign aimed at raising influenza vaccination uptake among undergraduates while probing the potential of digital social networks for future interventions.
2. Materials and methods
2.1. Study design
Our study was conducted from October 2018 to May 2019 at a large midwestern public university. Students were recruited over a one month period from August 2018 to September 2018. This design received approval from the institution’s Institutional Review Board (IRB) in the Spring of 2018.
2.1.1. Enrollment and participant demographics
The target population for this study consisted of all undergraduate students registered in the 2018 autumn semester who had a Twitter account and were over the age of 18. Of the target population, we enrolled n = 702 participants through an email solicitation sent to all 46,820 enrolled undergraduates in the autumn of 2018. Low recruitment rates have been reported for email solicitations for other studies.Citation44,Citation45 Difficulty distinguishing legitimate content from spam is a challenge for email recruitment,Citation44 and may have played a role in the low response rate here. Students that expressed interest were sent a digital informed consent form.
Participants were randomized into an intervention group (n = 353) or a control group (n = 349). This split was stratified based on their self-reported gender and response to the question “Are you required to get a flu shot?” which we consider their self-reported flu requirements for the 2018–2019 flu season.
2.1.2. Intervention and control procedures
Intervention group members were asked to follow an intervention Twitter account that posted near daily tweets (1.24 tweets per day) promoting flu vaccination. In addition to direct tweet exposure, campaign engagement was incentivized with prize raffle entries. For each month of the study, an intervention group member could receive one raffle entry (up to seven over the study) by retweeting one of the promotional tweets, or by constructing their own tweet containing a hashtag that was unique to the campaign. In contrast, we requested that control group participants follow a control Twitter account that tweeted no content.
Indirect exposure is possible in this design. At the time of our study it was impossible to completely isolate content without also removing the main method of campaign engagement, retweeting. In order to emulate a realistic social media campaign we elected for a design that permitted indirect exposure and attempt to control for it in our analysis.
Monthly check-in surveys tracked flu vaccination status over the study period. Intervention group participants were incentivized to respond to these surveys with an additional prize raffle entry per response (a possible seven entries in addition to the seven possible entries as retweet incentives, for a total of fourteen maximum). Control group participants could receive two prize raffle entries per check-in survey response (up to fourteen maximum).
2.1.3. Intervention campaign content
Our campaign presented a mixture of comedic and informational content. All posts were created by the corresponding author. Any substantive information regarding seasonal influenza or the yearly flu shot was sourced from the CDC influenza website, https://www.cdc.gov/flu/. Comedic content leveraged tweet and meme formats that were popular among the intervention group. Popularity was anecdotally determined by browsing the Twitter feed of the intervention account. Informative posts were constructed by consulting the aforementioned CDC flu website and news sources found to contain relevant factual information. In an additional attempt to tailor the campaign to our audience we included content specific to their university. For example, a rendition of the school’s mascot kicking an influenza virus was utilized as the intervention account avatar.
Starting in December 2018 the intervention account also began tweeting an image unrelated to flu shots at the end of each month. This was done to measure actual exposure to the campaign across both groups. All images were found by scrolling to the bottom of Google images search results, so it was unlikely they would have independently appeared outside of the study account posts.
2.1.4. Data collection
All surveys were distributed via email and administered with Qualtrics software.
Each month of the observation period participants were sent check-in surveys in which they indicated whether they received a flu shot in the previous month. Starting in December each survey also contained a CAPTCHA-likeCitation46 question that presented respondents with four images, and then asked them to select any they recalled seeing in their Twitter feed. One of the images was tweeted multiple times by the intervention account in the week prior to the check-in survey (see Sec. 2.1.3), the other three were of a similar theme.
Participant Twitter data was scraped on a weekly basis. Data was obtained using the Tweepy Python Twitter API Wrapper version 3.6.0 that was run using Python version 3.6.9. Collected data included the tweets, followers, and accounts they followed, from here on referred to as their friends, for each participant.
To augment self-reported flu vaccination data, the univeristy’s Student Health Center (SHC) provided flu vaccination records of all participants over the course of the study. Only a small percentage of the student body receives a vaccine at SHC each year, so this data cannot be used to directly analyze the impact of the campaign on the intervention group. However, we do calculate what percentage of the participants that reported getting vaccinated at SHC actually got vaccinated at SHC, which will provide some measure of desirability bias.Citation47
2.2. Data analysis
2.2.1. Missing data
Reliance on self-reported vaccination status led to missing outcome data for a non-negligible portion of the study population.
In addition to the “Since <first of the past month > have you received a flu vaccination?” question standard to all monthly check-in surveys, our final survey contained the question “Did you get a flu shot at any point since October 1, 2018?”. Combining the responses to that question with our standard flu shot inquiry allowed us to classify each participant as having gotten a flu shot over the course of the study, not having gotten a flu shot over the course of the study, or having missing data. Participants were classified as follows:
Getting a flu shot if they answered “Yes” on any of the monthly surveys or they responded “Yes” to ever receiving a flu shot question in the final survey,
Not getting a flu shot if they answered “No” to all of the monthly surveys, or if they answered “No” to the flu vaccination question added in the final survey while not indicating “Yes” in any of the monthly check-ins,
Missing if they did not answer “Yes” on any of the monthly surveys, had some missing monthly survey responses and did not respond to the flu vaccination question added in the final survey.
Classification in this manner led to 156 (22.2%) participants with missing outcome data, of which 94 (26.6%) were in the intervention group and 62 (17.7%) were in the control. Unsure responses to demographic data survey questions were treated as missing data as well.
Missing values were not missing completely at random,Citation48 and thus we could not ignore them in any analysis. Multiple imputation,Citation49 performed in RCitation50 version 3.5.3 with the Multivariate Imputation by Chained Equations (mice) packageCitation51 was used to create 50 unique data sets with complete data. Statistical models discussed below were fit to each of the imputed data sets, and results were averaged for final analysis.
2.2.2. Analysis of intervention impact on vaccination outcome
If a participant indicated receiving a shot, they also identified a date in which they received that shot. Anyone that received a shot prior to the start of the intervention (October 1, 2018) was not included in the vaccination outcome analysis. This disqualified 75 of our participants, leaving 627 (315 in the intervention, 312 in the control) observations. The 156 participants missing vaccination outcome were not disqualified in this step.
Impact on vaccination rate was analyzed with a series of logistic regression models. We present four models, all of which have vaccination outcome as the dependent variable. Following Hsieh et al.Citation52 we calculated the minimum percentage point increase we would be able to detect at 95% confidence and 80% power for each model. As a baseline we utilized a vaccination level of 46% for the control group, which was chosen based on the self-reported vaccination level of the cohort in 2017–2018.
Models 1 and 2 constitute an intent-to-treat analysis, where the only treatment-related independent variable included was study group. These models were fit using the data from all 627 participants that were not disqualified. Model 1 is a parsimonious model containing an intervention group indicator and indicators for receiving a flu shot last year and being required to get a shot. Model 2 then adds in demographic variables.
Models 3 and 4 assess the impact of campaign engagement and control for indirect campaign exposure. Data used to estimate these models were restricted to the 564 individuals (287 intervention and 277 control) for which we collected Twitter data. Twitter data collection was not possible for any participant that had a private account and did not accept a follow request from one of our study accounts. Of the 564, 23.6% were missing vaccination outcome (28.2% intervention and 18.8% control).
Model 3 is the same simple model as Model 1, restricted to this subset of participants. Model 4 adds in several Twitter activity variables including an indicator of whether or not a participant followed the intervention account, the total number of check-in pictures a participant reported seeing, an indicator of whether or not a participant tweeted our campaign hashtag, and an indicator as to whether or not the person posted a tweet containing the word “health” over the course of the study. The first three of these variables control for campaign exposure, while the last variable is meant to serve as a measure of the health mindfulness of the online personas of our participants.
2.2.3. Digital social network types
The interaction between digital social network structure and participant vaccination outcome was examined using three distinct network types.
The participant direct followership network is the subset of the entire Twitter follower network comprised of the study participants and the edges between them. In particular, in this network there is an edge from participant A to participant B if A follows B on Twitter. This network was constructed using the friends and follower data pulled at the start of the study.
Cofollowing networks connect two participants based on the Twitter accounts they both follow. Formally, we define them as a weighted directed network in which the weight of the edge from participant A to participant B is given by:
(1)
(1)
where nA is the number of accounts followed by A, and nAB is the number of accounts that both A and B follow.
Conversely, cofollower networks connect participants using mutual Twitter followership. In a cofollower network the edge from participant A to B is also given by (1), where in this setting n* refers to the number of accounts that follow * instead. Cofollower and cofollowing networks were constructed using the friend and follower data from the end of the study.
2.2.4. Vaccination Behavior over digital social networks
Over the direct followership network we calculated the assortativity coefficientCitation53 of vaccination outcome. Assortativity in this context measures how likely a participant is to follow another participant that has the same vaccination outcome as them in comparison to a random wiring of the nodes. A positive coefficient indicates that participants were more likely to make connections with those of the same vaccination status, while a negative coefficient indicates that participants were more likely to make connections with those of opposite vaccination status. To see how the observed coefficient compared to what was possible on the given network we peformed a permutation test, in which we randomly shuffled the vaccination states among the nodes and recorded the assortativity coefficient 10,000 times.
For the cofollower and cofollowing networks we examined the distribution of vaccinations across tighly-knit groups of participants, or so-called communities. Community labels for each participant were produced using a generalizationCitation54,Citation55 of the Louvain algorithm, a modularity maximation technique. Modularity is a measurement of community structure due to Newman and GirvanCitation56,Citation57 that compares the inter- and intra-community edge weights compared to an equivalent random network for a given community assignment. A community assignment with high modularity has dense within community connections and sparse between community ties.
Generalizations of the Louvain algorithm for weighted directed networks have been applied to various Twitter networks.Citation58–60 We cross-referenced the resulting assignments with participant Twitter and demographic data to ensure that the detected groups were meaningful. Groups within the cofollowing network should reflect who the participants follow on Twitter, and may be indicative of group interests. Cofollower networks contain information on who follows our participants, and thus, given the modest number of followers of the cohort on Twitter, communities may be representative of offline friendships in the university community.
Multiple imputation was used to create 50 complete vaccination outcome data sets. We then examined the percent vaccinated in each of these sets across the Louvain-detected communities. An important step in determining if our communities exhibit differential coverage is comparing what is observed over the detected communities to what would be seen given an equivalent random community assignment.
In order to make this comparison we perform the same hypothesis testCitation61–63 using the Louvain detected communities, and random permutations of that labeling. Formally, the null hypothesis for this test assumes that pi = pj for all communities i and j, where pk is vaccine coverage in community k. The alternative hypothesis was that there exists a pair of communities i and j such that pi ≠ pj. This test was performed for each of the 50 multiply imputed data sets and the resulting p-values were recorded. We refer to this collection as the set of nonrandom p-values. For every imputed data set, we randomly shuffled the participants between communities while maintaining the size of each community 1,000 times. The hypothesis test described above was then performed on each of the 50,000 random data sets, and we recorded the resulting p-values. We call this set of p-values the random p-values.
Random p-values were compared to nonrandom p-values with a one-sided Kolmogorov-Smirnov test.Citation64,Citation65 The null hypothesis was that the cumulative distribution function (CDF) of the underlying distribution for the nonrandom p-values was not greater than the CDF of the underlying distribution for the random p-values. The alternative hypothesis was that the CDF of the underlying distribution for the nonrandom p-values was greater. We used the one-sided alternative because if there was differential vaccination coverage across the detected communities, the p-values from the tests for equal proportions across the detected communities should be stochastically lower than the p-values from the tests using random community assignments.
3. Results
3.1. Participant demographics
We present a demographic breakdown by study group in and present aggregate medical history statistics relevant to flu vaccination in . The intervention and control groups are nearly identical with regard to race and age related variables. Both groups are also similar with respect to flu-relevant medical history.
Table 1. A Demographic breakdown comparing the intervention group to the control group.
Table 2. Medical history data by study group.
While participants were not randomized according to their Twitter activity, an analysis using participant Twitter data prior to the start of the intervention on October 1, 2018 demonstrates that activity across the study groups is similar. We present a Twitter activity measurement table and daily post timing plot in and respectively.
Figure 1. Total tweets by study group for each five-minute period of the day that were posted prior to October 1, 2018. Data reflects at most the 3,200 most recent tweets prior to October 1 per participant, and comes from 630 of the 702 participants with 320 from the intervention group and 310 from the control. The remaining 72 participants have missing tweet data. Time is presented in UTC. The control group can be seen on the left with points marked with blue circles and the intervention group can be seen on the right with points marked with red x’s. The timing of tweets between the two groups is similar, with the control group having sent out slightly more tweets than the intervention group prior to October 1, 2018.

Table 3. Summary statistics of participant twitter data prior to the start of the intervention.
3.2. Vaccination outcome
The 2018–2019 vaccination outcomes for both groups are presented in . Percent vaccinated is roughly 45% in both study groups, with the intervention group having a larger percentage of missing data (about 10 percentage points higher).
Table 4. Vaccination outcome in the 2018–2019 flu season by study group, percentages are based on the 353 in the intervention group and the 349 in the control group.
3.3. Intervention efficacy
3.3.1. Intent-to-treat
Following Hsieh et al.Citation52 we found that the minimal detectable percentage point increase from a control rate of 46% to intervention at 80% power and 95% confidence for model 1 was 13 points. For model 2 the minimal detectable increase was 16 points.
Odds ratio point estimates and 95% confidence intervals for models 1 and 2 are presented in . Estimates from both models suggest that there was not a statistically significant effect from the intervention, and that subjects from the intervention group were just as likely as those from the control group to receive a vaccine. Such an estimate suggests that if the intervention does have a positive effect on vaccination uptake, it is well below the minimal detectable levels our sample size and models are able to detect.
Table 5. Logistic regression results for the intent to treat analysis.
Across all covariates included in either model, the only significant effects came from being required to receive a flu shot or having received a flu shot in the 2017–2018 season. In this cohort we estimated that an individual that received their flu shot in 2017–2018 was over six times as likely to receive their flu shot in the 2018–2019 season. This effect was even larger than that attributed to a self-reported requirement to receive a flu shot.
Model 2 indicates that none of the demographic covariates had a significant effect on vaccination rate.
3.3.2. Campaign engagement
Following Hsieh et al.Citation52 we found that the minimal detectable percentage point increase from a control rate of 46% to intervention at 80% power and 95% confidence for model 3 was 13.5 points. For model 2 the minimal detectable increase was 21 points.
Estimates for models 3 and 4 can be found in . Results are consistent with models 1 and 2 in finding that individuals assigned to the intervention group were just as likely as those in the control to receive a flu shot. The estimates for model 4 provide additional evidence that the campaign was ineffective, as those who did not follow or share intervention content were just as likely to receive a vaccination as those who did.
Table 6. Logistic regression results for the twitter engagement analysis.
In we present the results of the CAPTCHA-like image identification question restricted to the 564 participants analyzed with models 3 and 4. At least a quarter of the intervention group reported seeing the image we tweeted out in all months except for February. In contrast, 8% or less of the control group identified the image we tweeted out in those same months. While a large portion of the intervention group may not have paid attention to the campaign, there is evidence that our posts were consistently viewed by a quarter of the intervention group. This allows us to rule out the possibility that the campaign was ineffective due to inattention.
Figure 2. Responses to the CAPTCHA-like image question in the December 2018–April 2019 monthly check-in surveys. The height of the blue bars correspond to the percentage of participants in either group that indicated seeing the image tweeted by the intervention account, red bars correspond to those that did not, and green bars correspond to participants that did not reply to the question. Percentages are with respect to the 277 total control group members and 287 intervention group members included in the fits of models 3 and 4.

Figure 3. The direct followership network from the beginning of the intervention period. Each node represents a participant and directed edges denote followership on Twitter. There are 260 participants in the network, remaining participants did not follow someone else in the study. Nodes are colored by their indicated flu shot coverage in the 2017–2018 season. The assortativity of this network by vaccination choice is examined in .

Figure 4. The histogram of possible assortativity coefficients for 10,000 random arrangements of 2017–2018 vaccination status on the network pictured in . Also denoted is the placement of the observed assortativity coefficient, 0.0793. The proportion of random coefficients larger than the observed coefficient was 0.0445 indicating that there is evidence of positive assortativity of 2017–2018 vaccination choice on this network.

Figure 5. Explatory plots for the nonrandom and random p-values for the May cofollowing network. On the left are the histograms from the two sets of p-values. On the right are the empirical cumulative distribution functions (CDFs). The Kolmogorov-Smirnov test for these data had D+= 0.26 with a corresponding p-value < 0.001.

Figure 6. Box and whisker plots for the multiply imputated vaccination percentages for the four Louvain-detected May cofollowing communities with more than ten nodes.

Odds ratio estimates for both models corroborate the finding that receiving a flu shot in 2017–2018, and being required to receive a shot had a significant effect on getting vaccinated in 2018–2019. The effect of prior vaccination was estimated to be slightly higher in this subset of the cohort, as those who received a flu shot in 2017–2018 were roughly seven times more likely to receive one in 2018–2019.
3.4. Vaccination choice over digital social networks
3.4.1. Assortativity of 2017–2018 choice on the direct followership network
We present the direct followership network with each participant colored by their self-reported 2017–2018 vaccination status. The assortativity coefficient associated with this network was calculated to be 0.0793. How this observed value compares to the distribution of 10,000 randomly drawn coefficients is displayed in . We find that our observed value is in the upper 5% of this distribution, indicating that our network displayed significant assortativity with respect to 2017–2018 vaccination choice. In other words, participants were more likely to follow other participants of the same vaccination status in 2017–2018.
3.4.2. Community structure of cofollowing and cofollower networks
The Louvain community detection algorithm found the cofollowing network to have four communities with more than ten participants, while the cofollower network had nine such communities.
Since cofollowing networks connect individuals based on mutual following habits, we cross-referenced the Louvain algorithm community labels with the most followed Twitter accounts among the participants within each community. While there were accounts shared across multiple communities (for example, the account belonging to the university mascot), each community had accounts unique to them that suggest broad group interests. Community 1 primarily followed accounts related to the university community (e.g. student life, the university president and the school paper), community 2 followed sports-related accounts (e.g. professional and collegiate athletes), community 3 tended to follow accounts related to popular culture, (e.g. actors and musicians), and community 4 followed accounts associated with various political figures.
The communities detected on our cofollower network were examined with the demographic data of our participants. We present the breakdown of each community by various demographic variables in and . Investigation of these tables suggests that the Louvain algorithm was able to capture meaningful community structure over the cofollower network. For example, communities seemed to segment participants according to school year, racial identity and extracurricular group participation.
Table 7. Louvain-detected community by demographic variables for the cofollower network constructed using data pulled at the end of the study period.
Table 8. Breakdown of louvain-detected communities by participation in extracurricular groups for the cofollower network from data pulled at the end of the study period.
These results suggest that our cofollowing and cofollower networks exhibit meaningful community structure. Cofollowing communities may be refelective of interest groups or market segments,Citation66 while cofollower communities may capture offline social circles.
3.4.3. Vaccination coverage of Louvain-detected communities
Given the overall vaccination coverage and the size of the cofollowing and cofollower communities it is unlikely for the random community rearrangements described in Sec. 4.4 to have violated the assumptions of the hypothesis test comparing community proportions.Citation67 We thus present the results of the Kolmogorov-Smirnov test in . We find statistically significant evidence that the p-values associated with the Louvain detected cofollowing communites were stochastically lower than those associated with equivalent random assignments. A graphical comparison of the two sets can be found in . This indicates the vaccination distribution across our observed communities is not the result of random noise, but rather a true feature of the data. In particular, this suggests that some cofollowing communities had lower vaccination coverage than others. While the missing data make it difficult to perform direct comparison tests between the four communities, we do include boxplots of the percent vaccinated within each Louvain-detected community across the 50 multiply imputed data sets in . These boxplots show cofollowing communities 1 and 4 as having lower vaccination levels than the remaining communities.
Table 9. Kolmogorov-Smirnov Test results for the cofollower and cofollowing networks.
The Kolmogorov-Smirnov test for the cofollower network did not provide evidence of differential vaccination coverage. As such, we do not examine the distributions of the nonrandom and random p-values nor do we provide boxplots as we did for the cofollowing data.
3.5. Cross-referencing with SHC vaccination records
We investigated desirability bias in participant self-reported vaccination decision by cross-referencing SHC vaccination records for the 2018–2019 season. Contingency tables comparing participants that reported receiving a shot at SHC to those that actually got one are presented in . We find that in both study groups roughly 70% of participants that indicated getting an SHC vaccine did not actually receive one. While this suggests a large desirability bias, we cannot confirm whether these students did not get a flu shot or just misclassified where they got their flu shot. If 70% was the true misreporting rate, the SHC data suggest that the rate is consistent across both study groups, so it should not have impacted the intent to treat analysis.
Table 10. Contingency tables for participants reporting receiving a flu shot at SHC vs actually receiving a vaccine from SHC.
4. Discussion
4.1. Past behavior explains future results
Across the entirety of our analysis we found that being required to receive a flu shot and having received a flu shot the year before were the only variables to have an impact on vaccination uptake in 2018–2019.
Prior vaccination status has been shown to impact an individual’s decision to get a flu shot across a number of prior studies.Citation8,Citation15,Citation43,Citation68,Citation69 As more independent studies reproduce this finding, it is likely that past behavior is predictive of future flu shot status. This highlights both the importance and potential benefits of recruiting the undergraduate population into this behavior. If public health entities are able to get college age individuals to start receiving vaccinations at their younger age, there is potential for it to transform into a lifelong habit.Citation69 Conversely, this could indicate that the key to raising collegiate vaccination rates lies in the pre-college/childhood years.
Our finding of misreporting of vaccination location using SHC data indicates that the association between prior and present vaccination status may not be as strong as our models suggest. Rather, these results could reflect that individuals who misreported their vaccination status in one year were also likely to misreport their vaccination status in the following year, but this is difficult to disentangle without actual vaccination records from the 2017–2018 flu season.
4.2. Campaign messaging and credibility
While we attempted to cater message content to our cohort, perhaps it was not persuasive enough to spur action. Effective messaging content is an active area of influenza vaccination promotion research.Citation9,Citation12,Citation25 Message effectiveness may have been improved by involving additional individuals and perspectives in crafting tweets. However, formative work and development may also be needed to receive feedback on content. Further, establishing behavioral health models that guide messaging content would improve interventions and the replicability of future studies.
Our campaign also used the same messages to appeal to the entire intervention group. However, the cofollowing network of our participants helped us identify four large subgroups of the cohort with unique interests. Catering the messaging to each specific communityCitation70 could result in a more effective intervention and is a direction for future research. For example, messaging that appeals to cofollowing community 1 (which had lower than total cohort vaccination coverage) could focus on university-specific content.
The credibility of our account as a source of health care information is an unknown factor. While the information we shared on seasonal influenza and vaccination came directly from the CDC and reputable news outlets, the account itself was not a health care provider, a hub for medical information, nor a close friend to the participants. A number of studies have found that people are more likely to trust and act on medical information from sources they deem to be credible, namely their primary healthcare provider or close friends/family.Citation42,Citation43,Citation69,Citation71–73
4.3. Effectiveness of reminder systems
In our intervention Twitter was utilized as a reminder system for the members of the intervention group. Reminder systems as the sole intervention have often not succeeded at significantly impacting the desired outcome over various promotional vaccination studies.Citation18,Citation74,Citation75 A study similar to oursCitation18 found that flu shot reminders sent through university email systems were ineffective at raising vaccination rates compared to control across different message types. However, they did find that combining the email reminder system with a thirty dollar incentive rendered upon getting a flu shot did significantly increase vaccination rates.
Paying students to receive a flu shot is probably not an economically viable recommendation, but that study is just one instance of many that have shown that reminder systems can be part of successful vaccination campaigns.Citation11,Citation18,Citation19,Citation76 Our results indicate that social media reminder campaigns may not be effective on their own, highlighting the need to pair them with additional interventions, especially given that they can be conducted for little financial cost and may be able to magnify the effects of other interventions by spreading the word.
For instance, manyCitation8,Citation13,Citation17,Citation19,Citation23,Citation42,Citation43,Citation77 cross-sectional surveys of collegiate populations have shown that the physical act of going to get a flu shot is a major barrier to increased rates. An intervention that removed this barrier with in-dormitory and dining hall vaccination clinics utilized SNS in a successful manner by having peer health care workers advertise these vaccination clinics with a personalized social media campaign.Citation11
4.4. Vaccination choice over digital and physical social networks
Physical social networks have been shown to have an impact on vaccination choice.Citation14,Citation41–43 People who were vaccinated were more likely to have friends and family members that received a shot, with an analogous result for the unvaccinated.Citation14,Citation41 Anecdotal stories from close friends or family can weigh heavily on a person’s decision to receive a flu shot,Citation42,Citation43 and a finding similar to ours has shown that the physical contact network of a high school exhibited positive network assortativity with regard to flu vaccination.Citation78
Conversely, other Twitter studies found that the networks underlying SNS can be highly assortative with regard to sentiments on flu shotsCitation36,Citation37 It has also been shown in mathematical models that if actual vaccination status was similarly polarized on physical social networks, the probability of large outbreaks would be greatly increased.Citation36,Citation79
Our results bridge and build upon these two research areas by demonstrating evidence of assortativity and differential coverage of flu vaccination choice over the digital social networks of a cohort. To our knowledge, this study is the first work to produce such a finding. The breakdowns of the Louvain-detected cofollower communities in and suggest that the digital social networks of our participants are representative of their physical social networks. It is thus possible that some highly connected segments of the undergraduate population could be more at risk for an influenza outbreak than others.
However, our study did not collect offline social network data, so we cannot directly examine relationships between offline and online vaccination coverages and thus future research is needed. One approach would be to adapt a standard technique for recruiting physical friendship networks in which randomly recruited participants are asked to identify friends for a second wave of recruitment.Citation14,Citation80 Having the first round of participants invite both a set of physical friends and a set of digital friends would allow for digital social networks to be layered on top of physical social networks. The distribution of vaccination choice could be examined over both networks. If a correlation is seen to exist between online and offline vaccination coverage, online networks could be used as a more cost-effective proxy for offline network coverage.
4.5. Conclusion
Given the ubiquity of social networking services,Citation26 understanding their potential impact on public health is of the utmost importance. This is particularly true during the COVID-19 pandemic,Citation81 which has seen widespread online misinformation on virus origins, ongoing vaccination campaigns, disease severity, and more.Citation82 As such, it is crucial for public health entities to know what does and does not work when it comes to encouraging preventative health behaviors using SNS.
To what extent the results of this study are applicable to COVID-19 vaccination campaigns remains to be seen. The COVID-19 pandemic continues to unfold against a highly polarized political landscape,Citation83 and the accompanying divergence in perceptions of disease severity, vaccine efficacy and risk, and attitudes toward public health interventions such as stay-at-home orders, social distancing, and mask mandates may influence responsiveness to vaccination campaigns. The COVID-19 vaccines available to American undergraduates have been found to be significantly more effectiveCitation84,Citation85 than influenza vaccines whose efficacy fluctuates from season to season.Citation86–88 Some universities have also decided to require a COVID-19 vaccination prior to returning to in-person activities in the Fall of 2021,Citation89 which would make promotion of this vaccine moot.
However, while our study may not be broadly applicable to all collegiate vaccination campaigns, our results suggest that campaigns encouraging best practices through social media messaging alone will not be sufficient. Effective campaigns will likely require additional interventions (e.g. in-dormitory vaccination clinics) or more sophisticated message delivery strategies (e.g. targeted marketing).
Conflict of interest disclosure
The authors report no conflicts of interest. The research presented in this article met ethical guidelines, including adherence to legal requirements of the United States and has been approved by the Ohio State University IRB. The funding source played no role in how the research was conducted.
Acknowledgments
The authors acknowledge the Ohio State University STEAM Factory “STEAM Powered Project” seed grant for providing funding for raffle incentives, and the Ohio State University Student Health Center for providing flu vaccination data.
Funding
No funding was used to support this research and/or the preparation of the manuscript.
References
- Molinari N-AM, Ortega-Sanchez IR, Messonnier ML, et al. The annual impact of seasonal influenza in the US: measuring disease burden and costs. Vaccine. 2007;25(27):5086–5096. doi:10.1016/j.vaccine.2007.03.046.
- Nichol KL, Heilly SD, Ehlinger E. Colds and influenza-like illnesses in university students: impact on health, academic and work performance, and health care use. Clin Infect Dis. 2005;40(9):1263–1270. doi:10.1086/429237.
- Nichol KL, D’Heilly S, Ehlinger E. Burden of upper respiratory illnesses among college and university students: 2002–2003 and 2003–2004 cohorts. Vaccine. 2006;24(44–46):6724–6725. doi:10.1016/j.vaccine.2006.05.033.
- Nichol KL, Tummers K, Hoyer-Leitzel A, Marsh J, Moynihan M, McKelvey S. Modeling seasonal influenza outbreak in a closed college campus: impact of pre-season vaccination, in-season vaccination and holidays/breaks. PLoS One. 2010;5(3):e9548. doi:10.1371/journal.pone.0009548.
- Pons VG, Canter J, Dolin R. Influenza A/USSR/77 (H1N1) on a university campus. Am J Epidemiol. 1980;111(1):23–30. doi:10.1093/oxfordjournals.aje.a112871.
- Centers for Disease Control and Prevention. CDC Guidance for Responses to Influenza for Institutions of Higher Education during the 2009–2010 Academic Year. Centers for Disease Control and Prevention, 2009.
- Kar-Purkayastha I, Ingram C, Maguire H, Roche A. The importance of school and social activities in the transmission of influenza A (H1N1) v: England, April–June 2009. Eurosurveillance. 2009;14(33):19311. doi:10.2807/ese.14.33.19311-en.
- Ryan KA, Filipp SL, Gurka MJ, Zirulnik A, Thompson LA. Understanding influenza vaccine perspectives and hesitancy in university students to promote increased vaccine uptake. Heliyon. 2019;5(10):e02604. doi:10.1016/j.heliyon.2019.e02604.
- Roberto AJ, Mongeau PA, Liu Y, Hashi EC. "Fear the flu, not the flu shot": a test of the extended parallel process model. J Health Commun. 2019;24(11):829–836. doi:10.1080/10810730.2019.1673520.
- Fall E, Izaute M, Chakroun-Baggioni N. How can the health belief model and self-determination theory predict both influenza vaccination and vaccination intention ? A longitudinal study among university students. Psychol Health. 2018;33(6):746–764. doi:10.1080/08870446.2017.1401623.
- Huang JJ, Francesconi M, Cooper MH, Covello A, Guo M, Gharib SD. Community health workers on a college campus: effects on influenza vaccination. J Am Coll Health. 2018;66(4):317–323. doi:10.1080/07448481.2018.1440582.
- Lee Y-I, Jin Y, Nowak G. Motivating influenza vaccination among young adults: the effects of public service advertising message framing and text versus image support. Soc Mark Q. 2018;24(2):89–103. doi:10.1177/1524500418771283.
- Rogers CJ, Bahr KO, Benjamin SM. Attitudes and barriers associated with seasonal influenza vaccination uptake among public health students; a cross-sectional study. BMC Public Health. 2018;18(1):1131. doi:10.1186/s12889-018-6041-1.
- Fu F, Christakis NA, Fowler JH. Dueling biological and social contagions. Sci Rep. 2017;7:43634. doi:10.1038/srep43634.
- Ratnapradipa KL, Norrenberns R, Turner JA, Kunerth A. Freshman flu vaccination behavior and intention during a nonpandemic season. Health Promot Pract. 2017;18(5):662–671. doi:10.1177/1524839917712731.
- Lu P-J, Srivastav A, Santibanez TA, et al. Knowledge of influenza vaccination recommendation and early vaccination uptake during the 2015-16 season among adults aged ≥18years - United States. Vaccine. 2017;35(34):4346–4354. doi:10.1016/j.vaccine.2017.06.074.
- Hashmi S, D’Ambrosio L, Diamond DV, Jalali MS, Finkelstein SN, Larson RC. Preventive behaviors and perceptions of influenza vaccination among a university student population. J Public Health (Oxf). 2016;38(4):739–745. doi:10.1093/pubmed/fdv189.
- Bronchetti ET, Huffman DB, Magenheim E. Attention, intentions, and follow-through in preventive health behavior: field experimental evidence on flu vaccination. J Econ Behav Organ. 2015;116:270–291. doi:10.1016/j.jebo.2015.04.003.
- Shropshire AM, Brent-Hotchkiss R, Andrews UK. Mass media campaign impacts influenza vaccine obtainment of university students. J Am Coll Health. 2013;61(8):435–443. doi:10.1080/07448481.2013.830619.
- Nichol KL, D’Heilly S, Ehlinger EP. Influenza vaccination among college and university students: impact on influenza-like illness, health care use, and impaired school performance. Arch Pediatr Adolesc Med. 2008;162(12):1113–1118. doi:10.1001/archpedi.162.12.1113.
- Schlenker EH, Tschetter RL, Straub HR. Influence of the H1N1 pandemic on university students knowledge of influenza. South Dakota Medicine 2013;66(11).
- Bednarczyk RA, Chu SL, Sickler H, Shaw J, Nadeau JA, McNutt L-A. Low uptake of influenza vaccine among university students: evaluating predictors beyond cost and safety concerns. Vaccine. 2015;33(14):1659–1663. doi:10.1016/j.vaccine.2015.02.033.
- Quinn SC, Hilyard KM, Jamison AM, et al. The influence of social norms on flu vaccination among African American and white adults. Health Educ Res. 2017;32(6):473–486. doi:10.1093/her/cyx070.
- Nyhan B, Reifler J, Richey S. The role of social networks in influenza vaccine attitudes and intentions among college students in the southeastern United States. J Adolesc Health. 2012;51(3):302–304. doi:10.1016/j.jadohealth.2012.02.014.
- Chien Y-H. Persuasiveness of online flu-vaccination promotional banners. Psychol Rep. 2013;112(2):365–374. doi:10.2466/01.13.PR0.112.2.365-374.
- Pew Reseach Center. Social media fact sheet. 2019. https://www.pewresearch.org/internet/fact-sheet/social-media/.
- Lee K, Agrawal A, Choudhary A. Real-time disease surveillance using Twitter data: demonstration on flu and cancer. Proceedings of the 19th ACM SIGKDD International Conference on Knowledge Discovery and Data Mining, pp. 1474–1477, 2013.
- Aslam AA, Tsou M-H, Spitzberg BH, et al. The reliability of tweets as a supplementary method of seasonal influenza surveillance. J Med Internet Res. 2014;16(11):e250. doi:10.2196/jmir.3532.
- Charles-Smith LE, Reynolds TL, Cameron MA, et al. Using social media for actionable disease surveillance and outbreak management: a systematic literature review. PLoS One. 2015;10(10):e0139701. doi:10.1371/journal.pone.0139701.
- Santillana M, Nguyen AT, Dredze M, Paul MJ, Nsoesie EO, Brownstein JS. Combining search, social media, and traditional data sources to improve influenza surveillance. PLoS Comput Biol. 2015;11(10):e1004513. doi:10.1371/journal.pcbi.1004513.
- Allen C, Tsou M-H, Aslam A, Nagel A, Gawron J-M. Applying GIS and machine learning methods to Twitter data for multiscale surveillance of influenza. PloS One. 2016;11(7):e0157734. doi:10.1371/journal.pone.0157734.
- Carneiro HA, Mylonakis E. Google trends: a web-based tool for real-time surveillance of disease outbreaks. Clin Infect Dis. 2009;49(10):1557–1564. doi:10.1086/630200.
- Dugas AF, Jalalpour M, Gel Y, et al. Influenza forecasting with Google flu trends. PloS One. 2013;8(2):e56176. doi:10.1371/journal.pone.0056176.
- Olson DR, Konty KJ, Paladini M, Viboud C, Simonsen L. Reassessing Google flu trends data for detection of seasonal and pandemic influenza: a comparative epidemiological study at three geographic scales. PLoS Comput Biol. 2013;9(10):e1003256. doi:10.1371/journal.pcbi.1003256.
- Nuti SV, Wayda B, Ranasinghe I, et al. The use of Google trends in health care research: a systematic review. PLoS One. 2014;9(10):e109583. doi:10.1371/journal.pone.0109583.
- Salathé M, Khandelwal S. Assessing vaccination sentiments with online social media: implications for infectious disease dynamics and control. PLoS Comput Biol. 2011;7(10):e1002199. doi:10.1371/journal.pcbi.1002199.
- Salathé M, Vu DQ, Khandelwal S, Hunter DR. The dynamics of health behavior sentiments on a large online social network. EPJ Data Sci. 2013;2(1):4. doi:10.1140/epjds16.
- Dunn AG, Leask J, Zhou X, Mandl KD, Coiera E. Associations between exposure to and expression of negative opinions about human papillomavirus vaccines on social media: an observational study. J Med Internet Res. 2015;17(6):e144. doi:10.2196/jmir.4343.
- Walton LR, Seitz HH, Ragsdale K. Strategic use of YouTube during a national public health crisis: the CDC’s response to the 2009 H1N1 flu epidemic. Case Stud Strateg Commun. 2012;1(3):25–37.
- @CDCFlu. July 2020. https://twitter.com/cdcflu?lang=en.
- Cauchemez S, Bhattarai A, Marchbanks TL, Pennsylvania H1N1 Working Group, et al. Role of social networks in shaping disease transmission during a community outbreak of 2009 H1N1 pandemic influenza. Proc Natl Acad Sci U S A. 2011;108(7):2825–2830. doi:10.1073/pnas.1008895108.
- Wheelock A, Parand A, Rigole B, et al. Socio-psychological factors driving adult vaccination: a qualitative study. PLoS One. 2014;9(12):e113503. doi:10.1371/journal.pone.0113503.
- Wheelock A, Thomson A, Sevdalis N. Social and psychological factors underlying adult vaccination behavior: lessons from seasonal influenza vaccination in the US and the UK. Expert Rev Vaccines. 2013;12(8):893–901. doi:10.1586/14760584.2013.814841.
- Koo M, Skinner H. Challenges of internet recruitment: a case study with disappointing results. J Med Internet Res. 2005;7(1):e6. doi:10.2196/jmir.7.1.e6.
- Lane TS, Armin J, Gordon JS. Online recruitment methods for web-based and mobile health studies: a review of the literature. J Med Internet Res. 2015;17(7):e183. doi:10.2196/jmir.4359.
- Von Ahn L, Blum M, Hopper NJ, Langford J. CAPTCHA: using hard AI problems for security. International Conference on the Theory and Applications of Cryptographic Techniques. Springer, 2003:294–311.
- Krumpal I. Determinants of social desirability bias in sensitive surveys: a literature review. Qual Quant. 2013;47(4):2025–2047. doi:10.1007/s11135-011-9640-9.
- Little RJ, Rubin DB. Statistical Analysis with Missing Data. Vol. 793. John Wiley & Sons, 2019.
- White IR, Royston P, Wood AM. Multiple imputation using chained equations: issues and guidance for practice. Stat Med. 2011;30(4):377–399. doi:10.1002/sim.4067.
- R Core Team. R: A Language and Environment for Statistical Computing. R Foundation for Statistical Computing, Vienna, Austria, 2019.
- van Buuren S, Groothuis-Oudshoorn K. Mice: multivariate imputation by chained equations in R. J Stat Softw 2011;45(3):1–67.
- FY, Hsieh DA, Bloch MD, Larsen A. simple method of sample size calculation for linear and logistic regression. Statist Med. 1998;17(14):1623–1634. doi:10.1002/(SICI)1097-0258(19980730)17:14<1623::AID-SIM871>3.0.CO;2-S.
- Newman ME. The structure and function of complex networks. SIAM Rev. 2003;45(2):167–256. doi:10.1137/S003614450342480.
- Mucha PJ, Richardson T, Macon K, Porter MA, Onnela J-P. Community structure in time-dependent, multiscale, and multiplex networks. Science. 2010;328(5980):876–878. doi:10.1126/science.1184819.
- Jutla IS, Jeub LG, Mucha PJ. A generalized Louvain method for community detection implemented in MATLAB. 2011. http://netwiki.amath.unc.edu/GenLouvain.
- Newman ME, Girvan M. Finding and evaluating community structure in networks. Phys Rev E Stat Nonlin Soft Matter Phys. 2004;69(2 Pt 2):026113. doi:10.1103/PhysRevE.69.026113.
- Newman ME. Finding community structure in networks using the eigenvectors of matrices. Phys Rev E Stat Nonlin Soft Matter Phys. 2006;74(3 Pt 2):036104. doi:10.1103/PhysRevE.74.036104.
- Tien JH, Eisenberg MC, Cherng ST, Porter MA. Online reactions to the 2017 ‘unite the right’ rally in Charlottesville: measuring polarization in Twitter networks using media followership. Appl Netw Sci. 2020;5(1):1–27. doi:10.1007/s41109-019-0223-3.
- Sánchez DL, Revuelta J, De la Prieta F, Gil-González AB, Dang C. Twitter user clustering based on their preferences and the Louvain algorithm. International Conference on Practical Applications of Agents and Multi-Agent Systems. Springer, 2016:349–356.
- Surian D, Nguyen DQ, Kennedy G, Johnson M, Coiera E, Dunn AG. Characterizing Twitter discussions about HPV vaccines using topic modeling and community detection. J Med Internet Res. 2016;18(8):e232. doi:10.2196/jmir.6045.
- Wilson EB. Probable inference, the law of succession, and statistical inference. J Am Stat Assoc. 1927;22(158):209–212. doi:10.1080/01621459.1927.10502953.
- Newcombe RG. Two-sided confidence intervals for the single proportion: comparison of seven methods. Statist Med. 1998;17(8):857–872. doi:10.1002/(SICI)1097-0258(19980430)17:8<857::AID-SIM777>3.0.CO;2-E.
- Newcombe RG. Interval estimation for the difference between independent proportions: comparison of eleven methods. Statist Med. 1998;17(8):873–890. doi:10.1002/(SICI)1097-0258(19980430)17:8<873::AID-SIM779>3.0.CO;2-I.
- Conover WJ. Practical Nonparametric Statistics. New York: Wiley, 1980.
- Marsaglia G, Tsang WW, Wang J. Evaluating Kolmogorov’s distribution. J Stat Soft. 2003;8(18):1–4. doi:10.18637/jss.v008.i18.
- Weinstein AT. Market Segmentation: Using Demographics, Psychographics and Other Niche Marketing Techniques to Predict Customer Behavior. Probus Publishing Co., 1994.
- McHugh ML. The chi-square test of independence. Biochem Med (Zagreb). 2013;23(2):143–149. doi:10.11613/bm.2013.018.
- MacDonald L, Cairns G, Angus K, De Andrade M. Promotional communications for influenza vaccination: a systematic review. J Health Commun. 2013;18(12):1523–1549. doi:10.1080/10810730.2013.840697.
- Uddin M, Cherkowski GC, Liu G, Zhang J, Monto AS, Aiello AE. Demographic and socioeconomic determinants of influenza vaccination disparities among university students. J Epidemiol Commun Health. 2010;64(9):808–813. doi:10.1136/jech.2009.090852.
- Nowak GJ, Sheedy K, Bursey K, Smith TM, Basket M. Promoting influenza vaccination: insights from a qualitative meta-analysis of 14 years of influenza-related communications research by us centers for disease control and prevention (CDC). Vaccine. 2015;33(24):2741–2756. doi:10.1016/j.vaccine.2015.04.064.
- Zottarelli LK, Sunil TS, Flott P, Karbhari S. College student adoption of non-pharmaceutical interventions during the 2009 H1N1 influenza pandemic: a study of two Texas universities in fall 2009. Prev Med. 2012;55(5):497–499. doi:10.1016/j.ypmed.2012.08.009.
- Winston CA, Wortley PM, Lees KA. Factors associated with vaccination of medicare beneficiaries in five US communities: results from the racial and ethnic adult disparities in immunization initiative survey, 2003. J Am Geriatr Soc. 2006;54(2):303–310. doi:10.1111/j.1532-5415.2005.00585.x.
- Tabbarah M, Zimmerman RK, Nowalk MP, et al. What predicts influenza vaccination status in older Americans over several years?J Am Geriatr Soc. 2005;53(8):1354–1359. doi:10.1111/j.1532-5415.2005.53424.x.
- Hull S, Hagdrup N, Hart B, Griffiths C, Hennessy E. Boosting uptake of influenza immunisation: a randomised controlled trial of telephone appointing in general practice. Brit J Gene Pract 2002;52(482):712–716.
- Dey P, Halder S, Collins S, Benons L, Woodman C. Promoting uptake of influenza vaccination among health care workers: a randomized controlled trial. J Public Health. 2001;23(4):346–348. doi:10.1093/pubmed/23.4.346.
- de Juanes JR, García de Codes A, Arrazola MP, Jaén F, Sanz MI, González A. Influenza vaccination coverage among hospital personnel over three consecutive vaccination campaigns (2001–2002 to 2003–2004). Vaccine. 2007;25(1):201–204. doi:10.1016/j.vaccine.2005.10.057.
- Nowak GJ, Gellin BG, MacDonald NE, Butler R, SAGE Working Group on Vaccine Hesitancy. Addressing vaccine hesitancy: the potential value of commercial and social marketing principles and practices. Vaccine. 2015;33(34):4204–4211. doi:10.1016/j.vaccine.2015.04.039.
- Barclay VC, Smieszek T, He J, et al. Positive network assortativity of influenza vaccination at a high school: implications for outbreak risk and herd immunity. PLoS One. 2014;9(2):e87042. doi:10.1371/journal.pone.0087042.
- Salathé M, Bonhoeffer S. The effect of opinion clustering on disease outbreaks. J R Soc Interface. 2008;5(29):1505–1508. doi:10.1098/rsif.2008.0271.
- Christakis NA, Fowler JH. Social network sensors for early detection of contagious outbreaks. PLoS One. 2010;5(9):e12948. doi:10.1371/journal.pone.0012948.
- CDC COVID Team Response. Severe outcomes among patients with coronavirus disease 2019 (COVID-19)—United States, February 12–March 16, 2020. MMWR. 2020;69(12):343–346.
- The Lancet Infectious Diseases. The COVID-19 infodemic. Lancet Infect Dis. 2020;20(8):875. doi:10.1016/S1473-3099(20)30565-X.
- Green J, Edgerton J, Naftel D, Shoub K, Cranmer SJ. Elusive consensus: polarization in elite communication on the COVID-19 pandemic. Sci. Adv. 2020;6(28):eabc2717. doi:10.1126/sciadv.abc2717.
- Baden LR, El Sahly HM, Essink B, et al. Efficacy and safety of the mRNA-1273 SARS-CoV-2 vaccine. N Engl J Med. 2021;384(5):403–416. doi:10.1056/NEJMoa2035389.
- Polack FP, Thomas SJ, Kitchin N, C4591001 Clinical Trial Group, et al. Safety and efficacy of the BNT162b2 mRNA Covid-19 vaccine. N Engl J Med. 2020;383(27):2603–2615. PMID: 33301246. doi:10.1056/NEJMoa2034577.
- Flannery B, Chung JR, Thaker SN, et al. Interim estimates of 2016–17 Seasonal Influenza Vaccine Effectiveness - United States, February 2017. MMWR Morb Mortal Wkly Rep. 2017;66(6):167–171. doi:10.15585/mmwr.mm6606a3.
- Flannery B, Chung JR, Belongia EA, et al. Interim estimates of 2017–18 seasonal influenza vaccine effectiveness - United States, February 2018. MMWR. 2018;67(6):180–185. doi:10.15585/mmwr.mm6706a2.
- Doyle JD, Chung JR, Kim SS, et al. Interim estimates of 2018–19 seasonal influenza vaccine effectiveness - United States, February 2019. MMWR. 2019;68(6):135–139. doi:10.15585/mmwr.mm6806a2.
- Moody J. Colleges requiring a coronavirus vaccine for fall. May 2021. https://www.usnews.com/education/best-colleges/articles/colleges-requiring-a-coronavirus-vaccine-for-fall-what-to-know.