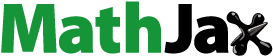
Abstract
Antimicrobial resistance results from the widespread use of antimicrobial agents and is a significant obstacle to the effectiveness of these agents. Numerous methods are used to overcome this problem with moderate success. Besides efforts of antimicrobial stewards, several artificial intelligence (AI)-based technologies are being explored for preventing resistance development. These first-generation systems mainly focus on improving patients’ adherence. Chronobiology is inherent in all biological systems. Host response to infections and pathogens activity are assumed to be affected by the circadian clock. This paper describes the problem of antimicrobial resistance and reviews some of the current AI technologies. We present the establishment of a second-generation AI chronobiology-based approach to help in preventing further resistance and possibly overcome existing resistance. An algorithm-controlled regimen that improves the long-term effectiveness of antimicrobial agents is being developed based on the implementation of variability in dosing and drug administration times. The method provides a means for ensuring a sustainable response and improved outcomes. Ongoing clinical trials determine the effectiveness of this second-generation system in chronic infections. Data from these studies are expected to shed light on a new aspect of resistance mechanisms and suggest methods for overcoming them.
IMPORTANCE SECTION
The paper presents the establishment of a second-generation AI chronobiology-based approach to help in preventing further resistance and possibly overcome existing resistance.
Antimicrobial resistance results from the widespread use of antimicrobial agents and is a significant obstacle to the effectiveness of these agents.
We present the establishment of a second-generation AI chronobiology-based approach to help in preventing further resistance and possibly overcome existing resistance.
Key messages
Introduction
In 1928, penicillin was discovered, and it took more than a decade for the drug to be widely used [Citation1]. Penicillin is still a cornerstone in the antimicrobial field almost a century later, although significant problems have emerged. Penicillinase, which inactivates penicillin, was identified before the commercial use of penicillin, suggesting an inherent property of some bacteria which have evolved over millennia preceding its discovery by humankind [Citation2]. Numerous antibiotic agents were developed, and accordingly, mechanisms of resistance evolved [Citation3]. More than 20,000 potential resistance genes (r genes) were identified [Citation4].
Nevertheless, although new antibiotics are being studied, the last antibiotics class was discovered in 1987, with Lipopeptides, specifically daptomycin [Citation5,Citation6]. With the rising prevalence of antibiotic resistance and the development of multidrug-resistant (MDR) strains, better strategies to overcome drug resistance are needed. Targeting the bacteria’s antimicrobial resistance mechanism is widely used, such as beta-lactamase inhibitors [Citation7]. Recent advances focus on targeting biofilms, quorum sensing, and other virulence factors [Citation8] and education for more judicious use of antibiotics [Citation9].
The development of resistance is a significant obstacle to antiviral therapy. Most active antiviral agents have been shown to select resistance mutations [Citation10]. Virus replication generates a large number of quasispecies. Viral resistance has implications for treatment regimens and the design of new therapeutics [Citation11].
The cyclical nature over a 24-h period is a fundamental feature conserved across most life on earth. Processes are compartmentalized concerning time in a manner that mirrors the rotation of the planet and accompanying diurnal cycles of light and darkness [Citation12]. Chronobiology is the study of biological rhythms, which examines the effects of time on biological events and internal biological clocks [Citation13]. Chronobiology is inherent in all biological systems. Host response to infections and pathogens activity are affected by the circadian clock [Citation14].
The paper reviews the relevant papers describing the mechanisms associated with drug resistance in chronic infections. We reviewed the relevant studies using digital platforms to deal with this problem, mainly by reminding patients to adhere to their treatments. It presents the establishment of a second-generation artificial intelligence (AI) chronobiology-based approach which implements personalized variability signatures to help prevent and possibly overcome existing resistance.
The problem of drug resistance in chronic infection and the role of chronobiology in the pathogenesis of infections
Several infections are prone to become chronic. The biological processes leading to cure failure at the acute stage, progression to chronicity, and lack of response to drugs are multifactorial, poorly understood, and associated with worse prognoses [Citation15–18].
Numerous antimicrobial resistance mechanisms affect a wide variety of antimicrobial agents [Citation19]. Following are prime examples of resistance-limited effective therapies for infectious diseases that often persist despite initial antimicrobial-active therapy:
Tuberculosis (TB) is the leading cause of death from an infectious disease. It is estimated that 1.7 billion people around the globe are infected with Mycobacterium tuberculosis, and reactivated disease is the most common manifestation in the elderly and immunocompromised patients [Citation20]. Drug-resistant TB accounts for 4–6% of TB cases and exceeds 25% in some areas, especially former soviet-union countries and India [Citation21]. The proposed solutions for MDR TB include prolonged courses of up to seven different agents associated with a high incidence of side effects, 45% of which are moderate to severe [Citation22].
Staphylococcus aureus (S. aureus) is the leading pathogen causing acute and chronic osteomyelitis. It produces biofilm, which increases bacterial antibiotic tolerability by up to 1000-fold [Citation23]. The mechanisms by which biofilm affects antibiotic resistance are not well understood, as some antibiotics can diffuse well through the extracellular matrix of the biofilm [Citation24]. Additional factors leading to treatment failure exist, including altered metabolic activity, slow bacterial growth, and intracellular survival of S. aureus [Citation25].
Chronic rhinosinusitis is one of the more prevalent chronic illnesses that evolves following the acute phase and is challenging to treat. Few of the commonly involved bacteria can appear in their drug-resistant form. For example, 20% of S. aureus species are methicillin-resistant, and Pseudomonas aeruginosa isolates showed a 27% resistance rate to quinolones and a 36% resistance rate to aminoglycosides [Citation26].
The World Health Organization has recognized Clarithromycin-resistant Helicobacter pylori as a ‘high priority’ issue, requiring new antibiotics. Resistance to metronidazole is also emerging. Tetracycline and amoxicillin resistance rates are still low but rising [Citation27]. These reported resistances led to profound treatment failures. When strains are resistant to clarithromycin, the eradication failure rate is as high as 88%, even if the bacteria are susceptible to other antibiotics in the regimen, while when strains are susceptible, the failure rate is 18% [Citation28]. In this setting, eradication failure may lead to severe complications, such as gastric cancer and peptic ulcer [Citation29].
These chronic infections represent some clinical challenges where initial failed therapy and rising antibiotic resistance rates complicate effective therapy. It is plausible that other host- and pathogen-related factors could enhance the effectiveness of antimicrobial treatment, thus limiting progression to chronicity.
Chronobiology examines timing processes, including periodic phenomena in biological systems and their adaptation to solar and lunar rhythms. These cycles are known as biological rhythms [Citation14,Citation30,Citation31]. Chronobiology plays a role in the function of multiple organs, including the immune system. Several inflammatory diseases, such as rheumatoid arthritis, myocardial infarctions, and ischemic stroke, have shown diurnal variation [Citation32].
The molecular clock’s critical aspects also control the immune system’s properties. The suprachiasmatic nucleus (SCN) is responsible for maintaining circadian rhythm. Its function is based on several transcription-translation feedback loops. The SCN also synchronizes the peripheral clocks found in most systems via the hypothalamic-pituitary-adrenal axis and the autonomic nervous system (ANS) [Citation33].
Several vital proteins, including CLOCK (Circadian locomotor output cycles kaput), BMAL1 [Brain and muscle aryl hydrocarbon receptor nuclear translocator (ARNT)-like 1], and REV-ERB have been implicated in immune function [Citation33,Citation34]. BMAL1 affects chemokines and can suppress them, thereby exerting an anti-inflammatory effect. REV-ERB
affects interleukin-6 and macrophage activity [Citation32]. The role of CLOCK is not fully understood, but its overexpression has been shown to increase the activity of the NF-
B complex [Citation33].
The number of mature circulating leukocytes is higher during the resting phase, and mature cells are released from the bone marrow at the commencement of this phase [Citation34]. Cytokine release is affected by the circadian rhythm, increasing proinflammatory cytokines in the resting phase. The circadian nature of the immune system impacts the response to vaccines. Studies on hepatitis A and influenza immunization showed higher antibody titers for those vaccinated in the morning [Citation35].
Numerous parasites, viruses, bacteria, and gut microbiome species manifest circadian rhythms [Citation14]. Studies in mice infected with Listeria monocytogenes showed that mice infected at the beginning of the rest phase have a higher colonization burden than at the end of the rest phase [Citation36]. Alternatively, mice infected with subspecies of Salmonella enterica have a higher bacterial load when infected in the rest phase than in the active phase [Citation37]. Studies done with Streptococcus pneumoniae, and Chlamydia muridarum also show circadian variation [Citation38]. The host’s circadian rhythm affects the gut microbiome, while some species, such as Klebsiella aerogenes, have an intrinsic rhythm [Citation38]. Last, for parasites, replication, and transmission of malaria, a pathogen with a high death toll, is negatively affected when not in sync with the host’s circadian rhythm [Citation39].
Increasing evidence suggests that bacteria have a circadian rhythm that may affect antibiotic resistance [Citation14]. Studies on S. aureus and clinically significant Enterobacteriaceae showed minimal inhibitory concentration (MIC) changes at certain times [Citation40,Citation41]. A study on cystic fibrosis patients showed that when Ceftazidime was administered in the morning, the blood’s drug concentration was higher compared to the afternoon [Citation42]. The administration of antibiotics at certain times of day can also be relevant to reducing adverse effects. For example, when aminoglycosides are administered during the resting phase, their nephrotoxicity is greatest [Citation43].
The circadian clock regulates various aspects of viral replication, host responses, and associated pathogenesis. A disrupted circadian clock has been linked to increased susceptibility to several viral-associated diseases. Viruses are obligate parasites reliant on their hosts for replication and dissemination. As more than 80% of protein-coding genes in various tissues show daily rhythmic expression, and given the dependency of virus replication on cellular pathways, host clock components directly or indirectly influence virus replication [Citation44]. Numerous factors that synchronize peripheral clocks, including the autonomic nervous system, body temperature, fasting/feeding cycles, and cytokines and hormones, may impact viral-mediated pathologies [Citation44]. Viruses can reprogramme cellular metabolism, affecting the mechanisms involved in circadian rhythm [Citation45]. Chronobiology is important for designing therapeutic strategies against SARS-CoV-2 and COVID-19, and other viruses [Citation46].
Using first-generation digital platforms for improving the effectiveness of anti-infectious agents
The use of digital platforms has steadily increased over the last decade [Citation47]. These include systems that analyze data for assisting in diagnosis and treatment and apps provided to patients to remind them to take their medications [Citation48,Citation49]. With the growing use of cellular phones and smartphones and the expanding coverage of mobile networks in low-resource and high-resource countries, an advancement was made in adapting digital platforms to various diseases and medical conditions [Citation50]. A significant adaptation of such is the development of multiple digital adherence technologies (DAT) [Citation51] in patients with human immunodeficiency virus (HIV) [Citation52], hepatitis C virus (HCV) [Citation53], and TB [Citation51].
Numerous studies in Africa and Asia have examined short messaging service (SMS) automated texts sent to patients’ family members’ phones, reminding them to take medications. In several intervention studies, patients could respond by returning an SMS or placing a telephone call when the task was performed. Such an intervention has a well-established adherence improvement in HIV and TB [Citation52,Citation54–56].
Several studies assessed instant live-streaming video conference calls allowing health care providers to perform a directly observed therapy (DOT) from a distance watching the patient take his/her medications at home. It was primarily used in high-income countries, such as Singapore [Citation57], United States [Citation58], and Mexico [Citation59].
Medication event and reminder monitoring consistent with digital pillboxes with a pre-determined reminder to take medications can improve patient adherence. Opening and closing the box is used as a digital indicator for regimen adherence and is stored on internal memory allowing the health care provider to access the dosing history created by the patient [Citation60,Citation61].
A novel platform comprises ingestible sensors implanted in medications for TB, HCV, HIV, and other medical conditions where adherence is crucial to avoid significant morbidity and mortality [Citation62]. Upon ingestion, the sensor interacts with the patient’s gastric fluid sending a signal transmitted to a monitor adhered to the patient’s skin, which sends a signal to the patient’s smartphone allowing sharing of the information with the patient’s physician.
These technologies, while improving adherence, do not assist in overcoming drug resistance.
Implementing a second-generation AI chronobiology-based regimens for overcoming drug resistance in infections
Implementing variability in administration times and dosing regimens was proposed to overcome resistance to chronic drugs [Citation63–74]. Drug holidays and dosage modifications were shown to improve clinical response to drugs [Citation75–80]. Drug holidays were evaluated while using chronic anti-infection agents [Citation81].
Preventing virological failure following HIV treatment is complicated by the emergence of drug resistance. A mathematical model that predicts HIV virological response toward various compounds was developed. The model is based on drug penetration into lymph nodes, refined adherence, pharmacokinetic and pharmacodynamic variability, drug interaction, and cross-resistance. The model showed that limited lymph node drug penetration accounts for a large proportion of virological failure and drug resistance [Citation82].
A stochastic model was developed that incorporates compartments of latently infected cells and virus genotypes with different drug susceptibilities. Parameters that promoted resistance included a high reproductive number, extended drug holidays, and poor adherence. The model supports the importance of the interactions between imperfect adherence, pharmacodynamics, pharmacokinetics, and latently infected cells as factors in therapy failure in HIV anti-retroviral therapy [Citation83].
Models were developed for using drug holidays to overcome drug resistance to infections. There is a nongenetic variation in phenotypes in bet-hedging that induces drug resistance in both bacterial infections. A model was developed to determine how bet-hedging emerges via a molecular switch in genotype-phenotype (GP) mapping. The model used stochastic switches that map gene products to phenotypes. Bet-hedging emerged in this model and is robust to evolutionary loss through mutations to both the expression of individual genes and the network itself. The data support the use of treatment holidays to combat bet-hedging-driven resistant disease. It implies that the structure of the GP mapping can determine the efficacy of treatment breaks [Citation84].
A digital system was developed for implementing variability and chronobiology-based regimen for overcoming drug resistance and improving the effectiveness of chronic medications [Citation30,Citation31,Citation65,Citation68,Citation69,Citation85–100]. Using variability to improve organ function was proposed based on studies showing its fundamental role in complex biological systems [Citation64,Citation70–73,Citation99–105]. This second-generation digital system overcomes some of the major obstacles associated with the lack of penetration of first-generation systems in everyday clinical practice [Citation74,Citation106,Citation107].
The second-generation system targets a clinically meaningful endpoint that ensures adaption by caregivers and adherence by patients. Thus, rather than just reminding patients to take the drug, which is inherent to most first-generating platforms, both patients and physicians view the system as part of the therapy, as they can follow up on the clinical effect. While large datasets are mandatory for most first-generation algorithms, the second-generation system implements the n = 1 concept, thus overcoming the hurdles of using unclean datasets that affect the algorithm outputs [Citation74,Citation106,Citation107].
The system is being developed in three steps [Citation107]. In the first step, the algorithm randomizes the administration times within the approved pharmacokinetic range. The algorithm can also randomize the dose administrated within the regulatory-approved range. Introducing variability is expected to prevent the development of compensatory mechanisms associated with drug resistance [Citation74].
In the second step, the algorithm personalizes the regimen using a closed-loop system that uses clinical and laboratory endpoints. At this step, individualized chronotherapy-based responses are being implemented, enabling further drug improvement. Using clinically meaningful endpoints adapts the algorithm to optimize the therapeutic regimen [Citation74,Citation106,Citation107].
In the third step, implementing signatures of variability, which are relevant to the chronobiology of the infections agents, or the host response, are implemented into the algorithm. The learning algorithm develops chronobiology signatures by identifying the ideal timing for administering an anti-infective agent in correlation with host, disease, and virulence factor-related outcomes. Additional signatures comprise ANS variables relevant to chronobiology, such as heart rate variability [Citation20,Citation69]; variability is pro and anti-inflammatory cytokines that determine the host response towards the infectious agent [Citation64,Citation92]; and modifications in the infectious agent epitopes and genes.
In summary, drug resistance is a significant obstacle to the use of most drugs, mainly in patients who require chronic therapeutic regimens. An algorithm-controlled regimen that improves the long-term effectiveness of anti-infectious agents is being developed. It provides a means for ensuring a sustainable response and improved outcomes. Ongoing clinical trials determine the effectiveness of second-generation systems in chronic diseases, including chronic infections. Data from these studies are expected to shed light on the resistance mechanism to anti-infectious agents and methods for overcoming them.
Author contributions
Yotam Kolben, Henny Azmanov, Ram Gelman, Danna Dror, and Yaron Ilan wrote the manuscript. Yaron Ilan conceptualized. All authors reviewed the final version of the manuscript. All authors have made substantial contributions to the design of the work and writing the manuscript. All authors are accountable for all aspects of the paper in ensuring that questions related to the accuracy or integrity of the work are appropriately investigated and resolved.
Disclosure statement
YI is the founder of Oberon Sciences.
Data availability statement
All data is on public domains.
References
- Gaynes R. The discovery of penicillin—new insights after more than 75 years of clinical use. Emerg Infect Dis. 2017;23(5):849–853.
- Abraham E. An enzyme from bacteria able to destroy penicillin. Nature 1940;146:837.
- Davies J, Davies D. Origins and evolution of antibiotic resistance. Microbiol Mol Biol Rev. 2010;74(3):417–433.
- Liu B, Pop M. ARDB–antibiotic resistance genes database. Nucleic Acids Res. 2009;37(Database issue):D443–D447.
- Hutchings MI, Truman AW, Wilkinson B. Antibiotics: past, present and future. Curr Opin Microbiol. 2019;51:72–80.
- Ling LL, Schneider T, Peoples AJ, et al. A new antibiotic kills pathogens without detectable resistance. Nature. 2015;517(7535):455–459.
- Watkins RR, Papp-Wallace KM, Drawz SM, et al. Novel β-lactamase inhibitors: a therapeutic hope against the scourge of multidrug resistance. Front Microbiol. 2013;4:392.
- Annunziato G. Strategies to overcome antimicrobial resistance (AMR) making use of non-essential target inhibitors: a review. Int J Mol Sci. 2019;20(23):5844.
- Charani E, Castro-Sanchéz E, Bradley S, et al. Implementation of antibiotic stewardship in different settings – results of an international survey. Antimicrob Resist Infect Control. 2019;8:34.
- Nijhuis M, van Maarseveen NM, Boucher CA. Antiviral resistance and impact on viral replication capacity: evolution of viruses under antiviral pressure occurs in three phases. Handb Exp Pharmacol. 2009;189:299–320.
- Mason S, Devincenzo JP, Toovey S, et al. Comparison of antiviral resistance across acute and chronic viral infections. Antiviral Res. 2018;158:103–112.
- McKenna H, van der Horst GTJ, Reiss I, et al. Clinical chronobiology: a timely consideration in critical care medicine. Crit Care. 2018;22(1):124.
- Çalıyurt O. Role of chronobiology as a transdisciplinary field of research: its applications in treating mood disorders. Balkan Med J. 2017;34(6):514–521.
- Diallo AB, Coiffard B, Leone M, et al. For whom the clock ticks: clinical chronobiology for infectious diseases. Front Immunol. 2020;11:1457.
- Okoye IS, Houghton M, Tyrrell L, et al. Coinhibitory receptor expression and immune checkpoint blockade: maintaining a balance in CD8(+) T cell responses to chronic viral infections and cancer. Front Immunol. 2017;8:1215.
- Shrestha L, Fan HM, Tao HR, et al. Recent strategies to combat biofilms using antimicrobial agents and therapeutic approaches. Pathogens. 2022;11(3):292.
- Nadar S, Khan T, Patching SG, et al. Development of antibiofilm therapeutics strategies to overcome antimicrobial drug resistance. Microorganisms. 2022;10(2):303.
- Mogensen TH. Genetic susceptibility to viral disease in humans. Clin Microbiol Infect. 2022;28(11):1411–1416.
- Reygaert WC. An overview of the antimicrobial resistance mechanisms of bacteria. AIMS Microbiol. 2018;4(3):482–501.
- Furin J, Cox H, Pai M. Tuberculosis. Lancet. 2019;393(10181):1642–1656.
- World Health Organization. Global tuberculosis report 2018. Geneva: World Health Organization; 2018.
- Conradie F, Diacon AH, Ngubane N, et al. Treatment of highly drug-resistant pulmonary tuberculosis. N Engl J Med. 2020;382(10):893–902.
- Jefferson KK, Goldmann DA, Pier GB. Use of confocal microscopy to analyze the rate of vancomycin penetration through Staphylococcus aureus biofilms. Antimicrob Agents Chemother. 2005;49(6):2467–2473.
- Daddi Oubekka S, Briandet R, Fontaine-Aupart MP, et al. Correlative time-resolved fluorescence microscopy to assess antibiotic diffusion-reaction in biofilms. Antimicrob Agents Chemother. 2012;56(6):3349–3358.
- Gimza BD, Cassat JE. Mechanisms of antibiotic failure during. Front Immunol. 2021;12:638085.
- Manes RP, Batra PS. Bacteriology and antibiotic resistance in chronic rhinosinusitis. Facial Plast Surg Clin North Am. 2012;20(1):87–91.
- Alba C, Blanco A, Alarcón T. Antibiotic resistance in Helicobacter pylori. Curr Opin Infect Dis. 2017;30(5):489–497.
- Mégraud F. H. pylori antibiotic resistance: prevalence, importance, and advances in testing. Gut. 2004;53(9):1374–1384.
- Tshibangu-Kabamba E, Yamaoka Y. Helicobacter pylori infection and antibiotic resistance – from biology to clinical implications. Nat Rev Gastroenterol Hepatol. 2021;18(9):613–629.
- Kolben Y, Weksler-Zangen S, Ilan Y. Adropin as a potential mediator of the metabolic system-autonomic nervous system-chronobiology axis: implementing a personalized signature-based platform for chronotherapy. Obes Rev. 2021;22(2):e13108.
- Potruch A, Khoury ST, Ilan Y. The role of chronobiology in drug-resistance epilepsy: the potential use of a variability and chronotherapy-based individualized platform for improving the response to anti-seizure drugs. Seizure. 2020;80:201–211.
- Fagiani F, Di Marino D, Romagnoli A, et al. Molecular regulations of circadian rhythm and implications for physiology and diseases. Signal Transduct Target Ther. 2022;7(1):41.
- Curtis AM, Bellet MM, Sassone-Corsi P, et al. Circadian clock proteins and immunity. Immunity. 2014;40(2):178–186.
- Scheiermann C, Kunisaki Y, Frenette PS. Circadian control of the immune system. Nat Rev Immunol. 2013;13(3):190–198.
- Schmitz NCM, van der Werf YD, Lammers-van der Holst HM. The importance of sleep and circadian rhythms for vaccination success and susceptibility to viral infections. Clocks Sleep. 2022;4(1):66–79.
- Nguyen KD, Fentress SJ, Qiu Y, et al. Circadian gene Bmal1 regulates diurnal oscillations of Ly6C(hi) inflammatory monocytes. Science. 2013;341(6153):1483–1488.
- Bellet MM, Deriu E, Liu JZ, et al. Circadian clock regulates the host response to Salmonella. Proc Natl Acad Sci USA. 2013;110(24):9897–9902.
- Rijo-Ferreira F, Takahashi JS. Circadian rhythms in infectious diseases and symbiosis. Semin Cell Dev Biol. 2022;126:37–44.
- O'Donnell AJ, Schneider P, McWatters HG, et al. Fitness costs of disrupting circadian rhythms in malaria parasites. Proc Biol Sci. 2011;278(1717):2429–2436.
- Perunova NB, Fadeev SB, Paromova II, et al. Circadian rhythms of antibacterial resistance, coagulase and antilysozyme activity of Staphylococcus aureus. Zh Mikrobiol Epidemiol Immunobiol. 2008 May-Jun;(3):6–9.
- Bukharin OV, Perunova NB, Fadeev SB, et al. Biorhythms of antibiotic resistance of microorganisms. Z Mikrobiol Epidemiol Immunobiol. 2008;5:35–38.
- Vinks AA, Touw DJ, Heijerman HG, et al. Pharmacokinetics of ceftazidime in adult cystic fibrosis patients during continuous infusion and ambulatory treatment at home. Ther Drug Monit. 1994;16(4):341–348.
- Beauchamp D, Labrecque G. Chronobiology and chronotoxicology of antibiotics and aminoglycosides. Adv Drug Deliv Rev. 2007;59(9–10):896–903.
- Borrmann H, McKeating JA, Zhuang X. The circadian clock and viral infections. J Biol Rhythms. 2021;36(1):9–22.
- Blanco JR, Verdugo-Sivianes EM, Amiama A, et al. The circadian rhythm of viruses and its implications on susceptibility to infection. Expert Rev anti Infect Ther. 2022;20(8):1109–1117.
- Sengupta S, Ince L, Sartor F, et al. Clocks, viruses, and immunity: lessons for the COVID-19 pandemic. J Biol Rhythms. 2021;36(1):23–34.
- Kvedar JC, Fogel AL, Elenko E, et al. Digital medicine’s march on chronic disease. Nat Biotechnol. 2016;34(3):239–246.
- Senbekov M, Saliev T, Bukeyeva Z, et al. The recent progress and applications of digital technologies in healthcare: a review. Int J Telemed Appl. 2020;2020:8830200.
- Awad A, Trenfield SJ, Pollard TD, et al. Connected healthcare: improving patient care using digital health technologies. Adv Drug Deliv Rev. 2021;178:113958.
- Duncombe RA. Understanding the impact of mobile phones on livelihoods in developing countries. Dev Pol Rev. 2014;32(5):567–588.
- Subbaraman R, de Mondesert L, Musiimenta A, et al. Digital adherence technologies for the management of tuberculosis therapy: mapping the landscape and research priorities. BMJ Glob Health. 2018;3(5):e001018.
- Pop-Eleches C, Thirumurthy H, Habyarimana JP, et al. Mobile phone technologies improve adherence to anti-retroviral treatment in a resource-limited setting: a randomized controlled trial of text message reminders. AIDS. 2011;25(6):825–834.
- Beaulac J, Balfour L, Corace K, et al. Access and preferences for mobile technology among diverse hepatitis C patients: implications for expanding treatment care. J MTM. 2019;8(1):11–19.
- Bediang G, Stoll B, Elia N, et al. SMS reminders to improve the tuberculosis cure rate in developing countries (TB-SMS Cameroon): a protocol of a randomised control study. Trials. 2014;15(1):35–39.
- Nhavoto JA, Grönlund Å, Klein GO. Mobile health treatment support intervention for HIV and tuberculosis in Mozambique: perspectives of patients and healthcare workers. PLOS One. 2017;12(4):e0176051.
- Liu X, Lewis JJ, Zhang H, et al. Effectiveness of electronic reminders to improve medication adherence in tuberculosis patients: a cluster-randomised trial. PLOS Med. 2015;12(9):e1001876.
- Molton JS, Pang Y, Wang Z, et al. Prospective single-arm interventional pilot study to assess a smartphone-based system for measuring and supporting adherence to medication. BMJ Open. 2016;6(12):e014194.
- Chuck C, Robinson E, Macaraig M, et al. Enhancing management of tuberculosis treatment with video directly observed therapy in New York city. Int J Tuberc Lung Dis. 2016;20(5):588–593.
- Garfein RS, Collins K, Muñoz F, et al. Feasibility of tuberculosis treatment monitoring by video directly observed therapy: a binational pilot study. Int J Tuberc Lung Dis. 2015;19(9):1057–1064.
- Liu X, Blaschke T, Thomas B, et al. Usability of a medication event reminder monitor system (MERM) by providers and patients to improve adherence in the management of tuberculosis. IJERPH. 2017;14(10):1115.
- de Sumari-de Boer IM, van den Boogaard J, Ngowi KM, et al. Feasibility of real time medication monitoring among HIV infected and TB patients in a resource-limited setting. AIDS Behav. 2016;20(5):1097–1107.
- Chai PR, Vaz C, Goodman GR, et al. Ingestible electronic sensors to measure instantaneous medication adherence: a narrative review. Digit Health. 2022;8:20552076221083119.
- Shabat Y, Lichtenstein Y, Ilan Y. Short-term cohousing of sick with healthy or treated mice alleviates the inflammatory response and liver damage. Inflammation. 2021;44(2):518–525.
- El-Haj M, Kanovitch D, Ilan Y. Personalized inherent randomness of the immune system is manifested by an individualized response to immune triggers and immunomodulatory therapies: a novel platform for designing personalized immunotherapies. Immunol Res. 2019;67(4–5):337–347.
- Ilan Y. Randomness in microtubule dynamics: an error that requires correction or an inherent plasticity required for normal cellular function? Cell Biol Int. 2019;43(7):739–748.
- Ilan Y. Microtubules: from understanding their dynamics to using them as potential therapeutic targets. J Cell Physiol. 2019;234(6):7923–7937.
- Ilan-Ber T, Ilan Y. The role of microtubules in the immune system and as potential targets for gut-based immunotherapy. Mol Immunol. 2019;111:73–82.
- Forkosh E, Kenig A, Ilan Y. Introducing variability in targeting the microtubules: review of current mechanisms and future directions in colchicine therapy. Pharmacol Res Perspect. 2020;8(4):e00616.
- Ilan Y. Beta-glycosphingolipids as mediators of both inflammation and immune tolerance: a manifestation of randomness in biological systems. Front Immunol. 2019;10:1143.
- Ilan Y. Overcoming randomness does not rule out the importance of inherent randomness for functionality. J Biosci. 2019;44(6):132.
- Ilan Y. Generating randomness: making the most out of disordering a false order into a real one. J Transl Med. 2019;17(1):49.
- Ilan Y. Advanced tailored randomness: a novel approach for improving the efficacy of biological systems. J Comput Biol. 2020;27(1):20–29.
- Ilan Y. Order through disorder: the characteristic variability of systems. Front Cell Dev Biol. 2020;8:186.
- Ilan Y. Overcoming compensatory mechanisms toward chronic drug administration to ensure long-term, sustainable beneficial effects. Mol Ther Methods Clin Dev. 2020;18:335–344.
- Uehata N, Kouzu K, Tsujimoto H, et al. Long-term survival achieved by repeated administration of ramucirumab after drug holidays due to proteinuria in recurrent gastric cancer. Surg Case Rep. 2021;7(1):97.
- Hayes KN, Winter EM, Cadarette SM, et al. Duration of bisphosphonate drug holidays in osteoporosis patients: a narrative review of the evidence and considerations for decision-making. J Clin Med. 2021;10(5):1140.
- Garattini SK, Basile D, Bonotto M, et al. Drug holidays and overall survival of patients with metastatic colorectal cancer. Cancers. 2021;13(14):3504.
- Nayak S, Greenspan SL. Bisphosphonate drug holidays. Osteoporos Int. 2019;30(12):2525.
- Tanaka M, Kinoshita M, Tanaka K. Intermittent drug holidays in fingolimod therapy for multiple sclerosis. Mult Scler. 2018;24(2):236–237.
- Ibrahim K, Donyai P. Drug holidays from ADHD medication: international experience over the past four decades. J Atten Disord. 2015;19(7):551–568.
- Lau CL, Ramsey L, Mills LC, et al. Drug-free holidays: compliance, tolerability, and acceptability of a 3-day atovaquone/proguanil schedule for pretravel malaria chemoprophylaxis in Australian travelers. Clin Infect Dis. 2019;69(1):137–143.
- Sanche S, Sheehan N, Mesplede T, et al. A mathematical model to predict HIV virological failure and elucidate the role of lymph node drug penetration. CPT Pharmacometrics Syst Pharmacol. 2017;6(7):469–476.
- Cadosch D, Bonhoeffer S, Kouyos R. Assessing the impact of adherence to anti-retroviral therapy on treatment failure and resistance evolution in HIV. J R Soc Interface. 2012;9(74):2309–2320.
- Nichol D, Robertson-Tessi M, Jeavons P, et al. Stochasticity in the genotype-phenotype map: implications for the robustness and persistence of bet-hedging. Genetics. 2016;204(4):1523–1539.
- Kessler A, Weksler-Zangen S, Ilan Y. Role of the immune system and the circadian rhythm in the pathogenesis of chronic pancreatitis: establishing a personalized signature for improving the effect of immunotherapies for chronic pancreatitis. Pancreas. 2020;49(8):1024–1032.
- Ilan Y, Spigelman Z. Establishing patient-tailored variability-based paradigms for anti-cancer therapy: using the inherent trajectories which underlie cancer for overcoming drug resistance. Cancer Treat Res Commun. 2020;25:100240.
- Gelman R, Bayatra A, Kessler A, et al. Targeting SARS-CoV-2 receptors as a means for reducing infectivity and improving antiviral and immune response: an algorithm-based method for overcoming resistance to antiviral agents. Emerg Microbes Infect. 2020;9(1):1397–1406.
- Ishay Y, Kolben Y, Kessler A, et al. Role of circadian rhythm and autonomic nervous system in liver function: a hypothetical basis for improving the management of hepatic encephalopathy. Am J Physiol Gastrointest Liver Physiol. 2021;321(4):G400–G412.
- Hurvitz N, Azmanov H, Kesler A, et al. Establishing a second-generation artificial intelligence-based system for improving diagnosis, treatment, and monitoring of patients with rare diseases. Eur J Hum Genet. 2021;29(10):1485–1490.
- Azmanov H, Ross EL, Ilan Y. Establishment of an individualized chronotherapy, autonomic nervous system, and variability-based dynamic platform for overcoming the loss of response to analgesics. Pain Physician. 2021;24(3):243–252.
- Khoury T, Ilan Y. Platform introducing individually tailored variability in nerve stimulations and dietary regimen to prevent weight regain following weight loss in patients with obesity. Obes Res Clin Pract. 2021;15(2):114–123.
- Khoury T, Ilan Y. Introducing patterns of variability for overcoming compensatory adaptation of the immune system to immunomodulatory agents: a novel method for improving clinical response to anti-TNF therapies. Front Immunol. 2019;10:2726.
- Kenig A, Ilan Y. A personalized signature and chronotherapy-based platform for improving the efficacy of sepsis treatment. Front Physiol. 2019;10:1542.
- Ilan Y. Why targeting the microbiome is not so successful: can randomness overcome the adaptation that occurs following gut manipulation? Clin Exp Gastroenterol. 2019;12:209–217.
- Ishay Y, Kolben Y, Kessler A, et al. Role of circadian rhythm and autonomic nervous system in liver function: a hypothetical basis for improving the management of hepatic encephalopathy. Am J Physiol Gastrointest Liver Physiol. 2021;321(4):G400–G412.
- Kenig A, Kolben Y, Asleh R, et al. Improving diuretic response in heart failure by implementing a patient-tailored variability and chronotherapy-guided algorithm. Front Cardiovasc Med. 2021;8:695547.
- Isahy Y, Ilan Y. Improving the long-term response to antidepressants by establishing an individualized platform based on variability and chronotherapy. Int J Clin Pharmacol Ther. 2021;59(12):768–774.
- Ishay Y, Potruch A, Schwartz A, et al. A digital health platform for assisting the diagnosis and monitoring of COVID-19 progression: an adjuvant approach for augmenting the antiviral response and mitigating the immune-mediated target organ damage. Biomed Pharmacother. 2021;143:112228.
- Ilan Y. Digital medical cannabis as market differentiator: second-generation artificial intelligence systems to improve response. Front Med. 2021;8:788777.
- Gelman R, Berg M, Ilan Y. A subject-tailored variability-based platform for overcoming the plateau effect in sports training: a narrative review. Int J Environ Res Public Health. 2022;19(3):1722.
- Ilan Y. The constrained disorder principle defines living organisms and provides a method for correcting disturbed biological systems. Comput Struct Biotechnol J. 2022;20:6087–6096.
- Ilan Y. Next-Generation personalized medicine: implementation of variability patterns for overcoming drug resistance in chronic diseases. J Pers Med. 2022;12(8):1303.
- Ilan Y. Microtubules as a potential platform for energy transfer in biological systems: a target for implementing individualized, dynamic variability patterns to improve organ function. Mol Cell Biochem. 2022. doi: 10.1007/s11010-022-04513-1.
- Rotnemer-Golinkin D, Ilan Y. Personalized-inherent variability in a time-dependent immune response: a look into the fifth dimension in biology. Pharmacology. 2022;107(7–8):417–422.
- Azmanov H, Bayatra A, Ilan Y. Digital analgesic comprising a second-generation digital health system: increasing effectiveness by optimizing the dosing and minimizing side effects. J Pain Res. 2022;15:1051–1060.
- Ilan Y. Second-generation digital health platforms: placing the patient at the center and focusing on clinical outcomes. Front Digit Health. 2020;2:569178.
- Ilan Y. Improving global healthcare and reducing costs using second-generation artificial intelligence-based digital pills: a market disruptor. Int J Environ Res Public Health. 2021;18(2):811.