Abstract
Background
Asthma, a prevalent chronic respiratory disorder, remains enigmatic, notwithstanding considerable advancements in our comprehension. Continuous efforts are crucial for discovering novel molecular targets and gaining a comprehensive understanding of its pathogenesis.
Materials and methods
In this study, we analyzed gene expression data from 212 individuals, including asthma patients and healthy controls, to identify 267 differentially expressed genes, among which C1orf64 and C7orf26 emerged as potential key genes in asthma pathogenesis. Various bioinformatics tools, including differential gene expression analysis, pathway enrichment, drug target prediction, and single-cell analysis, were employed to explore the potential roles of the genes.
Results
Quantitative PCR demonstrated differential expression of C1orf64 and C7orf26 in the asthmatic airway epithelial tissue, implying their potential involvement in asthma pathogenesis. GSEA enrichment analysis revealed significant enrichment of these genes in signaling pathways associated with asthma progression, such as ABC transporters, cell cycle, CAMs, DNA replication, and the Notch signaling pathway. Drug target prediction, based on upregulated and downregulated differential expression, highlighted potential asthma treatments, including Tyrphostin-AG-126, Cephalin, Verrucarin-a, and Emetine. The selection of these drugs was based on their significance in the analysis and their established anti-inflammatory and antiviral invasion properties. Utilizing Seurat and Celldex packages for single-cell sequencing analysis unveiled disease-specific gene expression patterns and cell types. Expression of C1orf64 and C7orf26 in T cells, NK cells, and B cells, instrumental in promoting hallmark features of asthma, was observed, suggesting their potential influence on asthma development and progression.
Conclusion
This study uncovers novel genetic aspects of asthma, highlighting potential therapeutic pathways. It exemplifies the power of integrative bioinformatics in decoding complex disease patterns. However, these findings require further validation, and the precise roles of C1orf64 and C7orf26 in asthma warrant additional investigation to validate their therapeutic potential.
Introduction
Asthma is a prevalent non-communicable disease worldwide, typically characterized by complex chronic airway inflammation, mainly manifested as symptoms such as difficulty in breathing, wheezing, chest tightness, and coughing, severely impacting the daily life and work capacity of patients [Citation1,Citation2]. Globally, asthma is known to affect approximately 339 million individuals, with the majority being children and adolescents [Citation3]. The impact of asthma extends beyond the burden it imposes on healthcare systems worldwide; it substantially diminishes the quality of life for patients and their families [Citation4]. Despite considerable research on the etiology and treatment of asthma, its pathogenesis remains unclear, and certain limitations and deficiencies in the treatment methods exist [Citation5]. Hence, further extensive studies on the causes and remedies for asthma is necessary.
Bioinformatics is an interdisciplinary science whose applications have permeated various fields of life sciences, including genomics, transcriptomics, proteomics, and metabolomics [Citation6,Citation7]. The continuous progress of science and the deepening of bioinformatics research, more biological information data can be generated and shared. The generation of these data provides new ideas and methods for biomedical research and has become a powerful tool for studying diseases. Bioinformatics provides us with a more comprehensive approach to disease research, allowing us to gain deeper insights into the mechanisms and etiology of different diseases, and providing more effective methods for disease prevention, diagnosis, and treatment.
This research employed the Gene Expression Omnibus (GEO) repository, accessible through the US National Center for Biotechnology Information (NCBI) website (https://www.ncbi.nlm.nih.gov/geo/), to analyze Differentially Expressed Genes (DEGs) in individuals with asthma and those without the condition (). Various bioinformatics techniques were employed to examine and expound on the functions and linked pathways of these DEGs. In addition, we explored the application of gene co-expression networks and random forest algorithms in asthma research, and used GSEA and CMap to predict possible drug treatment options for asthma patients. This study is of great significance for exploring the pathogenesis of bronchial asthma, discovering potential therapeutic targets, and developing new treatment methods. Our objective is to provide novel insights and orientations for the exploration and management of asthma.
Materials and methods
Data download
The GSE43696 and GSE67940 datasets were acquired from the NCBI GEO public database [Citation8–11]. Both datasets utilized the GPL6480 analysis file and consisted of expression profile data from patients. GSE43696 included 108 patients, with 20 in the normal group and 88 in the disease group; while GSE67940 included 104 patients, with 31 in the normal group and 73 in the disease group.
Analysis of GO and KEGG functions
The clusterProfiler was utilized to carry out functional annotation to explore thoroughly the functional correlation among the distinct genes (The access date of the data is 23 February 2023) [Citation12]. The functional groups were assessed through Gene Ontology (GO) and Kyoto Encyclopedia of Genes and Genomes (KEGG). A functional group was considered significant when the enriched pathways of both GO and KEGG had p-values and q-values below 0.05.
Construction of MEGENA co-expression network
The Multiscale Embedded Gene Co-Expression Network Analysis (MEGENA) was employed to construct gene co-expression networks (the access date of the data is 23 February 2023) [Citation13]. Primarily, MEGENA employs a multiscale embedded algorithm for the construction of gene co-expression networks. This algorithm accomplishes the transposition of high-dimensional gene expression data into points on a three-dimensional topological sphere by mapping gene expression data onto a low-dimensional space. Each point symbolizes a gene, interconnected based on their mutual similarity. Subsequently, MEGENA calculates the correlation between each pair of linked genes. It utilizes correlation coefficients to quantify the correlation between genes. A higher correlation value signifies an increased similarity in expression patterns between two genes. Selection is made for gene pairs exhibiting significant correlation (FDR < 0.05). The meaningful gene pairs were subsequently sorted according to the Pearson correlation coefficient. Each gene pair was tested using the sorted genes pirs to determine whether it could be placed on the 3D topological sphere without passing through other nodes. The co-expression network obtained is categorized as planar filter networks (PFN), which are geometric networks that can be embedded on a surface of a sphere without intersecting connections. PFN identifies network clusters, also known as gene modules, at various resolutions through unsupervised clustering using multiscale cluster analysis (MCA). MCA partitions the main module by exploring and optimizing it into multiple submodules. Ultimately, we identified key genes for further analysis by examining genes with relatively high node values in critical modules.
Random forest feature selection
Random forest is an ensemble learning technique that utilizes a decision tree-based learner (the access date of the data is 23 February 2023) [Citation14]. It employs sampling and returning methodology to select multiple samples from the dataset as the training set and creates a decision tree using the sample dataset. At each generated node, features are selected randomly without repetition, and these features are employed to split the dataset, identify the optimal partitioning features, and predict outcomes. In this investigation, feature importance is assessed by the random forest algorithm using %IncMSE, and the top 5 important features are chosen for further analysis.
GSEA pathway enrichment analysis
GSEA analysis entails taking a pre-established gene set and ranking genes based on the extent of differential expression between two sample types (the access date of the data is 23 February 2023). Subsequently, the analysis assesses whether the gene set is enriched at the top or bottom of the list [Citation15]. In this specific research, GSEA was utilized to explore the disparities in KEGG signaling pathways between individuals with high and low gene expression. The purpose of this analysis was to reveal the underlying molecular mechanisms of core genes in both groups. The analysis was executed with 1000 permutations, and the phenotype was employed as the permutation type.
Cmap drug prediction
The Broad Institute developed the Connectivity Map (CMap) as a database for gene expression profiling that relies on intervention gene expression (the access date of the data is 24 February 2023) [Citation16]. Its primary purpose is to elucidate the functional relationship that exists between small molecule compounds, genes, and disease states. The database comprises microarray data that shows the gene expression before and after treatment with 1309 different small molecule drugs across five human cell lines. The treatments were conducted under varying conditions, including different drugs, concentrations, and treatment durations [Citation17,Citation18]. In this study, the DEGs of diseases are used to predict the targeted therapeutic drugs of diseases. The differentially upregulated and downregulated genes were uploaded to the cmap database to obtain potential compounds implicated by these differential genes. The 2D structural diagrams of these compounds were subsequently retrieved from the pubchem database for visualization.
Single-cell sequencing analysis
Firstly, the data was processed using the Seurat package, and the tSNE algorithm was used to analyze the positional relationships between each cluster (the access date of the data is 24 February 2023). The clusters were then annotated using the celldex package and were assigned to specific cell types that have an important relationship with disease [Citation19]. Finally, we employed the FindAllMarkers feature to derive the marker genes for each cell subtype from the single-cell gene expression profile, with a logfc.threshold setting of 1. Genes having |avg log2FC| > 1 and a p value adj < 0.05 were selected as characteristic marker genes for each cell subtype.
Fluorescence quantitative PCR detection of key gene expression
The research participants comprised of five bronchial asthma patients admitted to the Second Affiliated Hospital of Guangxi Medical University between October 2022 and May 2023. The group comprised of three males and two females aged between 25 to 60 years, and all the enrolled patients met the diagnostic criteria for bronchial asthma formulated by the Chinese Medical Association. The normal control group consisted of 4 males and 1 female who underwent fiberoptic bronchoscopy examination, aged 30–55 years old, and the airway mucosal tissue was obtained by bronchial mucosal biopsy (). qRT-PCR was used to validate the expression of hub genes in each group of mucosal tissue samples. According to the instructions of the kit, TRIzol reagent was used to process the mucosal tissue to extract total RNA. The RNA concentration and purity of each group were measured, and the standard value was set according to the A260/A280 value between 1.00–2.00. For each RNA sample (1 μg), cDNA was synthesized through reverse transcription, followed by PCR amplification with cDNA serving as a template. The 2−ΔΔCt method was utilized to assess the relative expression level of the target gene.
Table 1. The clinical characteristics and treatment history of the cases.
Statistical analysis
Statistical analyses were conducted using the R programming language (version 4.2.2) and following data packages: clusterProfiler: Used for functional enrichment analysis. MEGENA: Employed for module-based gene set analysis. Randomforest: Utilized for predictive modeling. GSEA: Applied for gene set enrichment analysis. Cmap: Used for drug sensitivity analysis. Seurat: Employed for single-cell RNA sequencing data analysis. The Wilcoxon test was used to compare the two cohorts, and Pearson’s method was applied for all correlational examinations. Significance levels were set at p < 0.05.
Informed consent statement
We have strictly adhered to the ethical principles concerning human participants in this research endeavor. Before their engagement in the study, all participants were comprehensively briefed about its objectives, methodologies, potential risks, and possible benefits. Moreover, they were duly apprised of their right to voluntary participation and the liberty to withdraw from the study at any given time without encountering any adverse repercussions. Subsequently, following the assurance of complete comprehension from each participant, we obtained written informed consent documents duly signed by all individuals involved. Furthermore, we shall be unwavering in upholding the confidentiality and privacy of the participants, utilizing the data exclusively for research purposes, thus ensuring the impervious protection of their personal identity information against any potential disclosure. This study has garnered approval from the esteemed Ethics Committee of the Second Affiliated Hospital of Guangxi Medical University (Approval number: 2022-KY(0777)), which is entrusted with reviewing and supervising the ethical dimensions of research involving human participants.
Results
Screening and functional enrichment of differential genes
We obtained the GSE43696 dataset from the NCBI GEO public database, which consisted of 108 patients, including 20 normal controls and 88 patients with the disease. To identify DEGs between the two groups of patients, we employed the limma package with a screening criterion of p-value < 0.05 and |logFC| > 0.585. A total of 267 DEGs were identified, comprising 116 upregulated genes and 151 downregulated genes (). The DEGs were analyzed further with respect to their pathways. GO enrichment analysis indicated that these candidate genes were primarily enriched in signaling receptor activator activity, response to molecule of bacterial origin, and receptor ligand activity pathways (). Furthermore, KEGG analysis demonstrated that these candidate genes were mainly enriched in IL-17 signaling pathway, cytokine-cytokine receptor interaction, and TNF signaling pathway ().
Identification of key genes via MEGENA network and machine learning techniques
We used the 267 DEGs from the dataset GSE43696 as a candidate gene set for MEGENE multiscale embedded co-expression network analysis. First, we selected gene pairs with significant correlation (FDR < 0.01) and ranked the meaningful gene pairs according to their correlation coefficients. The co-expression network was subsequently subjected to module clustering, and the interrelationships between the modules are shown in . We selected the module with the largest node size to generate a refined module network diagram, and retained the genes with node.shape as module.hub and child.module as class c as the candidate gene set for random forest analysis. Finally, 12 genes were selected as the final candidate gene set for random forest analysis (). In order to further identify key genes affecting asthma, we selected the candidate genes from the previous step for feature selection using random forest. Through screening, we identified the top 5 key genes as asthma feature genes. We validated further the GSE67940 dataset as an external dataset and found that the expression of C1orf64 and C7orf26 genes differed between the two groups of patients. Therefore, we selected C1orf64 and C7orf26 as the key genes for our subsequent research ().
Figure 3. Machine learning methods identify key genes: (A) Co-expression network of DEGs, where each node represents a module, with larger nodes indicating more genes. (B) Display of feature genes using the Random Forest algorithm. (C) Validation of expression patterns based on the external dataset GSE67940.

Co-expression patterns of immune genes
We employed the TISIDB database to retrieve the association between the 2 principal genes and a range of immune components, comprising immune regulatory factors, chemokines, and cell receptors (). The results of these analyses indicate a significant association between the key genes and immune cell infiltration levels, highlighting their crucial role in the immune microenvironment.
GSEA pathway and non-coding RNA network of key genes
Subsequently, we investigated the specific signaling pathways that were enriched by the two central genes and delved into the potential molecular mechanisms, which they influence the progression of asthma. We selected some highly significant pathways for focused presentation, and the GSEA results are shown in . The pathways enriched by C1orf64 include ABC transporters, cell cycle, cell adhesion molecules CAMs, and the Notch signaling pathway, while the pathways enriched by C7orf26 include cell adhesion molecules CAMs, DNA replication, cell cycle, and the Notch signaling pathway. In addition, we used the mircode database to perform reverse prediction on the two key genes and obtained 35 miRNAs and a total of 42 mRNA-miRNA interaction pairs, which were visualized using Cytoscape ().
Figure 5. GSEA enrichment analysis of key genes: (A,B) The specific display of two key gene-enriched KEGG signaling pathways, where the pathways enriched in high expression are shown above the vertical axis, and those enriched in low expression are shown below the vertical axis. (C) miRNA network of key genes based on the mircode database.
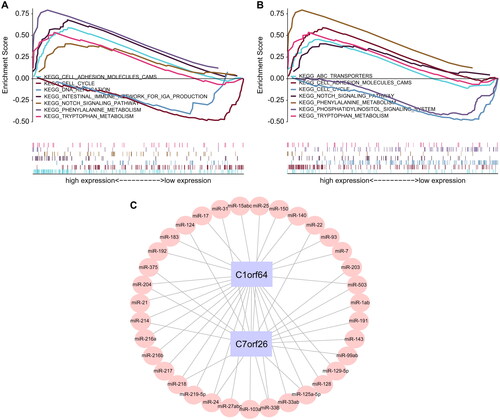
The relationship between key genes and asthma, and potential therapeutic drugs for asthma
A total of 8172 genes linked to asthma were extracted from the GeneCards database (https://www.genecards.org/). Through correlation analysis between the key genes and genes associated with asthma, we observed a significant relationship between the expression levels of the key genes and those of the asthma-related genes (). Specifically, the expression of C1orf64 exhibited a significant negative correlation with CARD11 (Pearson r = −0.423), while the expression of C7orf26 was positively correlated with HNMT (Pearson r = 0.453). The differentially expressed mRNAs were categorized into two groups: upregulated and downregulated and subsequently subjected to drug target prediction using the Connectivity Map database. The analysis revealed that the expression profiles affected by drugs such as Tyrphostin-AG-126, cephaeline, Verrucarin-a, and Emetine exhibited a significant negative correlation with the disease-disturbed expression profiles, suggesting that these drugs may have the potential to alleviate or even reverse the disease state ().
Single-cell atlas analysis of key gene expression
Finally, we downloaded single-cell data from GSE172495 and performed single-cell analysis using the seurat package. We used the tSNE algorithm to cluster cells and annotated each cluster using Human Primary Cell Atlas Data with the SingleR package. The annotation of all clusters revealed three distinct cell types, namely T cells, B cells, and NK cells (). The expression of C1orf64 and C7orf26 in these cell types is illustrated in .
Quantitative real-time PCR determines the relative expression levels of target genes
The PCR primers were produced by Sangon Biotech (Shanghai) Co., Ltd. and described as follows: β-actin forward, 5′-GTGCTATGTTGCTCTAGACTTCG-3′; β-actin reverse, 5′-ATGCCACAGGATTCCATACC-3′; C1orf64 forward, 5′-ACCAGCTCCGGTGGGAAG-3′; C1orf64 reverse, 5′-CAAGCCCTCGATTGGGACTG-3′; C7orf26 forward, 5′-CCGCCAAGGAGGTGTTGTA-3′; C7orf26 reverse, 5′-CTTGGTTTGCTCCTTGGGCT-3′. Consistent with the outcomes of earlier screening and validation, the mRNA expression levels of C1orf64 and C7orf26 were significantly raised (p < 0.05) and lowered (p < 0.01), respectively, in patients with bronchial asthma relative to the healthy controls ().
Discussion
Analyzing DEGs related to diseases using gene databases has become a popular method in modern biomedical research. In this study, we explored key genes involved in the occurrence and development of asthma through analysis of gene expression data from two different sources. The identified key hub genes, C1orf64 and C7orf26, are encoded in the human genome whose functions and regulation have not been fully elucidated. Currently, no large-scale studies have been conducted to explore the relationship between the C1orf64 and C7orf26 genes and asthma.
C1orf64 is a human gene located on chromosome 1. Information pertaining to the molecular and biochemical functions of C1orf64 is limited; hence, its specific role and contributions to disease processes remain elusive. Some reports suggest that the C1orf64 gene is crucial in amplifying intracellular estrogen receptor signaling pathways, and giving rise to regulatory proteins associated with steroid receptors [Citation20, Citation21]. However, further research is required to validate and elaborate these potential roles. Recently, genome-wide association studies (GWAS) have suggested that the C1orf64 gene may be associated with chronic obstructive pulmonary disease (COPD) [Citation22, Citation23]. Although asthma and COPD share some similarities, they are two different respiratory system diseases. Therefore, additional investigation is necessary to establish the association between the C1orf64 gene and asthma. Amid the swift evolution of genomics research, forthcoming investigations may proffer an abundance of insights regarding the functions and implications of C1orf64 in human biology and disease pathology. C7orf26 is a human gene located on chromosome 7. C7orf26 encodes a receptor protein called ‘interleukin-17 receptor E’ (IL-17RE) [Citation24, Citation25], a key receptor in the interleukin-17 (IL-17) signaling pathway. This pathway plays a significant role in promoting inflammation, a crucial component in the pathogenesis of many diseases including asthma [Citation26, Citation27]. The activation of IL-17RE is associated with the exacerbation of inflammation, suggesting that dysregulation of C7orf26 expression or function could contribute to the pathophysiological conditions in asthma. Our experiments verified the expression levels of C1orf64 and C7orf26 in the airway mucosal tissues of asthma patients and normal controls, consistent with the screening and prediction results, further demonstrating the important roles of C1orf64 and C7orf26 in the pathogenesis of asthma. However, an extensive sample collection is necessary to authenticate the accurate mechanisms and influence of C1orf64 and C7orf26 in the onset and progression of asthma.
The GSEA analysis showed highly significant enriched pathways for genes C1orf64 and C7orf26. The ABC transporters pathway enriched by C1orf64 refers to a family of ATP-binding cassette (ABC) transport proteins that are responsible for transporting compounds both inside and outside the cell. Dysregulation in this pathway, particularly in the transmembrane transport of various molecules, has been implicated in multiple lung disorders, due to altered inflammatory response or mucus production [Citation28–30]. Thus, the enrichment of this pathway suggests potential involvement of abnormal cellular transport processes in the pathogenesis of asthma. Stages of the cell life cycle are important, including cell proliferation and differentiation, which is essential for maintaining normal physiological function [Citation31]. Aberrant regulation of the cell cycle, particularly within bronchial smooth muscle cells, is reported to contribute to airway hyperresponsiveness in asthma [Citation32]. Abnormal cell proliferation and differentiation may lead to the occurrence and development of asthma. Cell adhesion molecules (CAMs) are a type of protein responsible for cell-cell and cell-matrix interactions, which play an important role in inflammation and immune cell migration [Citation33–35]. Aberrant expression of CAMs is known to affect leukocyte recruitment to the airways [Citation36], a hallmark of the inflammatory response in asthma. Therefore, the enrichment of the CAMs pathway suggests that altered intercellular communication might be an underlying factor in asthma pathogenesis. The Notch signaling pathway is an important cellular signaling pathway involved in determining proliferation and differentiation of various cells [Citation37, Citation38]. Notch signaling is implicated in airway remodeling, a key feature of severe asthma [Citation39]. Therefore, dysregulation of this pathway may significantly contribute to asthma progression. The pathway enriched by C7orf26 is also related to cell cycle, cell adhesion, DNA replication, and the Notch signaling pathway. DNA replication, a crucial process for cell proliferation. Dysregulation of this process, particularly in airway epithelial cells, has been linked to airway inflammation and remodeling in asthma [Citation32]. Hence, the enrichment of the DNA replication pathway further supports its potential role in asthma development. In conclusion, the identified enrichment of these pathways implies their substantial contribution to the development and progression of asthma. This study paves the way for future research into the specific roles and interactions of these pathways in asthma.
Drug therapy is one of the main ways to control asthma symptoms and prevent attacks. Analysis of drug target prediction using the Connectivity Map database of DEGs identified several drugs significantly associated with asthma, including Tyrphostin-AG-126, cephaeline, Verrucarin-a, and Emetine. Extensive research has been conducted on Tyrphostin-AG-126, a tyrosine kinase inhibitor, for its potential application in the treatment of several ailments, such as acute and chronic inflammation [Citation40–42]. Tyrosine kinases are enzymes responsible for the activation of many proteins by signal transduction cascades and are implicated in numerous cellular processes, such as cell growth and division [Citation43]. In asthma, tyrosine kinases play a role in the activation of immune cells and the production of inflammatory mediators, which are crucial elements of asthma pathogenesis [Citation44]. Therefore, Tyrphostin AG-126, through its inhibitory action on tyrosine kinases, may potentially attenuate the immune response and reduce inflammation, providing a rationale for its use as a potential therapeutic agent for asthma. Cephaeline is a biogenic alkaloid compound extracted from the ivy family of plants and has been shown to be highly effective in inhibiting viral infections such as ZIKV and EBOV [Citation45]. Viral infections, particularly respiratory ones, can exacerbate asthma symptoms by triggering an immune response and causing inflammation in the airways [Citation46]. Therefore, the antiviral properties of cephaeline could potentially make it beneficial for controlling asthma symptoms associated with viral infections. Verrucarin-a and Emetine are biogenic alkaloids of natural origin that have been utilized to cure multiple illnesses, such as cancer and viral infections [Citation47–50]. Meanwhile, the antiviral effects of cephaeline may have implications in the management of asthma symptoms caused by viral infections. Moreover, cancer and asthma share common pathways of chronic inflammation and tissue remodeling [Citation51, Citation52], thus suggesting potential benefits for asthma management. However, their efficacy and mechanisms of action for asthma remain unclear. Our study provides a scientific basis and theoretical foundation at the genetics level for targeted therapy of asthma drugs; however, it lacks relevant biological experimental verification. In the next step, we plan to establish cellular and animal models to further explore their mechanisms of action and efficacy.
We applied single-cell analysis to explore the cellular features of asthma. The results showed that all clusters could be classified into three types of cells: T cells, NK cells, and B cells. T cells are known to play a critical role in asthma, specifically Th2 cells, which lead to the production of cytokines such as IL-4, IL-5, and IL-13 [Citation53, Citation54]. These cytokines promote eosinophilic inflammation, mucus production, and IgE synthesis, which are all hallmark features of asthma [Citation55]. Dysregulation of genes C1orf64 and C7orf26 in T cells could potentially alter these inflammatory responses and contribute to asthma pathogenesis. NK cells, integral components of the immune system, execute a pivotal role in innate immune responses, particularly during initial reactions to viral invasions [Citation56]. Viral infections, specifically those affecting the respiratory tract, rank among the primary triggers of acute asthma exacerbations [Citation46]. As NK cells play a vital role in combatting viral infections, their functionality could potentially influence the development and severity of asthma directly or indirectly. Additionally, certain studies have discerned that NK cells can modulate the responses of Th2 cells [Citation57], the cellular elements inciting characteristics of allergic asthma. B cells, pivotal elements of the immune system, contribute significantly to the pathogenesis of asthma. They are chiefly known for their capacity to produce Immunoglobulin E (IgE), which instigates the allergic response, which is a key characteristic of asthma [Citation58]. Additionally, B cells serve as antigen-presenting cells and manufacture other immunoglobulins, such as IgG and IgA, playing a substantive role in the immune response and inflammation commonly associated with asthma [Citation58]. Consequently, aberrations in B cell functionality could contribute to the onset and progression of asthma. In this study, we found that C1orf64 and C7orf26 are expressed in these three cell types, indicating that they potentially play crucial roles in these cells and in asthma pathogenesis. Their precise roles warrant further investigation, which could open up new avenues for therapeutic interventions.
This study revealed key genes and pathways involved in the development and progression of asthma through comprehensive analysis of multiple datasets, providing new insights and directions for further research on the molecular mechanisms and treatment of asthma. However, these findings, derived from computational and bioinformatics analyses, may not encapsulate the entire intricacy of gene regulation and functionality. Therefore, more tangible experimental evidence is requisite to supplement these results, such as validation through independent cohorts using diverse methodologies, as well as ensuring their robustness and universality. We recommend several prospective avenues of research to elucidate the precise mechanisms through which C1orf64 and C7orf26 contribute to the exacerbation of asthma. For instance, we recommend conducting functional experiments to directly assess the influence of these genes on cellular processes germane to asthma. Moreover, studies involving gene knockout or overexpression within animal models would prove invaluable in exploring the impact of these genes on the initiation and progression of asthma.
Conclusions
This research elucidates the roles of key genes C1orf64 and C7orf26 in asthma pathogenesis, emphasizing their influence on critical biological pathways and immune responses. Potential therapeutic compounds are also identified, which are promising innovative avenues for asthma treatment. While these insights contribute substantially to our understanding of the molecular mechanisms of asthma, further investigation is warranted to confirm the precise roles of these se genes in the disease process.
Ethical approval
This study was conducted in accordance with the guidelines of the Ministry of Science and Technology of China and relevant national regulations, and was approved by the Ethics Committee of the Second Affiliated Hospital of Guangxi Medical University.
Authors contributions
Zhengzhao Li and Yun Ling designed the experiments. Lianshan Guo and Enhao Huang conducted data mining analysis and molecular experimental validation. Tongting Wang supported the data analysis. Lianshan Guo and Enhao Huang wrote the manuscript. All authors discussed and revised the manuscript.
Disclosure statement
No potential conflict of interest was reported by the author(s).
Data availability statement
The data that supports the findings of this study, including any relevant details needed to reproduce the published results, are available from the corresponding author upon reasonable request.
Additional information
Funding
References
- Cao Y, Chen S, Chen X, et al. Global trends in the incidence and mortality of asthma from 1990 to 2019: an age-period-cohort analysis using the global burden of disease study 2019. Front Public Health. 2022;10:1. doi: 10.3389/fpubh.2022.1036674.
- García-Marcos L, Chiang CY, Asher MI, et al. Asthma management and control in children, adolescents, and adults in 25 countries: a global asthma network phase I cross-sectional study. Lancet Glob Health. 2023;11(2):e218–14. doi: 10.1016/s2214-109x(22)00506-x.
- León B, Ballesteros-Tato A. Modulating Th2 cell immunity for the treatment of asthma. Front Immunol. 2021;12:637948. doi: 10.3389/fimmu.2021.637948.
- Chakaya J, Aït-Khaled N. Global asthma report 2022: a wake-up call to enhance care and treatment for asthma globally. Int J Tuberc Lung Dis. 2022;26(11):999–1000. doi: 10.5588/ijtld.22.0483.
- Wang R, Murray CS, Fowler SJ, et al. Asthma diagnosis: into the fourth dimension. Thorax. 2021;76(6):624–631. doi: 10.1136/thoraxjnl-2020-216421.
- Fu Y, Ling Z, Arabnia H, et al. Current trend and development in bioinformatics research. BMC Bioinformatics. 2020;21(Suppl 9):538. doi: 10.1186/s12859-020-03874-y.
- Paiva VA, Gomes IS, Monteiro CR, et al. Protein structural bioinformatics: an overview. Comput Biol Med. 2022;147:105695. doi: 10.1016/j.compbiomed.2022.105695.
- Voraphani N, Gladwin MT, Contreras AU, et al. An airway epithelial iNOS-DUOX2-thyroid peroxidase metabolome drives Th1/Th2 nitrative stress in human severe asthma. Mucosal Immunol. 2014;7(5):1175–1185. doi: 10.1038/mi.2014.6.
- Li X, Hawkins GA, Moore WC, et al. Expression of asthma susceptibility genes in bronchial epithelial cells and bronchial alveolar lavage in the severe asthma research program (SARP) cohort. J Asthma. 2016;53(8):775–782. doi: 10.3109/02770903.2016.1158268.
- Camiolo MJ, Zhou X, Wei Q, et al. Machine learning implicates the IL-18 signaling axis in severe asthma. JCI Insight. 2021;6(21), e149945. doi: 10.1172/jci.insight.149945.
- Li X, Hastie AT, Hawkins GA, et al. eQTL of bronchial epithelial cells and bronchial alveolar lavage deciphers GWAS-identified asthma genes. Allergy. 2015;70(10):1309–1318. doi: 10.1111/all.12683.
- Wu T, Hu E, Xu S, et al. clusterProfiler 4.0: a universal enrichment tool for interpreting omics data. Innovation (Camb). 2021;2(3):100141. doi: 10.1016/j.xinn.2021.100141.
- Song WM, Zhang B. Multiscale embedded gene co-expression network analysis. PLoS Comput Biol. 2015;11(11):e1004574. doi: 10.1371/journal.pcbi.1004574.
- Speiser JL. A random Forest method with feature selection for developing medical prediction models with clustered and longitudinal data. J Biomed Inform. 2021;117:103763. doi: 10.1016/j.jbi.2021.103763.
- Subramanian A, Tamayo P, Mootha VK, et al. Gene set enrichment analysis: a knowledge-based approach for interpreting genome-wide expression profiles. Proc Natl Acad Sci U S A. 2005;102(43):15545–15550. doi: 10.1073/pnas.0506580102.
- Lamb J, Crawford ED, Peck D, et al. The connectivity map: using gene-expression signatures to connect small molecules, genes, and disease. Science. 2006;313(5795):1929–1935. doi: 10.1126/science.1132939.
- Lamb J. The connectivity map: a new tool for biomedical research. Nat Rev Cancer. 2007;7(1):54–60. doi: 10.1038/nrc2044.
- Subramanian A, Narayan R, Corsello SM, et al. A next generation connectivity map: l 1000 platform and the first 1,000,000 profiles. Cell. 2017;171(6):1437–1452.e17. e1417. doi: 10.1016/j.cell.2017.10.049.
- Hao Y, Hao S, Andersen-Nissen E, et al. Integrated analysis of multimodal single-cell data. Cell. 2021;184(13):3573–3587.e29. doi: 10.1016/j.cell.2021.04.048.
- Naderi A. C1orf64 is a novel androgen receptor target gene and coregulator that interacts with 14-3-3 protein in breast cancer. Oncotarget. 2017;8(34):57907–57933. doi: 10.18632/oncotarget.17826.
- Naderi A. SRARP and HSPB7 are epigenetically regulated gene pairs that function as tumor suppressors and predict clinical outcome in malignancies. Mol Oncol. 2018;12(5):724–755. doi: 10.1002/1878-0261.12195.
- Shrine N, Guyatt AL, Erzurumluoglu AM, et al. New genetic signals for lung function highlight pathways and chronic obstructive pulmonary disease associations across multiple ancestries. Nat Genet. 2019;51(3):481–493. doi: 10.1038/s41588-018-0321-7.
- Wain LV, Shrine N, Miller S, et al. Novel insights into the genetics of smoking behaviour, lung function, and chronic obstructive pulmonary disease (UK BiLEVE): a genetic association study in UK biobank. Lancet Respir Med. 2015;3(10):769–781. doi: 10.1016/s2213-2600(15)00283-0.
- Cho NH, Cheveralls KC, Brunner AD, et al. OpenCell: endogenous tagging for the cartography of human cellular organization. Science. 2022;375(6585):eabi6983. doi: 10.1126/science.abi6983.
- Huttlin EL, Bruckner RJ, Navarrete-Perea J, et al. Dual proteome-scale networks reveal cell-specific remodeling of the human interactome. Cell. 2021;184(11):3022–3040.e28. e3028. doi: 10.1016/j.cell.2021.04.011.
- Agache I, Ciobanu C, Agache C, et al. Increased serum IL-17 is an independent risk factor for severe asthma. Respir Med. 2010;104(8):1131–1137. doi: 10.1016/j.rmed.2010.02.018.
- Lamb D, De Sousa D, Quast K, et al. RORγt inhibitors block both IL-17 and IL-22 conferring a potential advantage over anti-IL-17 alone to treat severe asthma. Respir Res. 2021;22(1):158. doi: 10.1186/s12931-021-01743-7.
- Rotoli BM, Barilli A, Visigalli R, et al. Characterization of ABC transporters in EpiAirway™, a cellular model of normal human bronchial epithelium. Int J Mol Sci. 2020;21(9):3190. doi: 10.3390/ijms21093190.
- Grigoreva TA, Sagaidak AV, Novikova DS, et al. Implication of ABC transporters in non-proliferative diseases. Eur J Pharmacol. 2022;935:175327. doi: 10.1016/j.ejphar.2022.175327.
- Sonett J, Goldklang M, Sklepkiewicz P, et al. A critical role for ABC transporters in persistent lung inflammation in the development of emphysema after smoke exposure. Faseb J. 2018;32(12):fj201701381–6736. doi: 10.1096/fj.201701381.
- Wang Z. Cell cycle progression and synchronization: an overview. Methods Mol Biol. 2022;2579:3–23. doi: 10.1007/978-1-0716-2736-5_1.
- Kaczmarek KA, Clifford RL, Knox AJ. Epigenetic changes in airway smooth muscle as a driver of airway inflammation and remodeling in asthma. Chest. 2019;155(4):816–824. doi: 10.1016/j.chest.2018.10.038.
- Eve M, Gandawijaya J, Yang L, et al. Neuronal cell adhesion molecules may mediate neuroinflammation in autism spectrum disorder. Front Psychiatry. 2022;13:842755. doi: 10.3389/fpsyt.2022.842755.
- Allen S, Moran N. Cell adhesion molecules: therapeutic targets for inhibition of inflammatory states. Semin Thromb Hemost. 2015;41(6):563–571. doi: 10.1055/s-0035-1556588.
- Fan Z, Sun H. Editorial: adhesion molecules and autoimmune diseases. Front Immunol. 2022;13:1009708. doi: 10.3389/fimmu.2022.1009708.
- Lim HF, Nair P. Airway inflammation and inflammatory biomarkers. Semin Respir Crit Care Med. 2018;39(1):56–63. doi: 10.1055/s-0037-1606217.
- Zhou B, Lin W, Long Y, et al. Notch signaling pathway: architecture, disease, and therapeutics. Signal Transduct Target Ther. 2022;7(1):95. doi: 10.1038/s41392-022-00934-y.
- Zeng Z, Wang L, Ma W, et al. Inhibiting the notch signaling pathway suppresses Th17-associated airway hyperresponsiveness in obese asthmatic mice. Lab Invest. 2019;99(12):1784–1794. doi: 10.1038/s41374-019-0294-x.
- Hussain M, Xu C, Ahmad M, et al. Notch signaling: linking embryonic lung development and asthmatic airway remodeling. Mol Pharmacol. 2017;92(6):676–693. doi: 10.1124/mol.117.110254.
- Menzfeld C, John M, van Rossum D, et al. Tyrphostin AG126 exerts neuroprotection in CNS inflammation by a dual mechanism. Glia. 2015;63(6):1083–1099. doi: 10.1002/glia.22803.
- Ahmad SF, Ansari MA, Nadeem A, et al. Protection by tyrosine kinase inhibitor, tyrphostin AG126, through the suppression of IL-17A, RORγt, and T-bet signaling, in the BTBR mouse model of autism. Brain Res Bull. 2018;142:328–337. doi: 10.1016/j.brainresbull.2018.08.020.
- Ahmad SF, Ansari MA, Nadeem A, et al. The tyrosine kinase inhibitor tyrphostin AG126 reduces activation of inflammatory cells and increases Foxp3(+) regulatory T cells during pathogenesis of rheumatoid arthritis. Mol Immunol. 2016;78:65–78. doi: 10.1016/j.molimm.2016.08.017.
- Merilahti JAM, Elenius K. Gamma-secretase-dependent signaling of receptor tyrosine kinases. Oncogene. 2019;38(2):151–163. doi: 10.1038/s41388-018-0465-z.
- Koziol-White CJ, Jia Y, Baltus GA, et al. Inhibition of spleen tyrosine kinase attenuates IgE-mediated airway contraction and mediator release in human precision cut lung slices. Br J Pharmacol. 2016;173(21):3080–3087. doi: 10.1111/bph.13550.
- Yang S, Xu M, Lee EM, et al. Emetine inhibits zika and ebola virus infections through two molecular mechanisms: inhibiting viral replication and decreasing viral entry. Cell Discov. 2018;4(1):31. doi: 10.1038/s41421-018-0034-1.
- Jackson DJ, Gern JE. Rhinovirus infections and their roles in asthma: etiology and exacerbations. J Allergy Clin Immunol Pract. 2022;10(3):673–681. doi: 10.1016/j.jaip.2022.01.006.
- Chen K, Si Y, Guan JS, et al. Targeted extracellular vesicles delivered verrucarin a to treat glioblastoma. Biomedicines. 2022;10(1), 130. doi: 10.3390/biomedicines10010130.
- Yan F, Yu Y, Chow DC, et al. Identification of verrucarin a as a potent and selective steroid receptor coactivator-3 small molecule inhibitor. PLoS One. 2014;9(4):e95243. doi: 10.1371/journal.pone.0095243.
- Kumar R, Afsar M, Khandelwal N, et al. Emetine suppresses SARS-CoV-2 replication by inhibiting interaction of viral mRNA with eIF4E. Antiviral Res. 2021;189:105056. doi: 10.1016/j.antiviral.2021.105056.
- Snoussi M, Redissi A, Mosbah A, et al. Emetine, a potent alkaloid for the treatment of SARS-CoV-2 targeting papain-like protease and non-structural proteins: pharmacokinetics, molecular docking and dynamic studies. J Biomol Struct Dyn. 2022;40(20):10122–10135. doi: 10.1080/07391102.2021.1946715.
- Banno A, Reddy AT, Lakshmi SP, et al. Bidirectional interaction of airway epithelial remodeling and inflammation in asthma. Clin Sci. 2020;134(9):1063–1079. doi: 10.1042/cs20191309.
- Nigam M, Mishra AP, Deb VK, et al. Evaluation of the association of chronic inflammation and cancer: insights and implications. Biomed Pharmacother. 2023;164:115015. doi: 10.1016/j.biopha.2023.115015.
- Bellini A, Marini MA, Bianchetti L, et al. Interleukin (IL)-4, IL-13, and IL-17A differentially affect the profibrotic and proinflammatory functions of fibrocytes from asthmatic patients. Mucosal Immunol. 2012;5(2):140–149. doi: 10.1038/mi.2011.60.
- Kandikattu HK, Upparahalli Venkateshaiah S, Mishra A. Synergy of interleukin (IL)-5 and IL-18 in eosinophil mediated pathogenesis of allergic diseases. Cytokine Growth Factor Rev. 2019;47:83–98. doi: 10.1016/j.cytogfr.2019.05.003.
- Junttila IS. Tuning the cytokine responses: an update on interleukin (IL)-4 and IL-13 receptor complexes. Front Immunol. 2018;9:888. doi: 10.3389/fimmu.2018.00888.
- Osuna-Espinoza KY, Rosas-Taraco AG. Metabolism of NK cells during viral infections. Front Immunol. 2023;14:1064101. doi: 10.3389/fimmu.2023.1064101.
- Walwyn-Brown K, Guldevall K, Saeed M, et al. Human NK cells lyse Th2-polarizing dendritic cells via NKp30 and DNAM-1. J Immunol. 2018;201(7):2028–2041. doi: 10.4049/jimmunol.1800475.
- Oliveria JP, Agayby R, Gauvreau GM. Regulatory and IgE(+) B cells in allergic asthma. Methods Mol Biol. 2021;2270:375–418. doi: 10.1007/978-1-0716-1237-8_21.