Abstract
Objective
Breast cancer is the most common cancer in women, threatening both physical and mental health. The epidemiological evidence for association between sleep duration, depression and breast cancer is inconsistent. The aim of this study was to determine the association between them and build machine-learning algorithms to predict breast cancer.
Methods
A total of 1,789 participants from the National Health and Nutrition Examination Survey (NHANES) were included in the study, and 263 breast cancer patients were identified. Sleep duration was collected using a standardized questionnaire, and the Nine-item Patient Health Questionnaire (PHQ-9) was used to assess depression. Logistic regression yielded multivariable-adjusted breast cancer odds ratios (OR) and 95% confidence intervals (CI) for sleep duration and depression. Then, six machine learning algorithms, including AdaBoost, random forest, Boost tree, artificial neural network, limit gradient enhancement and support vector machine, were used to predict the development of breast cancer and find out the best algorithm.
Results
Body mass index (BMI), race and smoking were statistically different between breast cancer and non-breast cancer groups. Participants with depression were associated with breast cancer (OR = 1.99, 95%CI: 1.55–3.51). Compared with 7–9h of sleep, the ORs for <7 and >9 h of sleep were 1.25 (95% CI: 0.85–1.37) and 1.05 (95% CI: 0.95–1.15), respectively. The AdaBoost model outperformed other machine learning algorithms and predicted well for breast cancer, with an area under curve (AUC) of 0.84 (95%CI: 0.81–0.87).
Conclusions
No significant association was observed between sleep duration and breast cancer, and participants with depression were associated with an increased risk for breast cancer. This finding provides new clues into the relationship between breast cancer and depression and sleep duration, and provides potential evidence for subsequent studies of pathological mechanisms.
Introduction
Breast cancer was the third most common cancer, with an estimated 2.26 million incident cases, with a high prevalence among women in 2020 [Citation1]. Breast cancer is the leading cause of cancer death in women and a significant cause of cancer death in men worldwide, resulting in 181,004 lives and 17.7 million disability-adjusted life years, making it one of the top burden cancers globally [Citation2]. The incidence and mortality of breast cancer are still increasing in developing and developed countries. Epidemiological studies link different factors in the development or progression of breast cancer. In developed countries, lifestyle changes, late marriage, late birth of first child, late working hours and hormone replacement therapy are major risk factors for breast cancer [Citation3]. In developing countries, the main causes of high incidence and mortality from breast cancer are lack of awareness or knowledge, inadequate screening program, delayed diagnosis and inadequate medical facilities [Citation4]. Giving breast cancer patients a precise diagnosis and prediction is crucial to accurately tell them about the course of the disease and place them in the appropriate treatment.
Numerous epidemiological studies have found that sleep deprivation and depression are risk factors for breast cancer. The National Sleep Foundation recommends 7 to 9 h of sleep per day for adults ages 26 to 64, and 7 to 8 h for adults over 65 [Citation5]. Several studies have found an association between night shift work and a higher risk of breast cancer [Citation6,Citation7]. A 2014 meta-analysis showed no association between sleep duration and breast cancer [Citation8]. A Mendelian randomization study examined the causal relations between sleep traits and risk of breast cancer in women, and found that an adverse effect of increased sleep duration (1.19, 1.02 to 1.39 per hour increase) on breast cancer risk [Citation9]. Thus, the current evidence on the relationship between sleep duration and breast cancer risk is contradictory. It is necessary to explore the relationship between sleep duration and breast cancer based on representative data. Depression is a common mental illness that severely restricts psychosocial functioning and reduces quality of life, with the number of people with depression reported to have increased by 49.86% from 1990 to 2017, and has become a major public health problem [Citation10]. It is estimated that more than 350 million people worldwide suffer from depression, resulting in 76.4 million adjusted life years per year, or 10.3% of the total burden of disease [Citation11]. Depression is expected to rank first in the global burden of disease by 2030 based on the World Health Organization (WHO) [Citation12]. However, due to a lack of effective treatments and inadequate mental health resources, depression continues to take a serious long-term toll on people’s health and quality of life. A study found that the causative effect between genetic prediction of depression and breast cancer (OR = 1.09, 95% CI: 1.03-1.15) by using a two-sample bi-directional Mendelian randomization [Citation13]. A systematic review and meta-analysis of 282,203 patients highlighted the critical role of depression/anxiety as an independent predictor of breast cancer recurrence and survival [Citation14].
The National Health and Nutrition Examination Survey (NHANES) is a representative and comprehensive survey on health and nutrition of US population. Breast cancer risk can be predicted using existing risk assessment tools that have been developed based on populations with information about known risk factors. However, these models use simple statistical architectures with additional inputs from expensive and/or invasive procedures. In contrast, we developed machine learning models to predict breast cancer risk. from highly accessible personal health data. Machine learning, which is a data-driven application in which systems automatically learn and develop without explicit programming, has been widely used in medical studies [Citation15–17]. Various machine learning methods have been developed and applied in biomedical prediction. The performance of current models is constrained by their reliance on predetermined variables. Compared with traditional statistical methods, when there are more independent variables and complex relationships, the machine learning model can get more accurate prediction results. The objective of our study was to explore the relationship between sleep duration, depression and breast cancer using the nationally representative NHANES. In addition, we used 6 machine learning algorithms (AdaBoost, Random Forest, Boost tree, Artificial neural network, Extreme Gradient Boosting (XGBoost), and Support vector machine) to predict the development of breast cancer.
Methods
Study population
Data were extracted from 5 independent cross-sectional waves of NHANES conducted by the Centers for Disease Control and Prevention (CDC) in the United States (2009-2010, 2011-2012, 2013-2014, 2015-2016 and 2017-2018). NHANES is a unique two-year cross-sectional survey of the health and nutrition status of the U.S. population that collects data on demographic, socioeconomic, and health-related issues through interviews, standardized exams, and biometric specimen collection. The health screening was conducted at a mobile Screening Center (MEC) after the participants had already participated in a household interview. The methods and processes used by NHANES for data collection are detailed elsewhere [Citation18–20]. A total of 55,018 participants were included in these 5 cycles, after the required covariates were included and missing values were removed, 1789 samples were finally included in this analysis. In the medical section, individuals were asked questions: “What kind of cancer?”. Based on this question, participants were divided into breast cancer and non-breast cancer groups. All the data could be accessed from the website of the American Centers for Disease Control and Prevention (https://www.cdc.gov/nchs/nhanes). The National Center for Health Statistics Ethics Review Board of the U.S. CDC authorized the NHANES methods, and all participants provided written informed consent.
The inclusion criteria were as follow:(a). samples should be in the same period between 2009 and 2018;(b). samples should be comparable; (c). related tests and examinations should be carried out; (d). outcome about breast cancer should be reported. The exclusion criteria included: (a). male; (b). the samples were not collected between 2009 and 201; (c). lack of relative examinations.
Assessment of sleep quality and depression
The Patient Health Questionnaire (PHQ-9) was used to determine the frequency of depressive symptoms in the past two weeks [Citation21]. PHQ-9 has been shown to be an effective tool for assessing symptoms of depression with high specificity and sensitivity, and this screening tool incorporates the diagnostic and statistical manual - IV (DSM-IV) criteria for depression [Citation22]. The PHQ-9 consists of 10 questionnaires, and for those who have answered the nine Depression Screening Tools (PDQ) in their entirety, an overall score can be calculated, ranging from 0 to 27. Major depression and depression severity can be assessed using predefined cut points. For each participant, a total score of ≥10 was diagnosed to be depressed [Citation23]. Sleep duration was created by asking participants a question “How much sleep do you usually get at night on weekdays or workdays?" According to the recommendation of the National Sleep Foundation for adults to sleep 7–9 h per day, this study defined sleep duration < 7 h as insufficient sleep. The sleep duration was categorized as short (<7 h per night), normal (7–9 h per night), or long (>9 h per night), and 7–9 h per night was used as the reference group.
Covariates
Age is calculated from the date of interview to the date of birth. Gender is coded as male or female by NHANES personnel. Race was assessed by two questions: (1) ‘Do you identify as Hispanic, Latino, or Hispanic?’ (2) ‘What race do you consider yourself?’ Based on the answers to these questions, the NHANES staff divided the respondents into five groups: Mexican American, Other Hispanic, Non-Hispanic White, Non-Hispanic Black and Other Race. Education level was grouped by the question: ‘What is the highest grade or level of school you have completed or the highest degree you have received?’ Based on responses to this question, education was divided into five groups: less than grade 9th, 9–11th grade, high school graduate, some college or associate degree and college graduate or above. Marital status was categorized as married, widowed, divorced, separated, never married, living with partner, and refused. PIR refers to the ratio of the family’s self-reported income to the family’s appropriate poverty threshold. Body mass index (BMI) was calculated as weight in kilograms divided by height in meters squared (kg/m2). Three BMI classifications were used <25, 25 to 30, and ≥30 kg/m2, reflecting underweight or normal, overweight and obesity, respectively. Both smoking and alcohol drinking was separated into two categories: yes or no. Blood pressure was measured using a mercury sphygmomanometer, three consecutively reading of systolic blood pressure (SBP) and diastolic blood pressure (DBP) were taken 5 min intervals. The mean of the three readings was calculated in the analysis. Hypertension was defined as a resting systolic blood pressure (SBP)≥140mmHg and/or a diastolic blood pressure (DBP)≥90 mmHg. Diabetes was defined a documented history in the medical record.
Statistical analysis
Means and standard deviations (SDs) for continuous variables with normally distributed distribution, medians and interquartile ranges (IQRs) for continuous variables with non-normally distributed distribution, and proportions for binary or categorical variables were displayed. The difference of continuous variables between groups was tested by Student’s t test, χ2 test for categorical variables between groups. Multivariate logistic regression model was used to calculate the odds ratios (ORs) and 95% confidence intervals (95%CIs) to estimate the association between sleep duration, depression and breast cancer. The covariables of the following four models were adjusted: Model 1= unadjusted; Model 2= sleep duration/depression; Model 3 = Model 2+ sex, age (continuous), PIR, education, race, marital status, BMI, hypertension and diabetes; Model 4= Model 3 + smoking and alcohol drinking. In order to better predict breast cancer, we first carried out random forest interpolation on all data to ensure sufficient sample size and variables. As shown in Supplementary Figure S1, we drew the density plot after interpolating the severely missing variables (hypertension and alcohol consumption), and found that the inserted value was consistent with the true value, and the interpolation effect was good. Then, we selected 6 machine learning algorithms: AdaBoost, Random Forest, Boost tree, Artificial neural network, Extreme Gradient Boosting (XGBoost), and Support vector machine.
The comparison between the 6 algorithms was based on the accuracy of the predictions and the Kappa value. All the analyses were conducted in R software 4.1.2 (The R Foundation for Statistical Computing, USA). A p-value < 0.05 was considered statistically significant.
Figure S1. Density plot of random forest interpolation for alcohol drinking and hypertension.
Results
The basic characteristics of participants according to breast cancer are summarized in . Of the 1,789 participants, 263 (14.7%) suffered from breast cancer and the mean age was 67.0 years. BMI, race and smoking were statistically different between the two groups. The ethnic distribution of breast cancer patients was 10.6% Mexican American, 7.98% other Hispanic, 53.2% Non-Hispanic White, 20.2% Non-Hispanic Black and 7.98% other race.
Table 1. Characteristics of included samples by breast cancer.
showed that the association between sleep duration, depression and breast cancer. In the fully adjusted model, participants with depression were associated with breast cancer (OR = 1.99, 95%CI: 1.55–3.51). We did not find a significant association between sleep duration and breast cancer, compared to those reporting 7-9 h per night, those who reported short sleep duration had a multivariate adjusted odd ratio (OR) with 95% confidence interval (CI) of 1.25 (0.85–1.37) and long sleep (OR = 1.05; 95% CI: 0.95–1.15).
Table 2. Logistic risk analysis for sleep duration, depression and breast cancer.
compares the fit plots after placing all covariables into 6 machine-learning algorithms. The comparison between 6 algorithms was based on the accuracy of the prediction and the Kappa value, and the AdaBoost model was found to fit best. ROC and calibration curves were conducted to evaluate the prediction effect of these 6 machine-learning algorithms (). The area under curve (AUC) values were calculated for 6 models (XGBoost AUC = 0.82; RF AUC = 0.84; Boost tree AUC = 0.83; ANN AUC = 0.83, AdaBoost AUC = 0.84, SVM AUC = 0.82 respectively). AdaBoost curve was closest to a 45-degree straight line, and predicted well. and showed evaluation parameters of 6 machine learning algorithms. In summary, AdaBoost algorithm has the best effect on breast cancer prediction based on clinical data.
Figure 1. Receiver operating characteristic (ROC) curve of caffeine intake, PA and prostate cancer by using four machine learning algorithms.
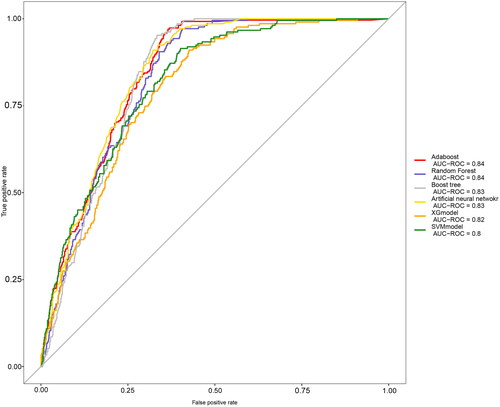
Table 3. Detailed comparison of ROC curve related indicators of six machine-learning algorithms.
Discussion
In this study, we found that participants with depression were associated with breast cancer (OR = 1.99, 95%CI: 1.55–3.51), which was similar to previous studies [Citation14]. Previous meta-analyses’ findings demonstrated that up to 32.2% of breast cancer patients had depression [Citation24]. Clinical studies have suggested that mental disorders are substantially more common in breast cancer patients than in the general population. Under constant psychological stress, breast cancer patients always experience negative emotions. Changes in physical status and function, a loss in quality of life and harmed social interactions are typically associated with breast cancer diagnosis and treatment [Citation25]. Studies have found that depression is a greater risk factor for breast cancer, a two-sample Mendelian randomization analyses demonstrated that depression can lead to higher risk of breast cancer. There are many mechanisms by which depression affects breast cancer, such as depression is accompanied by increased levels of proinflammatory cytokines and as a risk factor for cancer incidence and survival rates [Citation26]. In addition, depression promotes systemic chronic inflammation, which has been shown to be a key factor in breast cancer, by causing hepatic dysfunction and abnormalities of the hepatic epoxide metabolism [Citation27]. An increased risk of breast cancer may be linked to major depression, according to prior observational studies; however, the findings were not always reliable [Citation28]. Therefore, effective prevention and treatment measures should be developed to effectively improve breast cancer patients with depression.
We also found that no significant association between sleep duration and breast cancer, which is inconsistent with previous research. Wang P et al. [Citation6]. found that Chinese women had greater breast cancer risks for both short sleep (OR = 1.53, 95%CI: 1.10–2.12) and lengthy sleep (OR = 1.59, 95%CI: 1.17–2.17). However, no association between sleep duration and breast cancer was found in a large population-based, mostly white, prospective cohort study [Citation29,Citation30]. In a meta-analysis of 14 prospective studies and the Million Women Study, which included 65,410 breast cancer cases, short duration versus average sleep duration was not related with breast cancer risk [Citation31]. Evidence regarding the beneficial or adverse effects of sleep duration on breast cancer risk remains controversial and limited. Sleep disturbances can lead to immunosuppression and a shift in the dominance of cancer-stimulating cytokines. Some studies have shown that a shortened night’s sleep is associated with an increased risk of breast cancer.
Sleep disturbances, circadian rhythm disruptions due to nighttime illumination, melatonin reduction, and associated immune function impairment contribute to cancer onset and progression in high-risk populations [Citation32].
Although there have been numerous studies examining whether depression and sleep duration predict the onset of breast cancer, the findings to date have been inconclusive. Being able to predict the probability of later metastases occurring in each patient is critical. Because prediction can tailor treatment strategies for breast cancer patients to prevent metastasis and undertreatment or overtreatment of patients. Herein, we used 6 machine-learning algorithms to predict breast cancer, and finally found that the AdaBoost model fit best and the AUC was 0.84. The mean test AUC values of the machine learning models are quite close to each other. Xia Jiang et al. [Citation33]. demonstrated that deep learning with grid search overall performs at least as well as other machine learning methods when using non-image clinical data. Thus, in the future, we can use machine learning algorithms to fully utilize electronic health records to predict the occurrence of breast cancer
The strength of this study is that it is based on a nationally representative NHANES study that explored the relationship between sleep duration, depression, and breast cancer, and because of the wide range of covariates available, adjustments can be made for many known breast cancer risk factors. Meanwhile, we conducted various machine learning algorithms to predict breast cancer. The results of our study should be evaluated with care due to some limitation. Firstly, a cross-sectional study does not always infer causation. Therefore, it is impossible to rule out the potential of reverse-causation or bidirectionality. Secondly, sleep duration is self-reported rather than objectively measured, which may not be accurate, and other sleep factors besides sleep duration, such as sleep disturbances and sleep quality, which are also potential influencing factors for breast cancer, were not included in this study. Thirdly, although we controlled for some potential confounders, including demographics, health behaviors, and clinical status, residual confounders may still be present. Prospective cohort studies with higher sample numbers are required to further investigate the association between sleep duration, depression, and breast cancer.
Conclusions
In summary, the findings indicate that depression are associated with a higher risk of breast cancer, while no significant link between sleep duration and breast cancer was observed. AdaBoost model can accurately predict the occurrence of breast cancer. Further prospective studies are needed to clarify the causal relationship between sleep, depression, and breast cancer so that appropriate preventive measures can be taken early.
Author contributions statement
The conception and design: Haiyu Wang; analysis and interpretation of the data: Wei Zhu; the drafting of the paper: Yufan Cai and Yizhou Zhaoxiong; revising it critically for intellectual content: Yufan Cai; and the final approval of the version to be published: all authors; and that all authors agree to be accountable for all aspects of the work.
Supplemental Material
Download TIFF Image (3.1 MB)Disclosure statement
No potential conflict of interest was reported by the author(s).
Data availability statement
All the data could be accessed from the website of the American Centers for Disease Control and Prevention (https://www.cdc.gov/nchs/nhanes).
Additional information
Funding
References
- Mubarik S, Yu Y, Wang F, et al. Epidemiological and sociodemographic transitions of female breast cancer incidence, death, case fatality and DALYs in 21 world regions and globally, from 1990 to 2017: an age-Period-Cohort analysis. J Adv Res. 2022;37:1–10. Epub 2022/05/03. PubMed PMID: 35499053; PubMed Central PMCID: PMCPMC9039678. doi: 10.1016/j.jare.2021.07.012.
- Li N, Deng Y, Zhou L, et al. Global burden of breast cancer and attributable risk factors in 195 countries and territories, from 1990 to 2017: results from the global burden of disease study 2017. J Hematol Oncol. 2019;12(1):140. Epub 2019/12/23. PubMed PMID: 31864424; PubMed Central PMCID: PMCPMC6925497. doi: 10.1186/s13045-019-0828-0.
- Type and timing of menopausal hormone therapy and breast cancer risk: individual participant meta-analysis of the worldwide epidemiological evidence. Lancet. 394(10204):1159–1168. 2019Epub 2019/09/03. doi: 10.1016/s0140-6736(19)31709-x.PubMed PMID: 31474332; PubMed Central PMCID: PMCPMC6891893.
- Newman LA. Breast cancer screening in low and Middle-income countries. Best Pract Res Clin Obstet Gynaecol. 2022;83:15–23. Epub 2022/05/20. doi: 10.1016/j.bpobgyn.2022.03.018.
- Hirshkowitz M, Whiton K, Albert SM, et al. National sleep foundation’s sleep time duration recommendations: methodology and results summary. Sleep Health. 2015;1(1):40–43. Epub 2015/03/01. doi: 10.1016/j.sleh.2014.12.010.
- Wang P, Ren FM, Lin Y, et al. Night-shift work, sleep duration, daytime napping, and breast cancer risk. Sleep Med. 2015;16(4):462–468. Epub 2015/03/22. doi: 10.1016/j.sleep.2014.11.017.
- Kamdar BB, Tergas AI, Mateen FJ, Bhayani NH, Oh J. Night-shift work and risk of breast cancer: a systematic review and meta-analysis. Breast Cancer Res Treat 2013;138(1):291–301. Epub 2013/02/13. doi: 10.1007/s10549-013-2433-1.
- Qin Y, Zhou Y, Zhang X, et al. Sleep duration and breast cancer risk: a meta-analysis of observational studies. Int J Cancer. 2014;134(5):1166–1173. doi: 10.1002/ijc.28452.
- Richmond RC, Anderson EL, Dashti HS, et al. Investigating causal relations between sleep traits and risk of breast cancer in women: mendelian randomisation study. BMJ. 2019;365:l2327. Epub 2019/06/28 PubMed PMID: 31243001; PubMed Central PMCID: PMCPMC6592406 at http://www.icmje.org/coi_disclosure.pdf. MKR reports receiving research funding from Novo Nordisk, consultancy fees from Novo Nordisk and Roche Diabetes Care, and modest owning of shares in GlaxoSmithKline, outside the submitted work. DAL reports receiving research support from Medtronic and Roche Diagnostics for research outside the submitted work. All other authors declare no support from any organisation for the submitted work, no financial relationships with any organisations that might have an interest in the submitted work in the previous three years, no other relationships or activities that could appear to have influenced the submitted work. doi: 10.1136/bmj.l2327.
- Liu Q, He H, Yang J, et al. Changes in the global burden of depression from 1990 to 2017: findings from the global burden of disease study. J Psychiatr Res. 2020;126:134–140. Epub 2019/08/24. doi: 10.1016/j.jpsychires.2019.08.002.
- Hong C, Liu Z, Gao L, et al. Global trends and regional differences in the burden of anxiety disorders and major depressive disorder attributed to bullying victimisation in 204 countries and territories, 1999-2019: an analysis of the global burden of disease study. Epidemiol Psychiatr Sci. 2022;31:e85. Epub 2022/11/29. PubMed PMID: 36440549; PubMed Central PMCID: PMCPMC9714217. doi: 10.1017/s2045796022000683.
- Patel RB, Rao HR, Thakkar DV, et al. Comprehending the potential of metallic, lipid, and polymer-based nanocarriers for treatment and management of depression. Neurochem Int. 2022;153:105259. Epub 2021/12/24. doi: 10.1016/j.neuint.2021.105259.
- Zhu GL, Xu C, Yang KB, et al. Causal relationship between genetically predicted depression and cancer risk: a two-sample bi-directional mendelian randomization. BMC Cancer. 2022;22(1):353. Epub 2022/04/02 PubMed PMID: 35361153; PubMed Central PMCID: PMCPMC8973550. doi: 10.1186/s12885-022-09457-9.
- Wang X, Wang N, Zhong L, et al. Prognostic value of depression and anxiety on breast cancer recurrence and mortality: a systematic review and meta-analysis of 282,203 patients. Mol Psychiatry. 2020;25(12):3186–3197. Epub 2020/08/21. PubMed PMID: 32820237; PubMed Central PMCID: PMCPMC7714689. doi: 10.1038/s41380-020-00865-6.
- Handelman GS, Kok HK, Chandra RV, et al. eDoctor: machine learning and the future of medicine. J Intern Med. 2018;284(6):603–619. Epub 2018/08/14. doi: 10.1111/joim.12822.
- Li F, Su Y, Lin F, et al. A deep-learning system predicts glaucoma incidence and progression using retinal photographs. J Clin Invest. 2022;132(11) Epub 2022/06/02. PubMed PMID: 35642636; PubMed Central PMCID: PMCPMC9151694. doi: 10.1172/jci157968.
- Xia F, Li Q, Luo X, et al. Association between urinary metals and leukocyte telomere length involving an artificial neural network prediction: findings based on NHANES 1999-2002. Front Public Health. 2022;10:963138. Epub 2022/09/30. PubMed PMID: 36172207; PubMed Central PMCID: PMCPMC9511050. doi: 10.3389/fpubh.2022.963138.
- Xia F, Li Q, Luo X, et al. Machine learning model for depression based on heavy metals among aging people: a study with national health and nutrition examination survey 2017-2018. Front Public Health. 2022;10:939758. Epub 2022/08/23. PubMed PMID: 35991018; PubMed Central PMCID: PMCPMC9386350. doi: 10.3389/fpubh.2022.939758.
- Wang K, Xia F, Li Q, et al. The associations of weekend warrior activity patterns with the visceral adiposity index in US adults: repeated cross-sectional study. JMIR Public Health Surveill. 2023;9:e41973. Epub 2023/01/12. PubMed PMID: 36630179; PubMed Central PMCID: PMCPMC9878365. doi: 10.2196/41973.
- Xia F, Li Q, Luo X, et al. Identification for heavy metals exposure on osteoarthritis among aging people and machine learning for prediction: a study based on NHANES 2011-2020. Front Public Health. 2022;10:906774. Epub 2022/08/19. PubMed PMID: 35979456; PubMed Central PMCID: PMCPMC9376265. doi: 10.3389/fpubh.2022.906774.
- Kroenke K, Spitzer RL, Williams JB. The PHQ-9: validity of a brief depression severity measure. J Gen Intern Med. 2001;16(9):606–613. Epub 2001/09/15. PubMed PMID: 11556941; PubMed Central PMCID: PMCPMC1495268. doi: 10.1046/j.1525-1497.2001.016009606.x.
- Maske UE, Buttery AK, Beesdo-Baum K, et al. Prevalence and correlates of DSM-IV-TR major depressive disorder, self-reported diagnosed depression and current depressive symptoms among adults in Germany. J Affect Disord. 2016;190:167–177. Epub 2015/11/01. doi: 10.1016/j.jad.2015.10.006.
- Levis B, Benedetti A, Thombs BD, DEPRESsion Screening Data (DEPRESSD) Collaboration. Accuracy of patient health questionnaire-9 (PHQ-9) for screening to detect major depression: individual participant data meta-analysis. BMJ. 2019;365:l1476. Epub 2019/04/11. PubMed PMID: 30967483; PubMed Central PMCID: PMCPMC6454318 at www.icmje.org/coi_disclosure.pdf (available on request from the corresponding author) and declare: no support from any organisation for the submitted work other than that described above; no financial relationships with any organizations that might have an interest in the submitted work in the previous three years with the following exceptions: NJ and SP received a grant, outside the submitted work, from the University of Calgary Hotchkiss Brain Institute, which was jointly funded by the Institute and Pfizer; Pfizer was the original sponsor of the development of the PHQ-9, which is now in the public domain; JCNC is a steering committee member or consultant of Astra Zeneca, Bayer, Lilly, MSD, and Pfizer and has received sponsorships and honorariums for giving lectures and providing consultancy, and her affiliated institution has received research grants from these companies; UH was an advisory board member for Lundbeck and Servier, a consultant for Bayer Pharma, and a speaker for Roche Pharma and Servier and has received personal fees from Janssen, all outside the submitted work; MI has received a grant from Novartis Pharma and personal fees from Meiji, Mochida, Takeda, Novartis, Yoshitomi, Pfizer, Eisai, Otsuka, MSD, Technomics, and Sumitomo Dainippon, all outside of the submitted work; no other relationships or activities that could appear to have influenced the submitted work. doi: 10.1136/bmj.l1476.
- Pilevarzadeh M, Amirshahi M, Afsargharehbagh R, et al. Global prevalence of depression among breast cancer patients: a systematic review and meta-analysis. Breast Cancer Res Treat. 2019;176(3):519–533. Epub 2019/05/16. doi: 10.1007/s10549-019-05271-3.
- Carreira H, Williams R, Müller M, et al. Associations between breast cancer survivorship and adverse mental health outcomes: a systematic review. J Natl Cancer Inst. 2018;110(12):1311–1327. Epub 2018/11/08. PubMed PMID: 30403799; PubMed Central PMCID: PMCPMC6292797. doi: 10.1093/jnci/djy177.
- Kruk J, Aboul-Enein BH, Bernstein J, et al. Psychological stress and cellular aging in cancer: a meta-analysis. Oxid Med Cell Longev. 2019;2019:1270397–1270323. Epub 2019/12/10. PubMed PMID: 31814865; PubMed Central PMCID: PMCPMC6877941. doi: 10.1155/2019/1270397.
- Ye Z, Ganesan K, Wu M, et al. Crosstalk between depression and breast cancer via hepatic epoxide metabolism: a Central comorbidity mechanism. Molecules. 2022;27(21):7269. Epub 2022/11/12. PubMed PMID: 36364213; PubMed Central PMCID: PMCPMC9655600. doi: 10.3390/molecules27217269.
- Ren Q, Luo F, Ge S, et al. Major depression disorder may causally associate with the increased breast cancer risk: evidence from two-sample mendelian randomization analyses. Cancer Med. 2023;12(2):1984–1996. Epub 2022/07/20. PubMed PMID: 35852181; PubMed Central PMCID: PMCPMC9883582. doi: 10.1002/cam4.5043.
- Xiao Q, Signorello LB, Brinton LA, et al. Sleep duration and breast cancer risk among black and white women. Sleep Med. 2016;20:25–29. Epub 2016/06/19. PubMed PMID: 27318222; PubMed Central PMCID: PMCPMC4913026. doi: 10.1016/j.sleep.2015.11.010.
- Qian X, Brinton LA, Schairer C, et al. Sleep duration and breast cancer risk in the breast cancer detection demonstration project follow-up cohort. Br J Cancer. 2015;112(3):567–571. Epub 2014/12/05. PubMed PMID: 25474248; PubMed Central PMCID: PMCPMC4453641. doi: 10.1038/bjc.2014.600.
- Wong ATY, Heath AK, Tong TYN, et al. Sleep duration and breast cancer incidence: results from the million women study and meta-analysis of published prospective studies. Sleep. 2021;44(2)Epub 2020/09/05. PubMed PMID: 32886784; PubMed Central PMCID: PMCPMC7879408. doi: 10.1093/sleep/zsaa166.
- Blask DE. Melatonin, sleep disturbance and cancer risk. Sleep Med Rev. 2009;13(4):257–264. Epub 2008/12/20. doi: 10.1016/j.smrv.2008.07.007.
- Jiang X, Xu C. Deep learning and machine learning with grid search to predict later occurrence of breast cancer metastasis using clinical data. J Clin Med. 2022;11(19):5772. Epub 2022/10/15. PubMed PMID: 36233640; PubMed Central PMCID: PMCPMC9570670. doi: 10.3390/jcm11195772.