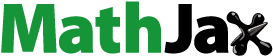
Abstract
Objectives
To analyze the factors influencing myopia and construct a nomogram to forecast the risk of myopia among school-age children, providing a reference for identifying high-risk groups to aid prevention and control.
Methods
This case-control study enrolled 3512 students from three primary schools in Shenzhen using random cluster sampling for a questionnaire survey, myopia screening and ocular biometric parameter measurement. Logistic regression was used to analyze the influencing factors of myopia, and a nomogram was constructed to forecast myopia risk. Bootstrap resampling was used to verify the practicability of the nomogram.
Results
Older age (odds ratio[OR] = 1.164; 95% confidence interval [CI]: 1.111–1.219), female sex (OR = 2.405; 95% CI: 2.003–2.887), maternal myopia (OR = 1.331; 95% CI: 1.114–1.589), incorrect posture during reading and writing (OR = 1.283; 95% CI: 1.078–1.528) and axial length (OR = 7.708; 95% CI: 6.044–8.288) are risk factors for myopia, whereas an increase in corneal radius (OR = 0.036; 95% CI: 0.025–0.052) is a protective factor against myopia. The area under the receiver operating characteristic (ROC) curve of the nomogram was 0.857, and the net benefit was high when the risk threshold of the decision curve analyses (DCA) ranged from 0.20 to 1.00. The measured values were consistent with the prediction.
Conclusion
The nomogram was accurate in predicting the risk of myopia among schoolchildren. This study provides a reference for screening high-risk students and for individualized myopia prevention and control.
KEY MESSAGES
Older age, female sex, maternal myopia, incorrect posture during reading and writing, and prolonged axial length are risk factors for myopia among primary school students.
The nomogram constructed based on age, sex, maternal myopia, incorrect posture during reading and writing, axial length and corneal radius showed good predictive accuracy and practicability.
The nomogram constructed in this study can be used for individualized myopia risk assessment.
Introduction
Myopia is harmful to students’ eyesight and places a heavy economic burden on society as it has become a public health problem [Citation1], and is one of the most common health problems among children and adolescents in China [Citation2]. Approximately half of the global population is projected to suffer from myopia by 2050 [Citation3], and it is listed by the World Health Organization as one of the five eye diseases targeted for improvement [Citation4]. Moreover, myopia greatly affects the lives of individuals who have it, and those with high myopia have a higher risk of eye diseases, including fundus lesions [Citation5], macular degeneration [Citation6], cataracts [Citation7] and irreversible visual impairment or blindness [Citation8]. Myopia usually occurs during childhood and adolescence, with the number of young people developing it increasing, especially in China [Citation9]. Therefore, school-age children are a key group to target in the prevention and control of myopia.
The mechanism by which myopia occurs and develops remains unclear; however, it is generally believed that myopia is multifactorial. On the one hand, studies have shown that screen time [Citation10] and the use of electronic devices [Citation11,Citation12] are significantly associated with myopia, and continuous near work is a risk factor for myopia [Citation13]. On the other hand, the more time spent outdoors, the lower the incidence of myopia [Citation14,Citation15]. Furthermore, sleep duration negatively correlates with the rate of refractive error [Citation16]. After controlling for environmental factors, parental myopia has been proven to be a risk factor for myopia [Citation17]. Analyzing the influencing factors of myopia can help identify high-risk groups and provide evidence for early intervention. However, few studies have evaluated the effects of various factors on myopia. In addition, the myopia risk predictive models that have been constructed to date are overly complicated for quantifying myopia risk reliably, limiting their application in clinical practice [Citation18].
Assessing disease risk can help clinicians intervene early. In this regard, nomograms have received increasing attention in the medical field in recent years as tools for disease risk assessment. Nomograms assign scores to the influencing factors of outcome events according to the degree of influence of the variables in the risk assessment model of a disease. The total score of a nomogram is obtained by adding the scores of each influencing factor. The prediction probability of an event is calculated using the function conversion relationship between the probability of an event and the total score. Finally, a graphical representation of the prediction model is presented. Nomograms can convert regression equations into visual graphs that can intuitively predict the individual risks of a disease and can be readily applied in clinical practice [Citation19]. This study aimed to develop a nomogram for preventing myopia in child and adolescents using data on eye health, living habits, genetic factors and ocular biometric parameters.
Methods
Research design and participants
This case-control study was conducted in June 2022, with 3512 students from three primary schools in Shenzhen (846, 629, 612, 443, 551 and 431 students from grades 1 to 6, respectively) recruited through random cluster sampling for a questionnaire survey, myopia screening and ocular biometric parameters measurement.
Students who were residents of Shenzhen for more than 6 months and those whose parents or guardians provided informed consent for participation in the study were included. Those with ocular diseases and inability to complete the tests and investigations in the study were excluded. The sample size was estimated based on the multifactor logistic regression formula proposed by Hsieh [Citation20]. This study was approved by the Ethics Committee of the Shenzhen Eye Hospital (No: 20201230-06), and informed consent was obtained from the participants’ legal guardians.
Questionnaire survey
An eye health questionnaire was designed for this study. The questionnaire included questions about sex, age, body mass index (BMI), paternal (PM) or maternal myopia (MM), premature infants (PI), breastfeeding, intake of sugared beverages (SB), sweet food, fried foods (FF), fruits and vegetables, sleep duration (SD), living area per person (LAP), per capita monthly income (CMI) and posture during reading and writing (PRW). The following postures during reading and writing were judged to be correct in this study: the distances between a book and the eyes, the end of a finger and nib, the chest and the desk were 33.3 cm, 3.3 cm and a fist, respectively. The questionnaire survey was conducted twice for 100 students, with an interval of one day. The results showed that the correlation coefficients between the two measurement results of each item were >0.95, indicating that the questionnaire had good stability.
Myopia screening
Visual screening was conducted in accordance with the Chinese Health Industry Standard (WS/T 663-2020) [Citation21]. All participants were examined by an optometrist utilizing an electronic visual acuity chart.
The refraction test was performed in accordance with the Chinese Health Industry Standard (WS/T 663-2020) [Citation21]. Noncycloplegic refraction was performed by an optometrist using an auto-refractor, and the spherical equivalent refraction (SER) of the participants was recorded.
Participants whose uncorrected vision acuity was <5.0 and SER was < −0.50 dioptre (D), as well as those who wore orthokeratology lens, were considered as having myopia.
Ocular biometric parameters
Optical biometry was used to measure the ocular biometric parameters. The parameters measured included central corneal thickness (CCT), corneal radius (CR), axial length (AL) and anterior chamber depth (AD).
Statistical analysis
R software (R Foundation for Statistical Computing, Vienna, Austria) was used for statistical analysis. The chi-square test was used to analyze the differences in the prevalence of myopia between groups, and the normality of the continuous data was analyzed using the Kolmogorov–Smirnov test. In the present study, age, BMI and ocular biometric parameters were normally distributed. Quantitative data are expressed as the mean ± standard deviation, and the correlation between quantitative data was analyzed using Pearson’s correlation. Multivariate logistic regression was performed to analyze factors influencing myopia, and OR and 95% CI were calculated. A nomogram for evaluating myopia risk was constructed using the influencing factors filtered out by multivariate logistic regression. Calibration and DCA curves were constructed to assess the discrimination, calibration and practicability of the nomogram. A ROC curve was constructed, and bootstrap resampling was repeated 100 times to internally verify the nomogram. Differences with p < 0.05 were considered statistically significant.
Results
A total of 3512 school-age children, of whom 1778 had myopia, were enrolled in the present study. The SER (r = 0.868, p < 0.05) between the left and right eyes was highly correlated. The SER of the right eye was analyzed in this study. Age, BMI, sex, FM, MM and CMI significantly differed between students with and without myopia (p < 0.05), whereas no differences in PI, breast feeding and LAP were observed (p > 0.05) ().
Table 1. Baseline characteristics of participants (n = 3512).
When the behavioural characteristics of students with and without myopia were compared, significant differences were found between groups in terms of fruit intake, SD and PRW (p < 0.05). However, SB, sweet food, FF and vegetable intake did not significantly differ (p > 0.05) ().
Table 2. Behaviour characteristics of participants (n = 3512).
AL (correlation coefficient [r] = 0.955, p < 0.05), CCT (r = 0.968, p < 0.05), AD (r = 0.942, p < 0.05) and CR (r = 0.975, p < 0.05) were highly correlated between the left and right eyes. Biometric parameters of the right eye were analyzed in this study. The AL, CCT and AD values in students with myopia were higher than those in students without myopia, whereas CR values were lower (p < 0.05) ().
Table 3. Biometric parameters of the eye of participants (n = 3512).
To construct the logistic regression model, the statistically significant variables in were considered independent variables, and myopia was the dependent variable. According to the collinearity diagnosis, the tolerance and the variance inflation factor of variables in the logistic regression model were >0.1 and <10, respectively. Furthermore, according to the principle of collinearity diagnosis [Citation22], no collinearity among the independent variables was observed in this study (). The results showed that age, sex, MM, PRW, AL and CR are risk factors for myopia. Older age (OR = 1.164, p < 0.05), female sex (OR = 2.405, p < 0.05), having a MM (OR = 1.331, p < 0.05), incorrect PRW (OR = 1.283, p < 0.05) and AL (OR = 7.708, p < 0.05) are risk factors of myopia, whereas an increase in CR (OR = 0.036, p < 0.05) is a protective factor against myopia ().
Table 4. Collinear diagnosis of the independent variables (n = 3512).
Table 5. Multivariate logistic regression analysis of risk factors for myopia among school-age children (n = 3512).
Six variables with p < 0.05, including age, sex, presence of MM, PRW, AL and CR, were used to construct a nomogram for predicting myopia risk. The results showed that with every 1-year increase in age, the score increased by 2.5 points; for every 1-mm increase in AL, the score increased by 12.5 points; for every 0.4-mm decrease in CR, the score increased by 12.5 points; female sex scored 5 points; MM scored 2.5 points and students with incorrect PRW scored 2.5 points ().
Figure 1. Nomogram for predicting the risk of myopia. MM, maternal myopia; PRW, posture during reading and writing; AL, axial length; CR, corneal radius. For sex, 0 = male and 1 = female; For MM, 0 = no and 1 = yes; For PRW, 0 = correct and 1 = incorrect.
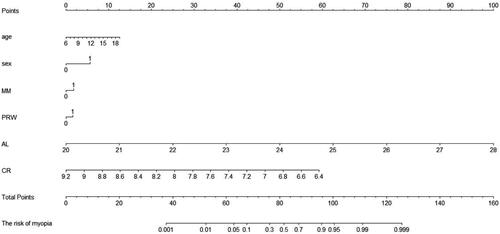
Differentiation analysis revealed that the area under the ROC curve of the nomogram was 0.857 (). The slope of the calibration curve was close to 1, suggesting that the model had high accuracy (). The DCA showed that the model had strong practicality in a wide threshold range (0.20–1.00) ().
Discussion
This study explored the factors influencing myopia in primary school students and constructed a nomogram consisting of age, sex, PRW, MM, AL and CR. The nomogram developed in the present study can be used for individualized myopia risk prediction among students and provides a reference for preventing myopia. The global prevalence of myopia among school-age children has been increasing [Citation23], reaching up to 20% in East and Southeast Asia [Citation24]. High myopia can lead to complications, such as severe visual impairment [Citation25,Citation26]. Therefore, early intervention measures must be implemented to prevent the occurrence and development of myopia.
The prevalence of myopia in the present study increased with age, consistent with previous studies [Citation27,Citation28]. Continuous near work is related to the prevalence and progression of myopia [Citation29], while sex has been confirmed to be associated with the occurrence of myopia [Citation30,Citation31]. The prevalence of myopia among female students was higher than that among male students in the present study, indicating that females are more likely to suffer from myopia than males, which is consistent with the results of studies in other countries [Citation32]. Myopia is influenced by several factors, including environmental and genetic factors [Citation33]. Genetic factors are often regarded as the cause of myopia [Citation34], and evidence for the same was found in the present study – maternal myopia influenced the occurrence and progression of myopia in children.
Measurement of ocular biometric parameters is an important method in clinical research and epidemiological investigations of myopia, and the resultant observations provide indispensable objective indices for evaluating the effects of myopia prevention. The main refractive parameters include corneal curvature, lens refractive power and AL. Studies have shown that axial growth is highly correlated with changes in refractive power [Citation35], and the main factor contributing to the occurrence and progression of myopia is axial growth. When axial growth exceeds the appropriate range for whole-eye refractive power, the overall refractive power of the eyeball develops toward myopia. The longer the axis, the more severe the degree of myopia [Citation36]. A Danish study found that the longer the AL in school-age children, the greater the probability of myopia among students [Citation37], which is similar to the results of the current study. Several studies have confirmed a positive correlation between CR and refractive power [Citation36, Citation38]; the greater the CR, the lower the risk of developing myopia. A similar trend was observed in the present study; thus, CR is considered a protective factor against myopia.
Nomograms can visualize the results of logistic regression, intuitively predict the risk of individual diseases and can be easily popularized and applied in clinical practice [Citation19]. Some researchers constructed nomograms for predicting myopia risk based on various factors [Citation39], which only included demographic characteristics and ocular biometric parameters; behavioural factors were not included in those analyses. Based on demographic characteristics, genetic and behavioural factors and ocular biometric parameters, the present study developed a nomogram for forecasting and visualizing myopia risk. The nomogram has the advantage of quantifying and individualizing myopia risk assessment. The ROC, calibration and DCA curves were utilized to confirm the good practicability of the nomogram. The nomogram in the present study was constructed based on the risk factors of myopia to quantitatively evaluate myopia risk in children and adolescents. The different values of each myopia influencing factor were converted into scores in the nomogram; the higher the total score of influencing factors, the greater the myopia risk. The nomogram constructed in the current study more intuitively showed myopia risk and is easier to implement in clinical practice compared with other risk calculators.
There are some limitations to the present study. First, the noncycloplegic refraction used in this study has high accuracy in myopia screening among children and adolescents [Citation40]. Consequently, we could not distinguish between myopia and pseudomyopia using noncycloplegic refraction and may have overestimated the prevalence of myopia [Citation41]. Second, the relevant indices of behavioural characteristics obtained in this study were self-reported by the participants; therefore, recall biases may exist. Third, this study was a case-control study and could not confirm a causal link between influencing factors and myopia [Citation42]; thus, we could not predict future myopia risk. Therefore, longitudinal cohort studies will be conducted to verify the association between the influencing factors and myopia risk.
Conclusion
The constructed nomogram, which was based on age, sex, presence of MM, PRW, AL and CR showed good predictive accuracy and practicability and can be used for individualized myopia risk assessment and provides a reference for preventing myopia among school-age children.
Authors contributions
Mu and Zhong contributed equally. Mu participated in the design, manuscript review and statistical analysis of this study, and participated in manuscript drafting. Zhong participated in the data collection and manuscript editing of this study. Jiang participated in the data collection. Wang and Zhang participated in the design of this study. All authors contributed to the article and approved the submitted version.
Acknowledgements
We are grateful to the doctors and participants who participated in this study.
Disclosure statement
No potential conflict of interest was reported by the author(s).
Data availability statement
All data supporting the results reported in this study are available from the corresponding author upon request.
Additional information
Funding
References
- Tsai TH, Liu YL, Ma IH, et al. Evolution of the prevalence of myopia among Taiwanese schoolchildren: a review of survey data from 1983 through 2017. Ophthalmology. 2021;128(2):1–7. doi: 10.1016/j.ophtha.2020.07.017.
- Qiao J, Wang Y, Li X, et al. A Lancet Commission on 70 years of women’s reproductive, maternal, newborn, child, and adolescent health in China. Lancet. 2021;397(10293):2497–2536. doi: 10.1016/S0140-6736(20)32708-2.
- Holden BA, Fricke TR, Wilson DA, et al. Global prevalence of myopia and high myopia and temporal trends from 2000 through 2050. Ophthalmology. 2016;123(5):1036–1042. doi: 10.1016/j.ophtha.2016.01.006.
- World Health Organization. Keeping the focus on Vision 2020. Perspect Public Health. 2017;137(4):210–211. doi: 10.1177/1757913917707911.
- Xu L, Wang Y, Li Y, et al. Causes of blindness and visual impairment in urban and rural areas in Beijing: the Beijing Eye Study. Ophthalmology. 2006;113(7):1134.e1–1134.e11. doi: 10.1016/j.ophtha.2006.01.035.
- Yokoi T, Ohno-Matsui K. Diagnosis and treatment of myopic maculopathy. Asia Pac J Ophthalmol (Phila). 2018;7(6):415–421. doi: 10.22608/APO.2018290.
- Lee YY, Lo CT, Sheu SJ, et al. Risk factors for and progression of myopia in young Taiwanese men. Ophthalmic Epidemiol. 2015;22(1):66–73. doi: 10.3109/09286586.2014.988874.
- Bar Dayan Y, Levin A, Morad Y, et al. The changing prevalence of myopia in young adults: a 13-year series of population-based prevalence surveys. Invest Ophthalmol Vis Sci. 2005;46(8):2760–2765. doi: 10.1167/iovs.04-0260.
- Ma J. Pay attention to implementing measures, promote prevention and control of myopia. Zhonghua Yu Fang Yi Xue Za Zhi. 2021;55(4):440–445. doi: 10.3760/cma.j.cn112150-20210223-00178.
- Lanca C, Saw SM. The association between digital screen time and myopia: a systematic review. Ophthalmic Physiol Opt. 2020;40(2):216–229. doi: 10.1111/opo.12657.
- Guan H, Yu NN, Wang H, et al. Impact of various types of near work and time spent outdoors at different times of day on visual acuity and refractive error among Chinese school-going children. PLoS One. 2019;14(4):e0215827. doi: 10.1371/journal.pone.0215827.
- Qian DJ, Zhong H, Li J, et al. Myopia among school students in rural China (Yunnan). Ophthalmic Physiol Opt. 2016;36(4):381–387. doi: 10.1111/opo.12287.
- Huang HM, Chang DS, Wu PC. The association between near work activities and myopia in children – a systematic review and meta-analysis. PLoS One. 2015;10(10):e0140419. doi: 10.1371/journal.pone.0140419.
- He M, Xiang F, Zeng Y, et al. Effect of time spent outdoors at school on the development of myopia among children in China: a randomized clinical trial. JAMA. 2015;314(11):1142–1148. doi: 10.1001/jama.2015.10803.
- Wu LJ, Wang YX, You QS, et al. Risk factors of myopic shift among primary school children in Beijing, China: a prospective study. Int J Med Sci. 2015;12(8):633–638. doi: 10.7150/ijms.12133.
- Jee D, Morgan IG, Kim EC. Inverse relationship between sleep duration and myopia. Acta Ophthalmol. 2016;94(3):e204–e210. doi: 10.1111/aos.12776.
- Mutti DO, Mitchell GL, Moeschberger ML, et al. Parental myopia, near work, school achievement, and children’s refractive error. Invest Ophthalmol Vis Sci. 2002;43(12):3633–3640.
- Zhang M, Gazzard G, Fu Z, et al. Validating the accuracy of a model to predict the onset of myopia in children. Invest Ophthalmol Vis Sci. 2011;52(8):5836–5841. doi: 10.1167/iovs.10-5592.
- Gu J, Liu J, Hong Y, et al. Nomogram for predicting risk factor of urosepsis in patients with diabetes after percutaneous nephrolithotomy. BMC Anesthesiol. 2022;22(1):87. doi: 10.1186/s12871-022-01629-1.
- Hsieh FY, Bloch DA, Larsen MD. A simple method of sample size calculation for linear and logistic regression. Statist Med. 1998;17(14):1623–1634. doi: 10.1002/(sici)1097-0258(19980730)17:14<1623::aid-sim871>3.0.co;2-s.
- National Health Commission of the People’s Republic of China. Specification for screening of refractive error in primary and secondary school students (WS/T 663-2020). Beijing: National Health Commission of the People’s Republic of China; 2020.
- Cheng J, Sun J, Yao K, et al. A variable selection method based on mutual information and variance inflation factor. Spectrochim Acta A Mol Biomol Spectrosc. 2022;268:120652. doi: 10.1016/j.saa.2021.120652.
- Naidoo KS, Fricke TR, Frick KD, et al. Potential lost productivity resulting from the global burden of myopia: systematic review, meta-analysis, and modeling. Ophthalmology. 2019;126(3):338–346. doi: 10.1016/j.ophtha.2018.10.029.
- Dong L, Kang YK, Li Y, et al. Prevalence and time trends of myopia in children and adolescents in China: a systemic review and meta-analysis. Retina. 2020;40(3):399–411. doi: 10.1097/IAE.0000000000002590.
- Lee GW, Kim JH, Kang SW, et al. Structural profile of dome-shaped macula in degenerative myopia and its association with macular disorders. BMC Ophthalmol. 2020;20(1):202. doi: 10.1186/s12886-020-01473-2.
- Hoang QV, Chan X, Zhu X, et al. Editorial: advances in management and treatment of high myopia and its complications. Front Med (Lausanne). 2022;9:846540. doi: 10.3389/fmed.2022.846540.
- Jiang D, Zhang D, Zhang Y, et al. The trend of myopia rate in 61350 children and adolescents: a cross-sectional research in Ningbo, Zhejiang. Acta Ophthalmol. 2020;98(4):e525–e526. doi: 10.1111/aos.14343.
- Wang J, Li Y, Zhao Z, et al. School-based epidemiology study of myopia in Tianjin, China. Int Ophthalmol. 2020;40(9):2213–2222. doi: 10.1007/s10792-020-01400-w.
- Harrington SC, Stack J, O’Dwyer V. Risk factors associated with myopia in schoolchildren in Ireland. Br J Ophthalmol. 2019;103(12):1803–1809. doi: 10.1136/bjophthalmol-2018-313325.
- Shapira Y, Mimouni M, Machluf Y, et al. The increasing burden of myopia in Israel among young adults over a generation: analysis of predisposing factors. Ophthalmology. 2019;126(12):1617–1626. doi: 10.1016/j.ophtha.2019.06.025.
- Zhang Y, Qiu K, Zhang Q. Ametropia prevalence of primary school students in Chinese multi-ethnic regions. Strabismus. 2020;28(1):13–16. doi: 10.1080/09273972.2019.1665691.
- Czepita M, Czepita D, Safranow K. Role of gender in the prevalence of myopia among polish schoolchildren. J Ophthalmol. 2019;2019:9748576–9748574. doi: 10.1155/2019/9748576.
- Morgan IG, Rose KA. Myopia: is the nature-nurture debate finally over? Clin Exp Optom. 2019;102(1):3–17. doi: 10.1111/cxo.12845.
- Pan CW, Ramamurthy D, Saw SM. Worldwide prevalence and risk factors for myopia. Ophthalmic Physiol Opt. 2012;32(1):3–16. doi: 10.1111/j.1475-1313.2011.00884.x.
- Gwiazda J, Hyman L, Hussein M, et al. A randomized clinical trial of progressive addition lenses versus single vision lenses on the progression of myopia in children. Invest Ophthalmol Vis Sci. 2003;44(4):1492–1500. doi: 10.1167/iovs.02-0816.
- He X, Zou H, Lu L, et al. Axial length/corneal radius ratio: association with refractive state and role on myopia detection combined with visual acuity in Chinese schoolchildren. PLoS One. 2015;10(2):e0111766. doi: 10.1371/journal.pone.0111766.
- Hansen MH, Kessel L, Li XQ, et al. Axial length change and its relationship with baseline choroidal thickness – a five-year longitudinal study in Danish adolescents: the CCC2000 eye study. BMC Ophthalmol. 2020;20(1):152. doi: 10.1186/s12886-020-01427-8.
- González Blanco F, Sanz Ferńandez JC, Muńoz Sanz MA. Axial length, corneal radius, and age of myopia onset. Optom Vis Sci. 2008;85(2):89–96. doi: 10.1097/OPX.0b013e3181622602.
- Zhou P, Wang DD, Fan L, et al. Thin central corneal thickness may be a risk factor for myopia progression in children. J Ophthalmol. 2023;2023:3815863–3815867. doi: 10.1155/2023/3815863.
- Lin S, Ma Y, He X, et al. Using decision curve analysis to evaluate common strategies for myopia screening in school aged children. Ophthalmic Epidemiol. 2019;26(4):286–294. doi: 10.1080/09286586.2019.1616774.
- Mu J, Zeng D, Fan J, et al. Epidemiological characteristics and influencing factors of myopia among primary school students in Southern China: a longitudinal study. Int J Public Health. 2023;68:1605424. doi: 10.3389/ijph.2023.1605424.
- Mu J, Zeng D, Fan J, et al. The accuracy of the axial length and axial length/corneal radius ratio for myopia assessment among Chinese children. Front Pediatr. 2022;10:859944. doi: 10.3389/fped.2022.859944.