Abstract
Anemia is a common complication of chronic kidney disease with major option treatment of erythropoiesis-stimulating agents (ESAs). This study aimed to investigate the influencing factors of erythropoietin resistance index (ERI) and its association with mortality in maintenance hemodialysis (MHD) patients. Patients enrolled from China Dialysis Outcomes and Practice Patterns Study (DOPPS) 5 were included. ERI was calculated as follows: ESA (IU/week)/weight (kg, post-dialysis)/hemoglobin level (g/dL). The Cox regression model was used to analyze the influencing factors on survival outcomes. Stepwise multivariate logistic regression was used to identify the related risk factors, and subgroup analyses were performed. A total of 1270 MHD subjects (687 males and 583 females) were included, with an average age of 60 (49.0, 71.0) years. All subjects were divided into two groups by the median ERI of 14.03. Multivariate logistic regression showed that dialysis vintage (OR 0.957, 95% CI: 0.929–0.986), white blood cells (OR 0.900, 95% CI: 0.844–0.960), high flux dialyzer use (OR 0.866, 95% CI: 0.755–0.993), body mass index (OR 0.860, 95% CI: 0.828–0.892), males (OR 0.708, 95% CI: 0.625–0.801), and albumin (OR 0.512, 95% CI: 0.389–0.673) had a negative association with high ERI baseline (all p < 0.05). There were 176 (13.9%) deaths in total including 89 cardiac/vascular deaths during follow-up. Cox regression analysis showed that ERI was positively associated with all-cause mortality, especially in some subgroups. ERI was associated with increased all-cause mortality in MHD patients, indicating the possibility of death prediction by ERI. Patients with high ERI warrant more attention.
Introduction
Chronic kidney disease (CKD) has emerged as a global concern and healthcare burden in recent years [Citation1,Citation2]. Data derived from the Global Burden of Disease Study 2017 demonstrated that there were 132.3 million people suffering from CKD in China, ranking first worldwide [Citation3]. According to the China Kidney Disease Network, the age-adjusted prevalence of patients receiving dialysis, a life-sustaining renal replacement therapy, was 402.15 per million population in China, accounting for 578,000 patients in 2016 [Citation4]. Anemia is one of the most common complications of CKD, including in patients undergoing hemodialysis (HD), and is mainly attributed to absolute or relatively reduced synthesis of erythropoietin by the kidney [Citation5,Citation6]. The Dialysis Outcomes and Practice Patterns Study (DOPPS) has already suggested that severe anemia in HD patients is related to increased mortality [Citation7]. Correction of anemia in CKD has shown improvements in quality of life and survival. For decades, erythropoiesis-stimulating agents (ESAs) have been a mainstay of treatment for CKD-related anemia among HD patients [Citation8,Citation9]. Kidney Disease: Improving Global Outcomes (KDIGO) Clinical Practice Guideline suggested that ESA be used to avoid having Hb fall below 9 g/dL among patients receiving HD and maintain in a reasonable range [Citation10]. However, some patients with ESA hyporesponsiveness, which is called erythropoietin resistance, require the use of ESAs at doses higher than usual to maintain the recommended hemoglobin concentration. Evidence of increased cardiovascular risks, mortality and other adverse outcomes regarding ESA hyporesponsiveness has been observed in HD patients [Citation11–13].
The hematopoietic response to ESAs is evaluated by the erythropoietin resistance index (ERI) [Citation13,Citation14]. Previous studies have shown that the ERI can predict the risk of all-cause mortality and cardiovascular events in patients. A recent retrospective observational study including 824 Chinese HD patients from 16 dialysis centers found that a higher ERI (12.9–17.9 and ERI > 17.9) can independently predict patient death. There is currently a lack of relevant research evidence for prospective data in China [Citation15].
This study aimed to investigate the influencing factors of ERI and its relationship to mortality in maintenance hemodialysis (MHD) patients in the prospective DOPPS study.
Materials and methods
Study population and design
As previously described, DOPPS is a prospective cohort study of HD patients in numerous countries [7,16–19]. China, the most populous country worldwide, joined DOPPS 4 (2009–2012) for the first time, which was designed as an observational, cross-sectional study [Citation16]. Thereafter, the top three cities (Beijing, Shanghai, and Guangzhou), including 15 study sites in each city, were included in DOPPS 5, a cohort study from 2012 to 2015. All study sites were randomly selected from a stratified list of all HD facilities treating more than 25 HD patients and included an average of 30 patients at each site [Citation17–19]. DOPPS 5 are multicenter prospective observational cohort studies of patients treated with maintenance HD. Simple data for all census patients and detailed data for sample patients were collected. Baseline characteristics included demographics, laboratory variables, and comorbidities.
A total of 1427 patients were enrolled at baseline with inclusion criteria of age ≥18 years and treatment with regular HD for >3 months. After excluding the absence of hemoglobin and ESA dosage at baseline (n = 157), all 1270 patients completed follow-up. This study was approved by the Ethics Committee of Peking University People’s Hospital (2018PHB028-01). All subjects provided written informed consent before participation.
Data collection and outcome
Since the collection of data in DOPPS5 was described in detail in our published essays [17–19], baseline data in DOPPS5, in short, were comprised of demographics data, biochemical measurements, history of past illness, current treatment, and comorbidities. The ERI was used to evaluate the hematopoietic response to ESAs. The ERI was obtained by the following formula: ERI = ESA (IU/week)/weight (post-dialysis, kg)/hemoglobin level (g/dL) [Citation14]. The dose of ESA referred to the average weekly dose of ESA over the most recent month of the data collection, and all variables were baseline data.
The primary outcome was all-cause mortality. The secondary outcome was CV mortality during the follow-up period. A ‘Termination form’ was set in advance to collect the death information of participants, including the date, place, primary cause of death, and secondary cause of death. All reasons for death were classified into several categories (cardiac, vascular, liver disease, infection, gastrointestinal, metabolic, other, and unknown), and cardiac/vascular death was defined as CV mortality.
Statistical analyses
Statistical analyses were performed using SAS, version 9.4 (SAS Institute, Cary, NC, USA), and a two-tailed p < 0.05, was considered statistically significant. All variables were tested for normality. Skewed distributed variables are represented by median and interquartile range (Q1, Q3), and normally distributed variables are represented as mean ± standard deviation. Chi-square test or Fisher’s test was used for comparison between groups. Multiple imputation was used to perform 25 imputations for variables with missing data > 5% [the imputed variables in this analysis were standardized Kt/V (StdKt/V), Albumin (Alb), body mass index (BMI), and intradialytic weight loss]. We performed multivariable logistic regression analyses to examine the influencing factors of ERI, as a categorical variable. Kaplan–Meier (KM) analysis with log-rank test was used to assess the all-cause mortality between different ERI groups by the follow-up time. The Cox proportional hazards model was conducted to explore the potential relationship between ERI, as a continuous variable, and all-cause mortality or CV mortality. All Cox models employed accounted for facility clustering effects by using the robust sandwich covariance estimate. Survival time for Cox models of all-cause mortality was the time from study entry to the end of study or to death, whichever occurred first. Similar calculation was taken for CV mortality. Cox regression models were with four incremental levels of covariate adjustment. Model 1: unadjusted; Model 2: adjusted for age, gender, BMI, dialysis vintage, primary kidney disease; Model 3: Model 2 variables plus modified Charleson Comorbidity Index, fistula use; Model 4: Model 3 variables plus albumin (Alb), standardized kt/v.
Results
Clinical characteristics of study subjects
A total of 1270 MHD subjects (including 687 males and 583 females) were included, with an average age of 60 (49–71) years (). The median dialysis vintage was 2.5 (0.9, 5.4) years. The use of ESA was mainly intravenous, accounting for 60.3%. The utilization rate of the iron agent was 53.2%. The brands of ESAs are mainly domestic, accounting for 96.4%, with the top five products being Yibiao, Yibao, Jimaixin, Saiboer, and Huanerbo (all containing recombinant human erythropoietin). All subjects were divided into two groups by the median ERI of 14.03. The clinical characteristics of the subjects are shown in . The top 2 causes of kidney disease were glomerulonephritis and diabetic nephropathy, accounting for 39.8% and 23.5%, respectively. The median ERI values in the low ERI group and high ERI group were 9.2 (6.4–11.6) and 19.4 (16.4–25.7), respectively. Subjects with a low ERI tended to have a longer dialysis vintage and higher white blood cell, hemoglobin and creatine levels than those with a high ERI at baseline (all p < 0.05). ESA (per month) and ESA (single dose) were higher in the high ERI group (all p < 0.05).
Table 1. Baseline characteristics of HD patients.
After a median follow-up of 1.9 (1.2, 2.1) years, there were 176 (13.9%) deaths in total, including 89 cardiac/vascular deaths. The all-cause mortality was 12.8% (n = 81) and 15% (n = 91), and the CV mortality was 6.6% (n = 42) and 7.4% (n = 47) in the low ERI and high ERI groups, respectively (all p > 0.05). The detailed classification of reasons for death is shown in .
Table 2. The distribution of primary causes of death.
Influencing factors of ERI at baseline
Logistic regression was used to explore influencing factors on ERI at baseline. Using the ERI as a categorical variable, univariate logistic regression analysis showed that male sex, dialysis vintage, BMI, albumin, white blood cell count, creatine, fistula use and high flux dialyzer use were statistically significant negatively correlated with a higher ERI (all p < 0.05). Multivariate logistic regression including all variables with p < 0.10 in univariate logistic regression analysis demonstrated that dialysis vintage (per year, OR 0.957, 95% CI: 0.929–0.986), white blood cells (per 1 × 109/L, OR 0.900, 95% CI: 0.844–0.960), high flux dialyzer use (yes/no, OR 0.866, 95% CI: 0.755–0.993), BMI (per kg/m2, OR 0.860, 95% CI: 0.828–0.892), male sex (yes/no, OR 0.708, 95% CI: 0.625–0.801), and albumin (per g/dL, OR 0.512, 95% CI: 0.389–0.673) remained negatively related to higher ERI (all p < 0.05), while the influence of creatine and fistula use on higher ERI disappeared. See for details.
Table 3. Logistics regression of ERI.
Association between ERI and mortality during follow-up
Kaplan–Meier analysis was used to determine the predictive ability of different ERI groups for all-cause mortality and CV mortality. The survival curves showed that all-cause mortality and survival probability have no difference between the low ERI group and the high ERI group (both p > 0.05) (). Cox regression analysis showed that the ERI, as a continuous variable, was positively associated with all-cause mortality (p < 0.05). While no correlation was found between the ERI and CV mortality in the crude model. After adjusting for age, sex, BMI, dialysis vintage, primary kidney diseases, modified Charleson Comorbidity Index and fistula use, the relationship between ERI and all-cause mortality remained positive (HR 1.021, 95% CI: 1.003–1.040, p < 0.05, model 3) ().
Figure 1. The proportional hazard regression (PHREG) curve of survival probability between high ERI and low ERI group. The shadow area was the 95% confidence interval. (a) all-cause mortality; (b) cardiovascular mortality.
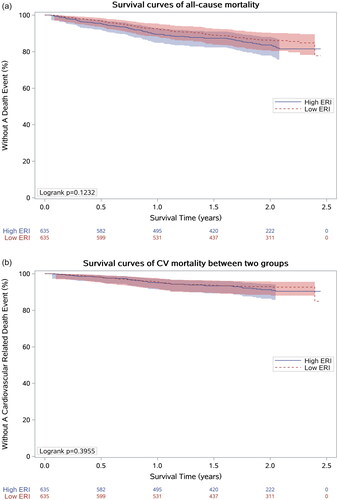
Figure 2. Cox regression analysis for ERI and all-cause mortality (a) or CV mortality (b). Model 1 was a crude model. Model 2: model 1 + age, gender, BMI, dialysis vintage, primary kidney disease. Model 3: model 2 + modified Charleson Comorbidity Index, fistula use. Model 4: model 3 + albumin (Alb), standardized kt/v.
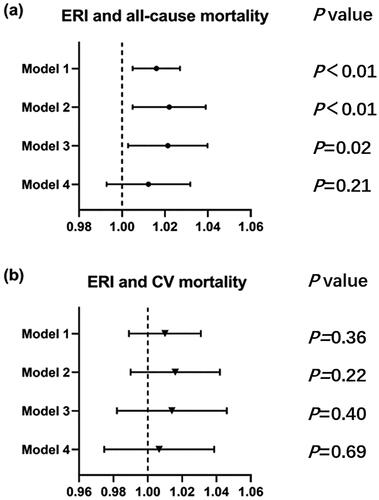
The association between the ERI and all-cause mortality among the various groups is shown in . Statistically significant direct associations between ERI and all-cause mortality were found among the different ages, Stdkt/V groups, prescribed dialysis frequency, high-flux dialyzer use and urine output, with all P values for interaction >0.05. The associations between ERI and all-cause mortality reached statistical significance in the following groups: age ≥60, those without diabetes or congestive heart failure, with fistula use, albumin <4 g/dL, nonhigh-flux users, and those with inadequate dialysis treatments.
Figure 3. Subgroup analyses by gender (male vs. female), age (<60 vs. ≥60 years), diabetes, stdkt/v (<2 vs. ≥2), type of vascular access (fistula vs. catheter), time of prescribed dialysis per week (<3 vs. ≥3), high flux dialyzer, albumin (<4 vs. ≥4 g/dL), urine output (<200 vs. ≥200 mL/day), and congestive heart failure using cox regression. The model was adjusted for age, gender, BMI, vintage, and primary kidney disease.
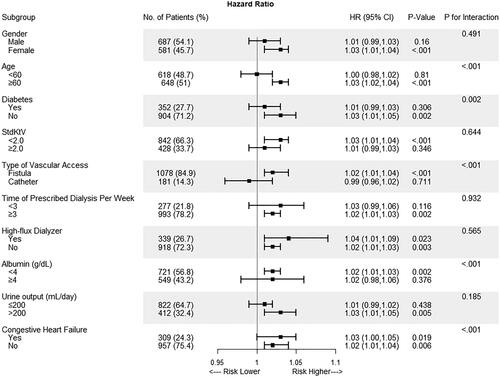
Discussion
This prospective study indicated that ESA resistance calculated by the ERI was associated with increased all-cause mortality after adjusting for most confounding factors in MHD patients. The association seems to be linear. The trend was significant though the effect was attenuated by adding laboratory indexes (Model 4). It was possible due to the relatively short follow-up period and small number of death cases. Male sex, longer dialysis vintage, larger BMI, higher albumin and white blood cell counts, and high-flux dialyzer use seem to be protective factors against higher ERI.
Concerning the global CKD pandemic, anemia in MHD patients has drawn much attention among physicians [8,20–22]. The Kidney Disease: Improving Global Outcomes (KDIGO) anemia guideline recommends the diagnosis and treatment of anemia in CKD, including the use of iron agents, ESAs, and red blood cell transfusions [10,23,24]. The appropriate use and dosage of ESA were of importance due to the controversial debate between ESAs and mortality. Although it was verified that ESAs could treat anemia and improve quality of life in MHD patients, ESA resistance has been considered as one of the most important reasons for high ESA dosage and the potential mechanism underlying the relationship between ESA and mortality [15,25,26]. ERI, an indicator calculated by ESA dosage and hemoglobin, could simply reflect ESA resistance and has already been applied in clinical research [Citation14].
A previous study verified that nutrition status, including albumin and BMI, was negatively related to a higher ERI [27–29]. A Spanish study including 1710 patients from 119 HD sites illustrated inverse correlations between both serum albumin levels and BMI and ERI [29]. Albumin is an acute-phase reactant that decreases inflammation. Therefore, the opposite correlation between low serum albumin and higher ESA resistance is more likely to be attributed to the presence of inflammation rather than malnutrition. Another single-center study in Japan also demonstrated that serum albumin levels and BMI were negatively associated with ERI, while a direct relationship was found between dialysis vintage and ERI, inconsistent with our study [30]. Goodkin et al. found that resistance to ESA among patients on HD might be typically transient, providing a possible explanation for the inconsistency [31]. Studies also suggested that long-acting ESA might reduce ERI [32]. A previous multicenter study in Spine showed that in HD patients, the ERI decreased from 15.1 IU/kg/week/g/dL with epoetin alfa to 7.9 IU/kg/week/g/dL with darbepoetin alfa. The conversion from epoetin alfa to darbepoetin alfa was associated with an approximate and persistent reduction of 65% of the required dose [32]. However, relevant analysis was not able to be performed in this study since there are few patients using darbepoetin alfa. Further studies are necessary to elucidate this debate.
Cohort data from our study also indicated that the ERI was associated with higher mortality in MHD patients, consistent with other studies. A prospective cohort in Japan including a relatively small sample of 248 MHD patients showed that a high ERI score was an independent risk factor for all-cause mortality in HD patients, while the abovementioned Chinese retrospective study also detected that a higher ESA dosage and resistance to EAS were related to all-cause mortality [30]. Data from the Cardiovascular risk in dialysis (RISCAVID study) also suggested higher all-cause mortality and CV mortality in relation to higher ERI after adjusting for other confounding factors with a median follow-up of 36 months [13]. Shizuka et al. reported that the high ERI group exhibited significantly higher rates of cardiovascular disease. Interestingly, they discovered that EPO hyporesponsiveness, when combined with low handgrip strength, was a significantly stronger predictor of a poor outcome [33]. However, in the current study, no association between ERI and CV mortality was observed, possibly due to an inadequate duration of follow-up leading to lower CV mortality.
The mechanism between the ERI and mortality remains unclear, and further studies are needed. Oxidative stress has been considered a significant risk factor for the development of anemia in MHD patients, representing a risk factor for the development of ESA resistance in MHD patients [12]. ESA plays a more important role in influencing ERI than hemoglobin because the doses are reportedly 9- to 13-fold more variable than hemoglobin [34]. A high ERI value was regarded as a marker of severe inflammation and latent comorbidities in MHD patients. Considering the easy calculation of the ERI, it is possible and important to add the ERI to clinical laboratory reports to help physicians develop precise medicine and improve clinical outcomes.
The most valuable part of the present study is the prospective design and high follow-up rate of relatively large samples in MHD patients to explore the relationship between ERI and all-cause mortality. However, this study still had several limitations. First, several laboratory indices were not routinely tested in many facilities. Although multiple imputation was used for missing data, there was still inevitable bias. Second, the median follow-up was relatively short, leading to lower all-cause mortality and CV mortality.
In conclusion, this prospective cohort of MHD patients revealed that the ERI value was associated with increased all-cause mortality in MHD patients, and more attention should be given to detecting ESA resistance and determining the appropriate dosage of ESA to achieve the target hemoglobin level. The underling influencing factors of high ERI should be determined and controlled and thus to achieve a better management of anemia. Future studies with longer follow-up should be conducted to obtain more robust evidence.
Ethical approval
The study was approved by the Ethics Committee of Peking University People’s Hospital (ethical approval number: 2018PHB028-01). Other participating subcenters also obtain ethics committee approval documents prior to the start of clinical trials.
Consent form
All patients signed the written informed consent.
Author contributions
Conception and design of research: Xinju Zhao and Li Zuo; analyzed data: Xinju Zhao; interpreted results of experiments: Xinju Zhao and Li Zuo; prepared figures: Xinju Zhao; drafted manuscript: Xinju Zhao; edited and revised manuscript: Liangying Gan, Fan Fan Hou, Xinling Liang, Zhaohui Ni, Xiaonong Chen, Yuqing Chen, and Li Zuo; approved final version of manuscript: Li Zuo.
Acknowledgements
The Dialysis Outcomes and Practice Patterns Study (DOPPS) Program in China is supported by Vifor Fresenius Renal Pharma, Sanofi Renal, Nipro Trading (Shanghai) Co., Ltd., 3SBio Inc., B. Braun, CEMMA MEDICAL, and Kyowa Kirin China Pharmaceutical Co., Ltd.
Disclosure statement
No potential conflict of interest was reported by the author(s).
Data availability statement
The data used in this study are available from the corresponding author on reasonable request.
Additional information
Funding
References
- Thurlow JS, Joshi M, Yan G, et al. Global epidemiology of end-stage kidney disease and disparities in kidney replacement therapy. Am J Nephrol. 2021;52(2):1–8. doi: 10.1159/000514550.
- Chen TK, Knicely DH, Grams ME. Chronic kidney disease diagnosis and management: a review. JAMA. 2019;322(13):1294–1304. doi: 10.1001/jama.2019.14745.
- GBD Chronic Kidney Disease Collaboration. Global, regional, and national burden of chronic kidney disease, 1990-2017: a systematic analysis for the global burden of disease study 2017. Lancet. 2020;395(10225):709–733. doi: 10.1016/S0140-6736(20)30045-3.
- Yang C, Gao B, Zhao X, et al. Executive summary for China kidney disease network (CK-NET) 2016 annual data report. Kidney Int. 2020;98(6):1419–1423. doi: 10.1016/j.kint.2020.09.003.
- Babitt JL, Lin HY. Mechanisms of anemia in CKD. J Am Soc Nephrol. 2012;23(10):1631–1634. doi: 10.1681/ASN.2011111078.
- Drawz P, Rahman M. Chronic kidney disease. Ann Intern Med. 2015;162(11):ITC1–IT16. doi: 10.7326/AITC201506020.
- Locatelli F, Pisoni RL, Combe C, et al. Anaemia in hemodialysis patients of five european countries: association with morbidity and mortality in the dialysis outcomes and practice patterns study (DOPPS) [published correction appears in nephrol dial transplant. 2004 jun;19(6):1666]. Nephrol Dial Transplant. 2004;19(1):121–132. doi: 10.1093/ndt/gfg458.
- Fishbane S, Coyne DW. How I treat renal anemia. Blood. 2020;136(7):783–789. doi: 10.1182/blood.2019004330.
- Shepshelovich D, Rozen-Zvi B, Avni T, et al. Intravenous versus oral iron supplementation for the treatment of anemia in CKD: an updated systematic review and meta-analysis. Am J Kidney Dis. 2016;68(5):677–690. doi: 10.1053/j.ajkd.2016.04.018.
- Stevens PE, Levin A, Kidney Disease: improving Global Outcomes Chronic Kidney Disease Guideline Development Work Group Members. Evaluation and management of chronic kidney disease: synopsis of the kidney disease: improving global outcomes 2012 clinical practice guideline. Ann Intern Med. 2013;158(11):825–830. doi: 10.7326/0003-4819-158-11-201306040-00007.
- Fort J, Cuevas X, García F, et al. Mortality in incident hemodialysis patients: time-dependent haemoglobin levels and erythropoiesis-stimulating agent dose are independent predictive factors in the ANSWER study. Nephrol Dial Transplant. 2010;25(8):2702–2710. doi: 10.1093/ndt/gfq073.
- Joksimovic Jovic J, Antic S, Nikolic T, et al. Erythropoietin resistance development in hemodialysis patients: the role of oxidative stress. Oxid Med Cell Longev. 2022;2022:9598210–9598211. doi: 10.1155/2022/9598211.
- Panichi V, Rosati A, Bigazzi R, et al. Anaemia and resistance to erythropoiesis-stimulating agents as prognostic factors in hemodialysis patients: results from the RISCAVID study. Nephrol Dial Transplant. 2011;26(8):2641–2648. doi: 10.1093/ndt/gfq802.
- Pınarbaşı AS, Dursun I, Günay N, et al. Erythropoietin resistance index and the affecting factors in children with peritoneal dialysis. Blood Purif. 2021;50(6):942–951. doi: 10.1159/000514060.
- Pan S, Zhao DL, Li P, et al. Relationships among the dosage of erythropoiesis-stimulating agents, erythropoietin resistance index, and mortality in maintenance hemodialysis patients. Blood Purif. 2022;51(2):171–181. doi: 10.1159/000506536.
- Sun YP, Yang WJ, Li SH, et al. Clinical epidemiology of mineral bone disorder markers in prevalent hemodialysis patients in the xinjiang uyghur autonomous region in China. Biomed Res Int. 2017;2017:2516934–2516935. doi: 10.1155/2017/2516934.
- Zhao X, Niu Q, Gan L, et al. Blood flow rate: an independent risk factor of mortality in chinese hemodialysis patients. Semin Dial. 2022;35(3):251–257. doi: 10.1111/sdi.13023.
- Niu Q, Zhao X, Gan L, et al. Physical function and clinical outcomes in hemodialysis patients: China dialysis outcomes and practice patterns study. Kidney Dis. 2021;7(4):315–322. doi: 10.1159/000513897.
- Zhao X, Niu Q, Gan L, et al. Thrombocytopenia predicts mortality in chinese hemodialysis patients- an analysis of the China DOPPS. BMC Nephrol. 2022;23(1):11. Published 2022 Jan 3. doi: 10.1186/s12882-021-02579-5.
- Kalantar-Zadeh K, Jafar TH, Nitsch D, et al. Chronic kidney disease. Lancet. 2021;398(10302):786–802. doi: 10.1016/S0140-6736(21)00519-5.
- Ma L, Zhao S. Risk factors for mortality in patients undergoing hemodialysis: a systematic review and meta-analysis. Int J Cardiol. 2017;238:151–158. doi: 10.1016/j.ijcard.2017.02.095.
- Hallan SI, Matsushita K, Sang Y, et al. Age and association of kidney measures with mortality and end-stage renal disease. JAMA. 2012;308(22):2349–2360. doi: 10.1001/jama.2012.16817.
- Kidney Disease: Improving Global Outcomes (KDIGO) Anemia Work Group. KDIGO Clinical Practice Guideline for Anemia in Chronic Kidney Disease. Kidney Int Suppl, 2 (2012), pp. 279–335
- Guideline Working Group for renal anemia, Chinese Medical Doctor Association Branch of Nephrophysicians. Clinical guidelines for diagnosis and treatment of renal anemia in China. Chin J Med. 2021;101(20):40.
- Fukuma S, Yamaguchi T, Hashimoto S, et al. Erythropoiesis-stimulating agent responsiveness and mortality in hemodialysis patients: results from a cohort study from the dialysis registry in Japan. Am J Kidney Dis. 2012;59(1):108–116. doi: 10.1053/j.ajkd.2011.07.014.
- Sakaguchi Y, Hamano T, Wada A, et al. Types of erythropoietin-Stimulating agents and mortality among patients undergoing hemodialysis. J Am Soc Nephrol. 2019;30(6):1037–1048. doi: 10.1681/ASN.2018101007.
- Feret W, Safranow K, Kwiatkowska EWA, et al. Malnutrition and erythropoietin resistance among patients with end-stage kidney disease: where is the perpetrator of disaster? Nutrients. 2022;14(24):5318.doi: 10.3390/nu14245318.
- Chiang WF, Hsiao PJ, Wu KL, et al. Investigation of the relationship between lean muscle mass and erythropoietin resistance in maintenance hemodialysis patients: a cross-sectional study. Int J Environ Res Public Health. 2022;19(9):5704. doi: 10.3390/ijerph19095704.
- López-Gómez JM, Portolés JM, Aljama P. Factors that condition the response to erythropoietin in patients on hemodialysis and their relation to mortality. Kidney Int Suppl. 2008;74(111):S75–S81. doi: 10.1038/ki.2008.523.
- Okazaki M, Komatsu M, Kawaguchi H, et al. Erythropoietin resistance index and the all-cause mortality of chronic hemodialysis patients. Blood Purif. 2014;37(2):106–112. doi: 10.1159/000358215.
- Goodkin DA, Zhao J, Cases A, et al. Resistance to erythropoiesis-stimulating agents among patients on hemodialysis is typically transient. Am J Nephrol. 2022;53(5):333–342. doi: 10.1159/000523947.
- Arrieta J, Moina I, Molina J, et al. Switch from epoetin to darbepoetin alfa in hemodialysis: dose equivalence and hemoglobin stability. Int J Nephrol Renovasc Dis. 2014;7:353–359. doi: 10.2147/IJNRD.S6189525336984.
- Kobayashi S, Tanaka K, Hoshino J, et al. Synergistic deterioration of prognosis associated with decreased grip strength and hyporesponse to erythropoiesis-stimulating agents in patients undergoing hemodialysis. Ren Fail. 2022;44(1):1801–1810. doi: 10.1080/0886022X.2022.2106873.
- Chait Y, Kalim S, Horowitz J, et al. The greatly misunderstood erythropoietin resistance index and the case for a new responsiveness measure. Hemodial Int. 2016;20(3):392–398. doi: 10.1111/hdi.12407.