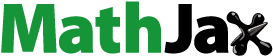
Abstract
Increasing evidence has shown that physical activity is related to a lower risk of chronic kidney disease (CKD), thus indicating a potential target for prevention. However, the causality is not clear; specifically, physical activity may protect against CKD, and CKD may lead to a reduction in physical activity. Our study examined the potential bidirectional relationship between physical activity and CKD by using a genetically informed method. Genome-wide association studies from the UK Biobank baseline data were used for physical activity phenotypes and included 460,376 participants. For kidney function (estimated Glomerular Filtration Rate (eGFR) and CKD, with eGFR < 60 mL/min/1.73 m2), CKDGen Consortium data were used, which included 480,698 CKD participants of European ancestry. Mendelian randomization (MR) analysis was used to determine the causal relationship between physical activities and kidney function. Two-sample MR genetically predicted that heavy DIY (do it yourself) (e.g., weeding, lawn mowing, carpentry, and digging) decreased the risk of CKD (odds ratio [OR] = 0.287, 95% CI = 0.117–0.705, p = 0.0065) and improved the level of eGFR (β = 0.036, 95% CI = 0.005–0.067, p = 0.021). The bidirectional MR showed no reverse causality. It is worth noting that other physical activities, such as walking for pleasure, strenuous sports, light DIY (e.g., pruning and watering the lawn), and other exercises (e.g., swimming, cycling, keeping fit, and bowling), were not significantly correlated with CKD and eGFR. This study used genetic data to provide reliable and robust causal evidence that heavy physical activity (e.g., weeding, lawn mowing, carpentry, and digging) can protect kidney function and further lower the risk of CKD.
1. Introduction
Chronic kidney disease (CKD) is a progressive disease with a very complex etiology and high morbidity and mortality that exists in various populations throughout the world [Citation1]. In 2017, 1.2 million people died of chronic kidney disease worldwide, and the mortality rate of chronic kidney disease increased by 41.5% from 1997 to 2017 [Citation2]. Due to the fact that there is no cure, the prevention and treatment of CKD is particularly important, especially for aged patients with hypertension and diabetes; according to current international guidelines, CKD is defined as decreased kidney function (regardless of the underlying cause) as demonstrated by a glomerular filtration rate (GFR) of less than 60 mL min−1 per 1.73 m2 or by kidney damage markers (or both) for at least three months [Citation3–5].
Physical activity (PA) and exercise are considered to have positive implications for the prevention and treatment of various chronic diseases [Citation6,Citation7]. People who reported of engaging in more physical activity were more likely to be in good health [Citation8]. However, there are still some controversies about the relationship between CKD and PA in some clinical studies. By using an accelerometer to objectively measure PA, the National Health and Nutrition Survey of the United States found that total and light PA were positively correlated with kidney function (log eGFR) [Citation9]. Other studies have shown that physical activity is beneficial for improving exercise tolerance and utilization of oxygen [Citation10–12]. However, a trial containing 18 participants demonstrated that a 1-year exercise intervention on the progression of kidney disease was inconclusive [Citation13]. Furthermore, a meta-analysis including 28 trials demonstrated that, compared to standard care, exercise increased peak oxygen usage; however, PA had no significant impact on eGFR or all-cause mortality [Citation14].
Physical activity (PA) engagement varies from person to person and is influenced by environmental factors, culture, and the economy [Citation15, Citation16]. Numerous studies have shown evidence of the genetic factors underlying the predisposition of humans to exercise [Citation17–21].
In routine clinical research, large-scale randomized controlled trials (RCTs) can be used to explore the relationship between physical activity and chronic kidney disease; however, confounding factors such as hypertension and diabetes cannot be excluded, and a robust causal relationship cannot be drawn because of the potential reverse causal effect. The specific distinctions about the type, duration, and intensity of exercise, as well as the best solution for the types of exercise that patients with chronic kidney disease can choose, are difficult to determine [Citation22, Citation23]. Thus, in this study, a two-sample Mendelian randomization (MR) method was used to detect the causal effect between different types of physical activities and CKD.
In the MR analysis, single nucleotide polymorphisms (SNPs) that are strongly related to exposure (e.g., heavy physical activity) are used as instrumental variables (IVs) to estimate the causal effect with outcome (e.g., CKD and eGFR) [Citation24]. In reality, MR is a ‘natural’ RCT [Citation25] that makes use of the random distribution of genetic variants that influence exposure [Citation26]. SNPs that are strongly associated with confounders, such as hypertension and diabetes, will be removed before performing MR analysis to exclude confounding factors [Citation27]. Reverse MR analysis can rule out potential reverse causality between exposures and outcomes [Citation28]. In this study, five types of physical activity with different intensities were used to explore their relationship with CKD. Physical activities included heavy DIY (e.g., weeding, lawn mowing, carpentry, and digging), light DIY (e.g., pruning and watering the lawn), strenuous sports, walking for pleasure, and other exercises (e.g., swimming, cycling, keeping fit, and bowling). Bilateral MR was further performed by using previous genome-wide association study (GWAS) datasets to examine the causal association between physical activity, eGFR, and CKD.
2. Materials and methods
2.1. Physical activities and kidney function GWAS summary statistics
Summary statistics of different types of physical activity were extracted from a public online database (IEU OpenGWAS Project https://gwas.mrcieu.ac.uk/). Five types of physical activity with different intensities were applied to explore the causal relationship with kidney function: heavy DIY (197,006 cases, 263,370 controls), light DIY (236,244 cases, 224,132 controls), strenuous sports (47,468 cases, 412,908 controls), walking for pleasure (329,755 cases, 130,621 controls), and other exercises (222,470 cases, 237,906 controls). Data on physical activities were collected by using touchscreen questionnaires; participants were able to choose the specific type of physical activity that they had been involved in the last 4 weeks. Moreover, the survey covered 497,174 European participants, including both males and females (UK Biobank: https://biobank.ctsu.ox.ac.uk/crystal/field.cgi?id=6164). The physical activity assessment in this study was validated by 4 instances, including 497,235 participants, thus ensuring a large enough sample size for reliable results; specifically, instance 0 was the initial assessment visit (2006–2010), instance 1 was the first repeat assessment visit (2012–13), instance 2 was the imaging visit (2014), and the last instance was the first imaging visit (2019).
For reliable and professional MR analysis results, we downloaded CKD summary statistics (CKD Overall and eGFR) from the CKD Gen Consortium, which is a GWAS database concentrating on CKD[Citation29]. In the meta-analysis, the eGFR GWAS contained 42 studies and 783,978 European-ancestry individuals, and the CKD overall GWAS included 23 studies (n = 480,698 European ancestry individuals, 41,395 cases, and 439,303 controls) [Citation29]. Based on eGFR below 60 mL/min/1.73 m2, participants were classified as CKD GFR categories 3–5 (G3–G5) and were diagnosed by using ICD-10-based clinical diagnoses [Citation29]. The Schwartz formula was used to calculate eGFR for people aged <18 years [Citation30] and adults (>18 years of age) by using the Chronic Kidney Disease Epidemiology Collaboration equation [31].
2.2. Instrumental variable selection
The instrumental variables of exposure were selected by using the R package TwosampleMR with a precise filter setting [Citation32, Citation33]. Only SNPs with a p value of less than 5.0e–08 (considered to be genome-wide significant) were selected from all of the SNPs associated with our exposures [Citation34].
All of the SNPs with linkage disequilibrium (LD) were removed to avoid biased results (clumping defaults: R2 = 0.001, window size =10,000 kb, European Population). All of the SNPs for instrumental variables were uploaded to PhenoScanner to identify confounding SNPs associated with CKD, such as hypertension, diabetes, and cardiovascular diseases [Citation35]. Based on the assumption of the MR analysis, SNPs used as instrumental variables should be strongly associated with exposure. Thus, the F statistic (F = R2(N−k−1)/k(1−R2), N = sample size, k = number of instrumental variables, R2 = variance of exposure explained by instrumental variables) can likely exclude weak instrumental variable bias if the F-statistic is significantly larger than 10 [Citation36, Citation37].
2.3. Mendelian randomization analysis
All of the genetic variants must adhere to three instrumental variable assumptions: ① genetic variants are strongly associated with exposure; ② genetic variants influence outcome only by exposure; and ③ genetic variants are not affected by other confounding factors that affect the outcome [Citation38, Citation39]. In this study, several reliable MR methods were applied to explore the causality between physical activity (heavy DIY, light DIY, strenuous sports, walking for pleasure, and other exercises) and kidney function (CKD and eGFR), including inverse variance weighted (IVW), MR–Egger, weighted median (WMM), and MR-Pleiotropy RESidual Sum and Outlier (MR-PRESSO) methods.
In this MR study, our principal method was the inverse variance weighted (IVW) method, which combines the Wald ratio together, and the weight of each ratio is the inverse of the variance of the SNP-outcome association [Citation40, Citation41]. and
are the coefficients from the regressions of the outcome and exposure, respectively, on the SNP instrument [Citation42].
IVW is a reliable and robust MR method in the absence of horizontal pleiotropy, wherein it combines every single SNP’s Wald estimate and obtains the overall estimates of the effect of physical activities on kidney function. There are two types of IVW approaches, including the fixed effect model and random effect model, for existing heterogeneity [Citation32, Citation43]. Cochran’s Q test was performed for heterogeneity in the IVW method and MR–Egger regression; a P value < 0.05 was considered to be statistically significant [Citation41]. In this study, the IVW method was considered the principal method, and the statistical conclusions were based on this method. Compared with IVW, the MR–Egger method adds an intercept item, and its main function is to test the horizontal pleiotropy; if the intercept term differs from zero, then the genetic variants are not all valid instruments, and the IVW estimates are biased [Citation44]. When the instrument strength independent of direct effect (InSIDE) assumption is satisfied, the MR–Egger method can provide an estimate of the causal effect of horizontal pleiotropy [Citation41, Citation44]. The weighted median method can provide a robust effect estimate, although horizontal pleiotropy is unbalanced (even if 50% of instrumental SNPs are not valid) [Citation45]. The MR-PRESSO method has three types of detection functions: horizontal pleiotropic detection, horizontal pleiotropic correction (after removing outliers), and whether there is a difference in the causality estimation results before and after the correction [Citation46].
Bidirectional two-sample MR was performed; moreover, in the reverse MR analysis, CKD and eGFR were exposures, and physical activities were outcomes. After Bonferroni correction for multiple testing, a P value of 0.025 was considered to be statistically significant.
2.4. Sensitivity analysis
The MR Egger regression and MR-PRESSO methods (number of distributions was 5000) were used for horizontal pleiotropy testing. Cochran’s Q test including IVW and MR Egger was used to detect heterogeneity; if the p value was <0.05, we concluded that heterogeneity was significant. Finally, ‘leave-one-out’ (LOO) sensitivity analysis was performed to identify influential SNPs. If influential SNPs are present, causal estimates are not robust.
2.5. Ethics
All of the GWAS summary statistics were obtained from an online public database. No additional ethics approval or informed consent was needed because the GWAS summary statistics were the foundation for all of the publicly accessible study analyses.
3. Results
3.1. Physical activities and CKD
The MR analysis results for the three methods are contained in for physical activities and CKD; sensitivity analysis results are summarized in Supplementary Material S1 Table 1. The MR estimates showed that heavy DIY was a protective factor for CKD (IVW: OR [95% CI] 0.287 (0.117–0.705), p = 0.0065; WMM: OR [95% CI] 0.395 (0.128–1.221), p = 0.107; MR–Egger: OR [95% CI] 0.376 (0.001–133.495), p = 0.748). Under Bonferroni correction (p < 0.025), there was no causal effect of the other four types of physical activities on CKD. Heterogeneity was not detected in the Cochran’s Q test (IVW, Q = 35.04 [df = 17], p = 0.0937; MR–Egger, Q = 25.03 [df = 16], p = 0.069), and there were no outliers according to the MR-PRESSO test, which can lead to heterogeneity. Both MR–Egger and MRPRESSO suggested that horizontal pleiotropy did not exist (the intercept of MR–Egger regression was not different from zero, p = 0.929; MR-PRESSO global test p = 0.115). shows the scatter plots for the MR analysis of the causal effect of physical activity on CKD. Based on the LOO plots (S3. Figure 1), there were no influential SNPs that affected the causal effect between heavy DIY and CKD, and funnel plots showed overall symmetry (S4. Figure 1). The F-statistic for the instrumental variable of Heavy DIY was 35.0, which is much larger than 10. We can conclude that heavy DIY is associated with CKD, and this causal effect is reliable and robust.
Figure 1. Scatter plots for MR analysis of physical activities on CKD for exploring casual effects. a. Heavy DIY b. Light DIY c. Strenuous sports d. Walking for pleasure e. Other exercises.
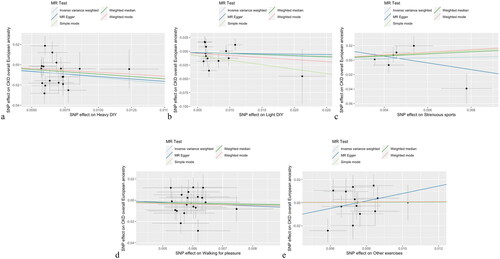
Figure 2. Scatter plots for MR analysis of physical activities on eGFR for exploring casual effects. a. Heavy DIY b. Light DIY c. Strenuous sports d. Walking for pleasure e. Other exercises.
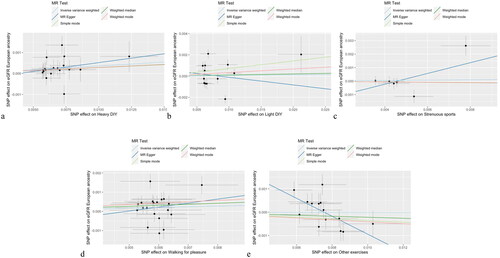
Table 1. MR analysis results from several methods for exploring casual effect of different physical activities on kidney function(CKD and eGFR).
3.2. Physical activities and eGFR
and Supplementary Material S1 Table 1 shows the MR estimates of physical activity on the eGFR and sensitivity analysis results. After Bonferroni correction (p < 0.025), there was a causal link between heavy DIY and eGFR (IVW: β [95% CI] 0.036 [0.005–0.067], p = 0.021; WMM: β [95% CI] 0.029 [−0.008–0.066], p = 0.129; MR–Egger: β [95% CI] 0.085 [−0.116–0.286], p = 0.420). Heterogeneity (Cochran Q: IVW, Q = 21.12 [df = 17], p = 0.22; MR–Egger, Q = 20.82 [df = 16], p = 0.19) and horizontal pleiotropy (intercept = [−0.0003], p = 0.64) were not detected in the MR analysis of heavy DIY on eGFR. shows the scatter plots for the MR analysis of the causal effect of physical activity on eGFR. However, in the LOO analysis, there was a potential influential SNP; thus, the causal effect was not robust (Supplementary Material S3 Figure 1). The other four types of physical activity were not associated with eGFR based on MR estimates and showed strong heterogeneity with horizontal pleiotropy; outliers were detected in the MR-PRESSO test, as shown in Supplementary Material S1 Table 1.
Table 2. MR analysis results from several methods for exploring casual effect of kidney function(CKD and eGFR) on different physical activities.
3.3. Reverse MR analysis
CKD and eGFR were used as exposures, and physical activities were used as outcomes for performing the reverse analysis. Based on the estimates of the reverse MR analysis, there was no reverse causal link between physical activity and kidney function. The MR estimates and sensitivity analysis results are presented in and Supplementary Material S1.
4. Discussion
Clinical studies have explored the association between physical activity and CKD. Some studies have reported that insufficient physical activity leads to poor kidney function. Anemia, changes in cardiovascular morphology and function, changes in skeletal muscle, and insufficient physical activity are risk factors for poor exercise tolerance in patients with chronic kidney disease [Citation47]. Poor exercise tolerance leads to more vicious cycles of insufficient physical activity, and insufficient physical activity itself leads to physical dysfunction and muscle atrophy, which leads to difficulties in daily activities, thus affecting the overall quality of life [Citation47]. A case–control study showed that basic markers of renal function (such as phosphorus and creatinine) in both groups improved after training [Citation48]. A retrospective cohort study including 559 participants in Taiwan found that diabetic patients who engaged in physical activity were less likely to develop CKD [Citation49]. A pilot trial studied the effect of moderate-intensity exercise training on the renal function of patients with progressive chronic kidney disease in stages 3–4 [Citation13]. There was a significant average difference in the change rate of eGFRcr between the rehabilitation group and routine treatment group, and the decline rate of the rehabilitation group was slower [Citation13]. During the 12-month research intervention, there was no significant difference in absolute eGFRcr, eGFRcr-cys, or eGFRcys between groups, which indicated that the influence of a one-year exercise intervention on the progression of kidney disease was uncertain [Citation13]. Additionally, a previous study demonstrated a link between CKD of undetermined origin (CKDu), which is a type of CKD that has been documented globally among rural agricultural groups, and certain risk factors, such as heat stress, dehydration, and particular vocations, such as farming [Citation50]. A recent systematic review and meta-analysis with trial sequential analysis demonstrated that aerobic exercise improves oxidative stress indicators in CKD patients compared to conventional treatment or no exercise [Citation51]. These differences in clinical research may be caused by the differences in research types, research populations, sample sizes, measurement methods, follow-up times, and follow-up results.
Although numerous observational studies have found that physical activity is beneficial for CKD, they cannot prove causality between them. The MR method can be used to prove the causal effect between exposure and the outcome of genetic variants. This two-sample MR study used summary statistics from the UK Biobank and CKDGen consortium. MR estimates proved that heavy physical activity, such as weeding, lawn mowing, carpentry, and digging, had casual effects on CKD and eGFR, which can lower the risk of CKD and increase the eGFR.
Other types of physical activities, such as walking for pleasure, strenuous sports, light DIY (pruning and watering the lawn), and other exercises (e.g., swimming, cycling, keeping fit, and bowling), had no significant correlation with CKD and eGFR. What should be mentioned is that MR estimates between heavy DIY and CKD were reliable and robust after Bonferroni correction and sensitivity analysis. However, one influential SNP was found in the ‘leave-one-out’ test between heavy DIY and eGFR; therefore, although heavy DIY was related to eGFR in MR, we cannot obtain a robust casual effect. The reason for this effect was that CKD source data were based on participants diagnosed with CKD with eGFR less than 60 mL/min/1.73 m2, whereas the median of mean eGFR values was 89 mL min−1 per 1.73 m2 for all of the study participants [Citation29]. This shows that the impact of heavy DIY on chronic kidney disease patients with eGFR below 60 mL min-1 per 1.73 m2 (CKD categories 3–5 [G3–G5]) is more significant.
There are no observational studies or Mendelian randomization analyses examining the correlation and causation between different types of physical activities and kidney function. The two-sample method that was used in this MR study has a large amount of summary genetic data and can avoid potential confounding factors and reverse causality in observational research. The second benefit is that genetically predicted heavy DIY was identified in a large GWAS of 480,698 European participants, and there were no weak instrumental variables (F-statistic > 30 for all exposures). By using bidirectional MR, we discovered evidence of only one direction of this relationship, wherein heavy DIY showed a potential causal relationship with CKD; however, CKD did not demonstrate such a relationship with heavy DIY. From clinical observations, it is illogical that swimming and cycling are less beneficial than weeding and lawn mowing. However, unlike clinical observations, Mendelian randomization focuses on causality at the genetic level, and the heritability of different types of exercise tendencies is different [Citation21].
In a male twin cohort study, the genetic and environmental components of moderate and intense PA were quantified, and familial clustering of this activity was estimated [Citation21]. Correlations between monozygotic and dizygotic twins were examined to ascertain the genetic and nongenetic causes of phenotypic variance, and a previous study found that familial effects accounted for a large portion of the phenotypic diversity for both moderate and vigorous activities [Citation21]. Genes may have a greater impact on regular engagement in some high-intensity exercises than on moderate activities [Citation21]. Another study used data from the UK Biobank to perform the largest genome-wide association study of PA to date. This study examined genetic correlations of PA with other traits and diseases and found that habitual PA appears to be heritable [Citation52]. therefrom these results, there is some concordance with our research. We studied different types of physical activity and found that heavy DIY (e.g., weeding, lawn mowing, carpentry, and digging) was a protective factor for kidney function.
The limitations of our MR study should be considered. Only European population GWAS summary statistics were used in this study; thus, the conclusion is not applicable to other populations, such as Asians. Additionally, there are no GWAS data on different stages of CKD or eGFR in the published databases. Sample overlap cannot be calculated in this study; however, strong instrumental variables overcame this bias. Potential confounders, such as environmental factors, occupational factors, and CKD treatment, were difficult to exclude.
In addition, although we performed the most recent array of sensitivity tests to detect horizontal pleiotropy, it is impossible to completely rule out pleiotropic mechanisms without comprehensive functional follow-up of these loci because we do not yet know the biological activity of these SNPs [Citation53]. Although vertical pleiotropy (wherein one exposure influences an outcome via other variables along the same causal pathway) is acceptable, horizontal pleiotropy is a risk factor for MR inference [Citation40].
Despite these limitations, our use of MR serves as a test to determine whether genetic tools can independently support the associations between heavy physical activity and CKD risk that may be protective. The MR method also avoids the potential confounding factors and reverse causality in observational studies. If heavy DIY truly lowers the risk of CKD, it would be beneficial to encourage physical activity, not only for the general population (where it can result in public health benefits at the level of increased productivity and decreased health care costs) but also for people who are at risk of developing CKD.
5. Conclusion
In this study, we obtained a valid conclusion using the MR method that heavy physical activity (weeding, lawn mowing, carpentry, and digging) has a reliable causal effect on CKD. Our results suggest a possible protective association between heavy physical activity and CKD. Overall, this study supports the hypothesis that heavy physical activity (weeding, lawn mowing, carpentry, and digging) is an effective preventative strategy for CKD.
Authors’ contributions
Rui Xiao: Conceptualization, Methodology, Software, Writing- Original draft preparation; Li Dong: Visualization, Investigation; Bo Xie and Beizhong Liu: Supervision, Writing- Reviewing and Editing.
Supplemental Material
Download MS Word (238.7 KB)Supplemental Material
Download MS Word (892.6 KB)Supplemental Material
Download MS Excel (18.8 KB)Supplemental Material
Download MS Word (19.3 KB)Acknowledgments
We would like to thank Zhenming Liu for providing for R language programming guidance.
Disclosure statement
The authors declare that the research was conducted in the absence of any commercial or financial relationships that could be construed as potential conflicts of interest.
Additional information
Funding
References
- Kalantar-Zadeh K, Jafar TH, Nitsch D, et al. Chronic kidney disease. Lancet. 2021;398(10302):1–9. doi: 10.1016/S0140-6736(21)00519-5.
- Global, regional, and national burden of chronic kidney disease, 1990–2017: a systematic analysis for the global burden of disease study 2017. Lancet. 2020;395(10225):709–733.
- Webster AC, Nagler EV, Morton RL, et al. Chronic kidney disease. Lancet. 2017;389(10075):1238–1252. doi: 10.1016/S0140-6736(16)32064-5.
- Goolsby MJ. National kidney foundation guidelines for chronic kidney disease: evaluation, classification, and stratification. J Am Acad Nurse Pract. 2002;14(6):238–242. doi: 10.1111/j.1745-7599.2002.tb00119.x.
- Cockwell P, Fisher LA. The global burden of chronic kidney disease. Lancet. 2020;395(10225):662–664. doi: 10.1016/S0140-6736(19)32977-0.
- Pedersen BK, Saltin B. Evidence for prescribing exercise as therapy in chronic disease. Scand J Med Sci Sports. 2006;16 (Suppl 1):3–63. doi: 10.1111/j.1600-0838.2006.00520.x.
- Pedersen BK, Saltin B. Exercise as medicine - evidence for prescribing exercise as therapy in 26 different chronic diseases. Scand J Med Sci Sports. 2015;25 (Suppl 3):1–72. doi: 10.1111/sms.12581.
- Kim Y, Wijndaele K, Sharp SJ, et al. Specific physical activities, sedentary behaviours and sleep as long-term predictors of accelerometer-measured physical activity in 91,648 adults: a prospective cohort study. Int J Behav Nutr Phys Act. 2019;16(1):41. doi: 10.1186/s12966-019-0802-9.
- Hawkins MS, Sevick MA, Richardson CR, et al. Association between physical activity and kidney function: national health and nutrition examination survey. Med Sci Sports Exerc. 2011;43(8):1457–1464. doi: 10.1249/MSS.0b013e31820c0130.
- Mustata S, Groeneveld S, Davidson W, et al. Effects of exercise training on physical impairment, arterial stiffness and health-related quality of life in patients with chronic kidney disease: a pilot study. Int Urol Nephrol. 2011;43(4):1133–1141. doi: 10.1007/s11255-010-9823-7.
- Kosmadakis GC, John SG, Clapp EL, et al. Benefits of regular walking exercise in advanced pre-dialysis chronic kidney disease. Nephrol Dial Transplant. 2012;27(3):997–1004. doi: 10.1093/ndt/gfr364.
- Wilkinson TJ, Shur NF, Smith AC. Exercise as medicine’ in chronic kidney disease. Scand J Med Sci Sports. 2016;26(8):985–988. doi: 10.1111/sms.12714.
- Greenwood SA, Koufaki P, Mercer TH, et al. Effect of exercise training on estimated GFR, vascular health, and cardiorespiratory fitness in patients with CKD: a pilot randomized controlled trial. Am J Kidney Dis. 2015;65(3):425–434. doi: 10.1053/j.ajkd.2014.07.015.
- Nakamura K, Sasaki T, Yamamoto S, et al. Effects of exercise on kidney and physical function in patients with non-dialysis chronic kidney disease: a systematic review and meta-analysis. Sci Rep. 2020;10(1):18195. doi: 10.1038/s41598-020-75405-x.
- Bauman AE, Reis RS, Sallis JF, et al. Correlates of physical activity: why are some people physically active and others not? Lancet. 2012;380(9838):258–271. doi: 10.1016/S0140-6736(12)60735-1.
- Trost SG, Owen N, Bauman AE, et al. Correlates of adults’ participation in physical activity: review and update. Med Sci Sports Exerc. 2002;34(12):1996–2001. doi: 10.1097/00005768-200212000-00020.
- den Hoed M, Brage S, Zhao JH, et al. Heritability of objectively assessed daily physical activity and sedentary behavior. Am J Clin Nutr. 2013;98(5):1317–1325. doi: 10.3945/ajcn.113.069849.
- Gielen M, Westerterp-Plantenga MS, Bouwman FG, et al. Heritability and genetic etiology of habitual physical activity: a twin study with objective measures. Genes Nutr. 2014;9(4):415. doi: 10.1007/s12263-014-0415-5.
- Stubbe JH, Boomsma DI, Vink JM, et al. Genetic influences on exercise participation in 37,051 twin pairs from seven countries. PLOS One. 2006;1(1):e22. doi: 10.1371/journal.pone.0000022.
- Joosen AM, Gielen M, Vlietinck R, et al. Genetic analysis of physical activity in twins. Am J Clin Nutr. 2005;82(6):1253–1259. doi: 10.1093/ajcn/82.6.1253.
- Lauderdale DS, Fabsitz R, Meyer JM, et al. Familial determinants of moderate and intense physical activity: a twin study. Med Sci Sports Exerc. 1997;29(8):1062–1068. doi: 10.1097/00005768-199708000-00012.
- Heiwe S, Jacobson SH. Exercise training in adults with CKD: a systematic review and meta-analysis. Am J Kidney Dis. 2014;64(3):383–393. doi: 10.1053/j.ajkd.2014.03.020.
- Johansen KL, Painter P. Exercise in individuals with CKD. Am J Kidney Dis. 2012;59(1):126–134. doi: 10.1053/j.ajkd.2011.10.008.
- Emdin CA, Khera AV, Kathiresan S. Mendelian randomization. Jama. 2017;318(19):1925–1926. doi: 10.1001/jama.2017.17219.
- Hingorani A, Humphries S. Nature’s randomised trials. Lancet. 2005;366(9501):1906–1908. doi: 10.1016/S0140-6736(05)67767-7.
- Bennett DA, Holmes MV. Mendelian randomisation in cardiovascular research: an introduction for clinicians. Heart. 2017;103(18):1400–1407. doi: 10.1136/heartjnl-2016-310605.
- Smith GD, Ebrahim S. Mendelian randomization’: can genetic epidemiology contribute to understanding environmental determinants of disease? Int J Epidemiol. 2003;32(1):1–22. doi: 10.1093/ije/dyg070.
- Haycock PC, Burgess S, Wade KH, et al. Best (but oft-forgotten) practices: the design, analysis, and interpretation of mendelian randomization studies. Am J Clin Nutr. 2016;103(4):965–978. doi: 10.3945/ajcn.115.118216.
- Wuttke M, Li Y, Li M, et al. A catalog of genetic loci associated with kidney function from analyses of a million individuals. Nat Genet. 2019;51(6):957–972. doi: 10.1038/s41588-019-0407-x.
- Schwartz GJ, Schneider MF, Maier PS, et al. Improved equations estimating GFR in children with chronic kidney disease using an immunonephelometric determination of cystatin C. Kidney Int. 2012;82(4):445–453. doi: 10.1038/ki.2012.169.
- Levey AS, Stevens LA, Schmid CH, et al. A new equation to estimate glomerular filtration rate. Ann Intern Med. 2009;150(9):604–612. doi: 10.7326/0003-4819-150-9-200905050-00006.
- Hemani G, Zheng J, Elsworth B, et al. The MR-Base platform supports systematic causal inference across the human phenome. Elife.2018;7:7. doi: 10.7554/eLife.34408.
- Hemani G, Tilling K, Davey Smith G. Orienting the causal relationship between imprecisely measured traits using GWAS summary data. PLOS Genet. 2017;13(11):e1007081. doi: 10.1371/journal.pgen.1007081.
- Risch N, Merikangas K. The future of genetic studies of complex human diseases. Science. 1996;273(5281):1516–1517. doi: 10.1126/science.273.5281.1516.
- Staley JR, Blackshaw J, Kamat MA, et al. PhenoScanner: a database of human genotype-phenotype associations. Bioinformatics. 2016;32(20):3207–3209. doi: 10.1093/bioinformatics/btw373.
- Burgess S, Thompson SG, Avoiding bias from weak instruments in mendelian randomization studies. Int J Epidemiol. 2011;40(3):755–764. doi: 10.1093/ije/dyr036.
- Burgess S, Thompson SG. Bias in causal estimates from mendelian randomization studies with weak instruments. Stat Med. 2011;30(11):1312–1323. doi: 10.1002/sim.4197.
- Didelez V, Sheehan N. Mendelian randomization as an instrumental variable approach to causal inference. Stat Methods Med Res. 2007;16(4):309–330. doi: 10.1177/0962280206077743.
- Greenland S. An introduction to instrumental variables for epidemiologists. Int J Epidemiol. 2018;47(1):358–358. doi: 10.1093/ije/dyx275.
- Burgess S, Butterworth A, Thompson SG. Mendelian randomization analysis with multiple genetic variants using summarized data. Genet Epidemiol. 2013;37(7):658–665. doi: 10.1002/gepi.21758.
- Bowden J, Del Greco MF, Minelli C, et al. Improving the accuracy of two-sample summary-data mendelian randomization: moving beyond the NOME assumption. Int J Epidemiol. 2019;48(3):728–742. doi: 10.1093/ije/dyy258.
- Boehm FJ, Zhou X. Statistical methods for mendelian randomization in genome-wide association studies: a review. Comput Struct Biotechnol J. 2022;20:2338–2351. doi: 10.1016/j.csbj.2022.05.015.
- Burgess S, Dudbridge F, Thompson SG. Combining information on multiple instrumental variables in mendelian randomization: comparison of allele score and summarized data methods. Stat Med.2016;35(11):1880–1906. doi: 10.1002/sim.6835.
- Burgess S, Thompson SG. Interpreting findings from mendelian randomization using the MR-egger method. Eur J Epidemiol. 2017;32(5):391–392. doi: 10.1007/s10654-017-0276-5.
- Bowden J, Davey Smith G, Haycock PC, et al. Consistent estimation in mendelian randomization with some invalid instruments using a weighted median estimator. Genet Epidemiol. 2016;40(4):304–314. doi: 10.1002/gepi.21965.
- Verbanck M, Chen CY, Neale B, et al. Detection of widespread horizontal pleiotropy in causal relationships inferred from mendelian randomization between complex traits and diseases. Nat Genet. 2018;50(8):1196–1698. doi: 10.1038/s41588-018-0164-2.
- Master Sankar Raj V, Patel DR, Ramachandran L. Chronic kidney disease and sports participation by children and adolescents. Transl Pediatr. 2017;6(3):207–214. doi: 10.21037/tp.2017.06.03.
- Silva-Filho A, Azoubel LA, Barroso RF, et al. A case-control study of exercise and kidney disease: hemodialysis and transplantation. Int J Sports Med. 2019;40(3):209–217.
- Lin HC, Peng CH, Chiou JY, et al. Physical activity is associated with decreased incidence of chronic kidney disease in type 2 diabetes patients: a retrospective cohort study in Taiwan. Prim Care Diabetes. 2014;8(4):315–321. Dec doi: 10.1016/j.pcd.2014.04.004.
- Singh NP, Gupta AK, Kaur G, et al. Chronic kidney disease of unknown Origin - What do we know? J Assoc Physicians India. 2020;68(2):76–79.
- Zhao M, Xiao M, Tan Q, et al. The effect of aerobic exercise on oxidative stress in patients with chronic kidney disease: a systematic review and meta-analysis with trial sequential analysis. Ren Fail. 2023;45(2):2252093.
- Klimentidis YC, Raichlen DA, Bea J, et al. Genome-wide association study of habitual physical activity in over 377,000 UK biobank participants identifies multiple variants including CADM2 and APOE. Int J Obes. 2018;42(6):1161–1176. doi: 10.1038/s41366-018-0120-3.
- Choi KW, Chen CY, Stein MB, et al. Assessment of bidirectional relationships between physical activity and depression among adults: a 2-sample mendelian randomization study. JAMA Psychiatry. 2019;76(4):399–408. doi: 10.1001/jamapsychiatry.2018.4175.