Abstract
Background
Acute kidney injury (AKI) is one of the most common complications for critically ill patients with cirrhosis, but it has remained unclear whether urine output fluctuations are associated with the risk of AKI in such patients. Thus, we explored the influence of 24-h urine-output trajectory on AKI in patients with cirrhosis through latent category trajectory modeling.
Materials and Methods
This retrospective cohort study examined patients with cirrhosis using the MIMIC-IV database. Changes in the trajectories of urine output within 24 h after admission to the intensive care unit (ICU) were categorized using latent category trajectory modeling. The outcome examined was the occurrence of AKI during ICU hospitalization. The risk of AKI in patients with different trajectory classes was explored using the cumulative incidence function (CIF) and the Fine-Gray model with the sub-distribution hazard ratio (SHR) and the 95% confidence interval (CI) as size effects.
Results
The study included 3,562 critically ill patients with cirrhosis, of which 2,467 (69.26%) developed AKI during ICU hospitalization. The 24-h urine-output trajectories were split into five classes (Classes 1–5). The CIF curves demonstrated that patients with continuously low urine output (Class 2), a rapid decline in urine output after initially high levels (Class 3), and urine output that decreased slowly and then stabilized at a lower level (Class 4) were at higher risk for AKI than those with consistently moderate urine output (Class 1). After fully adjusting for various confounders, Classes 2, 3, and 4 were associated with a higher risk of AKI compared with Class 1, and the respective SHRs (95% CIs) were 2.56 (1.87–3.51), 1.86 (1.34–2.59), and 1.83 1.29–2.59).
Conclusions
The 24-h urine-output trajectory is significantly associated with the risk of AKI in critically ill patients with cirrhosis. More attention should be paid to the dynamic nature of urine-output changes over time, which may help guide early intervention and improve patients’ prognoses.
Background
Worldwide, approximately 2 million people die from liver disease each year, and 1 million of them die from complications of cirrhosis [Citation1]. Cirrhosis and its complications are also a leading cause of death in patients in intensive care units (ICUs) [Citation2]. Acute kidney injury (AKI) is one of the most common complications of cirrhosis and is defined by an abrupt loss of renal function [Citation3–6]. A systematic review including 18,474 patients showed that more than 30% of patients with cirrhosis developed AKI during hospitalization [Citation7]. The occurrence of AKI in patients with cirrhosis is significantly associated with increased in-hospital and 1-year mortality [Citation8].
AKI in critically ill patients is often difficult to cure, has a poor prognosis, and requires a huge amount of public health resources. However, with timely intervention and effective treatment, AKI can be reversed at an early stage, thereby reducing the risk of AKI-related mortality [Citation9]. Therefore, there is a need to find effective tools and indicators to identify high-risk patients early and improve their prognosis. Serum biomarkers such as neutrophil gelatinase-associated lipocalin (NGAL) and interleukin-18 (IL-18) are closely related to endogenous AKI and may be reliable indicators for predicting AKI [Citation10,Citation11]. Moreover, several studies have suggested that a number of molecules could be used as biomarkers for the detection of kidney injury prior to serum creatinine elevation, including cystatin C, neutrophil gelatinase-associated lipocalin, kidney injury molecule-1, and liver-type fatty acid binding protein [Citation12]. However, these biomarkers have not contributed significant value in the clinical decision-making process, and there is still a large gap between theory and clinical application due to the high cost and inconvenience of obtaining measurements [Citation13].
According to the Improving Global Outcomes in Kidney Disease (KDGIO) standards, the most important clinical indicators for the diagnosis of AKI are blood creatinine levels and urine output [Citation14]. Studies have shown that urine output has better diagnostic value for AKI than creatinine [Citation15,Citation16]. Because an increase in serum creatinine usually follows a decline in renal function, serum creatinine has been considered a delayed predictor of renal function impairment during the course of critical illnesses [Citation17]. A decrease in urine output may be the first sign of renal dysfunction. Alferi et al. showed that variations in urine output could be used to predict the onset of AKI more than 12 h in advance in critically ill patients [Citation18].
Critically ill patients experience rapid changes in their condition during ICU stays, and accordingly, many indicators change dynamically. For monitoring critical illness, the level of a single index at a single point in time yields inaccurate results, and the dynamic change of the indicator with the severity of illness must also be considered. Yang et al. found that the prognosis of patients with acute brain injury was not only associated with the level of intracranial pressure at baseline, but also with dynamic changes in intracranial pressure [Citation19]. In addition, dynamic monitoring of urine output over 24 h has been shown to be valuable in predicting AKI in septic patients [Citation20].
However, it remains unclear whether fluctuations in urine output are associated with the risk of AKI in critically ill patients with cirrhosis. If such an association could be identified, it would provide a new approach to improve the management of critically ill patients with cirrhosis and thus improve survival. Therefore, the aim of this study was to investigate the association between urine-output trajectories within 24 h and the risk of AKI in patients with cirrhosis.
Methods
Data source
The Medical Information Mart for Intensive Care IV (MIMIC-IV) database was used to gather data for the study (https://mimic-iv.mit.edu/). The MIMIC-IV database contains thorough, de-identified data from over 50,000 admissions from 2008 to 2019 at the Beth Israel Deaconess Medical Center in Boston, Massachusetts. The data include demographics, clinical measurements, interventions, history, clinical laboratory testing, and medical data. This study used data from MIMIC-IV 2.0, of which the latest version was released in October 2022.
Because the study was conducted entirely using a third-party, anonymous, publicly available database, the requirement for individual patient consent was waived. Moreover, because the MIMIC project has been approved by the institutional review board (IRB) of Beth Israel Deaconess Medical Center, the study was exempt from the requirement for IRB approval from our organization. We completed the courses required for qualification to use the database and gained access to it (Record ID: 54410528; 54410529).
Study population
The inclusion criteria are as follows: (1) patients with cirrhosis identified according to the 9th revision codes (5712, 5715, and 5716) and 10th revision codes (K703, K7030, K7031, K741, K742, K743, K744, K746, K7460, and K7469) of the International Classification of Diseases (ICD) and (2) complete continuous urine output records within 24 h after ICU admission. The exclusion criteria were (1) patients who received renal replacement therapy, patients receiving any diuretics on day 1, or angiotensin receptor blocker treatment; (2) patients who had renal diseases, heart failure, severe infection, or other severe comorbidities; (3) AKI diagnosed within 24 h after ICU admission; and (4) patients with incomplete information. For patients who were admitted to the ICU more than once, only the first ICU stay was included in the analysis.
Exposure and outcome
After using the database’s identifiers (stay_id) to determine the study population, we utilized Structured Query Language (SQL) to acquire all the information from the MIMIC-IV database. Urine output (mL/kg/h) was defined as the average urine output (mL) per kilogram of body weight per hour for each time period as follows: urine output in the first six hours = total urine output in the first six hours/(body weight × 6). The urine-output changes at the 1st, 2nd, 3rd, and 4th six-hour intervals after ICU admission were used as the exposure variable in our investigation.
In epidemiology, latent class trajectory modeling (LCTM) is usually used to describe exposure during the life course, which is a method to identify different groups with similar underlying trajectories in longitudinal data with the purpose of simplifying heterogeneous populations into homogeneous patterns or categories [Citation21]. Longitudinal studies can be difficult to summarize due to the large amount of data provided by long-term studies. LCTM allows researchers to summarize data from multiple time points in an unbiased manner to identify patterns as this approach does not require prior knowledge of the number or direction of existing tracks in a given population. Therefore, LCTM is a useful tool to summarize data to identify high-risk groups, which can then be targeted for intervention or prevention strategies [Citation22,Citation23].
We used the LCTM to classify 24-h urine-output trajectories in patients with cirrhosis. Indicators reflecting the goodness of fit of the LCTM include log-likelihood, entropy, and information criteria such as the Akaike information criterion (AIC), Bayesian information criterion (BIC), and sample-adjusted BIC (SABIC) [Citation24]. The optimal number of classes was determined using the lowest information criteria, best goodness of fit, highest log-likelihood, and entropy.
In order to ensure the accuracy of the statistical analysis, we required the case number in each category to be at least 1% of the total cases. Moreover, a variety of posterior probability analyses were used to evaluate the model fitting effect. Posterior probability values ranged from 0% to 100%, with values closer to 100% indicating more accurate classification. The occurrence of AKI was defined using the International Club of Ascites (ICA) criteria as follows: (1) increased serum creatinine (SCr) ≥ 0.3 mg/dL (≥ 26.5 µmol/L) within 48 h and (2) increase in SCr to ≥ 1.5 times from baseline within 7 days [Citation25,Citation26]. Follow-up started with the ICU admission and continued until the earliest onset of AKI or discharge.
Covariates
The following information was used as covariates in this study: (1) demographic features, including age, sex, and ethnicity; (2) clinical therapy, including antibiotics, mechanical ventilation, and vasopressors; (3) disease severity scores, including the Sequential Organ Failure Assessment (SOFA), Simplify Acute Physiological Scores II (SAPSII), and Charlson comorbidity index; and (4) laboratory test indicators, including blood urea nitrogen (BUN), white blood cells (WBC), chloride, creatinine, sodium, potassium, anion gap, bicarbonate, hemoglobin, platelets, and glucose. Volume-related weight gain (VRWG) was also calculated as the difference between body weight at admission and after 24 h in the ICU. VRWG ≥ 10% was considered as indicative of a risk for fluid overload [Citation27]. To minimize bias due to loss of data, patients with incomplete data were excluded from the final cohort.
Statistical analyses
Continuous variables were expressed as the mean ± standard deviation (SD) or the median and interquartile range (IQR). Categorical variables are reported as numbers and percentages (n (%)). For comparison of differences between groups, the Wilcoxon rank-sum test was used for continuous variables, while the chi-squared test or Fisher’s exact test was used for categorical variables.
Using a crude cumulative incidence function (CIF), a cumulative incidence curve was created to show the occurrence of AKI in patients with various trajectory classifications. Four models were then established using the sub-distribution hazard function in the Fine-Gray model with the sub-distribution hazard ratio (SHR) and 95% confidence interval (CI) as size effects to analyze the effect of urine-output trajectory on AKI risk. Model 1 involved a univariate analysis without adjusting for any covariates, while Model 2 was adjusted for age, sex, and ethnicity. In addition to these adjustments, Model 3 also included adjustments for the SOFA, SAPSII, and Charlson comorbidity index based on Model 2. Model 4 included both the adjustments in Model 3 and adjustments for WBC, BUN, chloride, creatinine, sodium, potassium, anion gap, bicarbonate, hemoglobin, platelets, glucose, VRWG, and the use of antibiotics, mechanical ventilation, and vasopressors.
To assess the potential effects of urine-output trajectories, subgroup analyses were also performed based on the sub-distribution hazard function in the Fine-Gary model according to age, sex, ethnicity, use of antibiotics, mechanical ventilation, use of vasopressors, SOFA, SAPSII, and the Charlson comorbidity index. In the competing risk model, any cases of AKI occurring before discharge were treated as target events, and the deaths before AKI occurrence were treated as competitive events. Bilateral P values less than 0.05 were considered statistically significant. All statistical analyses were performed using R (version 4.2.3).
Results
Trajectory grouping based on LCTM model
The goodness-of-fit statistics for the LCTM model are displayed in . As the categories progressed from Class 1 to Class 5, AIC, BIC, and SABIC showed a trend of gradual decline. This trend was different from that of Class 6, which had higher results than Classes 1 to 5. The entropy of Class 5 models was lower than that of Classes 1 to 4 but higher than that of Class 6 models.
Table 1. Statistics for choosing the best number of classes.
In the Class 5 models, the proportion of trajectory samples with the smallest population was 2.97%, which met the preset standard. In contrast, the sample proportion of one of the Class 6 models was 0.67%, which is less than 1%. Therefore, the Class 5 model was considered the optimal classification. The mean posterior probabilities of the five categories of 24-h urine-output changes were 87.92%, 76.68%, 80.29%, 93.94%, and 88.90%, respectively. The average value of these probabilities exceeded 70%, indicating that our results were reliable (Supplementary Table 1).
The trajectories of 24-h urine-output changes for the five classes of models are shown in . Class 1 accounted for 7.95% of the total population, and urine output was always at a medium level between 2 and 2.5 mL/kg/h. Class 2 accounted for 67.15%, and urine output was always at a low level (≤ 1 mL/kg/h). Class 3 accounted for 18.78%, with urine output initially high (4–5 mL/kg/h) and then decreasing (1.2 mL/kg/h). Class 4 accounted for 3.14%, and the urine output started at 2–2.5 mL/kg/h, slowly decreased, and finally stabilized at a lower level of 1.3 mL/kg/h. Class 5 accounted for 2.98%, with the urine output first being 1.2 mL/kg/h, slowly increasing to about 2.5 mL/kg/h, and then slowly decreasing to the original level.
Baseline characteristics
Initially, a total of 18,347 patients with cirrhosis were considered for inclusion in the study. After applying the inclusion and exclusion criteria, 3,562 patients were eventually included, of which 69.26% developed AKI during hospitalization (). The median age of the included patients was 58 years, and the majority were male (64.49%) and White (68.70%). Compared with the other groups, patients in Class 2 were older, had more comorbidities, and had higher rates of using antibiotics, mechanical ventilation, and vasopressors. Moreover, the SOFA score, SAPSII score, and comorbidity index were higher in Class 2 than in other classes. The baseline characteristics of the five classes are shown in .
Figure 2. The flowchart of patient selection. MIMIC: Medical Information Mort for Intensive Care; ICU: intensive care unit; AKI: acute kidney injury.
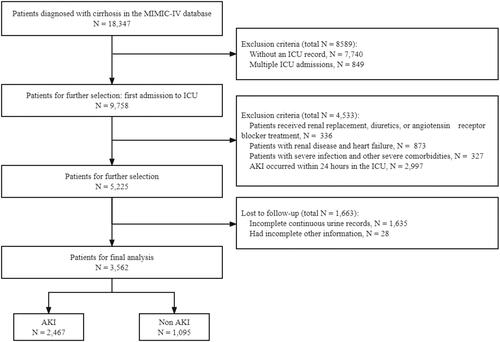
Table 2. Baseline characteristics of all included patients.
Univariate and multivariate analyses
The cumulative incidence curve was plotted using CIF, as shown in . The results showed that the cumulative AKI incidences were significantly different between the five trajectory classes (p < 0.0001). The cumulative AKI incidence in Class 2 was highest among all classes, followed by Classes 3 and 4, while the cumulative AKI incidence in Class 5 was similar to that in Class 1.
The results of the sub-distribution hazard function in the Fine-Gray model are shown in . In the univariate analysis with Class 1 as a reference, Class 2 had the highest risk of AKI (SHR = 2.34, 95% CI: 1.73–3.17, p < 0.001), followed by Class 3 (SHR = 1.78, 95% CI: 1.29–2.45, p < 0.001) and Class 4 (SHR = 1.74, 95% CI: 1.24–2.44, p < 0.001). After fully adjusting for various confounding factors, the same trends were obtained. In the fully adjusted models, Classes 2, 3, and 4 had a higher AKI risk than Class 1 with SHRs of 2.56 (95% CI: 1.87–3.51, p < 0.001), 1.86 (95% CI: 1.34–2.59, p < 0.001), and 1.83 (95% CI: 1.29–2.59, p < 0.001), respectively. Among the four models adjusted for various covariates, the AKI risk of Class 5 was similar to that of Class 1, and they showed no significant differences (p > 0.05).
Subgroup analyses
The outcomes of the subgroup analysis are displayed in . A significant association was observed between urine-output trajectories and the risk of AKI in all subgroups. Furthermore, patients with urine-output trajectory in Class 2 had the highest risk of developing AKI among critically ill patients with cirrhosis. Moreover, patients were more likely to have an increased risk of AKI if they were male, older than 65 years, received vasopressor treatment without antibiotics or mechanical ventilation therapy, and had higher SOFA or comorbidity index scores (P for interaction < 0.05 for all).
Table 3. Results of subgroup analyses.
Discussion
Critically ill patients with cirrhosis have high rates of AKI incidence and mortality [Citation1], and AKI greatly increases the medical burden associated with cirrhosis. Despite some progress in the management of cirrhosis, there is still a significant difference in medical costs between cirrhosis with and without AKI [Citation2]. Addressing this problem requires a better understanding of the mechanisms that drive AKI in the context of cirrhosis. Early identification of patients with a high risk of developing AKI is the basis for taking timely intervention measures and improving the prognosis.
Although urine output is currently included in the criteria for diagnosing and staging AKI, few studies have evaluated dynamic changes in urine output as a specific marker of AKI and its association with patient outcomes. An important finding in our study was a significant association between urine-output trajectories over a 24-h period and the occurrence of AKI. This suggests that closer monitoring of urine output and assessment of the duration and changes in the trajectory of oliguria could provide risk markers for adverse events. Urine output is a sensitive and early marker of AKI and has been associated with adverse outcomes in critically ill patients [Citation16]. Furthermore, it is also influenced by renal tubular function, as evidenced by responses to the furosemide pressure test [Citation28].
Our study has some novel contributions in comparison to some previous reports predicting AKI in critically ill patients. Urine output is a continuous and dynamic indicator that can be measured in the ICU. We classified the 24-h urine-output trajectories in patients with cirrhosis using the LCTM method and identified a total of five urine-output trajectories. Class 2 (persistently low urine output), Class 3 (initially high urine output followed by a rapid decline in urine output), and Class 4 (slowly declining urine output followed by persistently low urine output) had higher incidences of AKI compared to Class 1 (persistently moderate urine output) and Class 5 (increased first and then decreased output).
According to a multicenter cohort study in China, fluid overload was an independent risk factor for AKI in critically ill patients and was associated with increased severity of AKI [Citation29]. Considering that even ‘normal’ urine output may actually be insufficient when severe fluid overload occurs, it is necessary to consider the effect of VRWG on the relationship between urine output and AKI risk. In this study, a very small proportion of patients had VRWG ≥ 10%, and it had little effect on the final outcomes when adjusted as a covariate in multivariate analyses, which further illustrates the stability of our results.
It was not surprising that patients in Class 2 had a higher risk of developing AKI compared to those in Class 1. Paying close attention to the incidence and duration of early decreased urine output is important in the management of critically ill patients in the ICU as it is associated with AKI morbidity and mortality [Citation30]. Vaara et al. found that episodes of severe oliguria (< 0.1 mL/kg/h) over a period of more than 3 h were independently associated with the development of AKI and an increased risk of 90-day mortality, which was independent of serum creatine changes [Citation31].
Furthermore, ascites is a common complication of cirrhosis. A key emerging concept is that fluid accumulation in critical spaces, such as the abdominal cavity, can further impair critical organ function, and increased abdominal pressure from progressive ascites accumulation can impair renal venous discharge and trigger a vicious cycle of escalating organ dysfunction [Citation32]. In terms of parameters affecting renal congestion, changes in intra-abdominal pressure are associated with the risk of AKI [Citation33]. In a study of patients admitted to ICUs, increased intra-abdominal pressure was shown to be an independent risk factor for AKI [Citation34].
Patients in Classes 3 and 4 had a higher risk of AKI than those in Class 1. The rapid decline in urine output suggested that these patients might not have received effective treatment in time or might have had low urine output even after receiving rehydration therapy, leading to an increased incidence of AKI [Citation35]. Zhang et al. found that patients with oliguric AKI had increased urine volume and responded to the volume after receiving > 5 liters of fluid [Citation36]. Moreover, this phenomenon could be attributed in part to the insufficient control of inflammation in advanced cirrhosis. Systemic inflammation plays a critical role in the progression of cirrhosis [Citation37], and renal perfusion and urination are rapidly reduced as a result of a strong inflammatory response that causes endothelial failure, increased vascular permeability, and hypovolemia [Citation38].
In ICU settings, the risk of AKI is significantly higher in males, Black people, and older people [Citation39]. A study in the United States showed that the incidence of AKI gradually increased with age from 13.6 cases per 1000 patient-years in those aged 66–69 years to 46.9 cases per 1000 patient-years in those ≥85 years old [Citation40]. Compared with female patients, male patients tend to have more adverse habits, such as smoking and drinking. A meta-analysis of 28 studies reported sex-stratified data on the incidence of hospital-related AKI among 194 million patients, and men were 2.2 times more likely to develop AKI than women [Citation41].
Since decreased blood pressure is associated with AKI, vasopressors may be effective in preventing AKI [Citation42]. However, in our results, patients who did not receive vasopressors had a lower risk of AKI than those who did, which may be attributed to the inappropriate use of vasopressors in these patients. Busse et al. noted that at high doses, commonly used vascular vasopressors such as catecholamines are significantly associated with the risk of multiple adverse events, including AKI [Citation43].
Patients with cirrhosis are prone to infection, and the site of infection varies depending on the patient’s underlying disease. Common types of infection include spontaneous bacterial peritonitis, biliary tract infection, lung infection, intestinal infection, and urinary tract infections [Citation44]. Therefore, antibiotic therapy is also a routine treatment for critically ill patients with cirrhosis in the ICUs. Antibiotics benefit patients with critical cirrhosis by reducing systemic inflammation responses, thereby reducing the risk of AKI, hepatic encephalopathy, and death [Citation45]. Belcher et al. found that in patients with cirrhosis, greater severity of AKI is associated with increased risk of infectious complications, including bacteremia, pneumonia, and urinary tract infections. This suggested that early diagnosis of AKI and the rational use of antibiotics might result in improved outcomes [Citation46].
Mechanical ventilation is the most widely used support measure in the ICU. In this study, patients with cirrhosis who had a positive response to mechanical ventilation had a lower risk of developing AKI than those who did not receive treatment or who did not respond to mechanical ventilation. However, one issue to consider is that mechanical ventilation can significantly increase mortality in severe cases of cirrhosis [Citation47], so the timing of use should be considered.
This study has several advantages. First, the LCTM model was used to explore and classify the 24-h urine-output trajectory in patients with cirrhosis, and a series of Fine-Gray models were established to adjust for various confounding factors. The results are stable and reliable and could provide a basis for making clinical decisions for patients with cirrhosis. Secondly, the results of this research may help to identify people who are at high risk of AKI prior to diagnosis, thereby enabling early intervention to reduce disease burden and mortality. Another advantage is that urine output is routinely measured in ICUs and is more intuitive, inexpensive, and convenient than laboratory indexes.
However, there are some limitations to this study. First of all, this study was a retrospective, single-center study, making selection bias inevitable, and the inferences that can be made from our conclusions are thus limited. Second, due to the small number of patients with cirrhosis in the ICU, it was difficult to obtain a sufficient number of cases for external validation. Therefore, further multicenter studies are needed to verify the predictive value of 24-h urine-output trajectories for AKI. Third, urine output is the result of a combination of multiple factors, and not all of them were taken into account in this study. Further studies are needed to explore whether these factors affect the trajectories of dynamic changes in urine output. Finally, we only considered the trajectory of urine output within 24 h as a single indicator for predicting AKI and did not consider its synergistic effects in relation to other variables.
Conclusions
This study revealed that the 24-h urine-output trajectory in critically ill patients with cirrhosis is significantly associated with the risk of AKI. Patients with persistently low urine output and those with initially high output followed by a rapid decline had a greater risk of developing AKI than patients with consistently moderate and stable urine output. Therefore, we should pay close attention to changes in urine output, which could help to provide early intervention and reduce the incidence of AKI. Nevertheless, other multicenter and large sample studies are needed to confirm the findings.
Author contributions
Literature search: JSH, ELY and XFH; Study design: JW, YMZ and JYW; Data collection: XLL, SRY and DDN; Data analysis: YMZ, WJ and XFH; Model construction: ELY, SRY and JYW; Manuscript writing: JW and DDN. All authors read and approved the final manuscript.
Abbreviations | ||
AKI | = | Acute kidney injury |
SCr | = | Serum creatinine |
ICU | = | Intensive care unit |
MIMIC-IV | = | Medical Information Mart for Intensive Care IV |
ICA | = | International Club of Ascites |
CIF | = | Cumulative incidence function |
SHR | = | Sub-distribution hazard ratio |
CI | = | Confidence interval |
LCTM | = | Latent category trajectory model |
ICD-9 | = | International Classification of Diseases and Ninth Revision |
ICD-10 | = | International Classification of Diseases and Tenth Revision |
SQL | = | Structured Query Language |
AIC | = | Akaike information criterion |
BIC | = | Bayesian information Criteria |
SABIC | = | ample-adjusted BIC |
SOFA | = | Sequential organ failure assessment |
SAPSII | = | The customized simplified acute physiology score |
BUN | = | Blood urea nitrogen |
WBC | = | White blood cell |
VRWG | = | Volume-related weight gain |
SD | = | Standard deviation |
IQR | = | Interquartile range |
GFR | = | Glomerular filtration rate |
NGAL | = | neutrophil gelatinase-associated lipocalin |
IL-18 | = | Interleukin-18 |
Supplemental Material
Download PDF (50.3 KB)Acknowledgements
The authors appreciate the researchers at the MIT Laboratory for Computational Physiology for publicly sharing of the MIMIC-IV clinical database.
Disclosure statement
No potential conflict of interest was reported by the author(s).
Data availability statement
Data of this study were obtained from the MIMIC-IV database, which was a publicly available dataset, which were exhibited in Materials and Methods.
Additional information
Funding
References
- Mokdad AA, Lopez AD, Shahraz S, et al. Liver cirrhosis mortality in 187 countries between 1980 and 2010: a systematic analysis. BMC Med. 2014;12(1):1. doi: 10.1186/s12916-014-0145-y.
- Weil D, Levesque E, McPhail M, et al. Prognosis of cirrhotic patients admitted to intensive care unit: a meta-analysis. Ann Intensive Care. 2017;7(1):33. doi: 10.1186/s13613-017-0249-6.
- Huelin P, Piano S, Solà E, et al. Validation of a staging system for acute kidney injury in patients with cirrhosis and association with acute-on-Chronic liver failure. Clin Gastroenterol Hepatol. 2017;15(3):438–11. doi: 10.1016/j.cgh.2016.09.156.
- Wong F, O’Leary JG, Reddy KR, et al. Acute kidney injury in cirrhosis: baseline serum creatinine predicts patient outcomes. Am J Gastroenterol. 2017;112(7):1103–1110. doi: 10.1038/ajg.2017.122.
- Patidar KR, Shamseddeen H, Xu C, et al. Hospital-Acquired versus Community-Acquired acute kidney injury in patients with cirrhosis: a prospective study. Am J Gastroenterol. 2020;115(9):1505–1512. doi: 10.14309/ajg.0000000000000670.
- Desai AP, Knapp SM, Orman ES, et al. Changing epidemiology and outcomes of acute kidney injury in hospitalized patients with cirrhosis - a US population-based study. J Hepatol. 2020;73(5):1092–1099. doi: 10.1016/j.jhep.2020.04.043.
- Tariq R, Hadi Y, Chahal K, et al. Incidence, mortality and predictors of acute kidney injury in patients with cirrhosis: a systematic review and meta-analysis. J Clin Transl Hepatol. 2020;8(2):135–142. doi: 10.14218/JCTH.2019.00060.
- Ning Y, et al. Impact of acute kidney injury on the risk of mortality in patients with cirrhosis: a systematic review and meta-analysis. Ren Fail. 2022;44(1):1–14.
- Coelho S, Cabral G, Lopes JA, et al. Renal regeneration after acute kidney injury. Nephrology (Carlton). 2018;23(9):805–814. doi: 10.1111/nep.13256.
- Schrezenmeier EV, Barasch J, Budde K, et al. Biomarkers in acute kidney injury - pathophysiological basis and clinical performance. Acta Physiol (Oxf). 2017;219(3):554–572. doi: 10.1111/apha.12764.
- Vanmassenhove J, Vanholder R, Nagler E, et al. Urinary and serum biomarkers for the diagnosis of acute kidney injury: an in-depth review of the literature. Nephrol Dial Transplant. 2013;28(2):254–273. doi: 10.1093/ndt/gfs380.
- Ronco C, Bellomo R, Kellum JA. Acute kidney injury. Lancet. 2019;394(10212):1949–1964. doi: 10.1016/S0140-6736(19)32563-2.
- Lameire NH, Vanholder RC, Van Biesen WA. How to use biomarkers efficiently in acute kidney injury. Kidney Int. 2011;79(10):1047–1050. doi: 10.1038/ki.2011.21.
- Kellum JA, Lameire N, KDIGO AKI Guideline Work Group. Diagnosis, evaluation, and management of acute kidney injury: a KDIGO summary (part 1). Crit Care. 2013;17(1):204. doi: 10.1186/cc11454.
- Kellum JA, Sileanu FE, Murugan R, et al. Classifying AKI by urine output versus serum creatinine level. J Am Soc Nephrol. 2015;26(9):2231–2238. doi: 10.1681/ASN.2014070724.
- Macedo E, Malhotra R, Bouchard J, et al. Oliguria is an early predictor of higher mortality in critically ill patients. Kidney Int. 2011;80(7):760–767. doi: 10.1038/ki.2011.150.
- Uchino S. Creatinine. Curr Opin Crit Care. 2010;16(6):562–567. doi: 10.1097/MCC.0b013e32833ea7f3.
- Alfieri F, Ancona A, Tripepi G, et al. A deep-learning model to continuously predict severe acute kidney injury based on urine output changes in critically ill patients. J Nephrol. 2021;34(6):1875–1886. doi: 10.1007/s40620-021-01046-6.
- Yang F, Peng C, Peng L, et al. Group-based trajectory modeling of intracranial pressure in patients with acute brain injury: results from multi-center ICUs, 2008-2019. CNS Neurosci Ther. 2022;28(8):1218–1228. doi: 10.1111/cns.13854.
- Zhang L, Xu F, Han D, et al. Influence of the trajectory of the urine output for 24 h on the occurrence of AKI in patients with sepsis in intensive care unit. J Transl Med. 2021;19(1):518. doi: 10.1186/s12967-021-03190-w.
- Lennon H, Kelly S, Sperrin M, et al. Framework to construct and interpret latent class trajectory modelling. BMJ Open. 2018;8(7):e020683. doi: 10.1136/bmjopen-2017-020683.
- Petrick JL, Kelly SP, Liao LM, et al. Body weight trajectories and risk of oesophageal and gastric cardia adenocarcinomas: a pooled analysis of NIH-AARP and PLCO studies. Br J Cancer. 2017;116(7):951–959. doi: 10.1038/bjc.2017.29.
- Kelly SP, Graubard BI, Andreotti G, et al. Prediagnostic body mass index trajectories in relation to prostate cancer incidence and mortality in the PLCO cancer screening trial. J Natl Cancer Inst. 2017;109(3):djw225. doi: 10.1093/jnci/djw225.
- Eriksson J, Nelson D, Holst A, et al. Temporal patterns of organ dysfunction after severe trauma. Crit Care. 2021;25(1):165. doi: 10.1186/s13054-021-03586-6.
- Angeli P, Gines P, Wong F, et al. Diagnosis and management of acute kidney injury in patients with cirrhosis: revised consensus recommendations of the international club of ascites. Gut. 2015;64(4):531–537. doi: 10.1136/gutjnl-2014-308874.
- Xiong J, Zhang M, Guo X, et al. Acute kidney injury in critically ill cirrhotic patients with spontaneous bacterial peritonitis: a comparison of KDIGO and Ica criteria. Arch Med Sci. 2020;16(3):569–576. doi: 10.5114/aoms.2019.85148.
- Fülöp T, Pathak MB, Schmidt DW, et al. Volume-related weight gain and subsequent mortality in acute renal failure patients treated with continuous renal replacement therapy. Asaio J. 2010;56(4):333–337. doi: 10.1097/MAT.0b013e3181de35e4.
- Chawla LS, Davison DL, Brasha-Mitchell E, et al. Development and standardization of a furosemide stress test to predict the severity of acute kidney injury. Crit Care. 2013;17(5):R207. doi: 10.1186/cc13015.
- Wang N, Jiang L, Zhu B, et al. Fluid balance and mortality in critically ill patients with acute kidney injury: a multicenter prospective epidemiological study. Crit Care. 2015;19(1):371. doi: 10.1186/s13054-015-1085-4.
- Goldstein SL. Urine output assessment in acute kidney injury: the cheapest and most impactful biomarker. Front Pediatr. 2019;7:565. doi: 10.3389/fped.2019.00565.
- Vaara ST, Parviainen I, Pettilä V, et al. Association of oliguria with the development of acute kidney injury in the critically ill. Kidney Int. 2016;89(1):200–208. doi: 10.1038/ki.2015.269.
- Malbrain MLNG, Cheatham ML, Kirkpatrick A, et al. Results from the international conference of experts on intra-abdominal hypertension and abdominal compartment syndrome. I. Definitions. Intensive Care Med. 2006;32(11):1722–1732. doi: 10.1007/s00134-006-0349-5.
- Kopitkó C, Medve L, Gondos T. The value of combined hemodynamic, respiratory and intra-abdominal pressure monitoring in predicting acute kidney injury after major intra-abdominal surgeries. Ren Fail. 2019;41(1):150–158. doi: 10.1080/0886022X.2019.1587467.
- Dalfino L, Tullo L, Donadio I, et al. Intra-abdominal hypertension and acute renal failure in critically ill patients. Intensive Care Med. 2008;34(4):707–713. doi: 10.1007/s00134-007-0969-4.
- Hoste EA, Maitland K, Brudney CS, et al. Four phases of intravenous fluid therapy: a conceptual model. Br J Anaesth. 2014;113(5):740–747. doi: 10.1093/bja/aeu300.
- Zhang Z, Ho KM, Hong Y. Machine learning for the prediction of volume responsiveness in patients with oliguric acute kidney injury in critical care. Crit Care. 2019;23(1):112. doi: 10.1186/s13054-019-2411-z.
- Bernardi M, Moreau R, Angeli P, et al. Mechanisms of decompensation and organ failure in cirrhosis: from peripheral arterial vasodilation to systemic inflammation hypothesis. J Hepatol. 2015;63(5):1272–1284. doi: 10.1016/j.jhep.2015.07.004.
- Kindgen-Milles D, Dimski T, Brandenburger T. [Acute kidney injury: epidemiology, pathophysiology, repair mechanisms]. Anasthesiol Intensivmed Notfallmed Schmerzther. 2021;56(2):90–100. doi: 10.1055/a-1105-0587.
- Fisher M, Neugarten J, Bellin E, et al. AKI in hospitalized patients with and without COVID-19: a comparison study. J Am Soc Nephrol. 2020;31(9):2145–2157. doi: 10.1681/ASN.2020040509.
- Chronopoulos A, Rosner MH, Cruz DN, et al. Acute kidney injury in elderly intensive care patients: a review. Intensive Care Med. 2010;36(9):1454–1464. doi: 10.1007/s00134-010-1957-7.
- Neugarten J, Golestaneh L. Female sex reduces the risk of hospital-associated acute kidney injury: a meta-analysis. BMC Nephrol. 2018;19(1):314. doi: 10.1186/s12882-018-1122-z.
- Lamia B, Chemla D, Richard C, et al. Clinical review: interpretation of arterial pressure wave in shock states. Crit Care. 2005;9(6):601–606. doi: 10.1186/cc3891.
- Busse LW, Ostermann M. Vasopressor therapy and blood pressure management in the setting of acute kidney injury. Semin Nephrol. 2019;39(5):462–472. doi: 10.1016/j.semnephrol.2019.06.006.
- Piano S, Singh V, Caraceni P, et al. Epidemiology and effects of bacterial infections in patients with cirrhosis worldwide. Gastroenterology. 2019;156(5):1368–1380.e10. doi: 10.1053/j.gastro.2018.12.005.
- Fernández J, Navasa M, Planas R, et al. Primary prophylaxis of spontaneous bacterial peritonitis delays hepatorenal syndrome and improves survival in cirrhosis. Gastroenterology. 2007;133(3):818–824. doi: 10.1053/j.gastro.2007.06.065.
- Belcher JM, Garcia-Tsao G, Sanyal AJ, et al. Association of AKI with mortality and complications in hospitalized patients with cirrhosis. Hepatology. 2013;57(2):753–762. doi: 10.1002/hep.25735.
- Juneja D, Gopal PB, Kapoor D, et al. Profile and outcome of patients with liver cirrhosis requiring mechanical ventilation. J Intensive Care Med. 2012;27(6):373–378. doi: 10.1177/0885066611400277.