Abstract
Background
Cardiovascular disease (CVD) is a major complication in peritoneal dialysis (PD) patients. Previous studies have demonstrated that platelet distribution width (PDW) is associated with cardiovascular events in hemodialysis (HD) patients. In this study, we hypothesized that elevated PDW can predict all-cause and cardiovascular mortality in PD patients.
Methods
We recruited PD patients for a single-center retrospective cohort study from 1 January 2007, to 30 June 2020. Receiver-operating characteristic (ROC) curves were made to determine the PDW cutoff value for predicting all-cause mortality. The propensity score matching (PSM) method was used to improve the equilibrium between groups. The relation of PDW with all-cause and cardiovascular mortality was analyzed by Cox proportional hazards models. Restricted cubic spline (RCS) models were used to determine whether there was a linear relationship between PDW and all-cause and cardiovascular mortality.
Results
A total of 720 PD patients were screened, and 426 PD patients were enrolled after PSM. After adjusting for confounders, Cox proportional hazards models showed that the PDW value was positively correlated with the risk of all-cause and cardiovascular mortality (HR = 1.162, 95% CI 1.057–1.278, p = 0.002 and HR = 1.200, 95% CI 1.041–1.382, p = 0.012). The adjusted RCS analysis further showed that the relationship of PDW with all-cause and cardiovascular mortality was linear (p for nonlinearly = 0.143 and 0.062).
Conclusion
Elevated PDW is independently associated with all-cause and cardiovascular mortality in PD patients.
Introduction
Peritoneal dialysis (PD) is a common kidney replacement therapy for patients with end-stage kidney disease [Citation1]. It is estimated that there were more than 272,000 patients undergoing PD globally in 2017, accounting for about 11% of all dialysis patients [Citation2]. However, the long-term survival rate for PD patients was relatively low [Citation1], and cardiovascular disease (CVD) is the leading cause of death in PD patients [Citation3].
Platelet distribution width (PDW) is a parameter that reflects platelet volume variation and indicates the platelet volume dispersion in the blood [Citation4]. PDW is also a special marker of platelet activity, and a high PDW value indicates platelet activation [Citation4–6]. A cohort study of patients on maintenance hemodialysis (HD) has revealed that PDW is independently associated with all-cause and cardiovascular mortality [Citation5]. However, the relationship of elevated PDW with all-cause and cardiovascular mortality in PD patients has not been adequately explored [Citation7].
Randomized controlled trials are the gold standard of clinical research design [Citation8]. In practice, however, it is difficult to take this approach due to the constraints of research objects, duration, expense, and ethics. Propensity score matching (PSM) is a statistical method that is used to control confounding factors by balancing baseline covariates [Citation9,Citation10]. PSM allows the balance between groups to achieve the condition of randomized control design by simple statistical processing, so it is increasingly used in non-randomized observational studies [Citation9]. In this study, we investigated the relationship of elevated PDW with all-cause and cardiovascular mortality in PD patients using the method of PSM, and tested the hypothesis that elevated PDW at baseline predicts all-cause and cardiovascular mortality during the course of subsequent PD.
Materials and methods
Study population
This was an observational retrospective cohort study. We included patients who had PD catheter insertion and started PD treatment in the National Clinical Research Center of Kidney Disease, Jinling Hospital, Nanjing University School of Medicine, from 1 January 2007 to 30 June 2020. The inclusion criteria were as follows: age ≥ 18 years, more than 3 months of PD treatment, lack of the history of CVD, PD catheter inserted, and follow-up performed by this center. The patients who had PD catheter insertion by other hospitals, switched from HD or kidney transplant to PD, received PD treatment for less than 3 months, had a history of tumors, or lacked baseline data were excluded from this study.
Data collection
All patients were followed up until cessation of PD, death, switch to HD or renal transplantation, loss to follow-up, or on 30 June 2020. The demographic characteristics included age, sex, a history of diabetes, and hypertension. The baseline clinical and laboratory parameters included body mass index (BMI), Charlson comorbidity index (CCI), albumin, total cholesterol (CH), triglycerides (TG), serum urea nitrogen (BUN), serum creatinine (Scr), cystatin C (Cys C), serum uric acid (UA), alanine transaminase (ALT), glutamate transaminase (AST), serum calcium, serum phosphorus, hemoglobin (Hb), platelet counts (PLT), estimated glomerular filtration rate (eGFR), PDW, and C-reactive protein (CRP). All baseline data were collected at the time of patient admission for PD catheterization. The primary endpoint was all-cause mortality, and the secondary endpoint was cardiovascular mortality. Cardiovascular mortality was defined as death due to acute myocardial infarction, atherosclerotic heart disease, congestive heart failure, arrhythmia, sudden cardiac death, cardiomyopathy, ischemic or hemorrhagic stroke [Citation11].
Statistical analysis
We used the receiver-operating characteristic (ROC) curves to determine the PDW cutoff value for predicting all-cause mortality. The PSM method was used to improve the equilibrium between groups. Data were expressed as mean ± standard deviation, median (25th–75th percentile), or number (percentages). Differences between groups were analyzed with the t-test, nonparametric test, or chi-square test. Survival curves were made by the Kaplan–Meier method. The association of PDW with all-cause and cardiovascular mortality was analyzed by Cox proportional hazards models, and the results were expressed as hazard ratios (HRs) and 95% confidence intervals (95%CI). Restricted cubic spline (RCS) models were used to assess linear relationship between PDW and all-cause and cardiovascular mortality. Factors associated with PDW were determined by Pearson correlation analysis, and the results were expressed as correlation coefficient (r). IBM SPSS version 22.0 (IBM SPSS Statistics, Armonk, NY) and R version 4.3.0 (R Foundation for Statistical Computing) were used for statistical analysis. Survival curves and scatter plots were prepared using GraphPad Prism version 9.0(GraphPad Software, La Jolla, CA). p < 0.05 was considered statistically significant.
Results
Demographic and clinical characteristics of the study population
A total of 1118 patients had PD catheter insertion and started PD treatment in our center between 1 January 2007 and 30 June 2020. Of them, 27 patients were younger than 18 years, 3 patients had kidney transplantation, 9 patients were switched from HD, 28 patients received PD treatment for less than 3 months, 39 patients were followed up for less than 3 months, and 292 patients lacked baseline PDW data. The remaining 720 patients were grouped according to the PDW cutoff values of ROC. After PSM, 426 patients were left for further analyses (shown in ).
Figure 1. Study flow chart. PDW: platelet distribution width; ROC: receiver-operating characteristic curves.
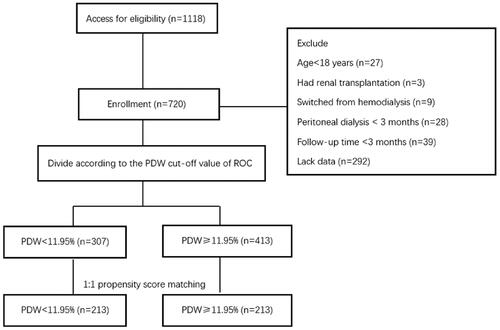
The median follow-up of patients before PSM was 31 (15, 61) months. There were 143 patients continued PD treatment, 247 patients switched to HD treatment, 118 patients underwent kidney transplantation, 103 patients lost in the follow-up, 14 patients stopped PD treatment for improved kidney function, and 95 patients died. Of the 95 deaths, 41 (43.2%) were caused by cardiovascular events.
The median follow-up of patients after PSM was 31 (14, 60) months. Of the patients, 82 continued PD treatment, 158 were switched to HD treatment, 73 underwent kidney transplantation, 49 were lost in the follow-up, 14 stopped PD treatment for improved kidney function, and 50 died. Of the 50 deaths, 20 (40%) were caused by cardiovascular events.
Differences between the high PDW group and the low PDW group before and after propensity score matching
The ROC analysis showed that the PDW cutoff value for predicting all-cause mortality was 11.95% (area under the curve = 0.718, 95% CI = 0.670–0.766, p < 0.001) (shown in ). With this cutoff, the 720 patients were divided into two groups, the high PDW group (PDW ≥11.95%, 413) and the low PDW group (PDW <11.95%, 307) for analyses without PSM. Comparisons of the two groups showed statistically significant differences in the levels of albumin, TG, UA, AST, Cys C, and PLT (p < 0.05), but not other parameters ().
Figure 2. Receiver operating characteristic curve of PDW that predicted all-cause mortality. AUC: Area under the curve.
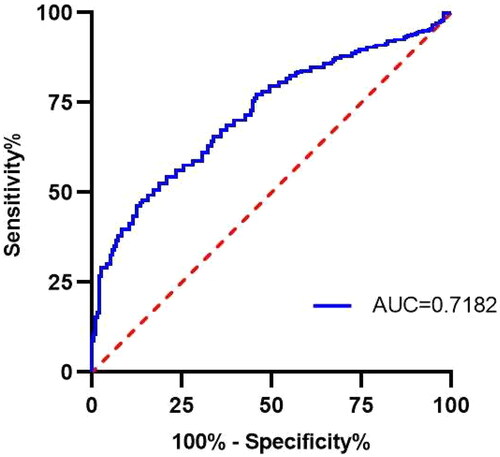
Table 1. Comparisons of baseline data between the two groups before PSM.
By using the 1:1 PSM with a caliper value setting at 0.02, 426 patients were matched, including 213 in the high PDW group and 213 in the low PDW group. There was no statistically significant difference in demographic characteristics and laboratory results between the two groups (p > 0.05). Clearly, the PSM processing improved the equilibrium of baseline data of the two groups ().
Table 2. Comparisons of baseline data between the two groups after PSM.
Association of PDW with all-cause and cardiovascular mortality after propensity score matching
In the high PDW group, all-cause mortality and cardiovascular mortality were 18.3% and 8.9%, respectively, while in the low PDW group, they were 5.2% and 0.5%. The Kaplan–Meier analysis suggested that all-cause mortality and cardiovascular mortality were significantly higher in the high PDW group than the low PDW group (p = 0.005 and p = 0.001, shown in and ).
Figure 3. The Kaplan–Meier survival analysis for all-cause mortality after propensity score matching. PDW: platelet distribution width.
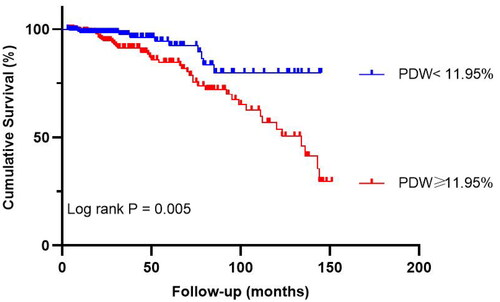
Figure 4. The Kaplan–Meier survival analysis for cardiovascular mortality after propensity score matching. PDW: platelet distribution width.
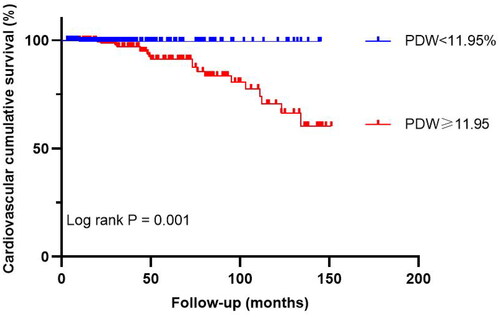
When PDW was included as a continuous variable in the Cox regression analysis, it was found to be associated with all-cause and cardiovascular mortality according to crude analysis (HR = 1.153, 95% CI 1.061–1.252, p = 0.001 and HR = 1.177, 95% CI 1.040–1.331, p = 0.010, , Model 1). When PDW was used as a dichotomous variable, we found that patients in the high PDW group had a higher risk of all-cause and cardiovascular mortality compared with those in the low PDW group (HR = 2.556, 95% CI 1.304–5.010, p = 0.006 and HR = 13.667, 95% CI 1.826–102.298, p = 0.011, , Model 1). In the adjustment models, the association remained (p < 0.05, , Model 2,3).
Table 3. Cox regression analysis of PDW in association with all-cause and cardiovascular mortality after propensity score matching.
The analysis of RCSs showed that PDW and all-cause mortality were positively and linearly correlated after adjustment of confounders (p for nonlinearly = 0.143) (shown in ). Similarly, in the adjusted RCS analysis, the relationship between PDW and cardiovascular mortality was linear (p for nonlinearly = 0.062). The cardiovascular mortality increased with the rising of PDW (shown in ).
Factors associated with PDW after propensity score matching
Pearson correlation analysis showed that PLT was negatively correlated with PDW (r = −0.108, p = 0.025, ) (shown in ).
Figure 7. Correlation analysis of platelet counts and PDW after propensity score matching. PDW: platelet distribution width.
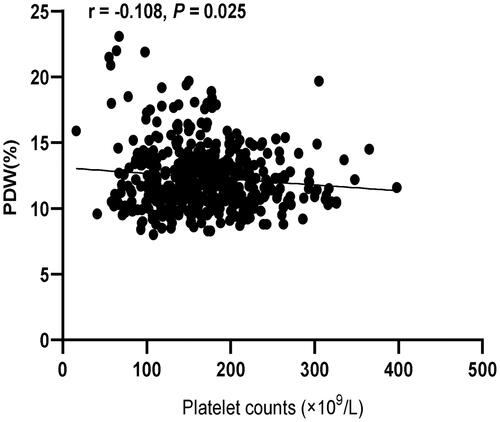
Table 4. Pearson correlation analysis of factors associated with PDW after propensity score matching.
Discussion
In this study, we have observed that higher PDW was independently associated with a high risk of all-cause and cardiovascular mortality. The adjusted RCS analysis showed that the relationship of PDW with all-cause and cardiovascular mortality was linear. We also found that PLT was negatively correlated with PDW. Thus, PDW may be a useful prognostic biomarker for PD patients. In addition, the major cause of end-stage renal disease was chronic glomerulonephritis, accounting for 77.1% of the PD patients, followed by diabetic nephropathy, accounting for 9.2% of the PD patients. This may be different from the distribution of dialysis cases in many studies, especially the low prevalence of diabetic nephropathy. The reason may be that the overall age of the patients in our cohort was relatively young, with an average age of 45 ± 14 years.
As the most common complication, CVD is currently considered to be the main cause of death in patients with chronic kidney disease (CKD) and PD [Citation3,Citation4]. Recent studies have suggested that inflammation and thrombosis play a critical role in the occurrence of CVD in CKD patients [Citation7,Citation12]. Platelets are not only a key component in regulating hemostasis and thrombosis, but also related to inflammation [Citation12]. PDW is a specific marker reflecting platelet activity [Citation6]. It was shown that a large PDW value indicated a large reticulated platelet volume and high metabolic activity [Citation5]. A high platelet activity means that the platelets can strongly promote inflammation and coagulation that are closely related to CVD [Citation13]. High PDW has been demonstrated to be associated with atherosclerosis, coronary artery disease, cerebrovascular disease, and systemic inflammatory disease, and it can be an independent predictor of poor prognosis in patients with heart failure [Citation14]. A retrospective cohort study of 1557 PD patients revealed that PDW > 13.2 fL was associated with the incidence of new-onset CVD in PD patients [Citation12]. In one study of HD patients, it was shown that PDW ≥ 16.31% was independently associated with a high risk of all-cause and cardiovascular deaths [Citation5]. Yu et al. [Citation4] observed that PLT and PDW were independent risk factors of CVD events in non-dialysis CKD patients. Our findings were similar to that in these studies. Peng et al. [Citation7] found that high PLT and platelet crit, but not PDW, were strongly associated with high risk of CVD mortality in PD patients. This result was inconsistent with our study, likely because it did not use PSM to correct the data. Interestingly, PDW could also be a reliable biomarker for the clinical evaluation of systemic lupus erythematosus (SLE). Chen et al. [Citation15] suggested that individuals with PDW ≥ 11.85% had an increased risk of SLE, and the SLE patients with PDW ≥ 13.65% were more likely to be at an active stage. Besides, the elevation of PDW level has been linked to a poor prognosis in several tumors, such as ovarian cancer, gastric cancer, non-small cell lung cancer, breast cancer, hepatocellular carcinoma, and melanoma [Citation16]. On the other hand, Gao et al. [Citation17] showed that a lower PDW may be related with a poor outcome at 3 months after intravenous thrombolysis in acute ischemic stroke patients. It can be seen that both high and low levels of PDW could have detrimental effects on the body. High PDW levels may be associated with increased platelet activation and a higher risk of thrombotic events. While low PDW levels may be linked to impaired platelet function and increased bleeding tendencies. Therefore, maintaining an optimal balance of PDW levels is crucial for proper platelet function and overall health.
Given that dialysis patients are known to be chronically inflamed, in particular those on PD [Citation5]. We speculate that there may be two potential mechanisms by which elevated PDW increases the risk of death in PD patients. First, an inflammatory response may elevate the levels of cytokines that are secreted by inflammatory cells, and the increased cytokines can stimulate the release of large platelets from megakaryocytes [Citation18]. Large platelets have a high enzyme content and active metabolism. They also contain a large number of particles that can release active substances, such as thromboxane A2 and β-thrombomodulin, which promote platelet adhesion, aggregation, and thrombus formation [Citation18,Citation19]. At this time, the heterogeneity of platelet volume in peripheral blood increases, leading to a rise in PDW. Therefore, the significant increase in PDW indicates an inflammatory response. Second, the elevation of PDW due to thrombosis may also contribute to the death of PD patients. It is known that thrombosis consumes a large number of platelets. When PLT in peripheral blood decrease, the number of megakaryocytes in the bone marrow is increased through a negative feedback in the human body, resulting in many large platelets to replenish the platelets in circulation and an increase in PDW. The increase in the number of large platelets in the circulation can accelerate the formation of thrombus. The mechanism also explains the correlation between PLT and PDW.
Our study has several limitations. This was a single-center retrospective study which could only establish association but not causality between PDW and the mortality. Due to lack of data, we failed to adjust inflammatory indicators and other risk factors related to death, so the impact of residual confounding on the results cannot be excluded. There are several factors that could potentially contribute to all-cause and cardiovascular mortality during the course of PD, such as insufficient urea removal, higher levels of glycated Hb, the use of stronger dextrose PD solutions, and higher blood pressure. However, in our study, we only collected baseline variables and did not assess these factors. Additionally, we did not consider changes in PDW during the follow-up period. Furthermore, we did not ascertain a PDW threshold as a high risk indicator of all-cause and cardiovascular mortality in PD patients.
In conclusion, this study has shown that elevated PDW is associated with the prognosis of PD patients, and the underlying mechanism deserves further study. As a common, easily accessible, and affordable biomarker, PDW may help clinicians identify PD patients with a high risk of death.
Author contributions
Chunyu Lu and Shuiqin Cheng contributed equally to this work; Jinquan Wang conceived and designed the study; Chunyu Lu and Shuiqin Cheng analyzed the data and wrote the article; Wenjing Fan and Zhihong Zhang collected the data; Jinquan Wang reviewed and edited the manuscript.
Ethical approval
This study was approved by the Ethics Committee of Jinling Hospital [No.2023DZKY-003-01] and was in accordance with the Helsinki Declaration of 1975. The informed consent was waived due to the retrospective study design.
Acknowledgment
The authors thank all members of the National Clinical Research Center of Kidney Disease, for their helpful advice and discussions.
Disclosure statement
The authors have no conflicts of interest to declare.
Data availability statement
The datasets in this study are available from the corresponding author on reasonable request.
Additional information
Funding
References
- Zhang J, Lu X, Li H, et al. Risk factors for mortality in patients undergoing peritoneal dialysis: a systematic review and meta-analysis. Ren Fail. 2021;43(1):1–7. doi:10.1080/0886022X.2021.1918558.
- Shi Y, Cai J, Shi C, et al. Low serum albumin is associated with poor prognosis in patients receiving peritoneal dialysis treatment. J Healthc Eng. 2022;2022:7660806–7660806. doi:10.1155/2022/7660806.
- Tang M, Fan JX, Fang JG, et al. Risk factors of cardiac complications in patients with end-stage renal disease undergoing maintenance peritoneal dialysis. Ann Palliat Med. 2022;11(7):2196–2201. doi:10.21037/apm-21-2987.
- Yu Z, Xiong J, Yang K, et al. The association between platelet indices and cardiovascular events in chronic kidney disease patients without dialysis. Int Urol Nephrol. 2021;53(5):961–971. doi:10.1007/s11255-020-02696-4.
- Ruiyan W, Bin X, Jianhua D, et al. Platelet distribution width and mortality in hemodialysis patients. Evid Based Complement Alternat Med. 2021;2021:6633845–6633846. doi:10.1155/2021/6633845.
- Vagdatli E, Gounari E, Lazaridou E, et al. Platelet distribution width: a simple, practical and specific marker of activation of coagulation. Hippokratia. 2010;14(1):28–32.
- Peng F, Li Z, Yi C, et al. Platelet index levels and cardiovascular mortality in incident peritoneal dialysis patients: a cohort study. Platelets. 2017;28(6):576–584. doi:10.1080/09537104.2016.1246716.
- Mad P, Felder-Puig R, Gartlehner G. Randomisiert kontrollierte studien [randomised controlled trials]. Wien Med Wochenschr. 2008;158(7–8):234–239. doi:10.1007/s10354-008-0526-y.
- Badhiwala JH, Karmur BS, Wilson JR. Propensity score matching: a powerful tool for analyzing observational nonrandomized data. Clin Spine Surg. 2021;34(1):22–24. doi:10.1097/BSD.0000000000001055.
- Dekkers IA, van der Molen AJ. Propensity score matching as a substitute for randomized controlled trials on acute kidney injury after contrast media administration: a systematic review. AJR Am J Roentgenol. 2018;211(4):822–826. doi:10.2214/AJR.17.19499.
- Yu J, Lin T, Huang N, et al. Plasma fibrinogen and mortality in patients undergoing peritoneal dialysis: a prospective cohort study. BMC Nephrol. 2020;21(1):349. doi:10.1186/s12882-020-01984-6.
- Su N, Tang X, Zhan X, et al. The relationship between platelet distribution width and new-onset cardiovascular disease events in patients with peritoneal dialysis. Ren Fail. 2022;44(1):1640–1648.
- Jennings LK. Mechanisms of platelet activation: need for new strategies to protect against platelet-mediated atherothrombosis. Thromb Haemost. 2009;102(2):248–257. doi:10.1160/TH09-03-0192.
- Sato Y, Yoshihisa A, Watanabe K, et al. Association between platelet distribution width and prognosis in patients with heart failure. PLoS One. 2020;15(12):e0244608. doi:10.1371/journal.pone.0244608.
- Chen SY, Du J, Lu XN, et al. Platelet distribution width as a novel indicator of disease activity in systemic lupus erythematosus. J Res Med Sci. 2018;23:48. doi:10.4103/jrms.JRMS_1038_16.
- Qin L, Li JY, Huang WJ, et al. Higher platelet distribution width is associated with unfavorable prognosis in ovarian cancer. Cancer Biomark. 2020;28(3):365–370. doi:10.3233/CBM-191190.
- Gao F, Chen C, Lyu J, et al. Association between platelet distribution width and poor outcome of acute ischemic stroke after intravenous thrombolysis. Neuropsychiatr Dis Treat. 2018;14:2233–2239. doi:10.2147/NDT.S170823.
- Zheng YY, Wang L, Shi Q. Mean platelet volume (MPV) and platelet distribution width (PDW) predict clinical outcome of acute ischemic stroke: a systematic review and meta-analysis. J Clin Neurosci. 2022;101:221–227. doi:10.1016/j.jocn.2022.05.019.
- Verdoia M, Barbieri L, Schaffer A, et al. Platelet distribution width and the risk of periprocedural myocardial infarction in patients undergoing percutaneous coronary intervention. J Thromb Thrombolysis. 2014;37(3):345–352. doi:10.1007/s11239-013-0954-4.