Abstract
Background
The high prevalence of mild cognitive impairment (MCI) in non-dialysis individuals with chronic kidney disease (CKD) impacts their prognosis and quality of life.
Objective
This study aims to investigate the variables associated with MCI in non-dialysis outpatient patients with CKD and to construct and verify a nomogram prediction model.
Methods
416 participants selected from two hospitals in Chengdu, between January 2023 and June 2023. They were categorized into two groups: the MCI group (n = 210) and the non-MCI (n = 206). Univariate and multivariate binary logistic regression analyses were employed to identify independent influences (candidate predictor variables). Subsequently, regression models was constructed, and a nomogram was drawn. The restricted cubic spline diagram was drawn to further analyze the relationship between the continuous numerical variables and MCI. Internally validated using a bootstrap resampling procedure.
Results
Among 416 patients, 210 (50.9%) had MCI. Logistic regression analysis revealed that age, educational level, occupational status, use of smartphones, sleep disorder, and hemoglobin were independent influencing factors of MCI (all p<.05). The model’s area under the curve was 0.926,95% CI (0.902, 0.951), which was a good discriminatory measure; the Calibration curve, the Hosmer–Lemeshow test, and the Clinical Decision Curve suggested that the model had good calibration and clinical benefit. Internal validation results showed the consistency index was 0.926, 95%CI (0.925, 0.927).
Conclusion
The nomogram prediction model demonstrates good performance and can be used for early screening and prediction of MCI in non-dialysis patients with CKD. It provides valuable reference for medical staff to formulate corresponding intervention strategies.
1. Introduction
Chronic kidney disease (CKD) has emerged as a substantial global quandary in the realm of public health, presenting a formidable peril to both the physical and psychological well-being of humanity, and by extension, their holistic quality of existence. As CKD advances, afflicted individuals frequently grapple with the concomitant manifestation of cognitive dysfunction, with mild cognitive impairment (MCI) standing as the predominant expression, exhibiting an incidence ranging from 54.7% to 62.5% [Citation1,Citation2]. This not only affects the prognosis and quality of life of patients but also increases the economic and care burden on families and society. Given that MCI is a transitional state between normal aging and dementia, it represents an important ‘window of intervention’ for the prevention and treatment of dementia [Citation3]. Therefore, it is of great significance to study the variables associated with MCI risk in CKD patients and construct risk prediction models. However, few studies have been conducted on the risk prediction model of MCI in non-dialysis patients with CKD in outpatient settings. Based on this, this study intends to construct a nomogram risk prediction model for MCI in outpatient CKD non-dialysis patients and visualize it, with the aim of providing a convenient and effective assessment tool for the early identification, prevention, and intervention of cognitive function in this population.
2. Objects and methods
2.1. Study population and study design
This study is designed as a cross-sectional investigation, employing the convenience sampling approach for the recruitment of non-dialysis CKD patients under the care of the nephrology department in two tertiary healthcare facilities located in Chengdu City. The data collection period spanned from January 2023 to June 2023. Inclusion criteria: (1) Patients afflicted by CKD who conform to the diagnostic standards set forth by the Kidney Disease Outcome Quality Initiative (K/DOQI): demonstrating signs of renal impairment (such as hematuria, proteinuria, or radiological anomalies) or a glomerular filtration rate below 60 mL/min/1.73 m2, with an anomaly within any of these parameters persisting for a minimum of three months; (2) Aged ≥18 years (3) Exhibiting the capability for conventional communication and granting informed consent. Exclusion criteria: (1) Individuals afflicted by severe states of consciousness disorders or those unable to collaborate due to a grave medical condition; (2) Primary hyper- or hypothyroidism; (3) Those consuming antipsychotic or sedative-hypnotic medications within the preceding month; (4) Individuals grappling with uremic encephalopathy; (5) Those having received a diagnosis of dementia or afflicted by neurological conditions concomitant with cognitive debilitation; (6) Individuals suffering from visual and auditory impediments that precluded their successful test completion. Following the methodology for ascertaining sample size within the multifactorial logistic regression model [Citation4], and informed by a comprehensive literature review, a total of 33 distinct independent variables were encompassed, necessitating 5 to 10 patients per each autonomous variable. Given the documented prevalence of MCI among non-dialysis CKD patients at approximately 62.5% [Citation2], and factoring in a 20% margin for potential sample invalidity, the minimal requisite sample size for the model development in this study was calculated as follows: 33 × 5 ÷ 62.5% ÷ (1 − 0.2) = 330 cases. The actual sample size incorporated in this investigation comprised 416 cases, thus fulfilling the stipulated minimum sample size criteria. Ethical approval for this study was obtained from the Ethics Department of Chengdu Medical College (CMCEC2023.NO.77), adhering rigorously to the tenets enshrined within the Helsinki Declaration. All participating subjects provided informed consent.
2.2. Study tools
2.2.1. General demographic information questionnaire
This questionnaire includes age, gender, education level, occupational status, Spouse, exercise, reading books and newspapers, chess and card activities, meat and vegetable combinations, and use of smartphones.
2.2.2. Disease-related information questionnaire
This questionnaire includes primary disease, CKD stage, hypertension, diabetes, coronary heart disease, as well as laboratory examination indexes, including: total protein, hemoglobin (Hb), albumin (Alb), triglyceride (TG), total cholesterol (TC), high-density lipoprotein (HDL), low-density lipoprotein (LDL), urea (Urea), estimation of glomerular filtration rate (eGFR), Uric acid (UA), Cystatin C (Cys C), Potassium (K), Calcium (CA), Sodium (NA), Phosphorus (P), White blood cells (WBC).
2.2.3. Chinese version of the Montreal Cognitive Assessment (MoCA) scale
This scale is used to assess patients’ cognitive function. It was compiled by Nasreddine et al. in 2004, specifically designed for MCI, and has been widely used in China and internationally. It includes 11 examination items in 8 cognitive domains, with a total score of 30. The presence of MCI is judged by a score <26. If the subject’s literacy level is <12 years, one point will be added to the total score. It has been proven to have good reliability and validity in CKD patients, with a Cronbach’s alpha coefficient of 0.818 [Citation5].
2.2.4. Chinese version of the Mini-Mental State Examination (MMSE) scale
This scale is used to exclude patients suspected of having dementia. It was compiled by Folstein et al. in 1975, with a total of 30 sub-items, mainly used to make a simple assessment of functions such as orientation, memory, attention and computation, language, and graphic simulation. The total score ranges from 0 to 30, and cognitive dysfunction is considered to exist if a score <27 is obtained. The diagnostic criteria for dementia are as follows: illiterate <17 points, elementary school level <20 points, secondary school level <22 points, and university level <23 points. The Cronbach’s alpha coefficient of this scale is 0.891 [Citation6].
2.2.5. Chinese version of the Self-Rating Depression Scale (SDS)
This scale is used to assess the depression status of patients and was compiled by Zung in 1965. It consists of 20 items, with 10 of them reverse-scored, and uses a four-point scale to rate the frequency of symptoms. Depression severity is calculated as the cumulative score for each item divided by 80, with scores categorized as follows: <0.5 as no depression; 0.5 to 0.59 as mild depression; 0.6 to 0.69 as moderate depression; and >0.7 as severe depression. The Chinese version has demonstrated good reliability and validity, with a Cronbach’s alpha coefficient of 0.94 [Citation7].
2.2.6. Pittsburgh Sleep Quality Index (PSQI)
This scale is used to assess patients’ sleep and was compiled by Buysse et al. in 1989. It includes 7 scoring components (18 items), with a total score ranging from 0 to 21. Scores between 8 and 11 indicate mild sleep disorders, scores between 12 and 16 indicate moderate sleep disorders, and scores >17 indicate severe sleep disorders. The Chinese version has been proven to have good reliability and validity in existing studies [Citation8].
2.3. Data collection methods
In this study, the variables to be investigated were determined through a literature review, subject group discussions, and expert consultations. Subsequently, a questionnaire for collecting general demographic information and disease-related data was developed. (1) Demographic data, depression, and sleep-related information were collected using questionnaires. Study participants were provided with a unified instruction explaining the purpose, content, and filling out method of the questionnaires, and the questionnaires were distributed after obtaining informed consent. In principle, participants filled out the questionnaires independently. For individuals unable to complete the questionnaire due to illness, the researcher provided assistance while asking for the exact answers to each entry. During the process, any questions that arose were clarified using the same language to ensure data homogeneity. After collecting the questionnaires, we conducted a thorough check for any omissions or errors in filling them out, which were corrected promptly. (2) Disease-related information was extracted from outpatient medical records and the hospital Health Information System (HIS). The data were then organized by two team members to ensure accuracy. (3) The diagnosis of MCI was based on the diagnostic criteria outlined in the 2018 China Dementia and Cognitive Impairment Diagnosis and Treatment Guidelines (V) [Citation6]. The criteria included: (1) Subjective report of memory loss, preferably supported by information from a knowledgeable person; (2) Demonstration of impairment of memory function or other cognitive functions in an objective test that is not in accordance with the individual’s age and level of education; (3) Overall cognitive functioning is relatively intact; (4) Functions of daily life are not affected; (5) does not meet the diagnostic criteria for dementia. Cognitive function tests were performed using the MMSE and MoCA. The MMSE was used to exclude patients with dementia, and the MoCA was used to differentiate between normal cognitive function and MCI. Two members of the investigation team were trained by neurologists in the use of MoCA and MMSE before the study was conducted, and the assessment and diagnosis of cognitive function in the later stages were completed jointly by these two members.
2.4. Statistical analysis
Microsoft Excel was employed for data compilation, entry, and the implementation of double-entry validation procedures. Subsequently, statistical analysis was performed using SPSS 26.0 (IBM Corp., Armonk, NY, USA) and R version 4.2.3 (https://cran.r-project.org). Initially, data organization was executed via Microsoft Excel, while the identification of missing values was accomplished through the ‘Start-Search-Location Criteria-Null Value’ procedure. Variables with missing data representing less than 10% of the dataset were imputed with the mean value, whereas variables with missing data exceeding 10% were omitted from the analytical framework, given their classification as laboratory indicators for nonstandard assessments with constrained utility in predictive modeling. In alignment with medical expertise and empirical insight, a determination regarding the presence of outliers in the dataset was made. In cases where outliers were identified, the decision to exclude the corresponding sample data was rendered.
2.4.1. Using SPSS26.0 to screen the variables associated with MCI risk
Initially, the normality of the measurements was assessed employing the Kolmogorov-Smirnov test. Measurements demonstrating adherence to a normal distribution were delineated using means ± standard deviation (SD), whereas non-normally distributed measurements were characterized via median and interquartile ranges(IQR). Categorical data were represented by frequency (constitutive ratio). Subsequently, the data was stratified based on the presence of MCI, and the comparative analysis of measurement data was executed through the utilization of the two-sample independent t-test or the Mann-Whitney U rank sum test. Similarly, comparisons of categorical data were undertaken using the χ2 test. Variables displaying a significance level of p < .05 in the inter-group comparison were subjected to univariate binary logistic regression analysis. Variables achieving a p < .05 threshold in the univariate binary logistic regression analysis were subsequently incorporated into the multivariate binary logistic regression analysis, aimed at identifying the independent variables on MCI within the cohort of non-dialysis outpatients afflicted with CKD. This process culminated in the compilation of candidate predictors essential for the predictive model. And collinearity test was performed on the candidate variables.
2.4.2. Use R4.2.3 to build a prediction model and draw a nomogram
Conduct a multivariate regression analysis on the variables selected through the multivariate binary logistic regression analysis, utilizing the rms package of R version 4.2.3. Subsequently, derive the mathematical computational formula of the predictive model based on the β coefficients of each influential factor, thus establishing the preliminary risk prediction model. Employing a restricted cubic spline diagram, proceed to scrutinize the connection between the continuous numerical variables encompassed within the model and the manifestation of MCI. The significance level of this investigation is set at α = 0.05, and statistical significance is confirmed for values where p < .05. On this foundation, a nomogram is created to render the model visually.
2.4.3. Model performance assessment and internal verification
The internal validation of the predictive model was executed through a 1000-fold bootstrap resampling procedure. The Receiver Operating Characteristic curve’s area under the curve (AUC) was employed for appraising the model’s discriminative capacity. The model’s predictive performance was assessed through a comprehensive array of metrics including sensitivity, specificity, Youden’s index, accuracy, positive predictive value (PPV), negative predictive value (NPV), F-score, positive likelihood ratio (LR+), and negative likelihood ratio (LR−). Furthermore, the calibration of the model was gauged through the utilization of a calibration curve. Decision Curve Analysis (DCA) was employed to ascertain the net income across various threshold probabilities, thus rendering an evaluation of the model’s clinical utility.
3. Results
3.1. Recruitment process and general clinical characteristics of non-dialysis outpatient patients with CKD
Throughout the investigative phase, a total of 469 non-dialysis CKD patients were enlisted from the Nephrology Clinic of two affiliated institutions under Chengdu Medical College. From this pool, 36 individuals who failed to meet the established criteria were excluded. An additional 4 patients did not fulfill the questionnaire survey, while 13 individuals exhibited notable gaps in crucial disease-related data. Ultimately, the analysis encompassed 416 patients, as delineated in the recruitment process diagram depicted in .
illustrates the clinical characteristics of the 416 patients ultimately included in the cohort and conducts a comparative analysis of the disparities between groups based on the presence or absence of MCI. The results showed that there were significant differences between the two groups in age, sex, education level, occupational status, spouse, CKD stage, exercise, hypertension, diabetes, coronary heart disease, meat and vegetable combinations, reading books and newspapers, chess and card activities, use of smartphones, primary disease, depression, sleep disorders, Alb, TC, LDL, eGFR, Urea, CysC, and Hb.
Table 1. Clinical characteristics of non-dialysis outpatient patients with CKD with or without MCI.
3.2. Results of cognitive function survey in non-dialysis outpatient patients with CKD
MCI occurred in 210 of the 416 patients (50.9%). The total score and the scores of each dimension in the MCI group were significantly lower than those in the non-MCI group (p < .05), with the biggest differences observed in the dimensions of visuospatial/executive function and attention. Detailed score comparisons are presented in .
Table 2. Comparison of total MoCA score and scores of each dimension in non-dialysis outpatient patients with CKD [M (P25, P75)].
3.3. Univariate binary logistic regression analysis
The research participants were categorized based on the presence or absence of MCI, denoted as (0= no, 1= yes), for the purpose of conducting univariate binary logistic regression analysis. The variables that exhibited a significance level of p < .05 in the cross-sectional comparison between the two cohorts were utilized as independent factors in the univariate binary logistic regression analysis. The findings revealed statistically meaningful distinctions in a range of factors, including age, sex, spouse, education level, occupational status, exercise, depression, sleep disorders, hypertension, diabetes, coronary heart disease, meat and vegetable combinations, reading books and newspapers, chess and card activities, use of smartphone, Alb, TC, LDL, Urea, Hb, eGFR, CKD stages and primary diseases (p < .05). Refer to for a detailed breakdown.
Table 3. Univariate binary logistic regression analyses of MCI in non-dialysis outpatient patients with CKD.
3.4. Multivariate binary logistic regression analysis
The study participants were stratified based on the presence or absence of MCI, categorized as (0= no, 1= yes), for the purpose of conducting multivariate binary logistic regression analysis. Of the 22 variables exhibiting a significance level of p < .05 in the univariate binary logistic regression analysis, they were employed as independent factors (Because CKD stage is converted from eGFR and has similar clinical significance, only one of them was included in the multifactorial regression analysis). Subsequently, a multifactorial binary logistic regression analysis was carried out. The outcomes revealed that age, education level, occupational status, sleep disorders, use of smartphone, and Hb were independent variables of MCI in non-dialysis patients with CKD, as delineated in . These six variables were subsequently employed as predictor variables within the prediction model. Then collinearity test showed that there was no collinearity between the six variables, all predictors and covariables had tolerance values greater than 0.10 and variance inflation less than 5.
Table 4. multivariate binary logistic regression analysis of MCI in non-dialysis outpatient patients with CKD.
3.5. Risk prediction model development
The final multifactorial binary logistic regression analysis was conducted with whether MCI occurred as the dependent variable and the six predictor variables screened (age, education level, occupational status, sleep disorders, use of smartphone, Hb) as the independent variables, and the results are shown in . The logistic risk prediction model was constructed based on the β coefficients and constants of the respective variables as follows: Logit(P)=1.079 × age(≥65 years old)−1.405 × educational level(high school/secondary school and above)−1.590 ×occupational status(employed)+0.977 × sleep disorder(yes)−2.348 × use of smartphone(yes)−0.017 × Hb +3.601. where P represents the probability of complication of MCI in non-dialysis patients with CKD, and P = e Logit (P)/(1+ e Logit(P)), e is a natural constant. On this basis, a nomogram was drawn using the rms package utilizing R4.2.3, see . For each independent variable, different values were obtained by vertical lines on the scoring scale at the top of the nomogram to obtain the corresponding scores (default 0–100), and then scores of each variable were summed to obtain the total score, which in turn was obtained by the total score line on the prediction line at the bottom of the column line graph nomogram to obtain the corresponding predicted probability values. In order to facilitate clinical use, this study made a dynamic nomogram using R software packages such as DynNom, and uploaded it to the website to form a network calculator for MCI risk prediction of outpatients with CKD. The website is as follows: https://1yixueliexiantu.shinyapps.io/CKD_MCI_DN/. See for an example.
Figure 3. Web calculator based on dynamic nomogram of MCI in non-dialysis outpatient patients with CKD.
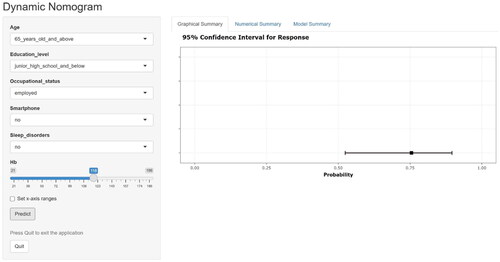
Table 5. The final multivariate binary logistic regression analysis.
3.6. The correlation between hemoglobin and MCI of non-dialysis patients in outpatient department of CKD
We used a four-node restricted cubic spline to explore the association between Hb, which was ultimately included in the model, and MCI in outpatient non-dialysis patients with CKD. As shown in , there was no nonlinear association between Hb and MCI (Total χ2 = 43.73, p < .001; nonlinear χ2 = 3.68, p = .1592). It can be seen that when Hb > 118.6699, the patients’ risk of concurrent MCI varied not very significantly with Hb; when Hb < 118.66991, the patients’ risk of concurrent MCI increased with decreasing Hb.
3.7. Risk prediction model performance assessment and internal validation
Using the original data of the prediction model as the dataset, the ROC curve for predicting MCI in outpatient CKD non-dialysis patients was plotted with an AUC of 0.926, 95% CI (0.902, 0.951), The optimal cutoff value determined by the maximum value of the Youden index (0.699) was 0.678, which divided patients into two groups, namely, the high-risk and low-risk MCI groups. At this threshold, the sensitivity and specificity of the model are 0.767 and 0.932, respectively (). See for the specific predictive performance of the model. Internal validation of the predictive model was performed by 1000 bootstrap resampling procedure, and the calibrated C-index was 0.926 with 95% CI (0.925, 0.927). The calibration curve showed that the calibration curve was approximately distributed along the reference line (y = x) with a mean absolute error of 0.022 (), and the Hosmer–Lemeshow test (p = .624) indicated that the predicted probability of the model fitted well with the actual probability. The results of the DCA curve showed that the model had a net clinical benefit for threshold probabilities ranging from 12.5% to 98% (). It can be seen that the model has good discrimination, calibration and clinical utility.
Table 6. Predictive performance of the model.
4. Discussion
4.1. Current status of MCI complication in outpatient non-dialysis patients with CKD
In this research endeavor, the prevalence of MCI among non-dialysis CKD patients seeking outpatient care was determined to be 50.9%. This figure exceeded the 38.78% documented in the study by Weixia [Citation9] and fell short of the 62.7% reported by Otobe et al. [Citation2]. The disparities in the documented incidence rates may be ascribed to disparities in sample magnitudes and the methodologies applied for assessing cognitive functionality in these investigations. Intriguingly, our outcomes are in concordance with the research undertaken by Yongyi et al. [Citation1], who delineated an incidence rate of 54.7%. These findings collectively signify a notable preponderance of MCI within the cohort of non-dialysis CKD patients, accentuating the suboptimal existing cognitive status within this demographic. Our investigation unveiled heterogeneous degrees of regression across all facets of cognitive acuity, with executive function experiencing the most pronounced deterioration, trailed by attention and deferred memory. Recent studies by Olejnik et al. [Citation10] also show that 8.6% of dialysis patients have no cognitive decline in cognitive screening test (MMSE), but they can find executive function impairment using the Executive Clock-Drawing Task (CLOX), which suggests that the executive dysfunction of CKD patients may appear before the onset of overall cognitive impairment and should be paid attention to as soon as possible. Former investigations have likewise documented diminished volume of frontal gray matter and perturbed amalgamation of the intermediate cerebellar region with prefrontal circuits in CKD patients concurrent with MCI. These anomalies are presumed to underlie impairments in memory and executive functions [Citation11]. Furthermore, CKD patients manifest an elevated susceptibility to cerebrovascular maladies and associated risk elements, including hypertension, diabetes mellitus, and dyslipidemia [Citation12]. These factors may additionally exacerbate the occurrence of cerebral white matter lesions, microinfarctions, microhemorrhages, and cerebrovascular incidents, ultimately resulting in compromises to memory, attention, and executive capabilities. The regression in memory, attention, and executive function, in turn, may impede patients’ aptitude to deliberate and address chronic disease self-management issues [Citation13], thereby exerting an influence on adherence to treatment and impacting the prognosis and clinical outcomes of the disease. Notwithstanding the elevated prevalence of MCI within the cohort of non-dialysis CKD patients, the practical diagnosis of cognitive dysfunction in clinical settings remains notably scarce, at less than 5% [Citation14]. This underscores the insufficiency of healthcare professionals’ vigilance regarding the cognitive prowess of CKD patients. Given that MCI represents an intermediary phase between conventional aging and dementia, one must underscore the imperative to augment scrutiny and evaluation of cognitive acumen among CKD patients. This emphasis carries paramount significance, not only from the vantage point of CKD malady administration but also with regard to averting cognitive impairment in the patient cohort. The primacy of bestowing attention and conducting assessments of cognitive proficiency in patients should be underscored, thereby enhancing their comprehensive well-being and clinical outcomes.
4.2. Analysis of the variables associated with MCI risk in non-dialysis outpatient patients with CKD
4.2.1. Age
The results of this study showed that the risk of MCI in elderly patients aged ≥65 years was 2.941 times higher than that in patients younger than 65 years. Consistent with our findings, Yongyi et al.’s study [Citation1] showed that the risk of MCI in middle-aged and elderly CKD patients was 4.976 times higher than that in young patients. Additionally, Luo et al. [Citation15] and Chien et al. [Citation16] independently confirmed that age is an independent risk factor for MCI, with OR and HR values of 1.09 and 1.07, respectively. The impact of age on cognitive function can be attributed to various factors. Firstly, with age, there is a natural decline in cognitive abilities, which affects patients’ cognitive function [Citation17]. Secondly, CKD patients are more susceptible to cardiovascular disease risk factors as they age, leading to impaired vascular endothelial function and increased occurrence of atherosclerosis. These conditions can result in cerebrovascular lesions, such as microembolism and low perfusion, leading to lacunar cerebral infarcts and cerebral white matter degeneration, which contribute to the occurrence of MCI [Citation18]. In light of these findings, it is crucial for medical personnel to strengthen the early screening of cognitive function in middle-aged and elderly patients during the outpatient follow-up management of CKD patients. Formulating appropriate interventions at this stage can help delay the onset and progression of MCI in patients. Management of traditional risk factors for cognitive impairment should also be emphasized, with a focus on enhanced follow-up and guidance for common cerebrovascular diseases and their risk factors (e.g., hypertension, diabetes mellitus, dyslipidemia) in elderly patients. Additionally, encouraging elderly patients to participate in more educational, family, and social activities can have a positive impact on their cognitive function. Involving family members in the training and management of patients’ cognitive functions can further enhance the effectiveness of cognitive management.
4.2.2. Educational level
The study results demonstrated that the risk of MCI in patients with a high school/secondary education and above was 0.245 times higher than that in patients with junior high school and below, suggesting that higher literacy is a protective factor for cognitive function. This finding aligns with previous studies [Citation1,Citation9,Citation19], and it can be attributed to the fact that highly literate individuals experience slower cognitive function decline. The stimulation and training of brain cells through work and daily life activities, as well as a more abundant neuronal reserve, contribute to this protective effect [Citation18]. However, it is noteworthy that more than 75% of the patients in this study had a low literacy level, placing them at a higher risk of MCI. In light of this, healthcare professionals should prioritize the management of cognitive function in this population. Encouraging patients to engage in activities such as reading books and newspapers, cultivating hobbies (e.g., chess, cards, calligraphy, and painting), and maintaining regular physical exercise can effectively increase brain activity and slow down the progression of MCI. Follow-up studies have also demonstrated that continuing education, like early education, can contribute to increased cognitive reserve and sustained cognitive function [Citation20]. Therefore, in the cognitive management of CKD patients, those with the opportunity and capacity should be encouraged to pursue vocational education and participate in senior universities to bolster their cognitive abilities and preserve cognitive function.
4.2.3. Occupational status
The study results indicated that employed patients had a 0.204 times higher risk of MCI compared to unemployed or retired patients, making occupational status a protective factor for cognitive functioning. This finding is in line with a study by Yuhuan et al. [Citation21]. Additionally, Ruet et al.’s cohort study [Citation22] with a seven-year follow-up revealed that a change in occupational status was significantly associated with cognitive deterioration in patients. Several factors could account for this observation. Firstly, employed patients experience lower economic stress and a reduced level of psychological burden due to their stable jobs and continuous income. Their involvement in work also provides opportunities for engaging in social activities and brain exercises, indirectly preventing and delaying cognitive deterioration. Conversely, retired and unemployed patients may face significant economic and psychological pressure, leading to reduced brain activity and social engagement. The lack of other hobbies and interests could further hasten cognitive decline. In light of these findings, it is essential to encourage unemployed and retired patients, once their condition stabilizes, to consider participating in work or part-time jobs that are compatible with their health status. This approach not only alleviates economic pressure and maintains social activities but also enhances mental health, thus preventing and delaying the onset of MCI.
4.2.4. Use of smartphone
An intriguing finding of this study was that smartphone usage was an independent factor influencing MCI in non-dialysis patients with CKD, with patients using smartphones having a 0.096 times higher risk of MCI compared to those who did not use smartphones. Among the 416 study participants, 126 did not use smartphones, and 118 of them suffered from MCI, resulting in a prevalence rate of 93.6%. In contrast, 290 participants used smartphones, and 92 of them had MCI, resulting in a prevalence rate of 31.7%. This difference was highly significant (χ2 = 134.751, p < .001), corroborating the findings of Wilmer et al. and Shujiao et al. [Citation23,Citation24], which are rarely reported in the field of CKD research. The reasons for this association can be summarized as follows: smartphones offer instant messaging, Internet access, multimedia playback, and multitasking capabilities. On one hand, these features strengthen communication between patients and the outside world, broaden social networks through social software for communication with relatives, friends, and colleagues, reducing the patients’ sense of loneliness and psychological pressure while enhancing their mental health. Additionally, smartphones facilitate communication with healthcare personnel, improving patients’ self-management abilities. On the other hand, smartphones promote reading and thinking by enabling information searches for current news, disease-related recipes, and health management information. Puzzle games and cognitive training available on smartphones also encourage patients to engage their brains more, providing valuable exercise for neurons. It has been suggested that smartphone usage can positively impact the overall cognitive level of the elderly due to exposure to vast amounts of external information, stimulating multidimensional thinking and maintaining a good mental state [Citation25]. Therefore, encouraging and guiding CKD patients to use smartphones appropriately may represent an effective intervention pathway for preventing MCI during follow-up management.
4.2.5. Sleep disorders
In this study, we observed that 51.7% of patients experienced a high incidence of combined sleep disorders. The risk of MCI in patients with comorbid sleep disorders was 2.658 times higher than that in patients without such disorders, making it a significant risk factor for MCI, consistent with the findings of Jingyu et al. [Citation26]. Sleep is one of the most fundamental physiological activities, accounting for one-third of human life. Adequate sleep ensures the rest and cognitive activities of brain nerve cells, promoting essential functions like decision-making, language processing, and information storage [Citation27]. However, sleep deprivation leads to decreased acetylcholine content in the brain, ultimately impairing memory function [Citation28]. Moreover, chronic insomnia reduces monoamine neurotransmitters in the brain, promoting cerebral vascular sclerosis and intracerebral hypoxia, which impairs cognitive functions such as memory, attention, abstract thinking, and orientation [Citation29]. Considering the high prevalence of sleep disorders in the CKD population, enhancing the assessment and screening of sleep quality and providing reasonable intervention guidance may benefit patients’ cognitive function. Strategies such as listening to sleep-aiding music, consuming a small amount of hot milk before bedtime, soaking feet, reading, and including more sleep-improving foods (e.g., pumpkin, lily of the valley, sour jujube) may be beneficial. Additionally, encouraging patients to engage in appropriate exercise after dinner and performing relaxation and stretching exercises before bedtime can also improve sleep quality and enhance the cognitive function of CKD patients.
4.2.6. Hemoglobin
The results of this study showed that hemoglobin was a protective factor for cognitive function with an OR of 0.984(For every 1 g/L increase in hemoglobin, the risk of MCI will decrease by 1.6%.). On the contrary, the lower the Hb, the higher the risk of developing MCI. However, in CKD patients, the impairment of renal function leads to reduced erythropoietin production, resulting in decreased Hb levels and often severe anemia. Low oxygen-carrying capacity of the blood cells and insufficient oxygen supply to brain tissues in these patients make them more susceptible to cognitive impairment [Citation1]. Furthermore, anemia in CKD patients may be accompanied by iron or vitamin B12 deficiency, which can independently lead to cognitive impairment [Citation30]. Additionally, anemia may cause a compensatory increase in cerebral blood flow, leading to an increased delivery of uremic toxins to the brain, exacerbating cognitive impairment. Wei et al. [Citation31] confirmed that cognitive function improved following erythropoietin treatment in patients with renal failure, suggesting that regular follow-up, blood analysis tests, and monitoring of Hb levels are essential in clinical practice. Instruct patients to take medication as prescribed by the doctor, as well as injections of erythropoietin and other treatments, pay close attention to the patient’s Hb up to standard, and actively correct the anemia, so as to improve the supply of cerebral tissues and reduce the cognitive function damage.
4.3. Scientific, intuitive, and practical analysis of the nomogram prediction model
The nomogram prediction model is built on the basis of multifactorial regression analysis, integrating multiple predictors to individualize and accurately predict the probability of an event’s occurrence, serving as a risk prediction tool. In this study, we quantified age, literacy, occupational status, smartphone usage, sleep disorders, and Hb levels to construct a column-line diagram model for predicting the risk of MCI in outpatient CKD non-dialysis patients. The model demonstrated excellent differentiation, calibration, and scientific validity. Moreover, its clinical translation is relatively simple, as the risk probability of MCI occurrence can be directly derived from the model based on the assigned scores for each variable. Healthcare professionals can utilize the paper version of the nomogram prediction model or transform it into a dynamic nomogram set up in a WeChat applet, and make it into a web calculator for use. making it easy to visualize and use intuitively. The model’s clinical utility was assessed through decision curve analysis, which indicated significant additional clinical net benefit at a threshold probability of 14% to 98%, demonstrating its practical value. Cognitive assessment in the clinical milieu conventionally comprises historical inquiry and neuropsychological evaluations, with the latter demonstrating extensive versatility across various maladies but evincing a dearth of specificity. Furthermore, traditional neuropsychological assessments are confined to the evaluation of cognitive functionality, they omit a comprehensive screening for pertinent contributory factors, and their administration necessitates a clinician’s prescription. In contrast to conventional neuropsychological evaluations, this investigation has yielded a nomogram risk prediction model tailored for MCI discernment among ambulatory non-dialysis patients. This model is grounded upon the distinctive context of CKD management, endowing it with a spectrum of advantages: it is explicitly contrived for the realm of CKD, thereby conferring it with enhanced specificity; it not only prognosticates the MCI hazard within CKD patients but also identifies and quantifies precise risk constituents and their relative influence, thereby facilitating the formulation of individualized interventional protocols. Furthermore, this model is characterized by user-friendliness, accessibility, and convenience for healthcare professionals, thereby augmenting the diagnostic and therapeutic proficiency in managing the cognitive well-being of CKD-afflicted patients. In addition, the model incorporates quantitative parameters that can be readily ascertained within the clinical environment, thereby fostering its utility and feasibility in clinical deployment. Consequently, this model stands poised to function as an indispensable screening instrument, empowering healthcare practitioners to evaluate the susceptibility to MCI among ambulatory patients afflicted by CKD, without necessitating dialysis.
4.4. Implications for CKD management
Risk assessment is the primary preventative measure to prevent cognitive impairment. This model can be used to assess the MCI risk in outpatients with CKD without dialysis, which may provide practical reference for grading and managing MCI. First, MCI risk screening should be included in the management of CKD. A risk score of MCI greater than 0.678 suggests a high risk of MCI, and personalized preventive interventions should be promptly conducted to delay or reverse the cognitive impairment process. A risk score near 0.678 also needs attention, and prospective preventive measures should be formulated. Furthermore, the degree of influence for each predictor was visualized by a nomogram, which can increase the utility and generalizability of this model in the clinical setting.
4.5. Study limitations
This study has several limitations that should be acknowledged. Firstly, it is imperative to acknowledge the constrained sample size assimilated within the ambit of this study, and the model has yet to be subjected to external validation through the utilization of an autonomous dataset. A subsequent validation endeavor becomes requisite in order to scrutinize the model’s scope of applicability and its dependability. Secondarily, it is imperative to elucidate that this investigation adopts a cross-sectional design, hence the establishment of causal associations between the variables and mild cognitive impairment might be susceptible to inherent biases. Consequently, it becomes indispensable to undertake additional prospective cohort studies to authenticate and augment the extant discoveries. Furthermore, during the course of this investigation, it emerged from an extensive review of the scholarly literature and consultations with experts that additional variables, including but not limited to 24-h proteinuria, glycosylated hemoglobin, parathyroid hormone, vitamin D, transferrin, and ultrasensitive C-reactive protein, might exert a notable influence on cognitive function. Nevertheless, owing to the omission of testing for these particular indices in a subset of patients, these variables were excluded from the present study. At the same time, the majority of CKD patients inhabit a realm of coexisting maladies, necessitating the routine consumption of a multitude of pharmaceutical agents, such as antihypertensive drugs, hypoglycemic agents, uric acid-lowering medications, hypolipidemic agents, calcium and phosphorus metabolism enhancers, and anemia correction agents. It is plausible that the interplay among these pharmaceuticals may bear relevance to the cognitive performance of patients. Regrettably, due to inherent constraints within the study’s design and the allotted timeframe, data pertaining to this dimension remains conspicuously absent. A prospective investigation can rectify this deficiency by introducing pertinent variables, thereby augmenting the model’s precision.
5. Conclusions
This study highlights the high prevalence of MCI in outpatient CKD non-dialysis patients, warranting increased attention from healthcare professionals. Age, educational level, occupational status, use of smartphone, sleep disorders, and hemoglobin level were identified as independent factors influencing MCI, emphasizing the need for targeted preventive strategies for these factors in patients. Moreover, the nomogram risk prediction model, developed based on these variables, provides a simple, rapid, and effective way to predict the probability of MCI risk in outpatient CKD non-dialysis patients. This model serves as a valuable screening tool for assessing the risk of MCI occurrence in this patient population. Implementing the model in clinical practice can aid in early detection and intervention, thus improving patient outcomes and quality of life.
Ethics statement
This study was approved by the institutional ethics committee for human research of the Chengdu medical college (CMCEC2023.NO.77). This research study was conducted in accordance with the guidelines of the Declaration of Helsinki. All patients have informed consent.
Author contributions
Qin Yang: study design, execution, data collection and analysis, manuscript writing and revision.
Yuhe Xiang: data collection and analysis, manuscript revision assistance.
Guoting Ma: conception, analysis and interpretation.
Min Cao: study design.
Yixi Fang: data collection.
Wenbin Xu and Lin Li: conception, study design.
Li Qin: Recruiting research patients.
Yu Feng: Guidance on professional knowledge of manuscript revision.
Qian Yang: conception, study design, manuscript review and revision.
Disclosure statement
No potential conflict of interest was reported by the author(s).
Additional information
Funding
References
- Yongyi P, Nirong G, Wenhong X, et al. Prevalence and influencing factors of mild cognitive impairment in outpatients with chronic kidney disease. Chin Nurs Manag. 2021;21(08):1–17.
- Otobe Y, Hiraki K, Hotta C, et al. Mild cognitive impairment in older adults with pre-dialysis patients with chronic kidney disease: prevalence and association with physical function. Nephrology. 2019;24(1):50–55. doi: 10.1111/nep.13173.
- Hampel H, Lista S. Dementia: the rising global tide of cognitive impairment. Nat Rev Neurol. 2016;12(3):131–132. doi: 10.1038/nrneurol.2015.250.
- Moons KG, Royston P, Vergouwe Y, et al. Prognosis and prognostic research: what, why, and how? BMJ. 2009;338(1):b375–b375. [doi: 10.1136/bmj.b375.
- Tiffin-Richards FE, Costa AS, Holschbach B, et al. The Montreal Cognitive Assessment (MoCA)-a sensitive screening instrument for detecting cognitive impairment in chronic hemodialysis patients. PLoS One. 2014;9(10):e106700. doi: 10.1371/journal.pone.0106700.
- Writing Group of China’s Guidelines for the Diagnosis and Treatment of Dementia and Cognitive Impairment, Professional Committee of Cognitive Impairment, Neurophysician Branch of Chinese Medical Association. 2018 China’s guidelines for the diagnosis and treatment of dementia and cognitive impairment (V): diagnosis and treatment of mild cognitive impairment. Chin Med J. 2018;98(17):1294–1301.
- Leu SH, Chou JY, Lee PC, et al. Validity and reliability of the Chinese version of the Sheehan Disability Scale (SDS-C). Asia Pac Psychiatry. 2015;7(2):215–222. doi: 10.1111/appy.12182.
- Taoying L, Yan L, Ping X, et al. Reliability and validity analysis of Pittsburgh Sleep Quality Index. Chongqing Med. 2014;43(03):260–263.
- Weixiao Z. Correlation between cognitive dysfunction and TCM syndrome types of CKD patients in stage 3-5 based on kidney-brain correlation research [master’s thesis]. Liaoning University of Traditional Chinese Medicine; 2021.
- Olejnik P, Golenia A, Maciejewska O, et al. The clock drawing task reveals executive dysfunction in the dialysis population - an underrecognized and underestimated problem. Ren Fail. 2024;46(1):2306232. doi: 10.1080/0886022X.2024.2306232.
- Shuyuan L. Clinical study of mild cognitive impairment in maintenance hemodialysis patients [master’s thesis]. Nanjing Medical University; 2016.
- Drew DA, Weiner DE, Sarnak MJ. Cognitive impairment in CKD: pathophysiology, management, and prevention. Am J Kidney Dis. 2019;74(6):782–790. doi: 10.1053/j.ajkd.2019.05.017.
- Ku Z, Lou R, Moore SF, et al. Stroke and dementia risk: a systematic review and meta-analysis. Alzheimers Dement. 2018;14(11):1416–1426. doi: 10.1016/j.jalz.2018.06.3061.
- Wang F, Zhang L, Liu L, et al. Level of kidney function correlates with cognitive decline. Am J Nephrol. 2010;32(2):117–121. doi: 10.1159/000315618.
- Luo Y, Murray AM, Guo YD, et al. Cognitive impairment and associated risk factors in older adult hemodialysis patients: a cross-sectional survey. Sci Rep. 2020;10(1):12542. doi: 10.1038/s41598-020-69482-1.
- Chien CW, Lin YC, Huang SK, et al. A population-based study of the association between hemodialysis and cognitive impairment. Asia Pac Psychiatry. 2020;12(4):e12404.
- Harada CN, Natelson Love MC, Triebel KL. Normal cognitive aging. Clin Geriatr Med. 2013;29(4):737–752. doi: 10.1016/j.cger.2013.07.002.
- Wang X. Clinical study of mild cognitive impairment in maintenance hemodialysis patients [master’s thesis]. Shihezi University; 2018.
- Siyang W. Cognitive dysfunction in patients with end-stage renal disease and its related factors [master’s thesis]. Anhui Medical University; 2022.
- Thow ME, Summers MJ, Saunders NL, et al. Further education improves cognitive reserve and triggers improvement in selective cognitive functions in older adults: the Tasmanian Healthy Brain Project. Alzheimers Dement. 2018;10(1):22–30. doi: 10.1016/j.dadm.2017.08.004.
- Yuhuan Y, Guangwen C, Shuang R, et al. Prevalence survey of mild cognitive impairment among the elderly in Huangshi community. Chin J Dis Control. 2017;21(08):767–771.
- Ruet A, Deloire M, Hamel D, et al. Cognitive impairment, health-related quality of life and vocational status at early stages of multiple sclerosis: a 7-year longitudinal study. J Neurol. 2013;260(3):776–784. doi: 10.1007/s00415-012-6705-1.
- Wilmer HH, Sherman LE, Chein JM. Smartphones and cognition: a review of research exploring the links between mobile technology habits and cognitive functioning. Front Psychol. 2017;8:605. doi: 10.3389/fpsyg.2017.00605.
- Shujiao L, Lu L, Huiling L, et al. Study on the correlation between the elderly’s hobbies and the use of smart products and their cognitive function. Chin J Pract Nurs. 2018;34(6):407–411.
- Manqiong Y, Xingliang W, Jia C, et al. Prevalence and influencing factors of mild cognitive impairment among the elderly in Xiamen. China Public Health. 2021;37(01):15–18.
- Jingyu L, Lianzhao Y, Ling C, et al. Construction and validation of risk prediction model of mild cognitive impairment in elderly hypertensive patients in community. J Nurs. 2021;28(24):42–50.
- Wu CR, Chen PY, Hsieh SH, et al. Sleep mediates the relationship between depression and cognitive impairment in older men. Am J Mens Health. 2019;13(1):1557988319825765. doi: 10.1177/1557988319825765.
- Zucheng L, Zechun K, Shucui L, et al. Effects of caffeine on learning and memory, antioxidant capacity and cholinergic system in hippocampus of sleep-deprived aged mice. Chin J Gerontol. 2016;36(11):2583–2585.
- Qiuyun Y, Xia J, Panpan H, et al. Research progress on neurogenesis of Alzheimer’s disease and sleep disorder. Chin J Tradit Chin Med. 2017;32(10):4562–4565.
- Yuzan Q, Ke Z, Xuemei L. Research status and progress of cognitive function in patients with chronic kidney disease. Chin J Blood Purif. 2019;18(07):495–498.
- Wei D, Kewen X, Shiwen X. Effect of rHuEPO on the cognitive function of uremic maintenance hemodialysis patients. Jiangxi Med. 2005;40(09):507–509.