Renal dysfunction is a prevalent condition affecting millions of individuals globally [Citation1–3]. The diagnosis and treatment of this condition pose significant challenges due to its intricate nature and diverse manifestations [Citation4–6]. In recent years, the field has witnessed remarkable progress in machine learning (ML) and artificial intelligence (AI), leading to profound impacts across various industries. Particularly in the medical domain, ML and AI have undergone significant development, emerging as powerful tools [Citation7,Citation8]. These advancements have opened up new avenues for exploring both the diagnosis and treatment of renal dysfunction [Citation9,Citation10]. Recognizing the transformative potential of ML and AI in addressing the complexities of renal dysfunction, we have curated this special issue to delve into the applications of machine learning and artificial intelligence in the context of patients with renal dysfunction. ()
Figure 1. An Overview of the Applications of Artificial Intelligence in Renal Dysfunction. EMR: electronic medical record; ECG: electrocardiogram; rs-fMRI: resting-state functional magnetic resonance imaging; AUC: the area under the receiver operating characteristic curve; AI: artificial intelligence; ML: machine learning; SHAP: SHapley Additive exPlanations; MHD-CI: maintenance hemodialysis patients with cognitive impairment; CI-AKI: contrast-induced acute kidney injury; NLR: Neutrophil-to-lymphocyte ratio; AKD: acute kidney disease; CKD: chronic kidney disease; FR: furosemide-responsive; FU: furosemide-unresponsive.
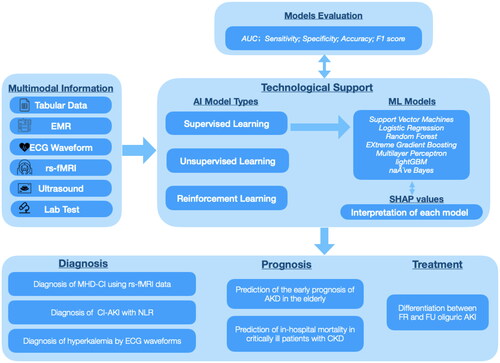
First, in the realm of renal dysfunction diagnosis, this special issue undertakes a comprehensive exploration of artificial intelligence applications, encompassing diverse facets such as predicting cognitive impairment (CI) in maintenance hemodialysis patients (MHD), exploring the correlation between neutrophil-to-lymphocyte ratio (NLR) and contrast-induced acute kidney injury (CI-AKI), making rapid predictions of different degrees of hyperkalemia by analyzing the ECG, screening the related factors affecting new-onset AKI in the ICU, assessing renal fibrosis in CKD patients and so on. The article by Zhou et al. [Citation11]. included 2230 patients who underwent elective vascular intervention, coronary angiography and percutaneous coronary intervention and divided them into a CI-AKI group and a non-CI-AKI group. Utilizing NLR and other pertinent high-risk factors, the researchers established logistic regression (LR), random forest (RF), gradient boosting decision tree (GBDT), extreme gradient boosting (XGBoost), and naïve Bayes (NB) models. The results showed a high NLR was an independent risk factor for CI-AKI. The area under the ROC curve (AUC) of the NB model was the largest, indicating that it had the best performance. This study reaffirmed that ML models is capable of handling complex relationships among numerous variables in real-world big data, and the predictive performance has been demonstrated superior to that of traditional statistical models for predicting AKI. In particular, Sun’s study innovatively applied the field of radiomics, it recruited 55 MHD patients with CI and 28 healthy controls, aimed to probe the relationship between spontaneous brain activity and CI by using resting-state functional magnetic resonance imaging (rs-fMRI) data. The result shows that compared with the MHD-NCI group, the patients with MHD-CI had more severe anemia and higher urea nitrogen levels, lower mALFF values in the left postcentral gyrus, lower mfALFF values in the left inferior temporal gyrus, and greater mALFF values in the right caudate nucleus. Furthermore, back propagation neural network (BPNN) was utilized to predict cognitive function. It indicated that the diagnostic efficacy of the model which inputs were hemoglobin, urea nitrogen, and mALFF value in the left central posterior gyrus was optimal. The study by Xu et al. [Citation12] is centered on the noninvasive and swift prediction of hyperkalemia, accomplished through the analysis of distinct waveforms on the electrocardiogram (ECG) using machine learning methodologies. The team exerted strenuous efforts in the processing of ECG signals, facilitating their quantification and identification through machine learning. Ultimately, a total of 48 features were extracted for the purpose of constructing the model. They also constructed different machine models and employed the Area Under the Curve (AUC) to evaluate the performance of each model. The results showed XGB had a higher AUC in mild hyperkalemia, but SVM performed better in predicting more severe hyperkalemia.
Patients with renal dysfunction typically face a poor prognosis [Citation13,Citation14], a matter of significant clinical concern addressed in this special issue [Citation15,Citation16]. Li et al. contributed an article that involved collecting a substantial amount of noninvasive parameters from the MIMIC-IV database. They extracted 33 such parameters as features for modeling, utilizing five supervised learning algorithms to predict categorical labels and enhancing model interpretability through SHAP. The best-performing model, validated externally using eICU-CRD, was selected for further development. They deployed a simplified online application incorporating the top 10 features from importance ranking. This web-based tool predicts the early prognosis of Acute Kidney Dysfunction (AKD) in the elderly based on readily available noninvasive parameters. Additionally, Li et al. [Citation17] successfully developed and validated machine learning models for predicting mortality in critically ill patients with Chronic Kidney Disease (CKD). Employing six machine learning approaches, the XGBoost model, with the highest AUC, stood out. SHAP values were used for interpretation, revealing that the SOFA score, urine output, respiratory rate, and SAPS II were the four most influential variables in the XGBoost model. These studies provide valuable insights for clinicians, facilitating accurate management and early interventions to potentially reduce mortality in high-risk patients [Citation16].
The application of machine learning (ML) and artificial intelligence (AI) in directly guiding clinical treatment is still not widespread adopted [Citation18]. Establishing an appropriate machine learning model and ensuring its explainability can empower clinicians to gain a deeper understanding of the rationale behind prediction outcomes, facilitating the implementation of individualized treatment approaches [Citation19]. The study of Jiang et al. aimed to develop an explainable ML model to differentiate between furosemide-responsive (FR) and furosemide-unresponsive (FU) oliguric AKI. The study features a significant sample size, encompassing 6897 patients from the MIMIC-IV database for the training cohort, and an additional 2235 patients from the eICU-CRD database for the validation cohort. The results indicated that XGBoost achieved the highest AUC, suggesting optimal model performance and implying practical guidance in determining the application of furosemide in clinically oliguric AKI patients. This paves the way for future applications of machine learning in clinical treatment decision-making.
Overall, this special issue presents cutting-edge research and insights on the intersection of machine learning, and multi-omics data analysis in the diagnosis, prognosis and treatment of renal dysfunction [Citation20]. As previously mentioned, we delved into employing deep learning algorithms for pattern identification within extensive, high-dimensional datasets acquired from diverse sources, including tabular data, resting-state functional magnetic resonance imaging (rs-fMRI), electrocardiogram (ECG) waveforms, and ultrasound (US), etc. Despite achieving some accomplishments, these studies have certain limitations [Citation21]. The majority of them are single-center retrospective studies with small sample sizes and a lack of external validation, posing challenges for the models in terms of generalization and large-scale application. To ascertain the effectiveness of these models, additional randomized controlled trials (RCTs) are still required. In the future, we will aim to establish additional multi-center, large-sample databases and create more innovative and practical algorithmic models. We aspire that these endeavors will ultimately enhance clinical decision-making, patient management, and healthcare outcomes for individuals with renal dysfunction.
Disclosure statement
No potential conflict of interest was reported by the author(s).
Additional information
Funding
References
- Bellomo R, Kellum JA, Ronco C, et al. Acute kidney injury in sepsis. Intensive Care Med. 2017;43(6):816–828. doi: 10.1007/s00134-017-4755-7.
- Kellum JA, Lameire N, Aspelin P, et al. Kidney disease: improving global outcomes (KDIGO) acute kidney injury work group. KDIGO Clinical Practice Guideline for Acute Kidney Injury. Kidney Int Suppl. 2012;2:1–138.
- Bikbov B, Purcell CA, Levey AS, et al. Global, regional, and national burden of chronic kidney disease, 1990– 2017: a systematic analysis for the global burden of disease study 2017. Lancet. 2020;395(10225):1–13.
- Kellum JA, Prowle JR. Paradigms of acute kidney injury in the intensive care setting. Nat Rev Nephrol. 2018;14(4):217–230. doi: 10.1038/nrneph.2017.184.
- Legrand K, Speyer E, Stengel B, et al. Perceived health and quality of life in patients with CKD, including those with kidney failure: findings from national surveys in France. Am J Kidney Dis. 2020;75(6):868–878. doi: 10.1053/j.ajkd.2019.08.026.
- Zhang L, Zhao MH, Zuo L, et al. China kidney disease network (CK-NET) 2016 annual data report. Kidney Int Suppl. 2020;10(2):e97–e185. doi: 10.1016/j.kisu.2020.09.001.
- Samad MD, Ulloa A, Wehner GJ, et al. Predicting survival from large echocardiography and electronic health record datasets: optimization with machine learning. JACC Cardiovasc Imaging. 2019;12(4):681–689. doi: 10.1016/j.jcmg.2018.04.026.
- Chen Z, Chen C, Chen F, et al. Bioinformatics analysis of potential pathogenesis and risk genes of immunoinflammation-promoted renal injury in severe COVID-19. Front Immunol. 2022;13:950076. doi: 10.3389/fimmu.2022.950076.
- Fleuren LM, Klausch TLT, Zwager CL, et al. Machine learning for the prediction of sepsis: a systematic review and meta-analysis of diagnostic test accuracy. Intensive Care Med. 2020;46(3):383–400. doi: 10.1007/s00134-019-05872-y.
- Luo XQ, Yan P, Zhang NY, et al. Machine learning for early discrimination between transient and persistent acute kidney injury in critically ill patients with sepsis. Sci Rep. 2021;11(1):20269. doi: 10.1038/s41598-021-99840-6.
- Zhou F, Lu Y, Xu Y, et al. Correlation between neutrophil-to-lymphocyte ratio and contrast-induced acute kidney injury and the establishment of machine-learning-based predictive models. Ren Fail. 2023;45(2):2258983. doi: 10.1080/0886022X.2023.2258983.
- Xu D, Zhou B, Zhang J, et al. Prediction of hyperkalemia in ESRD patients by identification of multiple leads and multiple features on ECG. Ren Fail. 2023;45(1):2212800. doi: 10.1080/0886022X.2023.2212800.
- Ronco C, Bellomo R, Kellum JA. Acute kidney injury. Lancet. 2019;394(10212):1949–1964. doi: 10.1016/S0140-6736(19)32563-2.
- Denic A, Glassock RJ, Rule AD. Structural and functional changes with the aging kidney. Adv Chronic Kidney Dis. 2016;23(1):19–28. doi: 10.1053/j.ackd.2015.08.004.
- Chertow GM, Burdick E, Honour M, et al. Acute kidney injury, mortality, length of stay, and costs in hospitalized patients. J Am Soc Nephrol. 2005;16(11):3365–3370. doi: 10.1681/ASN.2004090740.
- Alam N, Hobbelink EL, van Tienhoven AJ, et al. The impact of the use of the early warning score (EWS) on patient outcomes: a systematic review. Resuscitation. 2014;85(5):587–594. doi: 10.1016/j.resuscitation.2014.01.013.
- Li X, Zhu Y, Zhao W, et al. Machine learning algorithm to predict the in-hospital mortality in critically ill patients with chronic kidney disease. Ren Fail. 2023;45(1):2212790. doi: 10.1080/0886022X.2023.2212790.
- Vlieger D, Kashani G, Meyfroidt KG. Artificial intelligence to guide management of acute kidney injury in the ICU: a narrative review. Curr Opin Crit Care. 2020;26(6):563–573. doi: 10.1097/MCC.0000000000000775.
- Zhang Z, Ho KM, Hong Y. Machine learning for the prediction of volume responsiveness in patients with oliguric acute kidney injury in critical care. Crit Care. 2019;23(1):112. doi: 10.1186/s13054-019-2411-z.
- Norrie J. The challenge of implementing AI models in the ICU. Lancet Respir Med. 2018;6(12):886–888. doi: 10.1016/S2213-2600(18)30412-0.
- Wang F, Kaushal R, Khullar D. Should health care demand interpretable artificial intelligence or accept “black box” medicine? Ann Intern Med. 2020;172(1):59–60. doi: 10.7326/M19-2548.