Abstract
Background
Type 3 cardiorenal syndrome (CRS type 3) triggers acute cardiac injury from acute kidney injury (AKI), raising mortality in AKI patients. We aimed to identify risk factors for CRS type 3 and develop a predictive nomogram.
Methods
In this retrospective study, 805 AKI patients admitted at the Department of Nephrology, Second Hospital of Shanxi Medical University from 1 January 2017, to 31 December 2021, were categorized into a study cohort (406 patients from 2017.1.1-2021.6.30, with 63 CRS type 3 cases) and a validation cohort (126 patients from 1 July 2021 to 31 Dec 2021, with 22 CRS type 3 cases). Risk factors for CRS type 3, identified by logistic regression, informed the construction of a predictive nomogram. Its performance and accuracy were evaluated by the area under the curve (AUC), calibration curve and decision curve analysis, with further validation through a validation cohort.
Results
The nomogram included 6 risk factors: age (OR = 1.03; 95%CI = 1.009–1.052; p = 0.006), cardiovascular disease (CVD) history (OR = 2.802; 95%CI = 1.193–6.582; p = 0.018), mean artery pressure (MAP) (OR = 1.033; 95%CI = 1.012–1.054; p = 0.002), hemoglobin (OR = 0.973; 95%CI = 0.96-–0.987; p < 0.001), homocysteine (OR = 1.05; 95%CI = 1.03–1.069; p < 0.001), AKI stage [(stage 1: reference), (stage 2: OR = 5.427; 95%CI = 1.781–16.534; p = 0.003), (stage 3: OR = 5.554; 95%CI = 2.234–13.805; p < 0.001)]. The nomogram exhibited excellent predictive performance with an AUC of 0.907 in the study cohort and 0.892 in the validation cohort. Calibration and decision curve analyses upheld its accuracy and clinical utility.
Conclusions
We developed a nomogram predicting CRS type 3 in AKI patients, incorporating 6 risk factors: age, CVD history, MAP, hemoglobin, homocysteine, and AKI stage, enhancing early risk identification and patient management.
Introduction
Acute kidney injury (AKI) is a systemic syndrome that affects many organs, including the heart, lung, liver, spleen, brain, and intestine [Citation1], with high mortality and incidence [Citation2]. AKI was found in approximately 9% [Citation3] of in-hospital patients and over 35% of patients in the intensive care unit [Citation4]. Cardiovascular events are the main cause of death in AKI patients, accounting for 20% to 28% of AKI-related deaths [Citation5,Citation6]. The cardiac injury or dysfunction caused by AKI is called cardiorenal syndrome (CRS) type 3. CRS is a clinical entity that involves complex interactions between the heart and kidneys in both acute and chronic situations and has been classified into five types [Citation7].
For individuals experiencing AKI, it is crucial to acknowledge that beyond the mere existence of AKI, a range of other clinical characteristics and concurrent comorbidities can act as precipitating factors. These factors possess the potential to exacerbate the risk, severity, and duration of CRS type 3. According to previous studies, risk factors for acute decompensated heart failure (ADHF) after AKI include atrial fibrillation, arterial hypertension, kidney dysfunction, diabetes, anemia, and drugs such as non-steroidal anti-inflammatory drugs and glitazones [Citation8–10]. Among AKI patients, kidney artery stenosis (unilateral or bilateral) can also lead to ADHF, due to the excessive stimulation of the renin-angiotensin aldosterone axis, thereby elevating blood pressure and causes cardiac diastolic dysfunction [Citation11,Citation12].
The severity and duration of AKI may affect acute cardiac events directly or indirectly. Some evidence suggests that early activation of inflammatory mediators, oxidative stress, and the neuroendocrine system in AKI [Citation13] may directly promote apoptosis and cardiac inflammation. Moreover, the retention of water and salt along with increased demand of myocardial oxygen due to strong peripheral vasoconstriction could give rise to acute myocardial ischemia [Citation11,Citation12]. Similarly, AKI syndrome like volume overload, metabolic acidosis, retention of uremic toxins, hyperkalemia, and hypocalcemia can affect coronary vascular reactivity, ventricular remodeling, and cardiac fibrosis, which indirectly affect cardiac function [Citation14]. Despite this evidence, the understanding of complex heart-kidney interactions is still incomplete.
Compared to patients with chronic CRS (types 2 and 4), those with acute CRS (types 1 and 3) have more comorbidities and worse echocardiographic parameters [Citation15]. In clinical practice, acute cardiovascular events following AKI are very common, but there are currently few relevant clinical investigations. Insufficient research may explain why CRS type 3 is not as prevalent as CRS type 1. The clinical parameter data independently associated with the occurrence of CRS type 3 has not been thoroughly investigated, particularly for early prediction. Although there is evidence of complex interactions between the heart and kidney, our understanding of CRS type 3 is still incomplete. In this study, we aimed to identify the independent risk factors and develop a nomogram by analyzing the clinical characteristics of AKI patients as a model for predicting CRS type 3, providing a personalized, obvious-based, highly accurate risk prediction tool that is easy to use and can facilitate management-related decision-making.
Materials and methods
Design and study cohort
Data on consecutive inpatients between 1 January 2017, and 31 December 2021 were retrospectively collected from the Department of Nephrology, Second Hospital of Shanxi Medical University. According to Yang et al.’s research [Citation16], we have learned that AKI has a high missed diagnosis rate, so we screened AKI patients by using the 2012 Kidney Disease: Improving Global Outcomes (KDIGO) guideline [Citation17] and expanded diagnostic criteria [Citation16]. At the same time, we collected AKI's International Classification of Diseases Tenth Edition Clinical Modification (ICD-10-CM) codes [N17.0 (acute kidney failure with tubular necrosis), N17.1(acute kidney failure with acute cortical necrosis), N17.2 (acute kidney failure with medullary necrosis), N17.8 (other acute kidney failure), and N17.9 (acute kidney failure, unspecified)] to determine the reported AKI [Citation18]. This was a single-center retrospective cohort study on exploring the risk factors of CRS type 3 in AKI patients, conducted in accordance with Strengthening the Reporting of Observational Studies in Epidemiology (STROBE) guidelines (Supplementary Table S1) [Citation19].
Inclusion criteria:
Firstly, based on the electronic medical record system, we include all medical records for the first time admitted to the hospital from 1 January 2017 to 31 December 2021. Subsequently, patients with less than two serum creatinine (SCr) measurements during hospitalization were excluded. We use AKI defined by KDIGO as the main screening criterion [increase in SCr by ≥ 0.3 mg/dl (≥ 26.5 μmol/l) within 48 h; or increase in SCr to ≥ 1.5 times baseline, which is known or recommended to have occurred within the prior 7 days] [Citation17]. Confirm the AKI diagnosis by rechecking the change in SCr through the laboratory information system. For patients who repeated SCr measurements at intervals exceeding 7 days and those with AKI recovery, we expanded the screening criteria [an increase or decrease in SCr of at least 26.5 μmol/L during hospitalization; an increase or decrease in SCr of at least 50% during hospitalization (using SCr concentration as the baseline)] as additional indicators of AKI [Citation16].
Exclusion criteria:
1. Patients who have reached end-stage kidney disease (ESKD), begun kidney replacement therapy (KRT), performed kidney transplantation, or whose SCr peak was below 53 μmol/L at admission; 2. Patients who are experiencing acute cardiovascular events at the time of AKI diagnosis; 3. Patients under 18 years old or female during pregnancy or lactation; 4. Patients who have been hospitalized for less than 3 days. 5. Patients with SCr changes that meet the recognition criteria but cannot be attributed to AKI (such as a decrease in SCr after amputation).
At last, patients diagnosed with AKI were divided into two groups. The study cohort was 1 January 2017– 30 June 2021, and the validation cohort was 1 July 2021– 31 December 2021. The study was approved by the Medical Ethics Committee of the Second Hospital of Shanxi Medical University (No. 2023-YX-174).
CRS type 3 and acute cardiac dysfunction
CRS type 3 is a subclassification of CRS whereby AKI leads to acute cardiac dysfunction. Acute cardiac dysfunction is a broad term that includes a broad spectrum of heterogeneous conditions under the headings of acute decompensated heart failure (ADHF), acute coronary syndrome (ACS), arrhythmia, and cardiogenic shock [Citation5,Citation7,Citation8,Citation20–23]. The recommendation for identification of acute cardiac dysfunction or specific disorders is based on accepted definitions in the literature, such as the European Society of Cardiology guidelines, the American College of Cardiology Foundation/American Heart Association guidelines [Citation22,Citation23]. In our research, we defined any acute cardiovascular events that occurred shortly after AKI and could not be explained by other causes, as CRS type 3.
Collection of clinical variables
Consecutive data were gathered retrospectively. Baseline clinical and demographic data were collected on the first tests at admission, including sex (male, female), diabetes history (no, yes), cardiovascular disease (CVD) history (no, yes), AKI stage (stage 1, 2, 3), the causes of AKI (pre-renal, intrinsic, postrenal, unclassified), and the specific injury factors of AKI [decompensated cirrhosis (DCC), renal artery and occlusion (RAS and RAO), hemorrhage, acute gastroenteritis (AGE), nephrotoxic drugs/toxins, contrast-induced nephropathy (CIN), sepsis, surgery, rhabdomyolysis, urinary tract calculi or stenosis, prostatauxe, urologic/pelvic cancer, and neurogenic bladder] [Citation16,Citation24] as categorial variables; body mass index (BMI, kg/m2), albumin (ALB, g/L), and cystatin C (Cys C, mg/L), systolic blood pressure (SBP, mmHg), diastolic blood pressure (DBP, mmHg), mean artery pressure (MAP, mmHg), hemoglobin (g/L), uric acid (μmol/L), homocysteine (μmol/L), low-density lipoprotein (LDL, mmol/L), and triglyceride (TG, mmol/L) as continuous variables.
Statistical analysis
Continuous variables were summarized as means and standard deviations if the distribution was normal or as medians and interquartile ranges if the distribution was not normal. Categorical variables were reported as frequencies and percentages. Groups of quantitative variables were compared by using the Student t-test when the distribution was normal or the Mann-Whitney U test if the distribution was not normal. Groups of qualitative variables were compared by using chi-square or Fisher exact tests. Sensitivity analysis was performed in the study cohort to compare the data analysis on AKI diagnosis using KDIGO guideline versus KDIGO guideline and expanded criteria.
Then the data in the study cohort were used to establish a model, and the data in the validation set were applied to evaluate the efficacy of the model. Based on the data in the study cohort, univariable logistic regression analysis was performed for each variable. The p-values of the variables were calculated based on the univariable logistic proportional hazards regression model. The variables with p-values less than 0.05 were included in a multivariable logistic regression model. Then, the factors with p-values less than 0.05 were included in the prediction model to establish a nomogram. In the nomogram, the sum of these points, plotted on the ‘total points’ line, corresponded to the prediction of CRS type 3 occurrence-free rates in AKI patients. Receiver operating characteristic curve (ROC) analysis was used to assess the discrimination of the model, and a calibration plot was used for internal verification. Decision curve analysis (DCA) was utilized to evaluate the clinical application value of the model. All analyses were performed using SPSS (27.0 IBM, Armonk, NY, USA) and R (version 4.2.1) software.
Variables with a missing value ≥ 20% were excluded [Ratio of missing data for excluded variables: C-reactive protein 31% (165/532), B-type natriuretic peptide 39.7% (211/532), kidney pathology 78.6% (418/532)]. The rest variables with a missing value < 20% (Ratio of missing value of included variables: 0%-12.2% in total cohort; see Supplementary Table S2) were filled up with multiple imputation by performing the mice package for R [Citation25,Citation26].
Results
Clinical characteristics and univariate analysis results
In this study, after exclusion, 532 AKI patients who met inclusion criteria were enrolled. They were divided into 406 patients (the study cohort) between 1 January 2017 and 30 June 2021, and 126 patients (the validation group) between 1July 2021, and 31 December 2021 (). Out of the 532 AKI patients, 18 were diagnosed by expanded criteria, including 15 in the study cohort and 3 in the validation cohort. Among the 532 patients with AKI, 85 (approximately 15.977%) experienced CRS type 3, signifying an occurrence of around 15,977 cases per 100,000 AKI patients. In all acute cardiovascular events, ADHF is the most common one (44.7%), as shown in .
Figure 1. Flow chart.
Abbreviations: AKI, acute kidney injury; CRS, cardiorenal syndrome; ESKD, end-stage kidney disease; KRT, kidney replacement therapy.
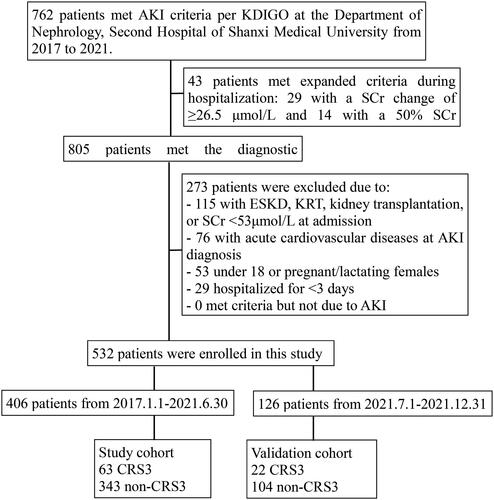
Table 1. Distribution of acute cardiovascular events.
Of the 406 patients in the study sample, 63 (15.5%) had CRS type 3 (CRS3 group), whereas 343 (84.5%) did not undergo CRS type 3 (non-CRS3 group). The clinical characteristics of the patients are listed in . The baseline clinicopathologic data were not statistically different between the study and validation cohorts. The univariate logistic analysis result of the study cohort showed that sex, age, CVD history, SBP, MAP, hemoglobin, homocysteine, and AKI stage were significantly different between the AKI patients with and without CRS type 3 (p < 0.05). Age, SBP, MAP, and homocysteine levels were greater in patients with CRS type 3 than in those without CRS type 3 (mean age: 62 ± 17 vs53 ± 18]; mean SBP level: 143 ± 22 vs 134 ± 21; mean MAP level: 103 ± 17 vs 98 ± 16; mean homocysteine level: 35 ± 16 vs 18 ± 19), while hemoglobin was lower in patients with CRS type 3 (mean hemoglobin level: 99 ± 22 vs 117 ± 29), with p values less than 0.05. Categorial variables such as sex (female), CVD history (yes), and higher AKI stage (stage 1 as reference) also showed greater significance in patients with CRS type 3 (sex: p = 0.018; CVD history: p = 0.03; AKI stage: p = 0.002).
Table 2. Characteristics of patients in the study and validation cohorts.
Multivariate analysis
The significant factors obtained in the univariate logistic analysis results were included in the multivariate logistic regression model to analyze whether each factor was an independent risk factor for inducing CRS type 3. Among them, MAP and SBP were both significantly different. We included MAP instead of SBP because MAP is better at describing the situation of blood pressure. In the multivariate analysis, results reported as older age (OR = 1.03; 95%CI = 1.009–1.052), CVD history (OR = 2.802; 95%CI = 1.193–6.582), MAP (OR = 1.033; 95%CI = 1.012–1.054), hemoglobin (OR = 0.973; 95%CI = 0.96–0.987), homocysteine (OR = 1.05; 95%CI = 1.03–1.069), and AKI stage [(stage 1: reference), (stage 2: OR = 5.427; 95%CI = 1.781–16.534), (stage 3: OR = 5.554; 95%CI = 2.234–13.805)] were independently associated with CRS type 3, with p values less than 0.05 (; ).
Figure 2. Independent risk factors of CRS type 3 occurrence among AKI patients.
Abbreviations: AKI, acute kidney injury; CRS cardiorenal syndrome; CVD, :cardiovascular disease; MAP: mean artery pressure.
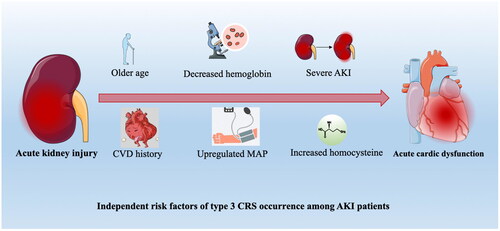
Table 3. Multivariate logistic analysis of the study cohort.
To further support the stability of the results based on the inclusion of expanded criteria, sensitivity analysis was performed and showed that the conclusions did not vary significantly (Supplementary Table S3).
Performance of the nomogram
The 6 independent risk factors were used to create a risk estimation nomogram based on the CRS type 3-occurrence-free rate (). As seen in the nomogram, the selected predictors were scored using the prediction model’s values. From that point, a vertical line was drawn perpendicular to the point’s axis. The score under the predicted value was represented by the intersection point on the point axis. For example, a 60-year-old patient with a MAP of 160 mmHg, hemoglobin of 90 g/L, and homocysteine of 50 μmol/L has a risk index between 70% to 90% (). Another example was shown in . We suggest that AKI patients with advanced age, high homocysteine, high MAP, a high AKI stage, low hemoglobin, and/or a history of CVD require regular medical monitoring and treatment to prevent CRS type 3.
Figure 3. Risk nomogram conducted by logistic regression.
Abbreviations: CVD: cardiovascular disease; MAP:mean artery pressure; AKI: acute kidney injury
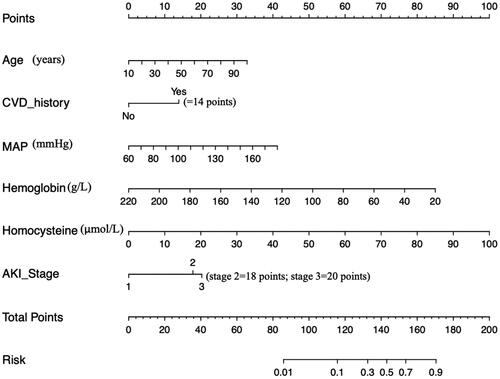
Figure 4. Examples of the nomogram in clinical practice.
Figures illustrate the process of calculating the risk scores of CRS3 and non-CRS3 using the nomogram. (A) A 60-year-old patient with MAP level of 160 mmHg, hemoglobin level of 90g/L, and homocysteine level of 50 μmol/L at admission, which corresponds to a risk between 0.7 to 0.9 (70%-90%). (B) A 30-year-old patient with AKI stage 3, CVD history, MAP level of 140mmHg, and homocysteine level of 35 μmol/L at admission, which corresponds to an CRS3 risk of less than 0.1 (<10%).
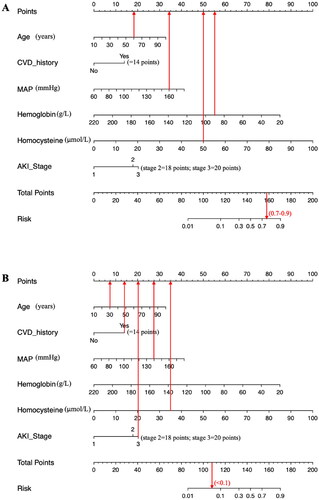
Validation and effect evaluation of the nomogram
The resulting model was internally validated based on the validation cohort. The performance of the nomogram was measured by the ROC curve (). The AUC of the nomogram was 0.907. In the validation cohort, the nomogram still had good discrimination (AUC = 0.892; ). Based on the cutoff (-1.833) value of the ROC in the study cohort, the sensitivity and specificity of the prediction model in the validation cohort were 77.3% and 81.7% (80.2% and 90.5% in the study cohort) (). The calibration curve showed good homogeneity between the prediction by nomogram and the actual observation (). The DCA demonstrated that the prediction model could provide a great net benefit and make valuable and profitable judgments ().
Figure 5. Receiver operating characteristic curves of the nomogram in the study cohort (A) and the validation cohort (B).
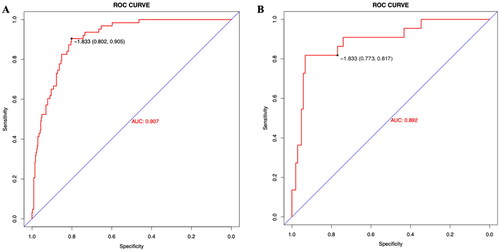
Figure 6. The calibration curve and results of the DCA of the nomogram in the validation cohort.
(A) Calibration curves represent the difference between the actual prediction and the ideal perfect prediction (45° line). (B) The DCA curve of the nomogram for predicting CRS type 3. It revealed that the nomogram could obtain a greater net benefit than either the ‘treat all’ or the ‘treat none’ strategy
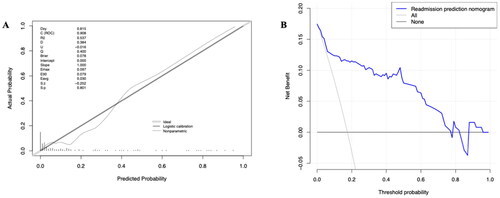
Table 4. Prediction model accuracy in the validation cohort.
Discussion
In our retrospective study, we examined 406 AKI patients as the study cohort and 126 patients as the validation cohort. After multivariable analysis, older age, CVD history, MAP, hemoglobin, homocysteine, and AKI stage were independently linked with CRS type 3.
Several studies have examined the involvement of kidney dysfunction in the progression of CVD events, as well as the effect of older age, preexisting ADHF, high blood pressure, and anemia in acute cardiac events [Citation8,Citation21,Citation27–30]. Our study validated their findings and further verified their functions as the main clinical features for CRS type 3 prediction. We use the term continuous variables to refer to age, hemoglobin levels, and all three blood pressure measures (systolic, diastolic, and mean arterial) to examine and quantify their impact on CRS type 3. Moreover, we identify preexisting ADHF as CVD, along with ACS, arrhythmia, and cardiogenic shock.
In accordance with the literature, elderly patients had a greater probability of acquiring cardiac events during follow-up. For example, the average age at which CVD events such as myocardial infarction or congestive HF occuris 65 years, and CVD incidence and mortality increase with age [Citation21,Citation27]. Another study found that AKI patients over 60 years old with HF are more likely to develop ESKD and have high mortality [Citation8]. The particular age changes, however, have not yet been studied in CRS type 3. Our study confirmed the independent risk factor role of age as a continuous variable for CRS type 3. Relevant studies show that people with congestive HF and coronary artery disease are more likely to develop CRS type 3 [Citation8]. Primary CVD, such as atherosclerosis, which causes plaque to build up in the arteries, can damage blood vessels and limit blood flow to the kidneys, causing kidney dysfunction. Moreover, CVD can alter heart and kidney hemodynamics, increasing pressure in the kidney’s filtration system and causing further kidney damage [Citation8,Citation21]. In this study, CVD history was an important risk factor for CRS type 3 in AKI patients. This may be because patients with a prior CVD history are more likely to have damaged blood vessels, reduced blood flow, and worse heart function than the normal population. Therefore, CRS type 3 is more likely to arise with preexisting cardiac damage. Consistent with previous studies [Citation31], our study found that blood pressure was an independent risk factor for CRS type 3. Our study demonstrated greater SBP in type 3 CRS patients (mean SBP: 143 ± 22), contrary to the prior finding. Furthermore, MAP was verified as an independent risk factor for CRS type 3. Clinicians should emphasize strict blood pressure control in AKI patients, especially those at risk of CRS type 3. Our study indicated that decreasing hemoglobin level was an independent risk factor for CRS type 3, which is consistent with prior research [Citation28]. Instead of using the term ‘anemia’, we treated hemoglobin levels as a continuous variable in our analysis. We also identified homocysteine as a previously unexplored risk factor for CRS type 3 in AKI. Homocysteine has been well-recognized in the role of CVD [Citation29], but it has not been studied in CRS type 3 previously. Our study found that homocysteine is the most valuable indicator in all features for early prediction. The study also examined the prediction effect of AKI stage on CRS type 3. Compared to stage 1, patients with stage 2 and 3 are at the higher risk of the occurrence of acute cardiac dysfunction. In stage 3, the patient is at the highest risk. This finding suggests that the severity of AKI is an important predictor of CRS type 3 and patients with severer AKI should be closely monitored for the development of CRS type 3. Accordingly, a study by Heung et al. revealed that patients with AKI stage 3 had a higher risk of acute cardiac events and mortality compared to patients with AKI stage 1 or 2 [Citation30]. Our study highlights the importance of identifying and monitoring patients with higher AKI stages. As AKI progresses, there is an increasing risk of cardiac dysfunction.
As always for such observational study, several limitations have to be underlined. First, this is a retrospective study and not all potential risk factors for AKI were collected, such as kidney pathology, or heart pathology. Kidney biopsy was limited in AKI patients because on many occasions, we are faced with fragile patients, severe increase of serum creatinine, or at high risk of complications of the biopsy technique [Citation32]. In additional, a part of the AKI patients had experienced acute heart failure, which also limited the use of antiplatelet and anticoagulant drugs and restricted the implementation of kidney biopsy [Citation33]. In our study, among 532 AKI patients, only 114 patients (21.4%) underwent kidney biopsy, leading to 78.6% missing data ratio for the exact pathological diagnosis. Therefore, we weren’t able to include the exact pathology of AKI in our study. Heart biopsy is also limited in our study given that performing a heart biopsy in patients experiencing acute cardiovascular events carries heightened risks, including conduction abnormalities, valvular damage, embolism, cardiac perforation, or death [Citation34]. Another limitation might be the using of expanded criteria for AKI [Citation16], which may cause a degree of selection bias. However, our sensitivity analysis has showed that the conclusions did not vary significantly, further supporting the stability of the inclusion criteria of our study. Third, we only included the patients in the Department of Nephrology of our hospital; the absence of other departments and even an external cohort for further validation are required in our future study. Finally, the sample size of the present study was relatively small. Further studies with larger cohorts are needed to confirm the identified risk factors and the nomogram’s accuracy. Despite all the limitations, our study has provided novel insights on recognizing CRS type 3 among AKI patients and it may, for the first time, constitute a useful risk scoring system for clinicians in practice. We hope that this study will enable clinicians to have a deeper understanding of and pay attention to the clinical judgment and diagnosis of CRS type 3.
In conclusion, our study identified 6 independent risk factors for the development of CRS type 3 in AKI patients, including age, MAP, homocysteine, hemoglobin, AKI stage, and prior history of CVD. The construction of nomograms provides a useful tool for visualizing the contributions of each risk factor to the overall risk, constituting a scoring system for early prediction and management of CRS type 3 in practice.
Authors’ contributions
Research idea and study design: Hui Lin, Xi Qiao, and Xiaole Su; acquisition of data: Hui Lin and Mengzhu Wang; analysis and interpretation of data: Hui Lin, Xiaoyu Guo and Mengzhu Wang; drafting the article: Hui Lin, Mengzhu Wang and Xiaoyu Guo; revising it critically for important intellectual content: Xi Qiao and Xiaole Su; and final approval of the version to be published: all authors.
Supplemental Material
Download MS Word (42.7 KB)Disclosure statement
No conflicts of interest, financial or otherwise, are declared by the authors.
Additional information
Funding
References
- Kao CC, Yang WS, Fang JT, et al. Remote organ failure in acute kidney injury. J Formos Med Assoc. 2019;118(5):1–11. doi: 10.1016/j.jfma.2018.04.005.
- Kellum JA, Romagnani P, Ashuntantang G, et al. Acute kidney injury. Nat Rev Dis Primers. 2021;7(1):52. doi: 10.1038/s41572-021-00284-z.
- Uchino S, Bellomo R, Goldsmith D, et al. An assessment of the RIFLE criteria for acute renal failure in hospitalized patients. Crit Care Med. 2006;34(7):1913–1917. doi: 10.1097/01.CCM.0000224227.70642.4F.
- Morgan D. A multi-centre evaluation of the RIFLE criteria for early acute kidney injury in critically ill patients. Nephrol Dial Transplant. 2008;23(12):4072–4073; author reply 4073. doi: 10.1093/ndt/gfn390.
- Ricci Z, Romagnoli S, Ronco C. Cardiorenal syndrome. Crit Care Clin. 2021;37(2):335–347. doi: 10.1016/j.ccc.2020.11.003.
- de Abreu KL, Silva Júnior GB, Barreto AG, et al. Acute kidney injury after trauma: prevalence, clinical characteristics and RIFLE classification. Indian J Crit Care Med. 2010;14(3):121–128. doi: 10.4103/0972-5229.74170.
- Ronco C, McCullough P, Anker SD, et al. Cardio-renal syndromes: report from the consensus conference of the acute dialysis quality initiative. Eur Heart J. 2010;31(6):703–711. doi: 10.1093/eurheartj/ehp507.
- Di Lullo L, Bellasi A, Russo D, et al. Cardiorenal acute kidney injury: epidemiology, presentation, causes, pathophysiology and treatment. Int J Cardiol. 2017;227:143–150. doi: 10.1016/j.ijcard.2016.11.156.
- Kashani K, Al-Khafaji A, Ardiles T, et al. Discovery and validation of cell cycle arrest biomarkers in human acute kidney injury. Crit Care. 2013;17(1):R25. doi: 10.1186/cc12503.
- Gheorghiade M, Zannad F, Sopko G, et al. Acute heart failure syndromes: current state and framework for future research. Circulation. 2005;112(25):3958–3968. doi: 10.1161/CIRCULATIONAHA.105.590091.
- Gandhi SK, Powers JC, Nomeir AM, et al. The pathogenesis of acute pulmonary edema associated with hypertension. N Engl J Med. 2001;344(1):17–22. doi: 10.1056/NEJM200101043440103.
- Hirsch AT, Haskal ZJ, Hertzer NR, et al. ACC/AHA guidelines for the management of patients with peripheral arterial disease (lower extremity, renal, mesenteric, and abdominal aortic): a collaborative report from the American associations for vascular surgery/society for vascular surgery, society for cardiovascular angiography and interventions, society for vascular medicine and biology, society of interventional radiology, and the ACC/AHA task force on practice guidelines (writing committee to develop guidelines for the management of patients with peripheral arterial disease)–summary of recommendations. J Vasc Interv Radiol. 2006;17(9):1383–1397; quiz 1398. doi: 10.1097/01.RVI.0000240426.53079.46.
- Kelly KJ. Distant effects of experimental renal ischemia/reperfusion injury. J Am Soc Nephrol. 2003;14(6):1549–1558. doi: 10.1097/01.asn.0000064946.94590.46.
- Zager RA. Uremia induces proximal tubular cytoresistance and heme oxygenase-1 expression in the absence of acute kidney injury. Am J Physiol Renal Physiol. 2009;296(2):F362–F368. doi: 10.1152/ajprenal.90645.2008.
- Mavrakanas TA, Khattak A, Singh K, et al. Epidemiology and natural history of the cardiorenal syndromes in a cohort with echocardiography. Clin J Am Soc Nephrol. 2017;12(10):1624–1633. doi: 10.2215/CJN.04020417.
- Yang L, Xing G, Wang L, et al. Acute kidney injury in China: a cross-sectional survey. Lancet. 2015;386(10002):1465–1471. doi: 10.1016/S0140-6736(15)00344-X.
- Kdigo K. KDIGO clinical practice guideline for acute kidney injury. Kidney Int Suppl. 2012;1(2):1–138.
- World Health Organization. ICD-10: international statistical classification of diseases and related health problems: tenth revision., 2nd ed. Geneva, Switzerland: World Health Organization; 2004. https://apps.who.int/iris/handle/10665/42980.
- von Elm E, Altman DG, Egger M, et al. The strengthening the reporting of observational studies in epidemiology (STROBE) statement: guidelines for reporting observational studies. J Clin Epidemiol. 2008;61(4):344–349. doi: 10.1016/j.jclinepi.2007.11.008.
- Chuasuwan A, Kellum JA. Cardio-renal syndrome type 3: epidemiology, pathophysiology, and treatment. Semin Nephrol. 2012;32(1):31–39. doi: 10.1016/j.semnephrol.2011.11.005.
- Bagshaw SM, Hoste EA, Braam B, et al. Cardiorenal syndrome type 3: pathophysiologic and epidemiologic considerations. Contrib Nephrol. 2013;182:137–157. doi: 10.1159/000349971.
- McDonagh TA, Metra M, Adamo M et al. 2021 ESC guidelines for the diagnosis and treatment of acute and chronic heart failure. Eur Heart J. 2021;42(36):3599–3726. doi: 10.1093/eurheartj/ehab368.
- Jessup M, Abraham WT, Casey DE, et al. ACCF/AHA guidelines for the diagnosis and management of heart failure in adults: a report of the American College of Cardiology Foundation/American Heart Association Task Force on Practice Guidelines: developed in collaboration with the International Society for Heart and Lung Transplantation. Circulation. 2009;119(14):1977–2016. doi: 10.1161/CIRCULATIONAHA.109.192064.
- Lameire N, Van Biesen W, Vanholder R. Acute renal failure. Lancet. 2005;365(9457):417–430. doi: 10.1016/S0140-6736(05)17831-3.
- Dusseux E, Albano L, Fafin C, et al. A simple clinical tool to inform the decision-making process to refer elderly incident dialysis patients for kidney transplant evaluation. Kidney Int. 2015;88(1):121–129. doi: 10.1038/ki.2015.25.
- de Goeij MC, van Diepen M, Jager KJ, et al. Multiple imputation: dealing with missing data. Nephrol Dial Transplant. 2013;28(10):2415–2420. doi: 10.1093/ndt/gft221.
- Coca SG, Cho KC, Hsu CY. Acute kidney injury in the elderly: predisposition to chronic kidney disease and vice versa. Nephron Clin Pract. 2011;119 Suppl 1(Suppl 1):c19–c24. doi: 10.1159/000328023.
- Hoste EA, Bagshaw SM, Bellomo R, et al. Epidemiology of acute kidney injury in critically ill patients: the multinational AKI-EPI study. Intensive Care Med. 2015;41(8):1411–1423. doi: 10.1007/s00134-015-3934-7.
- Srisawat N, Kellum JA. The role of biomarkers in acute kidney injury. Crit Care Clin. 2020;36(1):125–140. doi: 10.1016/j.ccc.2019.08.010.
- Heung M, Steffick DE, Zivin K, et al. Acute kidney injury recovery pattern and subsequent risk of CKD: an analysis of veterans health administration data. Am J Kidney Dis. 2016;67(5):742–752. doi: 10.1053/j.ajkd.2015.10.019.
- Siirilä-Waris K, Lassus J, Melin J, et al. Characteristics, outcomes, and predictors of 1 year mortality in patients hospitalized for acute heart failure. Eur Heart J. 2006;27(24):3011–3017. doi: 10.1093/eurheartj/ehl407.
- Zheng XZ, Gu YH, Su T, et al. Elevation of erythrocyte sedimentation rate and C-reactive protein levels reflects renal interstitial inflammation in drug-induced acute tubulointerstitial nephritis. BMC Nephrol. 2020;21(1):514. doi: 10.1186/s12882-020-02175-z.
- Sanchez-Alamo B, Cases-Corona C, Fernandez-Juarez G. Facing the challenge of Drug-Induced acute interstitial nephritis. Nephron. 2023;147(2):78–90. doi: 10.1159/000525561.
- Kiamanesh O, Toma M. The state of the heart biopsy: a clinical review. CJC Open. 2020; Dec 13(4):524–531. doi: 10.1016/j.cjco.2020.11.017.