Abstract
Background: During the acute heart failure (AHF), acute kidney injury (AKI) is highly prevalent in critically ill patients. The occurrence of the latter condition increases the risk of mortality in patients with acute heart failure. The current research on the relationship between nutritional risk and the occurrence of acute kidney injury in patients with acute heart failure is very limited. Methods: This retrospective cohort study utilized data from the Medical Information Mart for Intensive Care IV (MIMIC-IV, version 2.1) database. We included adult patients with AHF who were admitted to the intensive care unit in the study. Results: A total of 1310 critically ill patients with acute heart failure were included. The AUC of geriatric nutritional risk index (GNRI) (0.694) is slightly superior to that of controlling nutritional status (CONUT) (0.656) and prognostic nutritional index (PNI) (0.669). The Log-rank test revealed a higher risk of acute kidney injury in patients with high nutritional risk (p < 0.001). Multivariate COX regression analysis indicated that a high GNRI (adjusted HR 0.62, p < 0.001) was associated with a reduced risk of AKI during hospitalization in AHF patients. The final subgroup analysis demonstrated no significant interaction of GNRI in all subgroups except for diabetes subgroup and ventilation subgroup (P for interaction: 0.057-0.785). Conclusion: Our study findings suggest a correlation between GNRI and the occurrence of acute kidney injury in patients hospitalized with acute heart failure.
1. Introduction
During acute heart failure, Acute Kidney Injury (AKI) is highly prevalent in critically ill patients [Citation1]. The occurrence of AKI increases the risk of mortality in patients with acute heart failure [Citation2], and its development relies on intricate pathophysiological mechanisms between the two [Citation3]. Nutritional status is closely associated with adverse outcomes in patients with acute heart failure (AHF) and acute kidney injury [Citation4,Citation5]. The Geriatric Nutritional Risk Index (GNRI) is a tool for screening malnutrition and has been confirmed to be associated with the prognosis of various diseases. This index was first reported by Bouillanne et al. [Citation6], who divided patients into four groups: a major-risk group (GNRI: <82), a moderate-risk group (GNRI: 82–92), a low-risk group (GNRI: 92–98), and a no-risk group (GNRI: >98). Previous research has explored various risk factors for the development of acute kidney injury in patients with acute heart failure, including serum lactate levels, cellular casts, triglyceride glucose index, soluble thrombomodulin, among others [Citation7–10]. However, limited investigation has been conducted into whether nutritional risk can impact the incidence of acute kidney injury in patients with acute heart failure. Therefore, this study aims to investigate the relationship between malnutrition and the incidence of acute kidney injury in patients with acute heart failure.
2. Materials and methods
2.1. Database and study population
This retrospective cohort study utilized the fourth-generation Medical Information Mart for Intensive Care (MIMIC-IV version 2.1) database, a longitudinal single-center repository housing data from over 50,000 ICU admissions at Beth Israel Deaconess Medical Center in Boston from 2008 to 2019. Access to the database was obtained following completion of an online training course and exam (certification number: 51383956) by the author, Sen Zhang. The employment of the MIMIC-IV database received approval from the institutional review boards of both the Massachusetts Institute of Technology and Beth Israel Deaconess Medical Center. Due to anonymization of the data, the need for informed consent was waived. The study population consisted of patients diagnosed with heart failure selected from MIMIC-IV. Exclusion criteria included: (1) patients lacking ICU records or with multiple ICU admissions, (2) patients with ICU stays of less than 24 h, (3) patients under 65 years of age, (4) patients with missing baseline data, and (5) patients with end-stage kidney disease. Figure S1 illustrates the flowchart for patient selection.
2.2. Assessment of nutritional status
GNRI was calculated using formula as follows: GNRI = [1.489 × albumin (ALB) (g/L)] + [41.7 × (body weight/ideal body weight by Lorentz equation)]. The ideal body weight was calculated as follows: for men: H–100–[(H − 150)/4], for women: H–100–[(H − 150)/2.5], where H indicates height (cm)[Citation6]. Prognostic Nutritional Index (PNI) was calculated using formula as follows: PNI = albumin (ALB) (g/L) + 0.005 × lymphocyte count (/mm3) [Citation11]. Controlling Nutritional Status (CONUT) was determined based on lymphocyte count, TC (total cholesterol), and ALB as previously described [Citation12].
2.3. Data extraction
We utilized the PostgreSQL tool (v.16, PostgreSQL Global Development Group, Berkeley, California, USA) to extract data. Using structured query language (SQL), the diagnoses of AHF and AKI in this study were extracted from the MIMIC-IV database based on International Classification of Diseases (ICD) codes, whether ICD-9 or ICD-10. Relevant variables were extracted within 24 h of patient admission, including: (1) baseline characteristics: age, gender, race; (2) comorbidities: myocardial infarction, diabetes, chronic obstructive pulmonary disease, hypertension, cerebrovascular disease, chronic kidney disease; (3) vital signs: heart rate, systolic blood pressure, diastolic blood pressure, mean arterial pressure, and mean respiratory rate; (4) disease severity scores: Sequential Organ Failure Assessment (SOFA) score and Glasgow Coma Scale (GCS); (5) laboratory parameters: hemoglobin, white blood cell count, platelet count, lymphocyte count, blood urea nitrogen (BUN), creatinine, albumin, bicarbonate, blood glucose, chloride, sodium, potassium, lactate, and anion gap; (6) hospitalization and ICU data: admission and discharge times, ICU admission and discharge times, and date and time of death. All measured parameters used in this study were the average values obtained within 24 h of ICU admission.
3. Statistical analysis
To analyze the data, we employed various statistical methods and models. Initially, we delineated the baseline characteristics of patients using two stratification approaches: one categorized patients based on acute kidney injury occurrence, while the other stratified them into high and low nutritional risk groups, determined by the optimal cutoff value of GNRI. Continuous variables were expressed as median or quartiles, and categorical variables as frequency and percentage. Differences between continuous variables were compared using analysis of variance or rank-sum tests, while differences between categorical variables were assessed using chi-square or Fisher’s exact tests. Subsequently, we conducted univariate and multivariate analyses to explore factors influencing AKI occurrence in AHF patients. Cox regression models were employed to ascertain whether GNRI independently correlated with AKI incidence in AHF, with results presented as hazard ratios (HR) and 95% confidence intervals (CI). Model adjustments were made as follows: Model II adjusted for age, gender, and mean blood pressure (MBP); Model III further adjusted for hemoglobin, BUN, creatinine, bicarbonate, chloride, anion gap, and lactate; Model IV additionally adjusted for ventilation, contrast media, chronic kidney disease (CKD), Sequential Organ Failure Assessment (SOFA), Glasgow Coma Scale (GCS), and Charlson’s comorbidity index. We utilized a restricted cubic spline model to explore potential linear associations between GNRI and AKI incidence rates, stratifying the sample based on optimal thresholds. Kaplan-Meier and log-rank tests were used to plot survival curves. Receiver Operating Characteristic (ROC) analysis evaluated the predictive ability of GNRI, CONUT and PNI for AKI occurrence during hospitalization, calculating sensitivity, specificity, and the Area Under Curve (AUC). Threshold analysis of the GNRI association with the outcome was conducted using a likelihood ratio test comparing models with linear and cubic spline terms. Furthermore, subgroup analyses assessed potential differences in AKI incidence during ICU stay among AHF subgroups based on GNRI levels. All analyses were performed using R statistical software version 4.2, with statistical significance set at p < 0.05 (two-tailed) without adjustment for multiple comparisons.
4. Results
4.1. Baseline characteristics of the study patients
We extracted data from 1310 eligible patients from the MIMIC-IV database (Figure S1). The median age of the patients was 78.7 years (interquartile range, 72.3–85.0). There were no significant differences between the two groups of patients in terms of age and race. However, patients who experienced AKI had lower GNRI levels (87.1 [80.4, 92.3] vs. 93.3 [87.9, 98.3], p < 0.001). Additionally, patients with AKI had lower levels of hemoglobin, platelets, lymphocytes, albumin, bicarbonate, SBP, DBP, MBP, and GCS score (all p < 0.05), as well as higher levels of white blood cells, BUN, creatinine, blood glucose, potassium, anion gap, lactate, SPO2, heart rate, and respiratory rate (all p < 0.05). Furthermore, patients who developed AKI were more likely to undergo renal replacement therapy, ventilation, and contrast agent use, and were more likely to have comorbid chronic kidney disease (). In the subgroup with lower GNRI, higher rates of AKI occurrence (70.8% vs 40.5%, p < 0.001), as well as poorer laboratory parameters and vital signs, were observed (Table S1).
Table 1. Baseline characteristics of participants according to AKI occurrence.
4.2. Restricted cubic splines (RCS) analysis
We employed restricted cubic splines (RCS) to examine and illustrate the relationship between GNRI and AKI incidence in AHF patients. Following adjustment for all pertinent variables, we detected a linear correlation between GNRI and AKI occurrence (non-linear p = 0.085, ). As GNRI levels decrease, the risk of AKI occurrence in AHF patients rises. The optimal cutoff value is GNRI = 89.4.
4.3. ROC curve analysis
We plotted ROC curves for three indicators, GNRI, CONUT, and PNI, to predict the occurrence of AKI in patients with acute heart failure, and the information from is listed in Table S2. The AUC of GNRI [0.694 (95% CI: 0.665–0.722)] is slightly higher than that of CONUT [0.656 (95% CI: 0.626–0.685)] and PNI [0.669 (95% CI: 0.639–0.698)]. Therefore, there is a reliable relationship between GNRI and the occurrence of AKI in AHF patients. Additionally, we obtained the optimal cutoff value for GNRI as 89.4, with a sensitivity of 0.651 and a specificity of 0.657. We used this value as the stratification basis to plot Kaplan–Meier curves for different nutritional risk groups.
4.4. Kaplan–Meier analysis
The Kaplan–Meier curve for GNRI is depicted in . It is evident that in the subgroup with higher nutritional risk (≤89.4), AHF patients are more prone to developing AKI (p < 0.001, as determined by the logarithmic rank test).
Figure 3. Kaplan–Meier curve for the occurrence of AKI in patients with AHF. GNRI: Geriatric Nutritional Risk Index; Time: The number of days of ICU stay.
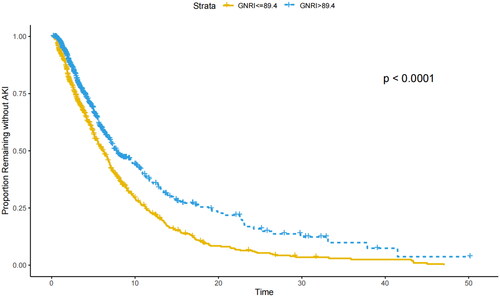
Utilizing a two-piecewise Cox regression model, we determined that the threshold of GNRI was 89.4 (). Above this threshold, for every 1-unit increase in GNRI, there was a 4.3% decrease in the occurrence of AKI (adjusted HR, 0.957; 95% CI, 0.939-0.976) ().
Table 2. Threshold analyses of GNRI on AKI occurrence using two-piecewise regression models.
4.5. Association between GNRI on admission and the occurrence of AKI in critically ill patients with AHF
We conducted a univariate Cox regression analysis of AKI occurrence in critically ill AHF patients. Significant variables (p < 0.05) from this analysis were further incorporated into our multivariate Cox regression model (Table S3). In the unadjusted model, higher GNRI levels were associated with a reduced occurrence of AKI in AHF patients (HR 0.67, 95% CI 0.58 to 0.78, p < 0.001) (). In Model II, after adjusting for age, gender, and MBP, GNRI remained associated with AKI occurrence (HR 0.98, 95% CI 0.97 to 0.99, p < 0.001). Compared to the lower GNRI group, higher GNRI levels were associated with a lower occurrence of AKI (HR 0.69, 95% CI 0.62 to 0.80, p < 0.001). In Model III, further adjustments were made, incorporating hemoglobin, BUN, creatinine, bicarbonate, chloride, aniongap, and lactate, revealing a continued relationship between GNRI and AKI occurrence (HR 0.99, 95% CI 0.98 to 1.00, p = 0.007). A decrease in AKI occurrence was still observed in the higher GNRI group (HR 0.78, 95% CI 0.66 to 0.91, p = 0.002). Model IV further adjusted for ventilation, contrast media, CKD, SOFA, GCS, and Charlson’s comorbidity index, where GNRI remained associated with AKI occurrence (HR 0.95, 95% CI 0.96 to 0.97, p < 0.001), and higher GNRI levels were also associated with a reduced occurrence of AKI (HR 0.62, 95% CI 0.53 to 0.72, p < 0.001). Similarly, in the multivariable logistic regression model, after adjusting for confounding factors, the relationship between GNRI and AKI occurrence in AHF patients persisted (OR 0.92, 95% CI 0.91 to 0.94, p < 0.001) (Table S4-5).
Table 3. The relationship between the GNRI and AKI occurrence in a cox regression model.
4.6. Subgroup analysis of the relationship between GNRI and the occurrence of AKI in critically ill patients with AHF
To further elucidate whether other common comorbidities influence the relationship between GNRI and AKI occurrence in critically ill AHF patients, we conducted subgroup analyses. Stratified analyses were performed for age, gender, cerebrovascular disease, hypertension, myocardial infarction, chronic pulmonary disease, chronic kidney disease, and renal replacement therapy. indicates that there was no significant interaction between GNRI and each subgroup (P for interaction: 0.057–0.785). Significant interactions were only observed in the diabetes and ventilation subgroups. This suggests that the association between GNRI and AKI occurrence in critically ill AHF patients remains stable.
Table 4. Subgroup analyses of association between GNRI on AKI occurrence in critically ill patients with AHF.
5. Discussion
The results of this retrospective study emphasize the independent correlation between GNRI and the incidence of acute kidney injury (AKI) in patients admitted with acute heart failure. GNRI performed remarkably well, comparable to CONUT and PNI, both of which have been proven effective in predicting AKI occurrence in patients [Citation13,Citation14]. Additionally, Kaplan-Meier survival analysis plots indicate that acute heart failure patients with lower GNRI values, stratified according to the optimal cutoff point, have a significantly elevated risk of developing AKI during hospitalization, providing further evidence for our hypothesis.
Nutritional assessment is pivotal for patients with heart failure [Citation15]. Malnutrition is highly prevalent in this patient population [Citation16] and can significantly influence their prognosis [Citation17,Citation18]. Multiple studies have investigated the prognostic significance of different nutritional assessment tools in heart failure patients, among which GNRI demonstrates a robust correlation with patient outcomes. Lower GNRI levels are associated with worse prognoses in heart failure patients [Citation19–22].
Additionally, as a common complication in patients with heart failure, the specific mechanisms underlying the relationship between nutritional status and the occurrence of AKI are not yet clear. There may be several mechanisms involved: Firstly, lower levels of hemoglobin were observed in both the AKI subgroup and the high nutritional risk subgroup. Higher nutritional risk may suppress bone marrow hematopoiesis, thereby increasing the occurrence of AKI. The bone marrow is an essential hematopoietic organ in the body, and malnutrition may disrupt the microenvironment of the bone marrow stroma and impair hematopoietic function, leading to reduced synthesis of red blood cells and hemoglobin [Citation23]. The deficiency of hemoglobin and red blood cells decreases oxygen supply to the kidneys, thereby increasing the incidence of AKI [Citation24]. Secondly, previous studies have indicated that severe malnutrition is associated with increased inflammation [Citation25]. Various inflammatory cytokines, including interleukins −1 and −6, as well as tumor necrosis factor-α, exacerbate vasoconstriction and oxidative stress, leading to renal medullary hypoxia, impairment of tubular cells, and endothelial cell dysfunction, ultimately affecting renal function and thereby increasing the risk of AKI [Citation26,Citation27]. Finally, there is an interaction between AKI and malnutrition. AKI is associated with disturbances in fluid, electrolyte, and acid-base balance, leading to specific alterations in protein, carbohydrate, and lipid metabolism, which result in changes in BMI and albumin levels [Citation28,Citation29]. A meta-analysis of 22 studies indicated that compared to individuals with normal BMI, underweight, overweight, or obese patients had a higher risk of developing AKI [Citation30], Sabaz et al. conducted a retrospective analysis of 4459 patients admitted to the ICU, which also demonstrated a higher incidence of AKI among obese patients [Citation31]. Xu et al. found that preoperative serum albumin level is an independent risk factor for the occurrence of acute kidney injury (AKI) after surgery for type A aortic dissection [Citation32], and multiple studies have demonstrated a significant association between albumin levels and the prognosis of AKI patients [Citation33–35]. The disruption of fluid and electrolyte balance and changes in albumin levels in patients after AHF may exacerbate the occurrence of AKI [Citation36,Citation37], and after AKI occurs, this factor may further affect its severity and prognosis. Furthermore, there is a potential association between AKI and intensive care unit-acquired weakness (ICU-AW), specifically, AKI and AKI treated with kidney replacement therapy (AKI-KRT) may independently contribute to the development of ICU-AW. Potential pathophysiological mechanisms include altered amino acid and protein metabolism, inflammatory signaling, and deleterious removal of micronutrients by KRT [Citation38]. These studies all suggest that in critically ill patients, the relationship between AKI and nutritional status may be bidirectional.
Previous studies have rarely delved into the nutritional aspects of nutritional assessment tools in critically ill patients with acute heart failure and their potential influence on the development of acute kidney injury. Our research results indicate that GNRI performs remarkably well, comparable to CONUT and PNI, both of which have been proven highly effective in predicting AKI occurrence in patients. Additionally, because albumin, height, and weight are all easily obtainable indicators, GNRI can be conveniently used in clinical practice.
6. Strengths and limitations
Our study has several notable strengths. Firstly, to the best of our knowledge, research on the relationship between nutritional assessment tools and the occurrence of AKI in patients with acute heart failure (AHF) is limited. Our study revealed an association between lower GNRI levels and a higher incidence of AKI in AHF patients. This finding could potentially serve as a valuable theoretical foundation for clinicians in devising treatment strategies. Additionally, our study demonstrated the advantages of using nutritional indices, including simplicity, accessibility, speed, non-invasiveness, and high accuracy. However, there are certain limitations inherent to our study. Firstly, our research is a retrospective single-center study based on electronic medical records, introducing potential selection bias that may be unavoidable. Furthermore, as an observational study, our findings can only demonstrate correlation and cannot establish causality. Secondly, GNRI also has its limitations; it takes into account body weight, which might be influenced by fluid status and could underestimate malnutrition in obese patients. The identification of high nutritional risk individuals with heart failure would benefit from the integration of more precise nutritional markers. Thirdly, due to the reliance on ICD codes rather than criteria based on creatinine and urine output, the study was unable to evaluate the staging or severity of AKI. This constitutes a notable limitation of the dataset. Lastly, due to the constraints of our study design, we focused solely on analyzing the correlation between the initial nutritional index measurements taken upon admission and prognosis. We did not examine the dynamic interplay between nutritional indices and prognosis over time. Thus, further validation of the predictive efficacy of nutritional indices necessitates the conduct of multicenter prospective studies.
7. Conclusion
Our study findings suggest a correlation between GNRI and the occurrence of acute kidney injury in patients hospitalized with acute heart failure.
Statement of ethics
Institutional Review Board Statement: The MIMIC-IV database has received ethical approval from the Institutional Review Boards of the Massachusetts Institute of Technology and BIDMC. Because the database does not contain protected health information, this study did not require written informed consent for participation.
Authors’ contribution
SZ participated in data collection, processing, and analysis, writing of the article, literature search and screening. NC, ZH, NY participated in the formatting of the article, and XG,LM participated in the supervision and modification of the article. All authors have read and agreed to the published version of the manuscript.
Supplemental Material
Download Zip (324.4 KB)Disclosure statement
No potential conflict of interest was reported by the author(s).
Availability of data and materials
The datasets used and analyzed during the current study are available from the corresponding author upon reasonable request.
References
- Diebold M, Kozhuharov N, Wussler D, et al. Mortality and pathophysiology of acute kidney injury according to time of occurrence in acute heart failure. ESC Heart Fail. 2020;7(5):1–10. doi: 10.1002/ehf2.12788.
- Son HE, Moon JJ, Park JM, et al. Additive harmful effects of acute kidney injury and acute heart failure on mortality in hospitalized patients. Kidney Res Clin Pract. 2022;41(2):188–199. doi: 10.23876/j.krcp.21.111.
- De Santo NG, Cirillo M, Perna A, et al. The kidney in heart failure. Semin Nephrol. 2005;25(6):404–407. doi: 10.1016/j.semnephrol.2005.05.010.
- Xiong J, Yu Z, Huang Y, et al. Geriatric nutritional risk index and risk of mortality in critically ill patients with acute kidney injury: a multicenter cohort study. J Ren Nutr. 2023;33(5):639–648. doi: 10.1053/j.jrn.2023.06.004.
- Li H, Cen K, Sun W, et al. Prognostic value of geriatric nutritional risk index in elderly patients with heart failure: a meta-analysis. Aging Clin Exp Res. 2021;33(6):1477–1486. doi: 10.1007/s40520-020-01656-3.
- Bouillanne O, Morineau G, Dupont C, et al. Geriatric nutritional risk index: a new index for evaluating at-risk elderly medical patients. Am J Clin Nutr. 2005;82(4):777–783. doi: 10.1093/ajcn/82.4.777.
- Higuchi S, Kabeya Y, Matsushita K, et al. Urinary cast is a useful predictor of acute kidney injury in acute heart failure. Sci Rep. 2019;9(1):4352. doi: 10.1038/s41598-019-39470-1.
- Lin SM, Chang CH, Lin TY, et al. Plasma thrombomodulin levels are associated with acute kidney injury in patients with acute heart failure. Ann Med. 2022;54(1):3169–3176. doi: 10.1080/07853890.2022.2142660.
- Kahyaoglu M, Karaduman A, Geçmen Ç, et al. Serum lactate level may predict the development of acute kidney injury in acute decompensated heart failure. Turk Kardiyol Dern Ars. 2020;48(7):683–689. doi: 10.5543/tkda.2020.25679.
- Yang Z, Gong H, Kan F, et al. Association between the triglyceride glucose (TyG) index and the risk of acute kidney injury in critically ill patients with heart failure: analysis of the MIMIC-IV database. Cardiovasc Diabetol. 2023;22(1):232. doi: 10.1186/s12933-023-01971-9.
- Buzby GP, Mullen JL, Matthews DC, et al. Prognostic nutritional index in gastrointestinal surgery. Am J Surg. 1980;139(1):160–167. doi: 10.1016/0002-9610(80)90246-9.
- Kuroda D, Sawayama H, Kurashige J, et al. Controlling nutritional status (CONUT) score is a prognostic marker for gastric cancer patients after curative resection. Gastric Cancer. 2018;21(2):204–212. doi: 10.1007/s10120-017-0744-3.
- Chen L, Zhang S, Luo M, et al. Assessing the predictive value of different nutritional indexes for contrast-associated acute kidney injury in patients undergoing percutaneous coronary intervention. Circ J. 2023. doi: 10.1253/circj.CJ-23-0479.
- Liu CC, Liu PH, Chen HT, et al. Association of preoperative prognostic nutritional index with risk of postoperative acute kidney injury: a Meta-Analysis of observational studies. Nutrients. 2023;15(13):2929. doi: 10.3390/nu15132929.
- Driggin E, Cohen LP, Gallagher D, et al. Nutrition assessment and dietary interventions in heart failure: JACC review topic of the week. J Am Coll Cardiol. 2022;79(16):1623–1635. doi: 10.1016/j.jacc.2022.02.025.
- Jeejeebhoy KN. Malnutrition, fatigue, frailty, vulnerability, sarcopenia and cachexia: overlap of clinical features. Curr Opin Clin Nutr Metab Care. 2012;15(3):213–219. doi: 10.1097/MCO.0b013e328352694f.
- Anker SD, Ponikowski P, Varney S, et al. Wasting as independent risk factor for mortality in chronic heart failure. Lancet. 1997;349(9058):1050–1053. doi: 10.1016/S0140-6736(96)07015-8.
- Wawrzeńczyk A, Anaszewicz M, Wawrzeńczyk A, et al. Clinical significance of nutritional status in patients with chronic heart failure-a systematic review. Heart Fail Rev. 2019;24(5):671–700. doi: 10.1007/s10741-019-09793-2.
- Sze S, Pellicori P, Kazmi S, et al. Prevalence and prognostic significance of malnutrition using 3 scoring systems among outpatients with heart failure: a comparison with body mass index. JACC Heart Fail. 2018;6(6):476–486. doi: 10.1016/j.jchf.2018.02.018.
- Lin H, Zhang H, Lin Z, et al. Review of nutritional screening and assessment tools and clinical outcomes in heart failure. Heart Fail Rev. 2016;21(5):549–565. doi: 10.1007/s10741-016-9540-0.
- Ni J, Fang Y, Zhang J, et al. Predicting prognosis of heart failure using common malnutrition assessment tools: a systematic review and meta-analysis. Scott Med J. 2022;67(4):157–170. doi: 10.1177/00369330221122300.
- Kinugasa Y, Sota T, Kamitani H, et al. Diagnostic performance of nutritional indicators in patients with heart failure. ESC Heart Fail. 2022;9(4):2096–2106. doi: 10.1002/ehf2.13886.
- Xavier JG, Favero ME, Vinolo MAR, et al. Protein-energy malnutrition alters histological and ultrastructural characteristics of the bone marrow and decreases haematopoiesis in adult mice. Histol Histopathol. 2007;22(6):651–660. doi: 10.14670/HH-22.651.
- Sreenivasan J, Zhuo M, Khan MS, et al. Anemia (hemoglobin ≤13 g/dL) as a risk factor for contrast-Induced acute kidney injury following coronary angiography. Am J Cardiol. 2018;122(6):961–965. doi: 10.1016/j.amjcard.2018.06.012.
- Kalantar-Zadeh K, Stenvinkel P, Bross R, et al. Kidney insufficiency and nutrient-based modulation of inflammation. Curr Opin Clin Nutr Metab Care. 2005;8(4):388–396. doi: 10.1097/01.mco.0000172578.56396.9e.
- Seeliger E, Sendeski M, Rihal CS, et al. Contrast-induced kidney injury: mechanisms, risk factors, and prevention. Eur Heart J. 2012;33(16):2007–2015. doi: 10.1093/eurheartj/ehr494.
- Zhang F, Lu Z, Wang F. Advances in the pathogenesis and prevention of contrast-induced nephropathy. Life Sci. 2020;259:118379. doi: 10.1016/j.lfs.2020.118379.
- Fiaccadori E, Sabatino A, Barazzoni R, et al. ESPEN guideline on clinical nutrition in hospitalized patients with acute or chronic kidney disease. Clin Nutr. 2021;40(4):1644–1668. doi: 10.1016/j.clnu.2021.01.028.
- Sabatino A, Regolisti G, Karupaiah T, et al. Protein-energy wasting and nutritional supplementation in patients with end-stage renal disease on hemodialysis. Clin Nutr. 2017;36(3):663–671. doi: 10.1016/j.clnu.2016.06.007.
- Lan J, Xu G, Zhu Y, et al. Association of body mass index and acute kidney injury incidence and outcome: a systematic review and Meta-Analysis. J Ren Nutr. 2023;33(3):397–404. doi: 10.1053/j.jrn.2023.01.005.
- Sabaz MS, Aşar S, Sertçakacılar G, et al. The effect of body mass index on the development of acute kidney injury and mortality in intensive care unit: is obesity paradox valid? Ren Fail. 2021;43(1):543–555. doi: 10.1080/0886022X.2021.1901738.
- Xu S, Wu Z, Liu Y, et al. Influence of preoperative serum albumin on acute kidney injury after aortic surgery for acute type a aortic dissection: a retrospective cohort study. J Clin Med. 2023;12(4):1581. doi: 10.3390/jcm12041581.
- Yin T, Wei W, Huang X, et al. Serum total protein-to-albumin ratio predicts risk of death in septic acute kidney injury patients: a cohort study. Int Immunopharmacol. 2024;127:111358. doi: 10.1016/j.intimp.2023.111358.
- Chen JH, Zhang LW, Lin ZJ, et al. The association between the albumin-Bilirubin score and contrast-associated acute kidney injury in patients undergoing elective percutaneous coronary intervention. Angiology. 2024. doi: 10.1177/00033197241228051.
- Liang M, Ren X, Huang D, et al. The association between lactate dehydrogenase to serum albumin ratio and the 28-day mortality in patients with sepsis-associated acute kidney injury in intensive care: a retrospective cohort study. Ren Fail. 2023;45(1):2212080. doi: 10.1080/0886022X.2023.2212080.
- Wu X, Wang Y, Hu X. Association of weight loss with cardiovascular or all-cause mortality in patients with heart failure: a meta-analysis. Int J Obes (Lond). 2024;48(5):626–634. doi: 10.1038/s41366-024-01484-9.
- Karki S, Gajjar R, Bittar-Carlini G, et al. Association of hypoalbuminemia with clinical outcomes in patients admitted with acute heart failure. Curr Probl Cardiol. 2023;48(11):101916. doi: 10.1016/j.cpcardiol.2023.101916.
- Teixeira JP, Mayer KP, Griffin BR, et al. Intensive care unit-Acquired weakness in patients with acute kidney injury: a contemporary review. Am J Kidney Dis. 2023;81(3):336–351. doi: 10.1053/j.ajkd.2022.08.028.