Abstract
Background
Despite growing knowledge regarding the pathogenesis of autoimmune diseases (ADs) onset, the current treatment remains unsatisfactory. This study aimed to identify innovative therapeutic targets for ADs through various analytical approaches.
Research design and methods
Utilizing Mendelian randomization, Bayesian co-localization, phenotype scanning, and protein–protein interaction network, we explored potential therapeutic targets for 14 ADs and externally validated our preliminary findings.
Results
This study identified 12 circulating proteins as potential therapeutic targets for six ADs. Specifically, IL12B was judged to be a risk factor for ankylosing spondylitis (p = 1.61E − 07). TYMP (p = 6.28E − 06) was identified as a protective factor for ulcerative colitis. For Crohn’s disease, ERAP2 (p = 4.47E − 14), HP (p = 2.08E − 05), and RSPO3 (p = 6.52E − 07), were identified as facilitators, whereas FLRT3 (p = 3.42E − 07) had a protective effect. In rheumatoid arthritis, SWAP70 (p = 3.26E − 10), SIGLEC6 (p = 2.47E − 05), ISG15 (p = 3.69E − 05), and FCRL3 (p = 1.10E − 10) were identified as risk factors. B4GALT1 (p = 6.59E − 05) was associated with a lower risk of Type 1 diabetes (T1D). Interestingly, CTSH was identified as a protective factor for narcolepsy (p = 1.58E − 09) but a risk factor for T1D (p = 7.36E − 11), respectively. External validation supported the associations of eight of these proteins with three ADs.
Conclusions
Our integrated study identified 12 potential therapeutic targets for ADs and provided novel insights into future drug development for ADs.
1. Introduction
The development of autoimmune diseases (ADs) is caused by a decrease in the patient’s autoimmune tolerance, and the overproduction of autoantibodies and sensitized lymphocytes in the body, resulting in tissue damage [Citation1]. Despite the growing knowledge regarding the pathogenesis of ADs, its prevalence is persistently escalating at an alarming rate [Citation2]. Globally, ADs have become one of the leading causes of death, especially among young and middle-aged women, significantly affecting the labor force and the quality of life of patients and imposing a huge burden on society [Citation3]. Aiming at reducing inflammatory damage and relieving symptoms, nonsteroidal anti-inflammatory drugs (NSAIDs), glucocorticoids and conventional synthetic disease-modifying anti-rheumatic drugs (csDMARDs) are the current routine treatments for ADs [Citation4,Citation5]. However, some patients with AD cannot achieve clinical remission or even a clinical response after the current drug treatments [Citation2,Citation6]. In this case, biological disease-modifying anti-rheumatic drugs (bDMARDs) provide new options for patients with AD. However, in addition to their high cost, serious side effects are another drawback of the bDMARDs currently in use [Citation7]. For example, the tumor necrosis factor-α (TNF-α) inhibitors such as infliximab and adalimumab are current medications for ADs, but they can increase the risk of serious infections and tuberculosis in ADs patients [Citation8]. As the current treatment for ADs remains unsatisfactory, there is an urgent need to explore more innovative anti-rheumatic drugs with better efficacy and fewer side effects.
Human proteins are critical modulators of molecular pathways and the main drug targets [Citation9]. Despite rising investments in the pharmacy industry, 90% of innovative drugs are eliminated during clinical trials, which significantly increases the burden on healthcare [Citation10]. Advanced exploration of the relationship between potential protein drug targets and diseases from a genetic perspective can help reduce the failure rate and costs of research on new drugs [Citation11]. Owing to advances in plasma proteomics technology, Mendelian randomization (MR) analysis and Bayesian co-localization analysis have been applied to identify potential therapeutic targets for refractory diseases [Citation9].
MR uses exposure-associated single nucleotide polymorphisms (SNPs) as agents of exposure to explore the causalities between plasma proteome and ADs. SNPs involved in the MR analysis were also identified as instrumental variables (IVs). The basic rationale for MR is Mendel’s law of segregation: alleles are randomly assigned during conception, similar to a randomized controlled trial (RCT), in which patients are randomly allocated to an experimental group or control group [Citation12]. Observational studies tend to draw inaccurate conclusions due to confounding factors or reverse causality when examining the risk factors for clinical outcomes [Citation13]. The random assignment of genetic variants in MR can simulate the random assignment process of RCTs, thereby preventing interference from confounding factors and reverse causality [Citation14]. Besides, the results of MR analysis may be affected by linkage disequilibrium (LD), and Bayesian co-localization analysis is often used to remove the LD bias of MR [Citation15,Citation16].
Here, we obtained summary data on the plasma proteome and ADs from a recently published study and performed an integrated analysis to identify potential therapeutic targets for ADs.
2. Patients and methods
2.1. Study design and ethics
This study identified potential therapeutic targets for 14 autoimmune diseases, including ankylosing spondylitis (AS), systemic lupus erythematosus (SLE), ulcerative colitis (UC), Crohn’s disease (CD), rheumatoid arthritis (RA), narcolepsy, type 1 diabetes (T1D), alopecia areata, vitiligo, localized scleroderma, autoimmune thyroiditis, cutaneous vasculitis, polyarteritis nodosa, myasthenia gravis, and erythema nodosum. The overall flowchart of this study is presented in . First, we extracted IVs for plasma proteome and ADs from published study and public database and performed a primary MR analysis to investigate the causality between circulating proteins and ADs preliminarily. To ensure the robustness of our findings, we conducted multiple sensitivity analyses, including reverse MR analysis, Steiger filtering, Bayesian co-localization analysis, and phenotype scanning. Moreover, we graphed the protein-protein interaction (PPI) network between the significant circulating proteins and the therapeutic targets of currently used drugs for ADs. Furthermore, we validated our results by using alternative data on the plasma proteome and autoimmune diseases for external validation. The specific ethical approval was stated in original article of GWAS, and therefore, no further ethical permissions are required.
2.2. Data sources of plasma proteome and ADs
Protein quantitative trait loci (pQTL), which are genetic variants associated with protein expression levels, were utilized as IVs for the MR analysis of the plasma proteome. The pQTL statistics of plasma proteome used in the primary MR analysis were obtained from a study by Zheng et al. published in 2020 [Citation9]. To screen SNPs for the primary MR, the following criteria need to be met: (i) the pQTLs were cis-acting pQTLs and were not located within the major histocompatibility complex region (chr6, 26–34Mb); (ii) the pQTLs exhibited significant associations with the respective protein expression levels (p < 5E − 08); (iii) the pQTLs were not interfered by LD (r2<0.001) [Citation17]. Additionally, the pQTL statistics published by Ferkingstad et al. in the Decode database were utilized for external validation [Citation18]. The GWAS datasets for the ADs used in the primary and external validation stage were accessible through the IEU OpenGWAS project (https://gwas.mrcieu.ac.uk/) [Citation19–23], and further details were presented in Supplementary Table 1.
2.3. Primary MR analysis
After filtering according to the conditions listed above, 738 pQTLs for 734 proteins were included in the primary MR (Supplementary Table 2). To assess the strength of IVs, F-statistics were calculated for each pQTL using the formula F = β2exposure/SE2exposure [Citation12]. The F-statistics of all pQTLs were larger than 10, indicating that the causal inference of MR was not interfered by weak IV bias [Citation24]. For proteins with only one associated pQTL, the Wald ratio would be applied to analyze the association between that protein and the corresponding disease [Citation25]. For proteins with multiple associated pQTLs, the inverse-variance weighted model (IVW) was used, followed by pleiotropy test and heterogeneity analysis [Citation26]. Proteins identified as potential therapeutic targets would undergo subsequent sensitivity analysis and external validation, provided they meet the Bonferroni correction threshold (p < 0.05/734 = 6.81E − 05). In addition, it is necessary to note that the associations identified by MR are actually between mutations in the genes encoding these proteins and ADs, which means that the findings of MR need to be interpreted cautiously in a clinical context [Citation27]. The MR analysis was performed with ‘TwoSampleMR’ package (version 0.5.6) and R software (version 4.2.1).
2.4. Bayesian co-localization analysis
Bayesian co-localization analysis is commonly employed to determine whether two phenotypes are driven by the same causal variant by calculating the posterior probabilities of five hypotheses, thereby strengthening the evidence of association between the two phenotypes [Citation15]. Given that the findings of MR analysis are likely to be influenced by linkage disequilibrium, Bayesian co-localization analysis was performed using the coloc.abf function in ‘coloc’ package to remove the LD bias of MR [Citation15,Citation16]. A posterior probability of hypothesis 4 (PPH4) greater than or equal to 0.75 was considered as strong evidence of co-localization [Citation28].
2.5. Reverse causality detection
To avoid the interference of reverse causality, Steiger filtering was implemented to confirm the direction of causality between circulating proteins and ADs [Citation29]. Additionally, a reverse MR analysis between ADs and the identified significant proteins to strengthen the findings of Steiger filtering. Multiple models of MR, including IVW method, weighted median model, MR-Egger regression, simple mode, and weighted mode, were applied in the reverse MR analysis to investigate the presence of reverse causality [Citation30]. The significance threshold for reverse MR was set at p < 0.05.
2.6. Phenotype scanning
Phenotype scanning was performed with PhenoScanner V2 to determine whether the pQTLs of the identified proteins were pleiotropic [Citation31]. If a pQTL for a protein is associated with a risk factor for the corresponding AD (p < 5E − 08) and the relevant GWAS research was conducted within the European ethnic population, the causality between this specific protein and AD would be considered unreliable.
2.7. Protein–protein interaction analysis
To explore the potential interaction between the identified proteins and well-known medication targets for ADs, we obtained the therapeutic targets of ADs drugs currently in use from the Drugbank database (https://go.drugbank.com) and constructed the protein-protein interaction (PPI) network between the significant circulating proteins and the therapeutic targets for ADs using the STRING database (https://string-DB.org/) [Citation32,Citation33].
2.8. External validation
In the external validation phase, two approaches were applied to validate the findings of primary MR. Firstly, the GWAS datasets of ADs applied in primary MR were substituted with alternative data. Secondly, implementing the significant-variant strategy that use genome-wide significant pQTL as IV, we extracted IVs for plasma proteome in the pQTL statistics published by Ferkingstad et al. and replaced the datasets of both plasma proteome and ADs with the alternative data (Supplementary Table 1). The significance threshold for external validation was set at p < 0.05.
3. Results
3.1. Results of primary MR analysis
This study aimed to investigate the association between 734 circulating proteins and 14 ADs. After filtering according to rigorous criteria mentioned above, 738 cis-pQTLs for 734 proteins were included in the primary MR analysis. Out of these, 34 protein-AD pairs met the criteria for significance after Bonferroni correction (p < 6.81E − 05) (, and Citation3). Since most proteins had only one corresponding cis-pQTL, certain sensitivity analyses including alternative MR algorithms, heterogeneity analysis, and pleiotropy detection were not applicable. However, the utilization of cis-pQTLs as IVs has the potential to reduce the risk of horizontal pleiotropy to some extent [Citation34]. Some of the significant proteins identified by the primary MR are known targets of currently marketed drugs for ADs, which could prove the credibility of our study. For instance, interleukin-23 receptor (IL23R) is targeted by ustekinumab in the treatment of CD.
Figure 2. Forest plot of the primary MR analysis. ADs, autoimmune diseases; AS, ankylosing spondylitis; SLE, systemic lupus erythematosus; UC, ulcerative colitis; CD, Crohn’s disease; RA, rheumatoid arthritis; T1D, type 1 diabetes.
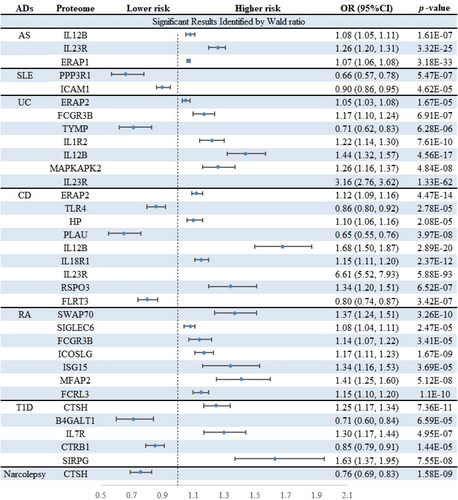
Figure 3. Volcano plots of primary MR analysis. A, B, C, D, E, F, and G show primary MR with Wald ratio method on circulating proteins on the risk of ADs. OR for increased risk of ADs were expressed as per SD increase in circulating protein levels. The black horizontal dashed line represents the filtering threshold for Bonferroni correction. ln = natural logarithm; PVE = proportion of variance explained.
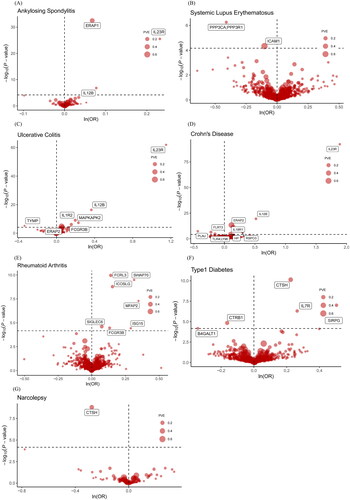
Table 1. Results of primary MR analysis after bonferroni correction.
3.2. Bayesian co-localization and reverse causality detection
Among the 34 protein-AD pairs, 26 pairs passed the Steiger filtering test and reverse MR analysis (Supplementary Tables 3 and 4). And 13 pairs survived the subsequent Bayesian co-localization analysis (). Specifically, interleukin-12 subunit beta (IL12B) was judged to be a risk factor for AS (OR = 1.08; 95%CI, 1.05–1.11; p = 1.61E − 07). Thymidine phosphorylase (TYMP) (OR = 0.71; 95%CI, 0.62–0.83; p = 6.28E − 06) was identified as a protective factor for UC. And we found endoplasmic reticulum aminopeptidase 2 (ERAP2) (OR = 1.12; 95%CI, 1.09–1.16; p = 4.47E − 14), haptoglobin (HP) (OR = 1.10; 95%CI, 1.06–1.16; p = 2.08E − 05), and R-spondin-3 (RSPO3) (OR = 1.34; 95%CI, 1.20–1.51; p = 6.52E − 07), all had a facilitating effect on CD, whereas fibronectin leucine rich transmembrane protein 3 (FLRT3) (OR = 0.80; 95%CI, 0.74–0.87; p = 3.42E − 07) had a protective effect. SWAP-70 protein (SWAP70) (OR = 1.37; 95%CI, 1.24–1.51; p = 3.26E − 10), sialic acid-binding Ig-like lectin 6 (SIGLEC6) (OR = 1.08; 95%CI, 1.04–1.11; p = 2.47E − 05), ubiquitin-like protein ISG15 (ISG15) (OR = 1.34; 95%CI, 1.16–1.53; p = 3.69E − 05), and Fc receptor-like protein 3 (FCRL3) (OR = 1.15; 95%CI, 1.10–1.20; p = 1.10E − 10) were judged to be risk factors for RA. Beta-1,4-galactosyltransferase 1 (B4GALT1) (OR = 0.71; 95%CI, 0.60–0.84; p = 6.59E − 05) was associated with a lower risk of T1D. Interestingly, pro-cathepsin H (CTSH) was identified as both a protective factor for narcolepsy (OR = 0.76; 95%CI, 0.69–0.83; p = 1.58E − 09) and a risk factor for T1D (OR = 1.25; 95%CI, 1.17–1.34; p = 7.36E − 11). Notably, the PPH4 values for TYMP-UC (PPH4 = 0.989), FLRT3-CD (PPH4 = 0.965), SWAP70-RA (PPH4 = 0.989), FCRL3-RA (PPH4 = 0.994), CTSH-T1D (PPH4 = 0.999), B4GALT1-T1D (PPH4 = 0.964), and CTSH-narcolepsy (PPH4 = 0.999) all exceeded 0.95, implying that the causalities between these identified proteins and ADs are strongly supported by evidence from co-localization analysis.
Table 2. Summary of reverse MR analysis, steiger filting, and Bayesian co-localization analysis.
3.3. Phenotype scanning
Phenotype scanning was performed to investigate whether the pQTLs of the identified proteins were pleiotropic and details were listed in Supplementary Table 5. No pleiotropy was detected among the 13 protein-AD pairs that passed the primary MR analysis, reverse causality detection, and Bayesian co-localization. Specifically, TYMP showed causal for certain blood cell traits such as mean corpuscular volume and mean corpuscular hemoglobin. ERAP2 was associated with diastolic blood pressure. HP showed causality for self-reported high cholesterol, height, left hand grip strength, and hematocrit. RSPO3 was linked to heel bone mineral density, waist hip ratio, waist circumference, and some blood cell traits. SWAP70 was revealed to be associated with coronary artery disease, hypertension, and some blood cell traits. SIGLEC6 was found to be related to blood protein levels. FCRL3 was associated with T1D. CTSH was discovered to be correlated with narcolepsy. Additionally, no traits were found to be associated with FLRT3, ISG15, and B4GALT1.
3.4. Interaction between identified proteins and drug targets of ADs
The PPI network of the significant circulating proteins and current therapeutic targets for ADs drugs was generated using STRING database (Supplementary Table 6 and Supplementary Figures 1–7). Among the 13 protein–AD pairs that survived all previous tests, the AS-associated protein IL-12B was found to interact with tumor necrosis factor (TNF), which is the therapeutic target of infliximab. Clinical trials have shown that ustekinumab, targeting IL12B and IL23R, is effective in treating AS, although it is not yet approved by the United States Food and Drug Administration [Citation35]. The CD-associated protein HP showed causality for TNF and IL23R, the therapeutic targets of infliximab and ustekinumab, respectively. For the RA-associated protein, SIGLEC6 was linked to Toll-like receptor 9 (TLR9), which is the target of chloroquine and hydroxychloroquine. Besides, ISG15 was associated with TLR9, nuclear factor NF-kappa-B p105 subunit (NFKB1), and G1/S-specific cyclin-D1 (CCND1), the targets of hydroxychloroquine, sulfasalazine, and aspirin. What’s more, FCRL3 was suggested to be correlated with cytotoxic T-lymphocyte protein 4 (CTLA4), which is the target of abatacept, and B-lymphocyte antigen CD20 (MS4A1), the target of rituximab.
3.5. External validation
In the external validation stage, the causalities between plasma proteome and ADs were tested with the alternative datasets (, Supplementary Tables 7 and 8). Among the 13 protein–AD pairs, the effects of 8 proteins on ADs were successfully replicated, including CD-associated proteins ERAP2, HP, RSPO3, and FLRT3, RA-associated proteins SIGLEC6 and FCRL3, and T1D-associated proteins CTSH and B4GALT1.
4. Discussion
We performed an integrative analysis to explore the causal effects of 734 circulating proteins on 14 types of ADs, which could offer preclinical leads for novel drugs exploration for ADs. Collectively, 13 proteins passed the bi-directional MR analysis, Steiger filtering, Bayesian co-localization, and phenotype scanning, eight of which survived the external validation, further demonstrating the credibility of our findings. In this part, we mainly focus on the effects of these eight robust plasma proteins on ADs.
Previous research has identified ERAP2 as a susceptibility locus for CD from the perspective of expression quantitative trait loci (eQTL), and our study further confirmed that circulating ERAP2 protein is a risk factor for CD from the perspective of pQTL [Citation36]. CD is an inflammatory disease of the intestinal tract that arises when the immune system attacks beneficial bacteria in the gut, resulting in destructive inflammation [Citation5]. ERAP2 is involved in the production of most human leukocyte antigen class I-binding peptides and plays an essential role in antigen presentation, contributing to the ability of the immune system to recognize and clear invasive foreign antigens [Citation37]. Just as there are two sides to every coin, so is the overly dutiful ERAP2. Studies have shown that increased expression of ERAP2 reduces the risk of serious infections, such as sepsis, but it can also be overly dutiful and indiscriminate, thus leading to the development of CD [Citation37,Citation38]. Thus, ERAP2 has the potential to be a therapeutic target for CD.
In addition, despite the lack of support from co-localization evidence, our primary MR analysis and external validation identified circulating endoplasmic reticulum aminopeptidase 1 (ERAP1) as a risk factor for AS, which is consistent with the findings of previous research [Citation39]. Studies have identified selective inhibitors of ERAP1 and ERAP2, and clinical trials on the safety, tolerability, and efficacy of GRWD5769 (an inhibitor of ERAP1) for the treatment of multiple solid tumors are underway [Citation40,Citation41]. Whether ERAP1 and ERAP2 can be used as potential therapeutic targets for ADs warrants further investigation.
Among CD-associated proteins, RSPO3 was identified as a risk factor for CD in our integrated analysis. A previous meta-analysis of GWAS also recognized an SNP within the RSPO3 gene as a susceptibility locus for CD [Citation42]. It has been found that the impaired mucosal barrier and inflammatory response in the intestine of patients with CD are associated with abnormal activation of the Wnt signaling pathway. RSPO3 enhances Wnt signaling by binding to LGR proteins and activating co-receptors of the Wnt pathway, such as Frizzled receptors [Citation43]. In addition, the core events of the Wnt signaling pathway are the stabilization and nuclear translocation of β-catenin. RSPO3 can inhibit the degradation of β-catenin and then allow the accumulated β-catenin to enter the nucleus, thus enhancing the Wnt signaling pathway [Citation44]. Currently, therapeutic agents targeting RSPO3 used to treat ovarian, pancreatic, and colon cancers are in clinical trials, increasing the feasibility of developing RSPO3 as a therapeutic target for CD [Citation45].
Our integrative study identified circulating FCRL3 level as a risk factor for RA. An imbalance between helper T cells (Th17) and regulatory T cells (Tregs) plays a vital role in RA [Citation46]. The level of IL17 secreted by Th17s is obviously elevated in patients with RA, which enhances the invasiveness of synovial cells and the expression of other pro-inflammatory cytokines [Citation47]. Additionally, the number and function of Tregs may be impaired in patients with RA, leading to an excessive auto-attack on the immune system and further promoting the development of RA [Citation48]. Retinoic acid-related orphan receptors-γt (RORγt) is a critical transcription factor of Th17, which dominates the translation of Th17-associated genes such as IL-17A [Citation49]. Studies have shown that FCRL3 can stimulate Tregs to express RORγt and shift to a Th17-like phenotype, as evidenced by the secretion of Th17-related pro-inflammatory cytokines including IL-17, IL-26 and IFN-γ [Citation50]. In addition, our PPI analysis revealed interactions between FCRL3 and CTLA4 (the target of Abatacept), or MS4A1 (the target of rituximab). Therefore, regulating the Th17/Treg balance and treating RA by targeting FCRL3 may be a promising strategy.
In our study, CTSH was also identified as a risk factor for T1D. The attack of the immune system on pancreatic β-cells and the impaired ability of insulin production are the pathogeneses of T1D [Citation51]. Toll-like receptor 3 (TLR3) helps the immune system recognize viral infections. As an endopeptidase, CTSH can shear the N-terminus of TLR3 in the pancreas and enhance its functionality [Citation52]. Increased CTSH expression may lead to abnormal activation of TLR3 and overreaction to viral infection, thereby triggering abnormal immune system attack on pancreatic β-cells and increasing the risk of T1D [Citation53]. Furthermore, the release of type I interferon also increases during this process. Type I interferon could increase pro-inflammatory cytokines production and enhance the ability of macrophages and natural killer cells to attack pancreatic β-cells, which exacerbates the inflammatory environment around pancreatic β-cells and accelerates their destruction [Citation54]. Notably, CTSH inhibitors have now been shown to treat bladder cancer. Thus, further studies on CTSH inhibitors targeting T1D should be conducted [Citation55].
Interestingly, CTSH was also identified as a protective factor against narcolepsy in our integration study, which is in line with the findings of Faraco et al. [Citation21]. However, the mechanism underlying the effects of CTSH on narcolepsy remains unclear and requires further investigation.
Among the eight pairs of protein–ADs that survived all previous tests, HP had a facilitating effect on CD, FLRT3 was associated with a lower risk of CD, SIGLEC6 was identified as a risk factor for RA, and B4GALT1 had a protective effect against T1D. No previous studies have examined the relationship between these circulating proteins and ADs. Our study is the first to identify the associations between these proteins and their corresponding ADs. Thus, our study may provide novel insights into the therapeutic target identification of these ADs.
Our study had several strengths. To the best of our knowledge, this is the first integrated study to identify potential therapeutic targets in ADs using a large-scale plasma proteomic dataset. Multiple methods, including bidirectional MR analysis, Bayesian co-localization, and PPI networks were integrated in the present study, and our findings were externally validated using alternative datasets. Secondly, our integrated study identified eight promising drug targets. Modulators of some proteins have been developed and considered for the treatment of tumors and other diseases, which boosts our confidence in developing these promising proteins as targets for ADs.
The present study has some limitations. Most of the proteins had only one corresponding pQTL, and some sensitivity analyses, including alternative MR algorithms, heterogeneity analysis, and pleiotropy detection, could not be performed because of the insufficient number of pQTLs. In addition, our study was mainly based on populations of European origin, which limits the generalizability of our findings. Third, the causality identified by MR is actually between mutations in the genes encoding these proteins and ADs, which means that the MR findings need to be interpreted cautiously in the clinical context. Moreover, to reduce the failure rate of drug target screening and the cost of subsequent animal experiments, we set up a rigorous screening process for drug targets, which may lead to the mistaken elimination of some known targets of ADs. The number of proteins in the GWAS data we obtained were limited, which also resulted in the omission of some known therapeutic targets. Finally, our results must be verified through further clinical and basic research.
5. Conclusions
Our integrated research identified twelve potential therapeutic targets for ADs and provided novel insights into future mechanistic studies for the treatment of ADs.
Author’s contributions
PS and QBJ designed the study. QBJ and FHR performed the computations and manuscript writing. All authors contributed to the article and approved the submitted version. QBJ and FHR contributed equally to this work and share first authorship.
Ethics approval and consent to participate
The GWAS data employed in the present research are publicly available on the database website. The specific ethical approval was expressed in the original GWAS article, so further ethical approval is not required.
Supplemental Material
Download Zip (9.8 MB)Acknowledgments
We would like to appreciate the participants and researchers of the IEU OpenGWAS project and FinnGen study. In addition, we want to acknowledge Figdraw (https://www.figdraw.com) for their assistance in .
Disclosure statement
No potential conflict of interest was reported by the author(s).
Data availability statement
Summary statistics of ADs can be downloaded from IEU consortium (https://gwas.mrcieu.ac.uk/) and the GWAS ID was presented in Supplementary Table 1. The pQTL statistics of plasma proteome are publicly available on the Decode database (https://download.decode.is/form/folder/proteomics).
Further inquiries can be directed to the corresponding author.
Additional information
Funding
References
- Li Y, Ming M, Li C, et al. The emerging role of the hedgehog signaling pathway in immunity response and autoimmune diseases. Autoimmunity. 2023;56(1):1.
- Fugger L, Jensen LT, Rossjohn J. Challenges, progress, and prospects of developing therapies to treat autoimmune diseases. Cell. 2020;181(1):63–10.
- Rogers MAM, Wei MY, Kim C, et al. Sex differences in autoimmune multimorbidity in type 1 diabetes mellitus and the risk of cardiovascular and renal disease: a longitudinal study in the United States, 2001-2017. J Womens Health (Larchmt). 2020;29(4):511–519.
- Fraenkel L, Bathon JM, England BR, et al. 2021 American college of rheumatology guideline for the treatment of rheumatoid arthritis. Arthritis Care Res (Hoboken). 2021;73(7):924–939.
- Nakase H, Uchino M, Shinzaki S, et al. Evidence-based clinical practice guidelines for inflammatory bowel disease 2020. J Gastroenterol. 2021;56(6):489–526.
- Lamberg T, Sipponen T, Valtanen S, et al. Short interruptions of TNF-inhibitor treatment can be associated with treatment failure in patients with immune-mediated diseases. Autoimmunity. 2022;55(5):275–284.
- Marinho A, Delgado Alves J, Fortuna J, et al. Biological therapy in systemic lupus erythematosus, antiphospholipid syndrome, and Sjögren’s syndrome: evidence- and practice-based guidance. Front Immunol. 2023;14:1117699.
- Atzeni F, Gianturco L, Talotta R, et al. Investigating the potential side effects of anti-TNF therapy for rheumatoid arthritis: cause for concern? Immunotherapy. 2015;7(4):353–361.
- Zheng J, Haberland V, Baird D, et al. Phenome-wide mendelian randomization mapping the influence of the plasma proteome on complex diseases. Nat Genet. 2020;52(10):1122–1131.
- Plenge RM, Scolnick EM, Altshuler D. Validating therapeutic targets through human genetics. Nat Rev Drug Discov. 2013;12(8):581–594.
- Nelson MR, Tipney H, Painter JL, et al. The support of human genetic evidence for approved drug indications. Nat Genet. 2015;47(8):856–860.
- Emdin CA, Khera AV, Kathiresan S. Mendelian randomization. JAMA. 2017;318(19):1925–1926.
- Smith GD, Ebrahim S. Mendelian randomization’: can genetic epidemiology contribute to understanding environmental determinants of disease? Int J Epidemiol. 2003;32(1):1–22.
- Li Y, Li Q, Cao Z, et al. The causal association of polyunsaturated fatty acids with allergic disease: a two-sample mendelian randomization study. Front Nutr. 2022;9:962787.
- Giambartolomei C, Vukcevic D, Schadt EE, et al. Bayesian test for colocalisation between pairs of genetic association studies using summary statistics. PLoS Genet. 2014;10(5):e1004383.
- Gu X, Dou M, Su W, et al. Identifying novel proteins underlying schizophrenia via integrating pQTLs of the plasma, CSF, and brain with GWAS summary data. BMC Med. 2022;20(1):474.
- Lin J, Zhou J, Xu Y. Potential drug targets for multiple sclerosis identified through mendelian randomization analysis. Brain. 2023;146(8):3364–3372.
- Kurilshikov A, Medina-Gomez C, Bacigalupe R, et al. Large-scale association analyses identify host factors influencing human gut microbiome composition. Nat Genet. 2021;53(2):156–165.
- Hom G, Graham RR, Modrek B, et al. Association of systemic lupus erythematosus with C8orf13-BLK and ITGAM-ITGAX. N Engl J Med. 2008;358(9):900–909.
- Cortes A, Hadler J, Pointon JP, et al. Identification of multiple risk variants for ankylosing spondylitis through high-density genotyping of immune-related loci. Nat Genet. 2013;45(7):730–738.
- Faraco J, Lin L, Kornum BR, et al. ImmunoChip study implicates antigen presentation to T cells in narcolepsy. PLoS Genet. 2013;9(2):e1003270.
- Okada Y, Wu D, Trynka G, et al. Genetics of rheumatoid arthritis contributes to biology and drug discovery. Nature. 2014;506(7488):376–381.
- Bentham J, Morris DL, Graham DSC, et al. Genetic association analyses implicate aberrant regulation of innate and adaptive immunity genes in the pathogenesis of systemic lupus erythematosus. Nat Genet. 2015;47(12):1457–1464.
- Burgess S, Thompson SG, CRP CHD Genetics Collaboration. Avoiding bias from weak instruments in mendelian randomization studies. Int J Epidemiol. 2011;40(3):755–764.
- Qin C, Diaz-Gallo LM, Tang B, et al. Repurposing antidiabetic drugs for rheumatoid arthritis: results from a two-sample mendelian randomization study. Eur J Epidemiol. 2023;38(7):809–819.
- Burgess S, Butterworth A, Thompson SG. Mendelian randomization analysis with multiple genetic variants using summarized data. Genet Epidemiol. 2013;37(7):658–665.
- Wang Q, Jia S, Lu Q, et al. Systemic lupus erythematosus is associated with the risk of coeliac disease: a mendelian randomisation study. Autoimmunity. 2023;56(1):2250103.
- Kia DA, Zhang D, Guelfi S, et al. Identification of candidate Parkinson disease genes by integrating genome-wide association study, expression, and epigenetic data sets. JAMA Neurol. 2021;78(4):464–472.
- Hemani G, Tilling K, Davey Smith G. Orienting the causal relationship between imprecisely measured traits using GWAS summary data. PLoS Genet. 2017;13(11):e1007081.
- Bowden J, Davey Smith G, Burgess S. Mendelian randomization with invalid instruments: effect estimation and bias detection through egger regression. Int J Epidemiol. 2015;44(2):512–525.
- Kamat MA, Blackshaw JA, Young R, et al. PhenoScanner V2: an expanded tool for searching human genotype-phenotype associations. Bioinformatics. 2019;35(22):4851–4853.
- Szklarczyk D, Gable AL, Nastou KC, et al. The STRING database in 2021: customizable protein-protein networks, and functional characterization of user-uploaded gene/measurement sets. Nucleic Acids Res. 2021;49(D1):D605–D612.
- Szklarczyk D, Kirsch R, Koutrouli M, et al. The STRING database in 2023: protein-protein association networks and functional enrichment analyses for any sequenced genome of interest. Nucleic Acids Res. 2023;51(D1):D638–D646.
- Ren F, Jin Q, Liu T, et al. Proteome-wide mendelian randomization study implicates therapeutic targets in common cancers. J Transl Med. 2023;21(1):646.
- Paine A, Ritchlin CT. Targeting the interleukin-23/17 axis in axial spondyloarthritis. Curr Opin Rheumatol. 2016;28(4):359–367.
- Franke A, McGovern DP, Barrett JC, et al. Genome-wide meta-analysis increases to 71 the number of confirmed crohn’s disease susceptibility loci. Nat Genet. 2010;42(12):1118–1125.
- Hamilton F, Mentzer AJ, Parks T, et al. Variation in ERAP2 has opposing effects on severe respiratory infection and autoimmune disease. Am J Hum Genet. 2023;110(4):691–702.
- Klunk J, Vilgalys TP, Demeure CE, et al. Evolution of immune genes is associated with the black death. Nature. 2022;611(7935):312–319.
- Wordsworth BP, Cohen CJ, Davidson C, et al. Perspectives on the genetic associations of ankylosing spondylitis. Front Immunol. 2021;12:603726.
- Maben Z, Arya R, Rane D, et al. Discovery of selective inhibitors of endoplasmic reticulum aminopeptidase 1. J Med Chem. 2020;63(1):103–121.
- Camberlein V, Fléau C, Sierocki P, et al. Discovery of the first selective nanomolar inhibitors of ERAP2 by kinetic target-guided synthesis. Angew Chem Int Ed Engl. 2022;61(39):e202203560.
- Jostins L, Ripke S, Weersma RK, et al. Host-microbe interactions have shaped the genetic architecture of inflammatory bowel disease. Nature. 2012;491(7422):119–124.
- Jin YR, Yoon JK. The R-spondin family of proteins: emerging regulators of WNT signaling. Int J Biochem Cell Biol. 2012;44(12):2278–2287.
- Kang E, Yousefi M, Gruenheid S. R-Spondins are expressed by the intestinal stroma and are differentially regulated during Citrobacter rodentium- and DSS-Induced colitis in mice. PLoS One. 2016;11(4):e0152859.
- Chartier C, Raval J, Axelrod F, et al. Therapeutic targeting of tumor-derived R-Spondin attenuates β-catenin signaling and tumorigenesis in multiple cancer types. Cancer Res. 2016;76(3):713–723.
- Tan L, Ouyang T, Li X, et al. Serum sirtuin-1 a potential marker in the diagnosis of rheumatoid arthritis. Autoimmunity. 2023;56(1):2181234.
- Abd-Allah AR, Ahmad SF, Alrashidi I, et al. Involvement of histamine 4 receptor in the pathogenesis and progression of rheumatoid arthritis. Int Immunol. 2014;26(6):325–340.
- Meyer A, Yan S, Golumba-Nagy V, et al. Kinase activity profiling reveals contribution of G-protein signaling modulator 2 deficiency to impaired regulatory T cell migration in rheumatoid arthritis. J Autoimmun. 2021;124:102726.
- Sun L, Fu J, Zhou Y. Metabolism controls the balance of Th17/T-regulatory cells. Front Immunol. 2017;8(:1632.
- Agarwal S, Kraus Z, Dement-Brown J, et al. Human Fc receptor-like 3 inhibits regulatory T cell function and binds secretory IgA. Cell Rep. 2020;30(5):1292–1299.e3.
- Krischer JP, Liu X, Vehik K, et al. Predicting islet cell autoimmunity and type 1 diabetes: an 8-year TEDDY study progress report. Diabetes Care. 2019;42(6):1051–1060.
- Qi R, Singh D, Kao CC. Proteolytic processing regulates toll-like receptor 3 stability and endosomal localization. J Biol Chem. 2012;287(39):32617–32629.
- Inshaw JRJ, Cutler AJ, Crouch DJM, et al. Genetic variants predisposing most strongly to type 1 diabetes diagnosed under age 7 years lie near candidate genes that function in the immune system and in pancreatic β-cells. Diabetes Care. 2020;43(1):169–177.
- Apaolaza PS, Balcacean D, Zapardiel-Gonzalo J, et al. Islet expression of type I interferon response sensors is associated with immune infiltration and viral infection in type 1 diabetes. Sci Adv. 2021;7(9):eabd6527.
- Wang M, Chen X, Tan P, et al. Acquired semi-squamatization during chemotherapy suggests differentiation as a therapeutic strategy for bladder cancer. Cancer Cell. 2022;40(9):1044–1059.e8.