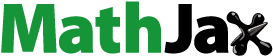
Abstract
It is often claimed that the structure of networks influences outcomes in environmental governance. For example, network motifs of social-ecological fit have been linked to positive environmental outcomes, but empirical tests of this link are rare. Social-ecological network fit represents a situation in which actors involved in the governance and management of linked ecological elements coordinate. We empirically analyze how motifs of social-ecological network fit are associated with actors’ outcome assessments in ten cases of Swiss wetlands governance. We combine social networks among organizational actors, networks of interrelated ecosystem management activities, and actors’ assessments of outcomes. Results show that – contrary to the prominent theoretical claim – more fit is linked to worse outcomes. Drawing on the so-called risk hypothesis, we argue that our negative findings likely highlight a complicated causal process between actors’ assessments of outcomes and their adjustment to risks through coordination in networks.
Introduction
Societies have been facing the destruction of ecosystems around the world, which, among other consequences, also threatens human well-being (Reid et al. Citation2005). The concept of social-ecological systems (SES) emphasizes the interdependencies of social and ecological systems. Improving the governance of SESs is key to sustainable development and, more specifically, to the protection and restoration of ecosystems. The governance of SESs can involve activities such as regulating the harvesting of fish stocks in rivers, lakes, or the sea or managing the recreational use of forests, wetlands, or mountain areas. The idea of social-ecological fit claims that environmental issues can generally not be addressed with one-size-fits-all institutional designs, but that governance, in order to be successful, needs to take into account specific contingencies of the underlying environmental issues (e.g., Ostrom (Citation1990)).
Social-ecological networks (SEN) that capture both social networks and ecological networks, as well as social-ecological interdependencies, have been proposed to operationalize and study the governance of SESs (Bodin et al. Citation2014; Barnes et al. Citation2019; Felipe-Lucia et al. Citation2022). SEN literature has proposed a very specific, network-focused conceptualization of social-ecological fit (Bergsten, Galafassi, and Bodin Citation2014; Epstein et al. Citation2015; Bodin et al. Citation2019), according to which collaborative interactions among actors should align with the structure of ecological networks (also “social-ecological alignment”, see Barnes et al. (Citation2019). Because in such a specific situation of fit, actors can take into account underlying dependencies in the ecological system, the presence of motifs of SEN fit is claimed to lead to positive outcomes (e.g., Bodin et al. (Citation2019). Motifs of fit are closed network structures that connect the social and ecological network levels, thereby representing social-ecological alignment. Also, beyond the literature on SEN, the literature claims that the structures of social networks influence network outcomes (Ostrom Citation1990; Provan and Kenis Citation2007; Lubell and Morrison Citation2021).
In recent years, there has been a strong increase in the number of studies dealing with SENs and network motifs of SEN fit, more specifically. The presence of motifs of fit in SENs has been claimed to be a positive outcome per se (e.g., Bodin et al. (Citation2019)), and the presence of fit motifs in SENs has been empirically studied in many different contexts (see, e.g., Sayles et al. (Citation2019). By contrast, only few studies have empirically assessed the link between SEN fit and outcomes (Bodin and Tengö Citation2012; Guerrero et al. Citation2015; Bodin et al. Citation2016; Widmer et al. Citation2019), be it at the individual level (e.g., actor success (Scott and Thomas Citation2017)), collective level (better decisions (Guerrero et al. Citation2015)) or ecological level (reef fish biomass (Barnes et al. Citation2019)). Given the prominence of the hypotheses of a link between network structures – and network fit in SESs more specifically – and outcomes and the rarity of empirical studies actually assessing that link, we ask: How do network motifs of social-ecological fit relate to outcomes?
To answer this question, we rely on a multi-case study of ten cases of wetland governance in Switzerland. The respective networks include information on (a) actors responsible for the governance of wetlands and their collaboration networks, (b) ecosystem management activities and their interdependencies, (c) actors’ links to ecosystem management activities, and (d) actors’ assessment of governance outcomes. The empirical information was gathered through a combination of document analysis, expert interviews, and online surveys. In line with our conceptualization of SEN fit, we then identify well-defined motifs representing such fit – closed triangles and four-cycles – and include them as independent variables in a multi-level model to explain outcome assessments.
With this article, we contribute to the literature in three ways. First, we propose one specific way to empirically link SEN motifs to outcomes, enabling us to test a prominent hypothesis in the literatures on networks, network governance, and SENs. Most existing research on SENs does not include empirical data that allows for assessing the link between network patterns and governance outcomes (Bodin et al. Citation2019; Kluger et al. Citation2020). Second, we link the arguments around SENs to arguments from governance networks. More specifically, the risk-hypothesis (Berardo and Scholz Citation2010) that we propose adapting to SENs suggests that actors form their networks depending on how they perceive the status of given issues they are dealing with, forming looser structures around low-risk and more closed structures around high-risk issues. Our results suggest that the causal pathway between network structure and governance outcomes is likely characterized by the simultaneous presence of adjusting to risk and forming performant structures. Third, our analysis goes beyond the study settings of most SEN studies that focus on single case studies (Bodin et al. Citation2019; Sayles et al. Citation2019; Kluger et al. Citation2020) and accounts for potential differences across ten cases of wetland governance.
Theory
Complex governance settings such as those related to SES governance are characterized by multiple interdependent environmental issues (Hedlund, Bodin, and Nohrstedt Citation2021), many parallel institutions and venues (Lubell Citation2013), and high levels of uncertainty (Burch et al. Citation2019). The SES framework by Ostrom (Citation2007) emphasizes collaborative, polycentric governance arrangements as a self-organizing system of many autonomous units that are in contact through processes such as collaboration, competition, or conflict (Ostrom Citation2007). Network theory provides conceptual and operationalizable background for analyzing SES governance and related actor interactions (Wasserman and Faust Citation1994; Lubell et al. Citation2012; Scott and Ulibarri Citation2019; Kluger et al. Citation2020). Network approaches allow capturing the complex interdependencies among actors, among environmental issues, as well as across both actors and issues.
One approach utilizing networks to analyze both social and ecological networks in SESs is the SEN concept (Bodin et al. Citation2016; Felipe-Lucia et al. Citation2022). The SEN concept builds on distinct social and environmental entities connected within and across network levels. Environmental entities can represent resources, ecosystem services, or biophysical characteristics of an SES. While original studies on SENs have used ecological entities such as forest patches or species as ecological network nodes, more recent SEN analyses have focused on more abstract “ecological” nodes as environmental issues or tasks (Bodin et al. Citation2019). For example, (Bodin et al. Citation2022) conceptualize “ecological nodes” as different tasks related to wildfire management (e.g., evacuation or logistics), while Hedlund, Bodin, and Nohrstedt (Citation2021) or Huber (Citation2022) rely on water-related policy issues such as water quality, eutrophication, or invasive species management. The advantage of this more abstract conceptualization of ecological networks is that it allows capturing the “ecological system” on an abstraction level directly relevant to actors engaging in different kinds of governance activities (and not, e.g., based on bird movement patterns between forest patches only).
Social-Ecological Fit in Social-Ecological Networks
The general theory of fit between ecosystems and the institutional arrangements set up for their governance (usually seen as normatively desirable) is well-established in the literature on natural resource governance (Folke et al. Citation2007). The main theoretical claim is that the governance of SESs should be aligned with the ecological dimensions and that different governance arrangements might be required depending on the underlying ecological challenges (Ostrom Citation1990). The SEN literature has adapted the idea of social-ecological fit to networks and has defined SEN fit in a specific way: SEN fit (also “social-ecological alignment”, see (Barnes et al. Citation2022) represents a situation where two actors that both have a tie to the same or two connected ecological entities would need to be connected in order to create fit (Folke et al. Citation2007; Lebel et al. Citation2013; Bodin et al. Citation2016; Widmer et al. Citation2019) (see ). Thus, the application of the fit idea to SENs does not emphasize how different governance arrangements might fit with different ecological challenges, but posits that given SEN motifs represent situations of fit, whereas other open motifs represent misfit. An example of SEN fit would be given by, e.g., two tourism operators that both provide river excursions in the same wetland area and collaborate, as such collaboration can help distribute the visiting tourists better over one day and across different river parts, and thus prevent traffic jams during peak hours (see triangle motif on the top right of ). The interaction between actors that both have the same ecosystem management activities supports the sharing of knowledge, facilitates learning from past governance mistakes, and consequently increases the resilience of the SESs (Lubell and Morrison Citation2021).
Other examples in the literature illustrate the idea of SEN fit. For example, Guerrero et al. (Citation2015) analyze different governance challenges related to issues of social-ecological fit using network data from a biodiversity conservation initiative. The results show that actors indeed tend to collaborate to manage vegetation parcels – but only if they manage the same parcels. As the fit is essential for resolving environmental governance problems, the authors call for additional measures, such as collaboration across individual vegetation parcels, that increase fit for interconnected issues. Similarly, Bergsten, Galafassi, and Bodin (Citation2014) use a network approach to analyze interconnected wetlands in Sweden and how municipalities collaborate to manage these wetlands. As the authors identify only a weak social-ecological fit, they highlight the importance of meaningfully increasing collaboration between the municipalities to avoid isolated, disconnected wetland areas. Both Bergsten, Galafassi, and Bodin (Citation2014) and Guerrero et al. (Citation2015) are mainly concerned with establishing network measures of fit and do not empirically measure governance outcomes to test a relation between SEN fit and outcomes in their case studies.
Beyond the SEN literature, in the broader literature on governance networks, there have long been claims linking network patterns and outcomes (Provan and Kenis Citation2007; Raab, Mannak, and Cambre Citation2015). In structurally explicit studies, for example, Carlsson and Sandström (Citation2007) or Marwell, Oliver, and Prahl (Citation1988) describe structural network properties such as density, centralization, and heterogeneity, as well as how they can benefit performance in collective action situations and help resolve issues in natural resource management. The underlying idea is that networks themselves are a type of resource that can be leveraged for the governance of SESs. In a more structurally implicit network study, Van Meerkerk, Edelenbos, and Klijn (Citation2015) analyze the connective management of water governance networks and how such management influences the network performance of complex water projects in the Netherlands. Their water governance networks are not made explicit through formalization in terms of nodes and ties but are rather based on different connective activities of governance actors. Analyzing connective management, they find that connective activities positively affect network performance in Dutch water projects.
As discussed above, the idea of fit is not restricted to the application to SENs, as in this study, but generally refers to the idea that governance, in order to be successful, needs to take into account specific contingencies of the underlying environmental issues (e.g., Ostrom (Citation1990)). As such, other conceptualizations of social-ecological fit have also been linked to outcomes. For example, Haller et al. (Citation2013) analyze institutional fit based on institutional change in pastoral commons in African floodplains using different theoretical approaches. Based on data from the precolonial and postcolonial times, they identify structural changes over time in the level of institutional fit. The results indicate that fit and misfit alternate but that some factors, such as flexible institutions or mutual economic benefits under specific relations of bargaining power of actors, can promote higher levels of fit. Lebel et al. (Citation2013) similarly analyze institutional fit between institutional arrangements and features of ecosystems not based on networks but on different measures for water governance regimes. Those measures are then analyzed based on important dimensions of fit, such as allocation, integration, or adaption. Lebel et al. (Citation2013) and Haller et al. (Citation2013) both benefit from a research design that includes multiple comparable cases that allow them to make a statement about the impact of fit on governance outcomes controlling for case context.
Linking Social-Ecological Network Fit to Outcomes
While the hypothesis of SEN fit being beneficial for outcomes is omnipresent in the literature (Guerrero et al. Citation2015; Epstein et al. Citation2015; Bodin et al. Citation2016; Scott and Thomas Citation2017), there are only very few empirical studies that explicitly link an empirical measurement of SEN fit to a measurement of governance outcomes (Bodin and Tengö Citation2012; Epstein et al. Citation2015). The hypothesis of SEN fit claims that motifs of social-ecological network fit are associated with “positive” outcomes.
As expressed in this hypothesis, research on SENs often implicitly assumes that the social system influences the ecological system rather than the other way around (Bodin et al. Citation2014; Widmer et al. Citation2019). Still, it is important to highlight that causality is likely to go both ways. While network motifs of fit might improve governance outcomes, the patterns in those networks are also influenced by their environment, and the specific challenges stemming from that environment. Such an impact from the environment on the network structure has been prominently described by Berardo and Scholz (Citation2010) based on the so-called risk hypothesis. The risk hypothesis suggests that in an environment characterized by high-risk situations, bonding ties between actors gain importance, while in low-risk situations, bridging ties are more important. The underlying assumption is that in high-risk settings, actors benefit from closely knitted network structures that create trust and allow for punishment of defection, while in low-risk settings, actors favor the creation of bridging structures that solve coordination problems across distant parts of the network. Bridging structures in networks can be characterized by open network motifs and bonding structures by closed network motifs. In line with the risk hypothesis, we might expect to see more closed motifs in situations where outcomes are perceived as “negative”.
Materials and Methods
Cases
This study deals with the governance of Swiss wetlands. Wetlands are an important source of biodiversity in Switzerland (and worldwide). Even though wetlands only cover 0.7% of the area of Switzerland, they are critical for the survival of many species (MüLler-Wenk et al. Citation2003; Verhoeven Citation2014). While wetlands in Switzerland benefit from increasingly strict regulations and available funding for restoration projects, many wetlands nevertheless remain in poor condition (MüLler-Wenk et al. Citation2003; Verhoeven Citation2014). Here, we analyze ten cases of wetland governance across Switzerland. All our data is available and documented with additional details in Huber, Angst, and Fischer (Citation2022).
We selected a set of ten wetlands for our study, broadly representative in terms of institutional settings at the national level, including all linguistic regions of Switzerland and a variety of cantons (the constituent states in Switzerland). Relevant wetlands need to show characteristic features of wetlands, such as a direct connection to a lake or river. Given our interest in wetlands governance, we also focused on cases with a high density of different social activities and excluded very small wetlands. Finally, the boundaries of the case studies are based not only on the geographical dimensions of the wetlands in question but also include associated regions (e.g., close amphibian spawning areas or farming lands with potential spillover effects) that impact the governance of the wetlands. Looking for diversity across the wetlands’ geographical, socio-cultural, and biophysical structures, we can ensure that the selected case studies are representative of issues of wetland governance in Switzerland (for details, see Supplementary Appendix section: Case study selection).
The data gathering in the ten cases of wetland governance was structured in two phases. For the first data gathering phase, we identified 3–4 actors for each case for exploratory expert interviews. The face-to-face interviews focused on relevant governance outcomes and how ecosystem management activities and interdependencies between them influence governance outcomes. Ecosystem management activities for wetlands are related to recreational activities such as fishing, the maintenance of paths in protected areas, or the operation of hydropower plants (for details on the conceptualization of the interdependencies, see Supplementary Appendix section: Conceptualization of multi-level networks). In the second phase of data gathering, a customized online survey was sent out to all state, civil society, and private sector actors identified as relevant for each of the ten cases to be filled in individually by the identified actors. The surveys asked questions about actor collaboration and actors’ involvement in the governance of the wetlands based on ecosystem management activities. Further, the surveys also included questions about the evaluation of the governance processes and outcomesFootnote1. Within those ten cases of wetland governance, 521 actors were identified to be relevant, 349 of those actors responded to the survey, and 314 filled in all the relevant survey questions (response rate based on AAPOR’s definition excluding partial responses: 63% (Pitt, Schwartz, and Chu Citation2021))Footnote2. Respondents per case ranged from 26 to 52, with a median of 32. This is roughly in line with other studies of small to medium-sized natural resource governance networks (Bodin et al. Citation2019).
Data Structure
To test our hypothesis, we built a dataset of governance outcome assessments per actor for all relevant governance outcomes. For each governance outcome, we then calculated the proportion of closed versus open fit motifs associated with the outcome based on a multi-level network model. shows how a typical record in our data looks in stylized form for some key variables.
Table 1. Assessments of governance outcomes by actors and associated share of open network motifs.
Assessment of Governance Outcomes
The outcome assessment is the dependent variable of the analysis. The governance outcomes included in this analysis represent social and ecological dimensions relevant to the governance of wetlands (see Rhodes et al. (Citation2020) for another example of how outcomes can be used to evaluate governance networks). The governance outcomes (e.g., the biodiversity or recreational value of a wetland) are evaluated based on the peer assessments of all relevant actors. Peer assessments are especially valuable for evaluating diverse elements at a comparable level if not much detailed information is available (Huang and Provan Citation2007; Cebrián-Piqueras, Karrasch, and Kleyer Citation2017).
The governance outcome assessment of the actors is based on a survey item where actors were asked to assess all the relevant governance outcomes in their particular case of wetland governance identified in the previous expert interviews on a scale from “goal achieved”, “goal partially achieved”, to “goal not achieved”Footnote3. The dependent variable is, therefore, an ordinal variable on a three-point rating scale giving information about each actors’ specific assessment of the qualitative state of multiple governance outcomes present in an actor’s case (see Figure A9 in the Supplementary Appendix for details on actors’ outcome assessments by case).
Network Motifs of Social-Ecological Fit
To identify open and closed motifs of SEN fit, we identify triangle and four-cycle motifs using the R package motifr (Angst and Seppelt Citation2020). The reason for our focus on smaller motifs of triangles and four-cycles is that actors’ awareness of social-ecological interdependencies decreases with larger network motifs, as excessive complexity can hinder governance (Bodin et al. Citation2016; Widmer et al. Citation2019; Huber Citation2022). In a triangle motif, two actors are connected to one common entity representing the ecosystem. In a four-cycle, two actors are connected to two different entities of the ecosystem that are also connected (see ). Each network entity is represented here by a specific ecosystem management activity. Actors are connected to an ecosystem entity when they conduct or oversee the ecosystem management activity. The triangle or four-cycle motifs between actors and ecosystem entities are closed if both actors collaborate.
Finally, we calculate the relevant share of closed triangle and four-cycle motifs for all outcome assessments of the actors. To identify the relevant share of closed triangle and four-cycle motifs for the outcome assessments, we include all motifs of actors that impact the specific governance outcomes. Actors have an impact on governance outcomes if their ecosystem management activities have an impact on the governance outcomes based on the underlying multi-level network representation of the SES governance structure of the wetlands (for further details on the multi-level network structure of the dataset and how the variables are operationalized based on the structure of the multi-level network, see Supplementary Appendix section: Conceptualization of multi-level networksFootnote4.
To calculate the share of closed triangle motifs linked to a given outcome, we first count all open and closed triangle motifs present in the specific case for which this outcome is relevant. Second, we assess which (open or closed) triangle motifs impact that specific governance outcome for that case and only keep those. Finally, all these remaining motifs are summed up, and the share of closed triangle motifs vs. open triangle motifs is calculated. Therefore, all outcomes link to an individual share of closed triangle motifs. This share of closed triangle motifs for an outcome might differ between cases but not between actors.
Popularity of Governance Outcomes
To control for differences in the structural embeddedness of outcomes in the multi-level networks, we also include a measure of popularity of governance outcomes in the analysis. Popularity is a measure that functions as an indicator for the level of embeddedness based on the connecting ties of a node – here, outcomes – traditionally used in social network analysis (Robins, Lewis, and Wang Citation2012). The popularity of a governance outcome, in our case, refers to the number of factors influencing the specific outcome. The underlying idea for the interpretation of the popularity of governance outcomes is that achieving good governance outcomes is more challenging in situations of high popularity when outcomes are influenced by many different ecosystem management activities that potentially have diverging effects (Waltner-Toews et al. Citation2008). In this regard, the popularity of outcomes is also a sign of governance complexity varying between cases and complexity varying between nested governance outcomes.
Variability Across Cases, Actors, and Governance Outcomes
Furthermore, we control for actor, governance outcome, and case-level variability. Accounting for variability is essential in our case as the outcome assessment potentially fundamentally differs depending on the actor, outcome, and case at stakeFootnote5. For actors, a key source of variability is that some actors may systematically assess outcomes lower or higher by internally referring to a different rating baseline. Another source of between-actor variability might be that assessments within one outcome category of high relevance to an actor might bias assessments in other categories. Further, also variability among governance outcomes is important to consider. For example, some governance outcomes might be of higher or lower priority, such as biodiversity versus security issues, or specific governance outcomes are more straightforward to achieve than others. Finally, it is essential to account for variability across cases as some cases are generally in a better condition in terms of outcome achievement than others. In our cases, particularly recent revitalization projects are likely to substantially impact the assessment of governance outcomes or, more generally, on the level of conflict (e.g., use conflicts) present in the governance of a particular wetland.
Model Specification
To analyze the dataset, we use a Bayesian multi-level ordinal regression model (Bürkner and Vuorre Citation2019) to predict the likelihood of each category of governance outcome assessment by an actor based on the proportion of closed triangle and four-cycle motifs (see for stylized example data for all variables). The multi-level structure of the model supports accounting for variability across cases, actors, and outcomes by capturing the inherent dependencies and correlations within and between levels. For the reporting of the Bayesian analysis, we use the Bayesian analysis reporting guidelines (BARG) by Kruschke (Citation2021) (for details, see Supplementary Appendix: Table of key reporting points based on Bayesian analysis reporting guidelines).
The model’s dependent variable, ηgovernance outcome, is the assessment of governance outcomes captured as an ordinal variable. We use a cumulative ordinal regression model to assess how network motifs of fit influence governance outcomes. The model has a multi-level structure as the dataset includes actors’ assessments of governance outcomes nested within ten cases for twelve outcomes.
The linear predictor ηgovernance outcome encodes our hypothesis about the influence of relevant network motifs of fit and popularity of governance outcomes in the β parameters. The α parameters stand in for the multi-level parameters of the model accounting for varying intercepts across cases, outcomes and actors. For our outcome of interest, the state of governance outcomes, the linear predictor ηgovernance outcome is of the form:
For the α and β parameters, we rely on weakly informative normal (0,5) priorsFootnote6 (Gelman et al. Citation2008). In the Supplementary Appendix (see Figure A1), the model’s prior and posterior predictive checks can be seen. Without empirical data, the model does not perform well in predicting the empirically observed outcome distribution. However, the predictive performance improves drastically when the model is updated with empirical data. Beyond model fit, this indicates that the observed results are mostly shaped by the empirical observations captured in the dataset and not by the prior choice.
We fit the model using Markov Chain Monte Carlo (MCMC) to derive the posterior distribution, using 4 chains with 10,000 iterations and a burn-in of 5000. The potential scale reduction factor (PSRF) values (Brooks and Gelman Citation1998) were consistently one for all parameters, indicating that chains converged successfully. The tail effective sample size (ESS) or, alternatively, the effective MCMC length was above the recommended 10,000 (Kruschke Citation2014) for all variables (for details on PSRF and ESS values, see Supplementary Appendix: Table A1). To calculate the models in R (R Core Team Citation2021), the brms package (Bürkner Citation2018) is used. All modeling steps can be replicated using the dataset and code provided in the open online repository.
Results
The marginal effects (posterior predictions)Footnote7 of the Bayesian multi-level regression model (see ) indicate that an effect for the network motifs of fit on our measurement of governance outcomes can be identified. The identified trends are similar for triangle and four-cycle motifs for good, neutral, and poor governance outcomes. Differences between the network motifs can be recognized in the comparative share of the three states of governance outcomes, as open triangle motifs are associated with higher shares of good governance outcomes and fewer shares of neutral and poor governance outcomes. As our primary interest is in the impact of open vs. closed network motifs on governance outcomes and not in the differences between different motifs, the description, and interpretation of the results will focus on trends for the impact of motifs of fit on governance outcomes. As these trends are similar for triangle and four-cycle motifs, we will generally refer to network motifs of fit and not distinguish between triangle and four-cycle motifs.
Figure 2. Marginal effects of the share of the closed triangle and four-cycle motifs on governance outcomes assessments. The probabilities of all three states of governance outcomes sum to one for every configuration of inputs. To interpret the figure, the likelihood of the state of a governance outcome falling within the highest versus the lowest category with an increasing share of closed versus open motifs of fit can be compared. Solid lines within ribbons show the median posterior density. Ribbons indicate the 88% credibility interval. The range of the x-axis differs for the share of closed triangles and four-cycle motifs as it is based on the empirically observed range.
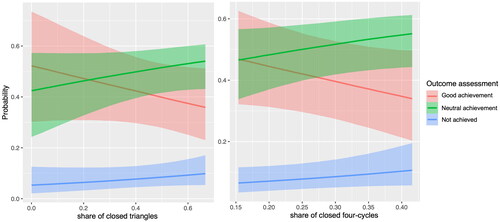
Based on the share of closed network motifs in , the relation between closed network motifs and good and neutral outcome assessments appears particularly strong. For the probability of good governance outcomes assessments, higher shares of closed network motifs have a likely negative and substantial effect. For neutral governance outcomes assessments, a reverse effect can be identified as the share of closed network motifs has a likely positive effect, increasing the chance of neutral governance outcome assessments. These observations indicate that the effect of network motifs of fit differs between the three states of outcome assessments.
For poor outcome assessments, the effect of network motifs of fit is relatively weak, which should, however, be put in the context of an overall low baseline level of occurrence of poor outcome assessments. The uncertainty for poor outcome assessments is relatively big compared to the change of the slope, making the recognition of any clear trends difficult. Therefore, the interpretation and discussion of the results focus less on poor governance outcomes.
We can summarize that, unlike hypothesized, we observe a negative relation between closed network motifs and good governance outcomes, given our model. This effect is particularly strong for good governance outcomes, which are more likely to be present in the absence of closed network motifs, even though the absence of closed motifs indicates a social-ecological misfit – therefore, results do not support the fit hypothesis. While these results are associated with considerable levels of uncertainty, we are confident that the identified trends are robust if not in size but verly likely in direction (see Supplementary Appendix Figures A2–A4 for more information on the posterior distributions). Therefore, even though the effect of network motifs on governance outcomes can likely be identified, the results do not support the fit hypothesisFootnote8.
Discussion
Network research related to governance is constantly developing. Still, empirical evidence on the relation between network patterns and governance outcomes is rare (Bodin et al. Citation2019; Kluger et al. Citation2020). Our study provides valuable information on the relation between SEN motifs of fit and the perceived state of governance outcomes based on a comparative study of ten wetland areas in Switzerland. Results show that SEN motifs are related to governance outcomes. Yet, contrary to our hypothesis, the probability of good governance outcomes decreases with an increasing share of closed network motifs representing social-ecological fit.We cannot find evidence for the often-claimed influence of network motifs of fit on “positive” governance outcomes, and can thus not confirm the fit hypothesis.
To interpret these findings, we suggest a “reverse” effect of governance outcomes on network motifs of fit, given that collaboration patterns are more flexible in adapting to the state of governance outcomes than vice versa (Bodin et al. Citation2014; Widmer et al. Citation2019). Following this line of thinking, we would more likely observe a change in network motifs as a reaction to the state of governance outcomes and only later and less likely an adaption of governance outcomes due to network motifs. Such logic corresponds with the risk hypothesis by Berardo and Scholz (Citation2010) that assumes an effect of governance settings on network structures with more bonding structures in high-risk situations and more bridging structures in low-risk situations. Such bonding and bridging structures corresponds to the network motifs of fit anaylsed in this study setting as bridging structures in networks can be characterized by open motifs and bonding structures by closed motifs.
While causality between network patterns and governance outcomes is likely to go both ways, the literature linking motifs of fit with an improvement in governance outcomes builds on the underlying causal assumption that network motifs of fit (or network patterns in general) influence governance outcomes (Folke et al. Citation2007; Guerrero et al. Citation2015; Widmer et al. Citation2019). In the same vein, research on SENs often implicitly assumes that the social system influences the ecological system rather than the other way around (Bodin et al. Citation2014; Widmer et al. Citation2019).
The level of risk associated with a governance setting can be characterized by different factors, such as the emergence of new policy arenas (Berardo and Scholz Citation2010), the associated levels of environmental stress (Berardo Citation2014), or differences in agenda-setting behavior among actors (McAllister, Taylor, and Harman Citation2015). A negative perception of the state of governance outcomes, as assessed in this article, could also indicate such risks. It can be assumed that poor states of governance outcomes are characteristic of higher levels of risk, and good governance outcomes are characteristic of lower levels of risk. Based on this characterization of risk, a possible alternative hypothesis accounting for our findings could be that good governance outcomes imply low-risk governance situations characterized by bridging structures.
For example, an actor perceiving a poor state of water quality is likely to seek collaboration with other actors responsible for water quality. To address such a potentially conflictive and risky situation, actors – according to the risk hypothesis – create bonding and closed network structures. While creating closed SEN motifs that would represent bonding structures is not easy for actors given complex and untractable issue interdependencies, our results indicate that actors might have specific incentives to create these structures whenever outcomes are perceived as negative. By contrast, in low-risk settings with positively assessed outcomes, actors likely favor the creation of more open network structures and bridging that link different environmental issues – here represented by ecosystem management activities – without the additional costs of many collaboration ties. If actors generally perceive an outcome to be good (e.g., water quality), they have little incentive to invest in an additional collaboration tie with actors related to water quality but might rather deal with the coordination between water quality and other environmental issues such as the use of pesticides close to waterways.
Integrating interdependencies between nodes that do not represent actors entails an extension of the original risk hypothesis. Similarly to Barnes et al. (Citation2022), we, however, think that an extension of the risk hypothesis to consider not only social interaction patterns but also more complex interdependencies of SESs is important. The logic remains similar as the underlying assumption that actors confronted with risky situations will form bonding network structures can also be translated to the present multi-level network. In the multi-level network used in this article, actors form bonding structures across network levels if actors responsible for the same ecosystem management activity also collaborate. Similarly, as bonding structures between actors only, bonding structures between actors and ecosystem management activities can decrease the risk of free-riding.
Based on the assumption that network patterns and governance settings relevant to the governance of SESs mutually influence each other (Berardo Citation2014; Barnes et al. Citation2022), we propose to extend the original risk hypothesis to social-ecologial network motifs. Later, based on the fit hypothesis, those closed SEN motifs increasing the level of fit might benefit the improvement of the state of governance outcomes. Detecting such complex co-occurrence of different network logics would need observations of network patterns and governance settings over a longer period. Based on our empirical observations, we could not investigate different causal directions for the interdependence between network patterns and governance outcomes but can only suggest the potential co-occurrence of both logics. Therefore, we want to highlight that the risk hypothesis implying that the state of governance outcomes affects network patterns does not necessarily compete with the original fit hypothesis tested in this article, but both logics likely co-exist. However, as the support of the extended risk hypotheses to also include interdependencies not only between actors but also across different units of an SES is only moderate (Barnes et al. Citation2022), more research is needed to consider under what settings the risk hypothesis is applicable.
While the mutual influence of network patterns and governance settings is rarely assessed in empirical studies, relevant theoretical frameworks suggest such effects exist. The SES framework (Ostrom Citation2007), for example, assumes that the governance system influences interaction situations between actors and that, at the same time, interaction situations also define the governance system. Similarly, Lubell and Morrison (Citation2021) highlight the importance of co-evolutionary dynamics of institutional navigation between actor networks and institutional structures. A goal for further research should be to fully account for such mutuality between network patterns and governance settings based on empirical network studies. For such studies, it would be necessary to go beyond the analysis of how network motifs and governance outcomes are linked on average and to analyze the link between specific network motifs and actors’ assessments of governance outcomes.
Further, it is highly likely that more complex autocorrelation processes are at play with regard to our network variables, which our relatively simple statistical model does not fully capture. To better account for network dynamics and interdependencies, future research could leverage temporal network data or adopt a mixed methods approach that delves deeper into the mechanisms underlying cross-case differences. To adequately capture these intricate autocorrelation processes, further development of new statistical models and conceptualizations, potentially informed by mixed methods approaches, is warranted.
On a conceptual level, it is important to emphasize that operationalizing social-ecological fit through networks and SEN motifs corresponds to a specific, potentially narrow view on social-ecological fit. The general idea of this conceptual approach is to argue that not all (environmental) issues can be addressed with one-size-fits-all institutional designs (e.g., Ostrom (Citation1990)). The SEN literature has a more specific understanding of fit, emphasizing that ecological interdependencies should be mirrored in the social network (e.g., Bodin et al. (Citation2019)). While alluding to specificities of the wetlands under study and how they might also influence analyses of social-ecological fit, other, broader conceptions of social-ecological fit are not the focus of this article.
Finally, we should also highlight that our conceptualization of ecological nodes as management activities and not as pure ecological nodes (such as species or forest patches) (see Bodin et al. (Citation2019) for a discussion) might influence the interpretation of the findings. More specifically, the interdependencies of different management activities (that represent the ecological network in our case) might be more open to different perceptions by actors than actual, biophysical representations of ecological dependencies between ecological nodes in a system. This “fuzziness” probably complicates the assessment of clear causal relations between SEN motifs of fit and outcomes, which again reinforces our claim for more research on the complex and mutual ways that governance and outcomes influence each other.
Conclusion
Our analysis of motifs in multi-level networks in ten cases of governance of ecosystems in Swiss wetlands provides empirical evidence of a link between network structures and governance outcomes. However, counter to both our hypothesis and a frequent claim in the literature, closed network motifs of fit are not associated with better governance outcomes. This finding does not mean that network motifs of fit cannot positively impact governance outcomes, but suggests that other mechanisms and directions of causality, such as proposed by an extension to the risk hypothesis by Berardo and Scholz (Citation2010) we incorporated here, are also at play. Such an interpretation implies that there are likely temporal interactions between the fit hypothesis and the risk hypothesis. We can, however, not assess if these closed network structures indeed improve the state of the governance outcomes in the long term, as suggested by the fit hypothesis (Bergsten, Galafassi, and Bodin Citation2014; Epstein et al. Citation2015; Bodin et al. Citation2019), as we lack temporal network data.
To sum up, this article illustrates the inherent complexity of governance networks and their causal relations to outcomes characterized by complex social-ecological interdependencies. The description of such complex networks, the simple detection of different network motifs, and the discussion of whether given network motifs are already, perse, positive outcomes are valuable. Still, we would encourage researchers to empirically test the relationship between networks and outcomes and to do so in different contexts and through different outcomes assessments. Research on the outcomes of given network structures could also greatly benefit from either observing networks and outcomes over time or testing the effects of network interventions (e.g., to increase SEN fit) to better grasp causal mechanisms at play.
Supplemental Material
Download MS Word (10.6 MB)Additional information
Funding
Notes
1 For all survey questions relevant to this article, see the Supplementary Appendix section: Survey questions.
2 A comparison between responding and nonresponding actors based on their actor type can be found in the Supplementary Appendix: Table A3.
3 Exact survey question that was used to elicit the actors’ assessments of governance outcomes: Below are possible outcomes for floodplain areas in the [case area]. How well do you think these outcomes are being achieved? Survey participants could choose one of the three options: “goal achieved,” “goal partially achieved”, to “goal not achieved”.
4 Importantly, our usage of the term multi-level, as used in the SEN literature, differs from more common usage outside of the SEN literature (Lomi, Robins, and Tranmer Citation2016) as we do not imply hierarchical nesting.
5 The Intraclass Correlation Coefficient (ICC) values for actor, governance outcome, and case-level variability are below 0.1, indicating that most of the variation in the dependent variable is due to within-group differences rather than between-group differences.
6 The posterior is not sensitive to more or less restrictive alternative prior specifications. For details see Supplementary Appendix Figure A5.
7 For a more complete picture of the posterior distribution for parameters not directly relevant to the hypotheses, see Supplementary Appendix, Figures A2, A3, and A4.
8 For further information on the control variables, see Supplementary Appendix section: Table of key reporting points based on Bayesian analysis reporting guidelines (BARG).
References
- Angst, M., & Seppelt, T. (2020). Motifr: Motif Analysis in Multi-Level Networks. R package.
- Barnes, M. L., L. Jasny, A. Bauman, J. Ben, R. Berardo, Ö. Bodin, J. Cinner, D. A. Feary, A. M. Guerrero, F. A. Januchowski‐Hartley, et al. 2022. Bunkering down’: How one community is tightening social‐ecological network structures in the face of global change. People and Nature 4 (4):1032–48. doi:10.1002/pan3.10364.
- Barnes, M. L., Ö. Bodin, T. R. McClanahan, J. N. Kittinger, A. S. Hoey, O. G. Gaoue, and N. A. J. Graham. 2019. Social-ecological alignment and ecological conditions in coral reefs. Nature Communications 10 (1):2039. doi:10.1038/s41467-019-09994-1.
- Berardo, R. 2014. The evolution of self-organizing communication networks in high-risk social-ecological systems. International Journal of the Commons 8 (1):236–58. doi:10.18352/ijc.463.
- Berardo, R., and J. T. Scholz. 2010. Self-organizing policy networks: risk, partner selection, and cooperation in estuaries. American Journal of Political Science 54 (3):632–49. doi:10.1111/j.1540-5907.2010.00451.x.
- Bergsten, A., D. Galafassi, and Ö. Bodin. 2014. The problem of spatial fit in social-ecological systems: Detecting mismatches between ecological connectivity and land management in an urban region. Ecology and Society 19 (4):22. doi:10.5751/ES-06931-190406.
- Bodin, Ö., A. M. Guerrero, D. Nohrstedt, J. Baird, R. Summers, R. Plummer, and L. Jasny. 2022. Choose your collaborators wisely: Addressing interdependent tasks through collaboration in responding to wildfire disasters. Public Administration Review 82 (6):1154–67. doi:10.1111/puar.13518.
- Bodin, Ö., and M. Tengö. 2012. Disentangling intangible social-ecological systems. Global Environmental Change 22 (2):430–9. doi:10.1016/j.gloenvcha.2012.01.005.
- Bodin, Ö., B. Crona, M. Thyresson, A. L. Golz, and M. Tengö. 2014. Conservation success as a function of good alignment of social and ecological structures and processes. Conservation Biology 28 (5):1371–9. doi:10.1111/cobi.12306.
- Bodin, Ö., G. Robins, R. R. J. McAllister, A. M. Guerrero, B. Crona, M. Tengö, and M. Lubell. 2016. Theorizing benefits and constraints in collaborative environmental governance: a transdisciplinary social-ecological network approach for empirical investigations. Ecology and Society 21 (1), 14. doi:10.5751/ES-08368-210140.
- Bodin, Ö., S. M. Alexander, J. Baggio, M. L. Barnes, R. Berardo, G. S. Cumming, L. E. Dee, A. P. Fischer, M. Fischer, M. Mancilla Garcia, et al. 2019. Improving network approaches to the study of complex social–ecological interdependencies. Nature Sustainability 2 (7):551–9. doi:10.1038/s41893-019-0308-0.
- Brooks, S. P., and A. Gelman. 1998. General methods for monitoring convergence of iterative simulations. Journal of Computational and Graphical Statistics 7 (4):434–55.
- Burch, S., A. Gupta, C. Y. A. Inoue, A. Kalfagianni, Å. Persson, A. K. Gerlak, A. Ishii, J. Patterson, J. Pickering, M. Scobie, et al. 2019. New directions in earth system governance research. Earth System Governance 1:100006. doi:10.1016/j.esg.2019.100006.
- Bürkner, P.-C. 2018. Advanced Bayesian multilevel modeling with the R package brms. The R Journal 10 (1):395–411. doi:10.32614/RJ-2018-017.
- Bürkner, P.-C., and M. Vuorre. 2019. Ordinal regression models in psychology: A tutorial. Advances in Methods and Practices in Psychological Science 2 (1):77–101. doi:10.1177/2515245918823199.
- Carlsson, L. G., and A. C. Sandström. 2007. Network governance of the commons. International Journal of the Commons 2 (1):33–54. doi:10.18352/ijc.20.
- Cebrián-Piqueras, M., L. Karrasch, and M. Kleyer. 2017. Coupling stakeholder assessments of ecosystem services with biophysical ecosystem properties reveals importance of social contexts. Ecosystem Services 23:108–15. doi:10.1016/j.ecoser.2016.11.009.
- Epstein, G., J. Pittman, S. M. Alexander, S. Berdej, T. Dyck, U. Kreitmair, K. J. Rathwell, S. Villamayor-Tomas, J. Vogt, and D. Armitage. 2015. Institutional fit and the sustainability of social-ecological systems. Current Opinion in Environmental Sustainability 14:34–40. doi:10.1016/j.cosust.2015.03.005.
- Felipe-Lucia, M. R., A. M. Guerrero, S. M. Alexander, J. Ashander, J. A. Baggio, M. L. Barnes, Ö. Bodin, A. Bonn, M. J. Fortin, R. S. Friedman, et al. 2022. Conceptualizing ecosystem services using social–ecological networks. Trends in Ecology & Evolution 37 (3):211–22. doi:10.1016/j.tree.2021.11.012.
- Folke, C., L. Pritchard, F. Berkes, J. Colding, and U. Svedin. 2007. The problem of fit between ecosystems and institutions: Ten years later. Ecology and Society 12 (1):30–30. doi:10.5751/ES-02064-120130.
- Gelman, A., A. Jakulin, M. G. Pittau, and Y.-S. Su. 2008. A weakly informative default prior distribution for logistic and other regression models. The Annals of Applied Statistics 2 (4):1360–83. doi:10.1214/08-AOAS191.
- Guerrero, A. M., Ö. Bodin, R. R. J. McAllister, and K. A. Wilson. 2015. Achieving social-ecological fit through bottom-up collaborative governance: An empirical investigation. Ecology and Society 20 (4), 14. doi:10.5751/ES-08035-200441.
- Haller, T., G. Fokou, G. Mbeyale, and P. Meroka. 2013. How fit turns into misfit and back: Institutional transformations of pastoral commons in African floodplains. Ecology and Society 18 (1):16. doi:10.5751/ES-05510-180134.
- Hedlund, J., Ö. Bodin, and D. Nohrstedt. 2021. Assessing Policy Issue Interdependencies in Environmental Governance. International Journal of the Commons 15 (1):82. doi:10.5334/ijc.1060.
- Huang, K., and K. G. Provan. 2007. Structural embeddedness and organizational social outcomes in a centrally governed mental health services network. Public Management Review 9 (2):169–89. doi:10.1080/14719030701340218.
- Huber, M. N. 2022. How the qualities of actor-issue interdependencies influence collaboration patterns. Ecology and Society 27 (4):14. doi:10.5751/ES-13536-270406.
- Huber, M. N., M. Angst, and M. Fischer. 2022. Multi-level network dataset of ten Swiss wetlands governance cases based on qualitative interviews and quantitative surveys. Data in Brief 43:108401. doi:10.1016/j.dib.2022.108401.
- Kluger, L. C., P. Gorris, S. Kochalski, M. S. Mueller, and G. Romagnoni. 2020. Studying human–nature relationships through a network lens: A systematic review. People and Nature 2 (4):1100–16. doi:10.1002/pan3.10136.
- Kruschke, J. 2014. Doing Bayesian data analysis: A tutorial with R, JAGS, and Stan Burlington: Elsevier Inc.
- Kruschke, J. K. 2021. Bayesian analysis reporting guidelines. Nature Human Behaviour 5 (10):1282–91. doi:10.1038/s41562-021-01177-7.
- Lebel, L., E. Nikitina, C. Pahl-Wostl, and C. Knieper. 2013. Institutional fit and river basin governance: A new approach using multiple composite measures. Ecology and Society 18 (1), 1. doi:10.5751/ES-05097-180101.
- Lomi, A., G. Robins, and M. Tranmer. 2016. Introduction to multilevel social networks. Social Networks 44 (44):266–8. doi:10.1016/j.socnet.2015.10.006.
- Lubell, M. 2013. Governing institutional complexity: The ecology of games framework. Policy Studies Journal 41 (3):537–59. doi:10.1111/psj.12028.
- Lubell, M., and T. H. Morrison. 2021. Institutional navigation for polycentric sustainability governance. Nature Sustainability 4 (8):664–71. doi:10.1038/s41893-021-00707-5.
- Lubell, M., J. Scholz, R. Berardo, and G. Robins. 2012. Testing Policy Theory with Statistical Models of Networks. Policy Studies Journal 40 (3):351–74. doi:10.1111/j.1541-0072.2012.00457.x.
- Marwell, G., P. E. Oliver, and R. Prahl. 1988. Social networks and collective action: A theory of the critical mass. III. American Journal of Sociology 94 (3):502–34. doi:10.1086/229028.
- McAllister, R. R., B. M. Taylor, and B. P. Harman. 2015. Partnership networks for urban development: how structure is shaped by risk. Policy Studies Journal 43 (3):379–98. doi:10.1111/psj.12103.
- Müller-Wenk, R., F. Huber, N. Kuhn, and, and A. Peter. 2003. Landnutzung in potenziellen Fliessgewässer-Auen – Artengefährdung und Ökobilanzen. Bern: Bundesamt für Umwelt, Wald und Landschaft (BUWAL).
- Ostrom, E. 1990. Governing the commons – The evolution of institutions for collective action. Cambridge: Cambridge University Press.
- Ostrom, E. 2007. A diagnostic approach for going beyond panaceas. Proceedings of the National Academy of Sciences 104 (39):15181–7. doi:10.1073/pnas.0702288104.
- Ostrom, E. 2007. Institutional rational choice: an assessment of the institutional analysis and development framework theories of the policy process. In Theories of the policy process, ed. P. Sabatier. New York: Routledge.
- Pitt, S. C., T. A. Schwartz, and D. Chu. 2021. AAPOR reporting guidelines for survey studies. JAMA Surgery 156 (8):785–6. doi:10.1001/jamasurg.2021.0543.
- Provan, K. G., and P. Kenis. 2007. Modes of network governance: Structure, management, and effectiveness. Journal of Public Administration Research and Theory 18 (2):229–52. doi:10.1093/jopart/mum015.
- R Core Team. 2021. R: A language and environment for statistical computing. Vienna, Austria: R Foundation for Statistical Computing.
- Raab, J., R. S. Mannak, and B. Cambre. 2015. Combining structure, governance, and context: A configurational approach to network effectiveness. Journal of Public Administration Research and Theory 25 (2):479–511. doi:10.1093/jopart/mut039.
- Reid, W. V., H. A. Mooney, A. Cropper, D. Capistrano, S. R. Carpenter, K. Chopra, P. Dasgupta, T. Dietz, A. K. Duraiappah, and R. Hassan. 2005. Ecosystems and human well-being-synthesis: A report of the millennium ecosystem assessment. Washington: Island Press.
- Rhodes, J. R., A. M. Guerrero, Ö. Bodin, and I. Chadès. 2020. Fundamental insights on when social network data are most critical for conservation planning. Conservation Biology 34 (6):1463–72. doi:10.1111/cobi.13500.
- Robins, G., J. M. Lewis, and P. Wang. 2012. Statistical Network analysis for analyzing policy networks. Policy Studies Journal 40 (3):375–401. doi:10.1111/j.1541-0072.2012.00458.x.
- Sayles, J. S., M. Mancilla Garcia, M. Hamilton, S. M. Alexander, J. A. Baggio, A. P. Fischer, K. Ingold, G. R. Meredith, and J. Pittman. 2019. Social-ecological network analysis for sustainability sciences: a systematic review and innovative research agenda for the future. Environmental Research Letters 14 (9):1–18. doi:10.1088/1748-9326/ab2619.
- Scott, T. A., and C. W. Thomas. 2017. Winners and losers in the ecology of games: Network position, connectivity, and the benefits of collaborative governance regimes. Journal of Public Administration Research and Theory 27 (4):647–60. doi:10.1093/jopart/mux009.
- Scott, T. A., and N. Ulibarri. 2019. Taking network analysis seriously: Methodological improvements for governance network scholarship. Perspectives on Public Management and Governance 2 (2):89–101. doi:10.1093/ppmgov/gvy011.
- Van Meerkerk, I., J. Edelenbos, and E.-H. Klijn. 2015. Connective management and governance network performance: the mediating role of throughput legitimacy. Findings from survey research on complex water projects in the Netherlands. Environment and Planning C 33 (4):746–64. doi:10.1068/c1345.
- Verhoeven, J. T. A. 2014. Wetlands in Europe: Perspectives for restoration of a lost paradise. Ecological Engineering 66:6–9. doi:10.1016/j.ecoleng.2013.03.006.
- Waltner-Toews, D., J. J. Kay, and N.-M E. Lister. 2008. The ecosystem approach: Complexity, uncertainty, and managing for sustainability. New York: Columbia University Press.
- Wasserman, S., and K. Faust. 1994. Social network analysis: Methods and applications. United States of America: Cambridge University Press.
- Widmer, A., L. Herzog, A. Moser, and K. Ingold. 2019. Multilevel water quality management in the international rhine catchment area: How to establish social-ecological fit through collaborative governance. Ecology and Society 24 (3):14. doi:10.5751/ES-11087-240327.