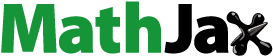
Abstract
Context: The potency of various mineral fiber types to produce mesothelioma was previously evaluated for numerous cohorts, but the differences in potencies for distinct fiber types have yet to be explained.
Objective: To develop an empirical model that would reconstruct mesothelioma potency factors for various types of fiber based on their chemical composition and dimensionality.
Methods: Typical chemical composition and dimensionality metrics (aspect ratios) were obtained and combined with mesothelioma potency factors estimated by Hodgson and Darnton method for Quebec chrysotile, South Africa amosite, South Africa and Australian crocidolite, Russian anthophyllite, Libby amphiboles, and Turkey erionite. The forward stepwise log–log regression method was utilized to determine the best combination of input parameters.
Results: Mesothelioma potency factors (RM) for selected cohorts were effectively reconstructed utilizing the median aspect ratio of fibers and equivalent fractions of SiO2, total Fe oxides or total equivalent Fe3+ as Fe2O3, and MgO. Modeled potency factors increase as the aspect ratio, SiO2, and total Fe oxide (or Fe2O3) content grow, and as the MgO content diminishes. Correlation coefficients up to 0.999, p < 0.01, were achieved. The models also yield reasonable estimates of mesothelioma potencies for other fiber types, including Bolivian crocidolite, Russian chrysotile, fluoro-edenite, and others.
Conclusion: In spite of the empirical approach, the proposed models provide a starting point for targeted studies of mesothelioma mechanisms by elucidating significant contributing physicochemical factors. The models have an exploratory and preliminary character but can potentially be useful to introduce quantitative structure–activity relationship approaches for the toxicology of fibrous minerals.
Introduction
Asbestos toxicological mechanisms and fiber-type cancer potencies remain one of the most controversial topics of modern epidemiology, toxicology, and industrial hygiene (Mossman et al. Citation2011; Moolgavkar et al. Citation2017). IARC recognizes all asbestos mineral types as human carcinogens, and it is clear that apparently all asbestos mineral types can increase risk of lung cancer (IARC Citation2012). However, various asbestos minerals demonstrate different propensities to cause both lung cancer and mesothelioma. For example, mesothelioma mortality in human cohorts exposed to chrysotile asbestos is significantly lower than in cohorts exposed to amphibole asbestos—crocidolite, amosite, tremolite, and others. Moreover, it is clear now that asbestos toxicity should not be considered in isolation, but in the wider context of the carcinogenic potential of elongate mineral particles expressing asbestiform habits, not necessarily corresponding to the commercial or regulatory definition of asbestos. For example, it is important to consider the ability of erionite fibers to produce adverse health effects similar to asbestos, even though erionite is a zeolite, and not an amphibole mineral. The goal of this article is to demonstrate that potency factors of various elongate mineral particles to produce mesothelioma can be effectively modeled based on fiber physicochemical characteristics.
The potency of some elongate mineral particle types has been quantitatively estimated based on available epidemiological data. In 2000, Hodgson and Darnton published a milestone meta-analysis study that demonstrated the statistical differences in mesothelioma potencies, not only among chrysotile and amphibole minerals in general but also between various amphiboles, with the following order of potencies: crocidolite > amosite ≫ chrysotile (Hodgson and Darnton Citation2000). In 2010, the Health & Safety Executive (HSE), based on the calculations of Darnton and the most recent results of epidemiological studies, updated the values of asbestos potency for different mineral types, finding similar relative potency differences (HSE Citation2010). Berman and Crump (Citation2008a) demonstrated a similar, but more striking proportion between the potencies of the various asbestos mineral types. However, they found no statistical differences between amosite and crocidolite mesothelioma potencies.
Based on animal test data, the difference in asbestos potency to produce cancer is less conclusive. This is probably related to the abundant anatomical and physiological differences between test animal species and humans, and due to differences in lung clearance rate of the various fibers. Furthermore, mesotheliomas are observed in animals at extremely high exposure levels that are not typical for human cohorts. Thus, human and animal data are often incomparable (Dodson and Hammar Citation2012).
There are different reasons for the observed variation in asbestos potency, demonstrated in the epidemiological studies. For example, the Agency for Toxic Substances and Disease Registry (ATSDR Citation2010) summarized that the three main determinants of asbestos toxicity are
fiber size,
durability, and
iron content.
Many different models can be applied to the process of asbestos-related cancer development, each of them utilizing their own assumptions and simplifications. We hypothesized that the risk of mesothelioma can be described by fiber size and chemical composition. In this case, ‘durability’ or biopersistence of the fibers (i.e. obviously a critically important factor of toxicity) is likely a function of fiber size and chemical composition, but direct measurement of biopersistence is difficult or even impossible in humans.
For modeling purposes, we decided to use only the cohorts where workers or populations were exposed directly to naturally occurring fibrous minerals, and not to manufactured, fiber-containing products. Therefore, we used published mesothelioma potency factors based on crocidolite cohorts from Australia and South Africa, on an amosite cohort from South Africa, on the Libby amphibole asbestos cohort from the United States, and on the Canadian chrysotile cohort. In addition, we included estimated mesothelioma potency factors for Russian anthophyllite and for Turkish erionite.
Materials and methods
All exposure concentrations of fibers in this article utilized phase-contrast microscopy units of exposure, fibers/cubic centimeters (f/cc). All 95% confidence intervals are two sided.
We used the mesothelioma potency factor, RM, as defined by Hodgson and Darnton (Citation2000) as a percentage of expected mortality from all causes (adjusted to the age of first exposure of 30) per unit of cumulative exposure.
where OM is the number of mesothelioma deaths, EAdj is the total expected deaths from all causes adjusted to the age of first exposure of 30, and X is the mean cumulative exposure in fibers/cubic centimeter-years (f/cc-years). Values of RM that we utilized in our models are summarized in .
Table 1. Mesothelioma potency factors (RM) used for the modeling.
It should be noted that for each of the fiber types, we utilized potency factors that were calculated for specific cohorts, and not combined, meta-analysis potency factors. That allowed us to directly correlate mineralogical characteristics and epidemiological data for specific locations, which avoided potential variability in fiber-associated model parameters for the various cohorts. For example, mineralogical information and aspect ratios for Quebec chrysotile were correlated with Quebec mesothelioma mortality data, but not general chrysotile potency determined from all available cohorts. In addition to the potencies published by Hodgson and Darnton (Citation2000) and Darnton (Citation2010), the mesothelioma potency for anthophyllite (RM = 0.056, 95% CI 0.003, 0.14) was estimated based on a study of mesothelioma among the population around a Russian anthophyllite mine (Korchevskiy et al. Citation2013).
Despite the significant interest in Turkish erionite as apparently one of the most toxic minerals on earth, there is scarce quantitative information about its mesothelioma potency in humans. An IARC publication from 1989 concluded that a cumulative dose of 1 f/cc-year of erionite induced a pleural mesothelioma rate of 996 per 100,000 person-years in exposed populations of Karain and Sarihidir villages in Cappadocia, Turkey (Simonato et al. Citation1989; Dogan Citation2005). Based on the IARC data, the average level of RM for erionite can be estimated as 4.67 (95% CI 1.84, 7.51).
The chemical composition of various minerals (except trace elements), used in the modeling process, is summarized in . Chrysotile and tremolite data are from USGS (Virta Citation2002). Amosite data were obtained from Hayashi et al. (Citation1978). Crocidolite composition was averaged from the data of Shedd (Citation1985). Libby amphibole species percentages were averaged from vein, outcrop, and float data of Bandli et al. (Citation2003). Balangeroite compositional data are from Compagnoni et al. (Citation1983). Erionite data were averaged from Dogan et al. (Citation2006) and USGS (Lowers et al. Citation2010). Anthophyllite data were obtained from Walitzi et al. (Citation1989), Kohyama et al. (Citation1996), and our own studies of samples from Russia. Fluoro-edenite chemical composition is from Gianfagna and Oberti (Citation2001). It was generally assumed that the chemical composition of fibers from the same mineral deposit should be close enough, if not identical. In mineralogical literature, it seems usual to quote chemical composition of a mineral without statistical characteristics. Therefore, it was assumed reasonable to ignore statistical variability of the mineralogical parameters for the purposes of the model’s development, though it can be an additional source of model uncertainty. Obviously, this type variability would come in play when the model is used for risk assessment purposes.
Table 2. Typical Chemical Composition of Asbestos Minerals and Erionite (%)
The median aspect ratio (AR) of fibers determined as fiber length divided by fiber width was used as a quantitative characteristic of their dimensionality. For modeling purposes, we used a corrected value of aspect ratio equal to AR-3. This artificial correction was made to describe the degree to which the aspect ratio was greater than 3:1. We assumed therefore that the mesothelioma potency of the fiber should tend to 0 when its aspect ratio decreases to the value of three. The choice of median aspect ratio was selected rather than mean values because aspect ratio, in general, appeared to be more log-normally distributed than normal.
Median aspect ratios of different airborne asbestos fibers are listed in . Number of counted fibers to determine the central tendency aspect ratios are also listed, when available. The ratios were derived for chrysotile and amosite from Hwang (Citation1983), for crocidolite from Shedd (Citation1985), for Libby amphiboles from US EPA SRC Inc. (2010), for Russian anthophyllite from Korchevskiy et al. (Citation2015), for Finnish anthophyllite from Karjalainen et al. (Citation1994), and for erionite from Carbone et al. (Citation2011). Geometric standard deviation values of the aspect ratio for each fiber type were estimated statistically from the data reported by the sources in each case, with the assumption of lognormal distribution of the data. In some cases, bulk measurements of fiber dimensionality were used as a surrogate for airborne fiber dimensions. If several estimations of median length and width of fibers were available, the geometric means of those values were utilized. The aspect ratio for tremolite and actinolite was assumed to be equal to that of Libby amphibole. The aspect ratio for Russian and Zimbabwean chrysotile was assumed to be the same as that for Quebec chrysotile. Airborne balangeroite length and width apparently was not reported. However, Oury et al. (Citation2014) concluded this mineral ‘has dimensional characteristics similar to amphibole asbestos fibers.’ Therefore, we assumed that the balangeroite aspect ratio was the same as for crocidolite.
Table 3. Assumed aspect ratio of airborne fibers.
The model was empirically assumed to have a log–log regression shape:
where PF is the potency factor for mesothelioma, Ci is the chemical composition or dimensionality parameter, included in the model, k is the number of parameters of the model, and ai is the coefficients of the model.
The choice of a ‘log–log’ shape for the regression model was determined by statistically testing the distribution of all data points for each of the variables. For example, a set of RM parameters, included in the modeling process for the seven cohorts, is distributed log-normally (p value for Shapiro–Wilk test 0.894), but significantly deviates from normality (p = 0.001). Similarly, aspect ratio of fibers for the seven cohorts is log-normally distributed (p = 0.765), as well as MgO content (p = 0.79), Fe2O3 content (p = 0.76), and other components of the chemical composition of the fibers.
The parameters, included for the selection of outcome regression models, are listed in .
Table 4. Variables considered for the modeling procedure.
Model variables were selected as follows:
Average chemical composition weight-percent for different oxides were included as potential independent variables along with median aspect ratio of fibers.
The level of the mesothelioma potency factor (RM) was used as a dependent variable.
We limited the final models to four independent variables (to avoid unacceptably low degree of freedom in the regression).
All combinations of possible model parameters were tested using the automated ‘forward stepwise’ regression method (with Statistica 13.2); the outcome models were selected for further analysis. A forward stepwise method sequentially adds or removes independent variables from the model depending on their F values (the combination with the highest goodness-of-fit criteria is selected).
Negative and positive regression coefficients were allowed, except for the variables characterizing iron content in fibers. The models yielding negative coefficients by Fe, FeO, or Fe2O3 were excluded from consideration.
For each selected model, correlation between independent variables was tested, to avoid potential bias.
The overall range of each of the independent variables over the entire distribution of mineral types was input into Crystal Ball software for Excel, as individual log-normal random variabilities (Oracle Crystal Ball, release 11.1.2.4.000). Sensitivity analysis for RM was then performed as an available routine within the software. The units were in percentage of overall predicted variance of RM for each of the independent variables. Statistica 13.2 software was used for statistical tests and regression analysis.
Initial data analysis demonstrated that there is a significant high correlation between total Fe and Fe2O3 (R = 0.93), as well as between Fe and FeO (R = 0.88). Also, FeO negatively correlates with K2O variable (R= −0.79, p < 0.05). At the same time, mesothelioma potency RM is positively correlated with the SiO2 variable (R = 0.80, p < 0.05) and negatively with the MgO variable (R= −0.91, p < 0.05). Models with correlated independent variables were not included for consideration. As a result, no collinearity was observed for any of the variables included for the modeling process.
Several models were excluded from consideration because of the negative coefficient by iron content variables. All of them contained Al2O3 variables. Exclusion of Al2O3 from the regression allowed to avoid the problem with negative coefficient by the iron variable. It is noteworthy that Al2O3 is not represented (‘nil’ content) in South African (Transvaal) crocidolite (Shedd Citation1985). Al2O3 was also not found in some samples of Cape Province crocidolite and Transvaal amosite (Hodgson Citation1979). Therefore, exclusion of Al2O3 seems justified for the modeling procedure.
Results
The following model was found the best among all models with various combinations of independent variables:
R = 0.998, R2=0.996, adjusted R2=0.988, p = 0.0077, goodness-of-fit criteria F(4,2)=129.67, number of observations 7, residuals normally distributed with mean 0, and standard deviation 0.08, no outliers.
Partial correlations for the model were found as 0.63 for SiO2, 0.91 for Fe2O3, −0.99 for MgO, and 0.90 for aspect ratio.
It is intriguing that the ‘forward stepwise’ regression procedure did not yield models with FeO as an independent variable, preferring the models with Fe2O3. Potentially, erionite, with the highest RM value, containing iron as Fe2O3 rather than FeO, was responsible for this result. However, the regression coefficient of FeO was not statistically significant when we tested the model with or without the erionite.
Regression models with Fe (total iron variable) were not considered for the stepwise selection process (because of the correlation with FeO and Fe2O3 variables). However, if the variable Fe2O3 is replaced with Fe, the following regression can be obtained, also with a high regression coefficient:
R = 0.994, R2 = 0.988, adjusted R2 = 0.965, p = 0.023, goodness-of-fit criteria F(4,2)= 42.78, number of observations 7, residuals normally distributed with mean 0, and standard deviation 0.13, no outliers.
Partial correlations for the model were found as 0.40 for SiO2, 0.71 for Fe, −0.97 for MgO, and 0.80 for aspect ratio.
In both the models, SiO2, aspect ratio and iron (ferrous or ferric) content of the fibers have positive coefficients (positively correlate with mesothelioma potency). However, MgO content is negatively correlated with the potency. The toxicological meaning of this finding will be discussed further.
The regressions between predicted and published levels of potency factors are illustrated in . Both models have high correlation coefficients although the model with Fe2O3 seems to be in slightly better agreement with the data, and accordingly, it was selected by the forward stepwise modeling procedure. Below, we use the two models interchangeably, but specify the model under discussion.
Figure 1. (a) Regression between published and modeled (using Fe3+ oxide content) potency factors for mesothelioma . Red dots, published potency; green dots, UCL 95% potency; blue dots, LCL 95% potency; red line, modeled to published levels regression; dotted line, prediction interval. (b) Regression between published and modeled (using total iron oxides content) potency factors for mesothelioma. Red dots, published potency; green dots, UCL 95% potency; blue dots, LCL 95% potency; red line, modeled to published levels regression; dotted line, prediction interval.

Sensitivity analysis demonstrates that the proposed model with Fe2O3 is prevailingly affected by MgO (72% of the variance) and AR (16% of the variance), to a lesser extent SiO2 (6% of the variance) and Fe2O3 (5% of the variance). At the same time, for example, for the Fe2O3 model, doubling SiO2 increases RM by approximately a factor of 4, doubling AR increases RM by a factor of 2.5, doubling Fe increases RM by a factor of 1.2, and doubling MgO decreases the mesothelioma potency by a factor of 2.6. For the model with total Fe, the variance of the model is affected by MgO (75%), AR (18%), SiO2 (5.5%), and Fe (1.5%).
The results of the model for various fiber types are listed in . For some of the fiber types, the table allows comparison modeled values for RM with previously published levels. For other fiber types, however, the potency has not been published, and the model provides an estimate of the unknown RM values.
Table 5. Modeled and published mesothelioma potency factor (RM) for selected minerals.
It is remarkable that we can reasonably estimate potency factors for the various mineral fiber types, independently, based on the properties of the other mineral fibers in relation to RM. To demonstrate this approach, we eliminated the RM value, systematically and separately, one at a time, for each of the fiber types shown in . Then, with the seven separate regression equations, we solved for the RM that was eliminated in each instance. The results of this ‘blinded’ modeling are shown in (for simplicity, only Fe2O3-containing model is used). The blinded model results are generally within less than a factor of about two to three from the published values.
Table 6. The reconstruction of mesothelioma potency factors based on a consequent exclusion of items from the original data set.
Also, when RM for Turkish erionite is varied systematically in small increments from the IARC-derived value of 4.67, and F is maximized in the Fe2O3 version of the model, a value of 3.99 is obtained. In similar fashion, illustrates how the potency level of RM = 0.0005 for Quebec chrysotile maximizes F criteria in the Fe3+ version, compared with the published RM of 0.0009. In the total iron version (which apparently is less robust), F increases with chrysotile potency approaching 0 and, separately, with erionite potency unlimitedly increasing.
Discussion
Mathematical modeling of the toxicity of various agents based on their chemical composition, structure, or other measurable parameters has been a focus of scientific study for several decades. For example, Barratt (Citation2000) emphasized that ‘the prediction of toxicity from chemical structure can make a valuable contribution to the reduction of animal usage in the screening out of potential toxic chemicals at the early stage and in providing data for making positive classifications of toxicity.’
The development of an empirical model that is consistent with reasonable toxicological mechanisms helps interpret epidemiologically observed differences in mesothelioma potency factors and contributes in overcoming a significant gap between asbestos mineralogy, epidemiology, and toxicology.
The proposed models have three main points of justification: (a) they are based on a meaningful choice of variables and a general mathematical form; (b) they fit published mesothelioma potency factors well; and (c) they yield reasonable estimations of potency factors for fibers with absent epidemiological information, as demonstrated below.
For example, the ratio between modeled mesothelioma potency of crocidolite (average between South African and Australian), South African amosite, and Quebec chrysotile, is 541:116:1 for Fe model and 566:72:1 for Fe2O3 model, very close to the estimation proposed by Hodgson and Darnton (Citation2000) of 500:100:1.
The mesothelioma potency of erionite, calculated from the IARC data, or predicted by the models, appears to be ∼6 times higher than for Cape Province crocidolite (6.4 times for Fe2O3 model, 7.9 times for total iron model). The potency of erionite in animal experiments is significantly higher than for crocidolite (Wagner et al. Citation1985). It is remarkable that Coffin et al. (Citation1992) estimated mesothelioma potency of erionite fibers (as percentage excess lung carcinoma) being 5.6 times higher than for crocidolite, based on human data. Our assumed and modeled estimates of RM for erionite, therefore, seem reasonable.
The mesothelioma potency of anthophyllite is generally unknown and may depend on the morphology of the fibers in specific deposits. In the study of the Finland cohort of anthophyllite miners, four cases of malignant pleural mesothelioma were found among the cohort of 999 miners (Karjalainen et al. Citation1994). The Finnish authors stated that mesothelioma risk caused by anthophyllite was ‘higher than among chrysotile miners and almost as high as among amosite miners’ (Meurman et al. Citation1994). This statement is consistent with our modeling results. According to both of our models, Finnish anthophyllite seems to be 1.3 (Fe2O3 model) to 1.5 (total Fe model) times less potent than amosite, and respectively 62.5–66 times more potent than Quebec chrysotile.
We estimate mesothelioma potency for balangeroite as 0.004, 4.5–5 times higher than chrysotile, and 3.5–5.7 times lower than tremolite (respectively, for Fe2O3 and total iron models). It is consistent, for example, with Turci et al. (Citation2009) who concluded that, ‘considering the profound differences between the structure of balangeroite and amphiboles, previous results and observations on the poor ecopersistence of balangeroite…balangeroite traces may contribute to the overall toxicity of the airborne fibres in Balangero, but may not be compared to tremolite nor considered solely responsible for the excess of mesothelioma found in Balangero.’ Turci suggested also that balangeroite should have biopersistence similar to chrysotile.
Based on our calculations, the RM of tremolite (Pakistan) can be estimated as 0.015–0.023. This range is comparable to the published RM for Libby amphibole (0.03), and lower than that of amosite (0.06). Our calculations show, however, that actinolite (mineral very close to tremolite, but with higher iron oxide fraction), according to our model may have potency up to 0.024. As the tremolite–actinolite solid solution series move toward ferro-actinolite with high iron content, the modeled potency should increase slightly higher (Mustard Citation1992).
Based on our models, RM of Bolivian crocidolite ranged from 0.06 to 0.09, up to 10 times lower than for its South African counterpart. This is consistent with the observations of Ilgren (though not universally accepted) that magnesium-rich Bolivian crocidolite (magnesiorebeckite) apparently did not cause significant elevation of mesothelioma mortality in workers and the population (Ilgren et al. Citation2015).
The modeled potency of Quebec chrysotile is very close to the published result; the modeled Russian chrysotile RM appears to be about twice that of the Quebec variety (because of the apparent differences in chemical composition).
Fibrous minnesotaite and other phyllosilicates were suspected to increase risk of mesothelioma in taconite workers of the Western Mesabi Range in Minnesota (Lambert et al. Citation2016). Minnesotaite contains around 2% of Fe2O3, 6% of magnesium, and 51.3% of SiO (Gruner Citation1944). Although it appears that generally minnesotaite does not contain long and thin asbestiform fibers (NAS/NRC Citation1984), hypothetically, if some portion of airborne minnesotaite is of a fibrous nature with a median aspect ratio at least 5:1, its RM based on our models could be as high as 0.010–0.015, roughly equivalent to tremolite.
For fibrous fluoro-edenite, an amphibole mineral from Biancavilla, Sicily, that was acknowledged as a mesothelial carcinogen by the International Agency for Research on Cancer (IARC Citation2017), the model with Fe2O3 predicts RM at the level of 0.11, and the model with total Fe predicts RM=0.14, assuming median aspect ratio of fibers approximately as 50:1, based on Pugnaloni et al. (Citation2007). It is close to the level that can be derived from premitigation exposure data of Bruni et al. (Citation2014) and mesothelioma mortality data of Biggeri et al. (Citation2004). The published data referred to the range of 0.4–8.2 f/L for general outdoor fluoro-edenite concentrations, 93–183 f/L for unpaved roads concentrations, and <0.4 to 4.8 f/L indoor concentrations. In this case, the cumulative lifetime exposure of 0.43 f/cc-years, total expected cohort mortality 3267, 16 mesothelioma cases, and a Hodgson–Darnton age-correction coefficient of 9.75, yields RM=0.12. Apparently, the models work for fluoro-edenite as effectively as for other fiber types with limited epidemiological information.
It is important to assess for toxicological plausibility of the developed models. In particular, iron ‘overload’ was suggested to play an important role in asbestos fiber toxicity (Quinlan et al. Citation1994). Iron is a stoichiometric component (about 27%, w/w) in amphiboles such as crocidolite and amosite, while in chrysotile it is a contaminant (about 1–6%, w/w), replacing isomorphous magnesium and silicon (Schwarz and Winer Citation1971; De Waele et al. Citation1984). Retained in the lungs, asbestos fibers adsorb iron from the cellular environment creating an iron-containing coating around the fibers. Some view the iron coating as a step in the mechanism of toxicity while others believe the coating is protective (Gilmour et al. Citation1997; Jaurand Citation1997; Xu et al. Citation1999).
The role of the two chemical forms of iron, Fe3+ and Fe2+, in asbestos cancer development is not completely clear. Our result that Fe3+ and not Fe2+ may be responsible for some of the carcinogenic effects of fibrous minerals is somewhat supported by other publications. For example, iron is prevailingly in the Fe3+ form in erionite. Fe3+ was demonstrated to have a more pronounced effect on reduction of human enzyme activity than Fe2+ (Karami et al. Citation2010). Fisher et al. (Citation1998) emphasized the role of Fe3+ in fiber toxicity. Ghio et al. (Citation1992) determined that generation of hydroxyl radical by crocidolite asbestos is proportional to surface Fe3+. Toyokuni (Citation1996) emphasized that different compounds of Fe3+ are carcinogenic in animals. For example, intraperitoneal injections of ferric sacharate followed by administration of nitrilotriacetate (NTA) caused a high incidence of diffuse mesotheliomas. Intraperitoneal injections of Fe-NTA can induce peritoneal mesotheliomas in rats, as well (Okada et al. Citation1989; Toyokuni Citation1996).
It is also remarkable that the effect of the iron fraction in the regression model is quite low. Perhaps the role of iron is not based upon iron content in fibers but rather ‘deficiency’ of iron that fibers may create in the cell, attracting iron ions from elsewhere. Ghio et al. (Citation2008) suggested that differences between serpentine and amphibole fibers in oxidative stress and biological effects do not reflect the inclusion of iron in the crystal lattice, but rather larger numbers of surface functional groups (first, silanol) in amphiboles which can mobilize iron from the host. It is possible either or both iron in fibers and extracellular iron may play a mechanistic role in the carcinogenicity process.
Different authors have also emphasized the possible role of silicon content of asbestos fibers in carcinogenicity mechanisms. The National Academy of Sciences noted that in freshly fractured silicate minerals (including asbestos), broken bonds result in surface silica sites that exhibit ionic charge. As those sites interact with water and dissipate the surface charge, they generate free radicals in the fluid. According to Fubini et al. (Citation1990), this process can be particularly important in silica-induced pathogenesis. Ultimately, the fractured sites involve silanol groups (Si-OH) that will protonate or deprotonate in response to pH. Aust et al. (Citation2011) emphasized that such silanol groups may also contribute heavily to the reactions these minerals undergo in vivo. The surface silanols influence the interaction with cations, such as ionic states of Fe that were not originally part of the structure. For example, silanol groups bind cellular ferric iron to produce a silicon–oxygen–iron complex that may catalyze single electron transfer reactions producing cell injury effects.
Dimensionality of fibers is widely considered as a determinant of their toxicity (Berman et al. Citation1995; Berman and Crump Citation2008b; Lippmann Citation2009; Loomis et al. Citation2010; Barlow et al. Citation2017). Aspect ratio can be viewed as one of the possible characteristics, useful, even if not universal, to generalize the dimensional characteristics of fibers (Boulanger et al. Citation2014). It is obvious that using the aspect ratio of fibers (and not separate length and width) as a surrogate dimensionality characteristic has its limitations. However, it was suggested recently that aspect ratio can be an important toxicological characteristic not only for asbestos fibers but also for nanoparticles (Tran et al. Citation2008).
In spite of various views on the role of dimensionality in fiber toxic effects, the overall consensus is that exposure to long, thin elongate mineral particles increases the probability of disease in comparison with exposure to wider or shorter fibers.
The function of magnesium as a ‘risk reduction’ factor in fibers is far from self-obvious. Pascolo et al. (Citation2011) suggested that magnesium plays important role in biological responses of cells to asbestos. It was suggested that Mg should be considered as an active participant in the biochemical process coating asbestos fibers to isolate the fibers from the surrounding tissue. Citing recent concepts regarding possible participation of magnesium in many mechanisms of the antioxidant defenses of the body, the authors suggested that magnesium concentrates around asbestos fibers, especially in the cellular regions with high iron content, to counteract the oxidative stress reactions. Besides that, the authors also discussed a complex role of Mg in inflammation and tissue repair (Pascolo et al. Citation2011) and, interestingly, it was also demonstrated by the same research team that iron in asbestos bodies was prevailingly trivalent (Pascolo et al. Citation2013). Cellular Mg apparently is involved in general defense mechanisms, but the source of Mg is not necessarily related to asbestos fiber Mg content. As in the case of iron, Mg from fibers may play a role in cellular biochemistry. While the effect of Mg in fibers as a defense mechanism is not known, such a function should be considered as a topic for future research.
Alternatively, it is possible that biopersistence of asbestos fibers in the human respiratory system is approximately inversely proportional to magnesium content. For example, chrysotile, with a very short half-life in the human lung contains much higher levels of magnesium than amphibole asbestos, which has a half-life of decades (Rasmuson et al. Citation2014). Biopersistence data were generally lacking to fully test this hypothesis for various types of amphibole asbestos.
It is remarkable, however, that a combination of aspect ratio of fibers and Mg fraction by itself allows to model mesothelioma potency factor with good accuracy, though with a slightly lower correlation coefficient:
The validity of exposure data and mesothelioma mortality information used for calculation of potency factors is a critical aspect in evaluating the accuracy of the proposed models. As discussed in the Results section, we attempted to determine how varying the values of data points in the performed regressions influenced the resulting coefficients of the models. It was confirmed that, for example, when some of the cohorts were excluded from the initial data sets, the potency levels could be effectively reconstructed based on the remaining data.
Further exploration of the influence of the original data on the modeling outcomes is useful, as well. For example, it can be demonstrated that if noncensored mortality data from De Klerk (Citation1994) would be used to estimate mesothelioma potency factor in Wittenoom, Australia crocidolite cohort, a slightly lower estimation of potency for Australian crocidolite results (RM=0.27 vs. 0.48, in Hodgson and Darnton Citation2000). With the alternative RM, we obtain an even more robust regression model than original data set allowed. In this case, the model
has R = 0.9999, F = 71883, p = 0.00014, with all the regression coefficients being statistically significant at p < 0.05. Interestingly, Hodgson and Darnton (Citation2000) also considered the possibility of a lower potency factor for Wittenoom cohort because of potential underestimation of the exposure. It is noteworthy, however, that this ‘improved’ model would yield mesothelioma potency predictions for different cohorts very close to the values predicted by both the Fe2O3 and total Fe models described above (pairwise correlation coefficients between each of the three models being higher than 0.9994).
For our modeling, we included only mining or environmental cohorts, but not cohorts with exposure to asbestos products. However, the models can be applied for asbestos product exposure cohorts if chemical characteristics and dimensionality of fibers are known. Hodgson and Darnton (Citation2000), for example, estimated RM for the North Carolina asbestos textile cohort as 0.013 (14 times higher than for mining chrysotile cohort). It is well known, however, that the textile industry used longer grades of asbestos fibers. For example, Rood and Streeter (Citation1984) reported the aspect ratio of airborne asbestos fibers in a textile factory as 19.34. In combination with the typical chemical composition of chrysotile, our models yield levels of mesothelioma potency of 0.005 (for Fe2O3 model) or 0.006 (for total Fe model), much closer to the textile epidemiological data than the observed potency of chrysotile fibers in Quebec mines and mills (where lower grades of asbestos fibers apparently prevailed: for example, in 1954 asbestos textile grades of Quebec asbestos production comprised approximately 3.9% from all grades (Canadian Bureau of Statistics Citation1954–1955)). Further studies are neeeded, however, to fully understand applicability of the proposed models to the situations when various characteristics of asbestos products can affect the carcinogenic potency of fibers.
Uncertainties and limitations
Our work does not fully represent routine toxicological and statistical analysis in the sense that usually a priori hypotheses are selected and then tested by appropriate statistical methods. As discussed, we utilized the forward stepwise to come up with the ‘best models,’ and then we evaluated the toxicological plausibility of the results. Therefore, predicted confidence intervals and other presented statistics should be interpreted as approximate and qualitative findings, though they were helpful in optimizing model selection. While the presented data should be viewed semi-quantitatively rather than in a highly quantitative statistical sense, we have demonstrated that our models approximately predict published and derived mesothelioma potency factors, which is a significant finding.
All components of the models—chemical composition of fibers and their median aspect ratios, as well as potency factors RM—were included in the statistical calculations as single point estimates, or central tendencies. In the future, it is possible to consider expanding the methodology through utilization of the Monte-Carlo approach with ranges of variables rather than mean or median values. At the same time, we expect that the mean values of model input parameters will still be approximately the same as we utilized published central tendency values in estimating input variables.
Mineralogical composition of asbestos fibers used in our modeling was derived from available sources. The variability of the data could affect the modeling results, though it was assumed that for each asbestos deposit, the mineral composition should be stable enough to ignore the difference in various estimations. Also, the variability of the aspect ratio of the fibers reported in this article () could affect the prediction value of the results and should be taken into consideration for their prognostic applicability.
Another possible limitation of our modeling approach is that the amphibole hypothesis according to which potentially pure chrysotile asbestos may have little or no propensity to produce mesothelioma in humans (Mossman Citation1993) was not mathematically explored in this study. Otherwise, for modeling purposes, chrysotile would have to be assigned a mesothelioma potency value near zero, and not 0.0009, as we assumed. However, the developed models further confirm that chrysotile asbestos is about two orders of magnitude or less potent for mesothelioma, than typical amphibole asbestos on a fiber-by-fiber basis (Moolgavkar et al. Citation2017).
It is important to also underscore that Hodgson and Darnton (Citation2000) meta-analytical estimations of RM values for various fiber types were determined from cohorts with predominant exposures to single fiber type. This approach limited the number of underlying cohort studies in the Hodgson and Darnton analysis, while Berman and Crump included all available ‘pure’ and mixed-fiber cohorts in estimating KM values. That the Hodgson and Darnton (Citation2000) overall fiber potency results are in reasonable agreement with the Berman and Crump (Citation2008b) results also suggests that estimation of individual cohort exposures and mesothelioma mortality are not major limiting factors in determining asbestos fiber type mesothelioma potency factors or exploring potential mechanisms of respiratory carcinogenesis. This is because the potency factors determined by US and UK researchers have been determined to at least some extent by different cohorts and varying exposure estimation methods. It can also be demonstrated that Berman and Crump KM values can be also reconstructed by models similar to those proposed in this article based on Hodgson and Darnton RM values (Berman and Crump Citation2008a).
It is not clear as well, if tremolite and similar fiber types (such as actinolite and Libby amphibole) potencies can be evaluated without taking into account the proportion of asbestiform and non-asbestiform fibers. To the extent that the indicated median aspect ratios reflect this proportion, our model may reflect the fact of not all fibers being asbestiform rather than relying solely on the indicated variables. However, in future modeling efforts, it may be useful to take the habit of the fibers into account.
The results we obtained by modeling were potentially affected by the choice of chemical constituents of the fibers taken into consideration. For example, inclusion of Al2O3 changes the outcomes significantly and increases statistical significance, but apparently decreases toxicological sense (when e.g. iron oxide content would have negative coefficients, etc.). Our observations, however, demonstrate that aluminum oxide is not an essential component of mesothelioma-potent mineral fibers. Different authors referred to amphiboles (like amosite and crocidolite) with nil content of aluminum. Nevertheless, additional studies are needed to determine the role of aluminum and other elements in the toxicity of fibrous minerals. Other important parameters, not included in our consideration, might also affect the choice of the ‘best model.’ Among such variables that may require future attention include length, width, and effective area of the fibers as well as their biopersistence in human lungs.
Other limitations of the modeling approach can be also mentioned (predefined log–log shape of the model, a limited set of input cohorts for comparatively large number of variables, apparent sensitivity of the model to small changes in input variables, and representativeness of published fiber dimensionality values). Further studies to explore and hopefully overcome those limitations are warranted.
Conclusions
The empiric mathematical models that are proposed to estimate mesothelioma potency factors to chemical constituents of the fibers (silicon, iron, and magnesium oxides content) and their median aspect ratio appear to yield reasonably reliable results. The constructed models can be seen as a tool for preliminary assessment of mesothelioma potencies for various elongate mineral particles with unknown potency. The variability of mesothelioma potency factors for different mineral types of fibers may provide clues in explaining varying toxicological mechanisms in the carcinogenesis of fibrous materials. The models that were developed have obviously an exploratory and preliminary character, but can potentially be useful for the further introduction of quantitative structure–activity relationship approaches for the toxicology of fibrous minerals. It is our hope that our models will stimulate a wider discussion of the role of various measurable characteristics of asbestos and similar fibers in the process of carcinogenesis.
Disclosure statement
No outside interest paid for any part of this study. Some of the authors are involved in asbestos litigations as experts. No potential conflict of interest was reported by the authors.
Additional information
Funding
References
- Agency for Toxic Substances and Disease Registry [ATSDR]. 2010. Case studies in environmental medicine (CSEM): asbestos toxicity. Course WB/CB1093. www.atsdr.cdc.gov/csem/.
- Aust AE, Cook PM, Dodson RF. (2011). Morphological and chemical mechanisms of elongated mineral particle toxicities. J Toxicol Environ Health Part B. 14:40–75.
- Bandli BR, Gunter ME, Twamley B, et al. (2003). Optical, compositional, morphological, and X-ray data on eleven particles of amphibole from Libby, Montana, USA. Can Miner. 41:1241–1253.
- Barlow C, Grespin M, Best E. (2017). Asbestos fiber length and its relation to disease risk. Inhal Toxicol. 29:541–554.
- Barratt MD. (2000). Prediction of toxicity from chemical structure. Cell Biol Toxicol. 16:1–13.
- Berman DW, Crump KS. (2008a). Update of potency factors for asbestos-related lung cancer and mesothelioma. Crit Rev Toxicol. 38:1–47.
- Berman DW, Crump KS. (2008b). A meta-analysis of asbestos-related cancer risk that addresses fiber size and mineral type. Crit Rev Toxicol. 38:49–73.
- Berman DW, Crump KS, Chatfield EJ, et al. (1995). The sizes, shapes, and mineralogy of asbestos structures that induce lung tumors or mesothelioma in AF-HAN rats following inhalation. Risk Anal. 15:181–195.
- Biggeri A, Pasetto R, Belli S, et al. (2004). Mortality from chronic obstructive pulmonary disease and pleural mesothelioma in an area contaminated by natural fiber (fluoroe-edenite). Scand J Work Environ Health. 30:249–252.
- Boulanger G, Andujar P, Pairon J-C, et al. (2014). Quantification of short and long asbestos fibers to assess asbestos exposure: a review of fiber size toxicity. Environ Health. 13:59.
- Bruni BM, Soggiu MA, Marsili G, et al. (2014). Environmental concentrations of fibers with fluoro-edenitic composition and population exposure in Biancavilla (Sicily, Italy). Ann 1st Super Sanità. 50:119–126.
- Canadian Bureau of Statistics. 1954–1955. The asbestos mining industry. Ottawa, Canada: Queen's Printer and Controller of Stationery.
- Carbone M, Baris YI, Bertino P, et al. (2011). Erionite exposure in North Dakota and Turkish villages with mesothelioma. PNAS. 108:13618–13623.
- Coffin DL, Cook PM, Creason JP. (1992). Relative mesothelioma induction in rats by mineral fibers: comparison with residual pulmonary mineral fiber number and epidemiology. Inhal Toxicol. 4:273–300.
- Compagnoni R, Ferraris G, Fiora L. (1983). Balangeroite, a new fibrous silicate related to gageite from Balangero, Italy. Am Miner. 68:214–219.
- Darnton A. 2010. HSE assessment of recently updated information relating to cohorts with chrysotile exposure. [Accessed 2019 Feb 15.] www.hse.gov.uk/aboutus/meetings/iacs/acts/watch/271010/watch-october-2010-additional-chrysotile-exposure.pdf.
- De Klerk N, Musk AW, Armstrong BK, Hobbs M. 1994. Diseases in miners and millers of crocidolite from Wittenoom, Western Australia: a further follow-up to December 1986. Ann Occup Hyg. 38:647–655.
- De Waele J, Luys M, Vansant E, Adams F. (1984). Analysis of chrysotile asbestos by LAMMA and Mossbauer spectroscopy: a study of the distribution of iron. J Trace Microprobe Tech. 2:87–102.
- Dodson RF, Hammar SP. 2012. Asbestos: risk assessment, epidemiology, and health effects. Boca Raton, FL: CRC Press, Taylor & Francis Group.
- Dogan A. 2005. Malignant mesothelioma and erionite. In: Pass HI, Vogelzang NJ, Carbone M, editors. Malignant mesothelioma: advances in pathogenesis, diagnosis, and translational therapies. USA: Springer; p. 242–258.
- Dogan AU, Baris YI, Dogan M, Emri S, Steele I, Elmishad AG, Carbone M. 2006. Genetic predisposition to fiber carcinogenesis causes a mesothelioma epidemic in Turkey. Cancer Res. 66:5063–5068.
- Fisher C, Brown D, Shaw J, Beswick PH, Donaldson K. 1998. Respirable fibres: surfactant coated fibres release more Fe3+ than native fibres at both pH 4.5 and 7.2. Ann Occup Hyg. 42:337–345.
- Fubini B, Giamello E, Volante M, Bolis V. (1990). Chemical functionalities at the silica surface determining its reactivity when inhaled. Formation and reactivity of surface radicals. Toxicol Ind Health. 6:571–598.
- Ghio AJ, Stonehuerner J, Richards J, Devlin RB. (2008). Iron homeostasis in the lung following asbestos exposure. Antioxid Redox Signal. 10:371–377.
- Ghio AJ, Zhang J, Piantadosi CA. (1992). Generation of hydroxyl radical by crocidolite asbestos is proportional to surface [Fe3+]+. Arch Biochem Biophys. 298:646–650.
- Gianfagna A, Oberti R. (2001). Fluoro-edenite from Biancavilla (Catania, Sicily, Italy): crystal chemistry of a new amphibole end-member. Am Miner. 86:1489–1493.
- Gilmour PS, Brown DM, Beswick PH, et al. (1997). Free radical activity of industrial fibers: role of iron in oxidative stress and activation of transcription factors. Environ Health Perspect. 105:1313–1317.
- Gruner JW. (1944). The composition and structure of Minnesotaite, a common iron silicate in iron formations. Am Miner. 29:363–372.
- Hayashi H, Aita S, Suzuki M. (1978). Semiquantitative chemical analysis of asbestos fibers and clay minerals with an analytical electron microscope. Clays Clay Miner. 26:181–188.
- Health and Safety Executive. Darnton A [HSE]. 2010. Assessment of recently updated information relating to cohorts with chrysotile exposure. [Accessed 2013 Apr 3]. http://www.hse.gov.uk/aboutus/meetings/iacs/acts/watch/agendas.htm.
- Hodgson AA. 1979. Chemistry and physics of asbestos. In: Michaels L, Chissick SS, editors. Asbestos: properties, applications and hazards. New York (NY): John Wiley & Sons; p. 67–114.
- Hodgson JT, Darnton A. (2000). The Quantitative risks of mesothelioma and lung cancer in relation to asbestos exposure. Ann Occup Hyg. 44:565–601.
- Hwang C-Y. (1983). Size and shape of airborne asbestos fibres in mines and mills. Br J Ind Med. 40:273–279.
- Ilgren, E, van Orden, D, Lee, R, Kamiya Y, Hoskins J. (2015). Further studies of Bolivial crocidolite- Part IV: Fibre width, fibre drift and their relation to mesothelioma induction: preliminary findings. Epidemiol Biostat Public Health. 12:e11167.
- International Agency for Research on Cancer [IARC]. 2012. Arsenic, metals, fibres, and dusts. A review of human carcinogens. Vol. 100C. Lyon, France: IARC.
- International Agency for Research on Cancer. [IARC]. 2017. Some nanomaterials and some fibres. Monograph. Vol. 111. Lyon, France: IARC.
- Jaurand M. (1997). Mechanisms of fiber-induced genotoxicity. Environ Health Perspect. 105:1073–1084.
- Karami M, Ebrahimzadeh M, Mahdavi M, Kazemi A. (2010). Effect of Fe2+ and Fe3+ ions on human plasma cholinesterase activity. Eur Rev Med Pharmacol Sci. 14:897–901.
- Karjalainen A, Meurman LO, Pukkala E. (1994). Four cases of mesothelioma among Finnish anthophyllite miners. J Occup Environ Med. 51:212–215.
- Kohyama N, Shinohara Y, Suzuki Y. (1996). Mineral phases and some reexamined characteristics of the International Union Against Cancer standard asbestos samples. Am J Ind Med. 30:515–528.
- Korchevskiy AA, Rasmuson EJ, Rasmuson JO, Strode RD. 2013. Asbestos mining in Russia: approaches to public health risk assessment. Preprint 13-117 in: Mining: It’s All About People. Pre-prints of the Society for Mining, Metallurgy & Exploration Annual Meeting; February 24–27; Denver, Colorado.
- Korchevskiy AA, Rasmuson JO, Rasmuson EJ, Sanchez M. 2015. Russian anthophyllite: chemical composition and potency assessment. Proceedings of the American Industrial Hygiene Conference (AIHce), Salt Lake City, UT, May 30–June 4.
- Lambert CS, Alexander BH, Ramachandran G, MacLehose RF, Nelson HH, Ryan AD, Mandel JH. (2016). A case-control study of mesothelioma in Minnesota iron ore (taconite) miners. Occup Environ Med. 73:103–109.
- Lippmann M. 2009. Environmental toxicants: human exposures and their health effects. New Jersey: John Wiley & Sons.
- Loomis D, Dement J, Richardson D, Wolf S. (2010). Asbestos fibre dimensions and lung cancer mortality among workers exposed to chrysotile. Occup Environ Med. 67:580–584.
- Lowers HA, Adams DT, Meeker GP, Nutt CJ. 2010. Chemical and morphological comparison of erionite from Oregon, North Dakota, and Turkey. Open-File Report 2010-1286. Reston (VA): USGS.
- Meurman LO, Pukkala E, Hakama M. (1994). Incidence of cancer among anthophyllite asbestos miners in Finland. Occup Environ Med. 51:421–425.
- Moolgavkar S, Chang E, Mezei G, Mowat F. 2017. Epidemiology of mesothelioma. In Testa JR, editor. Asbestos and mesothelioma. Current cancer research. Switzerland: Springer International Publishing.
- Mossman B. 1993. Mechanisms of asbestos carcinogenesis and toxicity: the amphibole hypothesis revisited. Edit Br J Ind Med. 50:673–676.
- Mossman B, Lippmann M, Hesterberg T, Kelsey KT, Barchowsky A, Bonner JC. 2011. Pulmonary endpoints (lung carcinomas and asbestosis) following inhalation exposure to asbestos. J Toxicol Environ Health B Crit Rev. 14:76–121.
- Mustard J. 1992. Chemical analysis of actinolite from reflectance spectra. Am Miner. 77:345–358.
- National Academy of Sciences, National Research Council [NAS/NRC]. 1984. Asbestiform fibers: nonoccupational health risks. Washington, DC: National Academy Press.
- Okada S, Hamazaki S, Toyokuni S, Midorikawa O. 1989. Induction of mesothelioma by intraperitoneal injections of ferric saccharate in male Wistar rats. Br J Cancer. 60:708–711.
- Oury TD, Sporn TA, Roggli VL, editors. 2014. Pathology of asbestos-associated diseases. 3rd ed. Berlin Heidelberg: Springer-Verlag.
- Pascolo L, Gianoncelli A, Kaulich B, Rizzardi C, Schneider M, Bottin C, Polentarutti M, Kiskinova M, Longoni A, Melato M. 2011. Synchrotron soft X-ray imaging and fluorescence microscopy reveal novel features of asbestos body morphology and composition in human lung tissues. Part Fibre Toxicol. 8:7.
- Pascolo L, Gianoncelli A, Schneider G, Salomé M, Schneider M, Calligaro C, Kiskinova M, Melato M, Rizzardi C. 2013. The interaction of asbestos and iron in lung tissue revealed by synchrotron-based scanning X-ray microscopy. Sci Rep. 3:1123–1134.
- Pugnaloni A, Lucarini G, Giantomassi F, Lombardo L, Capella S, Belluso E, Zizzi A, Panico AM, Biagini G, Cardile V. 2007. In vitro study of biofunctional indicators after exposure to asbestos-like fluoro-edenite fibers. Cell Mol Biol. 53:OL965–OL980.
- Quinlan TR, Marsh JP, Janssen YMW, Borm PA, Mossman BT. 1994. Oxygen radicals and asbestos-mediated disease. Environ Health Perspect. 102:107–110.
- Rasmuson JO, Roggli VL, Boelter FW, Rasmuson EJ, Redinger CF. 2014. Cumulative Retrospective Exposure Assessment (REA) as a predictor of amphibole asbestos lung burden: validation procedures and results for industrial hygiene and pathology estimates. Inhal Toxicol. 26:1–13.
- Rood AP, Streeter RR. 1984. Size distributions of occupational airborne asbestos textile fibres as determined by transmission electron microscopy. Ann Occup Hyg. 28:333–339.
- Schwarz E, Winer A. 1971. Magnetic properties of asbestos, with special reference to the determination of absolute magnetite contents. CIM Bull. 64:55–59.
- Shedd KB. 1985. US Department of the Interior: fiber dimensions of crocidolite from Western Australia, Bolivia, and the Cape and Transvaal Provinces of South Africa. US Bureau of Mines Report of Investigations: 8998. Washington, DC: United States Department of the Interior. Bureau of Mines.
- Simonato L, Baris R, Saracci R, Skidmore J, Winkelmann R. 1989. Relation of environmental exposure to erionite fibers to risk of respiratory cancer. Vol 90. In: Bignon J, Peto J, Saracci R, editors. Non-occupational exposure to mineral fibers. Lyon, France: World Health Organization, International Agency for Research on Cancer (IARC), Scientific Publications; p. 398–405.
- Toyokuni S. 1996. Iron-induced carcinogenesis: the role of redox regulation. Free Radic Biol Med. 20:553–566.
- Tran CL, Hankin SM, Ross B, Aitken RJ, Jones AD, Donaldson K, Stone V, Tantra R, 2008. An outline scoping study to determine whether High Aspect Ratio Nanoparticles (HARN) should raise the same concerns as do asbestos fibers. Report on Project CB0406. August 13, 2008.
- Turci F, Tomatis M, Compagnoni R, Fubini B. 2009. Role of associated mineral fibres in chrysotile asbestos health effects: the case of Balangeroite. Ann Occup Hyg. 53:491–497.
- US EPA, SRC Inc. 2010. Particle size distribution data for Libby amphibole structures observed in air at the Libby Asbestos Superfund Site. US EPA Region 8, Denver, CO. July 14, 2010. [accessed 2019 Jul 9]. https://hero.epa.gov/hero/index.cfm/reference/download/reference_id/759201
- Virta RL, US Geological Survey [USGS]. 2002. Asbestos: geology, mineralogy, mining, and uses. Open-File Report 02-149. Kirk-Othmer Encyclopedia of Chemical Technology, Online Edition. New York (NY): Wiley Interscience.
- Wagner J, Skidmore J, Hill R, Griffiths D. (1985). Erionite exposure and mesotheliomas in rats. Br J Cancer. 51:727–730.
- Walitzi EM, Walter F, Ettinger K. (1989). Verfeinerung der kristallstruktur von anthophyllit vom Ochsenkogel/Gleinalpe. Österreich Zeits Für Krist. 188:237–244.
- Xu A, Wu L, Santella RM, Hei TK. (1999). Role of oxyradicals in mutagenicity and DNA damage induced by crocidolite asbestos in mammalian cells. Cancer Res. 59:5922–5926.