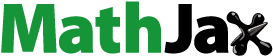
Abstract
Objective
To develop a model to identify women likely to be severely impacted by vulvovaginal atrophy (VVA), based on their experience of symptoms and non-clinical factors.
Methods
Multivariate statistics and machine-learning algorithms were used to develop models using data from a cross-sectional, observational, multinational European survey. A set of independent variables were chosen to assess subjective VVA severity and its impact on daily activities.
Results
A final composite model was selected that included three categories of variables: clinical severity, patient demographics/clinical characteristics and Day-to-Day Impact of Vaginal Aging (DIVA) variables related to emotion/mood, impact on lifestyle and frequency of sex. The model accurately classified 71% of women. Three DIVA variables (feeling bad about yourself, desire/interest in sex, physical comfort related to sitting) explained much of the variation in the dependent variable of the model. Over 90% of the impact of VVA relates to certain psychosocial and behavioral aspects that can be identified without the need to consider physical signs/symptoms.
Conclusion
Non-clinical factors can contribute significantly to the overall VVA burden.
Questions used in developing the composite model could form the basis of an instrument to help screen women prior to clinical consultation and improve VVA management.
Introduction
Vulvovaginal atrophy (VVA) is a common chronic, progressive condition affecting almost two-thirds of postmenopausal women [Citation1], with up to half reporting their VVA symptoms as moderate or severe [Citation2]. Beyond the clinical manifestations of VVA, there are broader biopsychosocial determinants that influence the level of distress associated with these symptoms and their impact on women’s wellbeing [Citation3].
Experts strongly recommend that healthcare professionals (HCPs) should proactively initiate open discussion with their postmenopausal patients about urogenital symptoms. However, very few diagnostic tools for VVA exist and those that do focus entirely on the clinical aspects of VVA, failing to capture the broader aspects of the condition [Citation3]. There is a need for better predictors of VVA, particularly simple tools that can be used by HCPs in both primary and secondary care, to help stimulate earlier conversations with patients.
The aim of this analysis was to gain a better understanding of women’s subjective experiences of VVA and develop a model to identify which women are likely to be more severely impacted by this condition, based on their experience of various clinical and non-clinical factors related to VVA.
Methods
Study sample
The model was developed using data collected from the multinational, cross-sectional, observational European Vulvovaginal Epidemiological Survey (EVES) that included 2,412 postmenopausal women aged 45–75 years attending menopause centers for a routine gynecological visit in Italy and Spain during 2015 [Citation4–6]. Among them, 2,403 women were included and were evaluable for symptom screening; 2,160 women complained of ≥1 VVA-related symptom, filled in the survey questionnaires and had an objective gynecological examination [Citation4].
Women with ≥1 VVA symptom were asked to complete a survey that included questions on demographics, lifestyle, therapies and the impact of VVA symptoms on physical status (assessed using the VulvoVaginal Atrophy Questionnaire (VVAQ), three-level EuroQol five-dimensional questionnaire [EQ-5D-3L], Vaginal Health Index [VHI] and Vulva Health Index); quality of life (assessed using the Day-to-Day Impact of Vaginal Aging questionnaire [DIVA]); and sexual life (assessed using the Female Sexual Function Index [FSFI] and the revised Female Sexual Distress Scale). A clinical gynecological assessment was performed to confirm the presence of VVA.
Model variables
It was planned to develop a model that included one dependent variable representing the subjective nature of women’s experience of VVA, together with a set of independent variables encompassing demographics, health, emotions, personal experiences and activities affected by VVA, to understand which of these variables best explained which women were likely to experience severe VVA, and the relative contribution of each variable to VVA severity.
For the dependent variable, an instrument was chosen that measured subjective severity across 19 VVA symptoms chosen by a scientific committee of experts in women’s health (). For each symptom, women were asked to select the number that best described the level of discomfort they experienced, using a four-point scale from 0 (no discomfort) to 3 (severe discomfort). A total score for all symptoms was calculated for each participant. This approach was chosen as it enabled subjective severity to be assessed via a comprehensive set of VVA-specific symptoms, unlike other scales such as the EQ-5D-3L and EuroQol Visual Analog Scale (EQVAS), that assess health more generally. The total scores for women in the study sample were well distributed. A total score of 0–14 was classed as mild VVA with ≥15 classed as moderate-to-severe. A score of 15 was chosen as the cutoff to create a binary dependent variable, with approximately 30% of participants having a total score above this value. As a further test of the robustness of the dependent variable, the final model was run with various cut-off values, ranging from 10 to 20 and continued to be stable, with few variations.
Table 1. Vulvovaginal atrophy symptoms included in the dependent variable used for model development.
A set of 37 independent variables were chosen from those included in EVES, broadly grouped into five categories: patient demographics, behaviors, medical, clinical severity and DIVA variables (). Leading indicators of VVA such as desire, quality of life (QoL) and urinary incontinence were among the items included. Clinical severity was also included to provide an objective measure of VVA. Six variables were excluded due to high multicollinearity, which would have reduced the accuracy of the model (age, feeling frustrated or resentful, ability to be spontaneous, ability to relax, amount of pleasure experienced and overall satisfaction with sex life). Missing values were imputed using averages.
Table 2. Independent variables included in the models.
Algorithms used for model development
Models were developed using logistic regression, random forest and support vector machine algorithms. Further information is provided in Appendix A. Variation between the models was evident in some significant variables. Therefore, to ensure that all relevant variables were captured in a single model, a composite model was developed using all of the significant variables from each of the earlier models as inputs.
Each model was internally validated; models were built using 30% of the data and then applied to the remaining 70% to assess how well the model performed in accurately predicting the dependent variable (i.e., total VVA symptom score). For each model, the level of accuracy in classifying VVA as mild or moderate-to-severe, and the significant variables and their relative contribution to the model, were determined.
Results
Study sample
A total of 2,403 women were included in the EVES screening sample. The demographics and clinical characteristics of these women have been described previously [Citation4]. Briefly, the mean age was 59.0 years, mean age at last menstruation was 49.1 years and mean time since menopause was 9.9 years. In total, 2,074 women (86.3%) experienced a physiological menopause, 228 (9.5%) had a surgically-induced menopause and 101 (4.2%) had a medication-induced menopause.
Of the 2,403 women included in the EVES screening sample, 2,152 answered all questions relevant to the 19 variables included in the model and were included in the current analysis.
Models
Logistic regression model
The logistic regression model had an accuracy of 70% and identified four significant variables from the following two categories: clinical severity and DIVA (desire or interest in sex, feeling bad about yourself, physical comfort related to sitting). The relative contribution of the DIVA variables varied between 28% and 36% and dominated the model, with clinical severity contributing only 7% ().
Random forest model
The random forest model had an accuracy of 67% and identified ten significant variables from the following three categories: clinical severity; demographics (height, weight, number of years since menopause, age at last menstruation) and DIVA variables (desire or interest in sex, feeling bad about yourself, able to sit for more than 1 h, use toilet, depressed or down). The relative contribution of these variables to the model ranged from 7% to 12% ().
Support vector machine model
The support vector machine model had an accuracy of 70% and identified ten significant variables from the following four categories: clinical severity; demographics (number of years since menopause, age at last menstruation), behaviors (number of times had sexual intercourse per month) and DIVA variables (desire or interest in sex, frequency of sex measured on a five-point scale, feel bad about yourself, embarrassed, depressed or down, able to sit for more than 1 h) (). The relative contribution of the ten variables ranged from 6% to 15%. In this model, the DIVA variables made a much greater contribution than in the random forest model.
Composite model
The composite model had an accuracy of 71%, and identified 13 significant variables from the following four categories: clinical severity, demographics (height, weight, number of years since menopause, age at last menstruation), DIVA variables (desire or interest in sex, frequency of sex – measured on a five-point scale, effect on frequency of sex, feel bad about yourself, embarrassed, depressed or down, able to sit for more than 1 h, use toilet) and behaviors (number of times had sexual intercourse per month). The relative contribution of the 13 variables ranged from 1% to 19%, with a high contribution of DIVA variables ().
Figure 1. Model characteristics: receiver operating characteristic curves (panels A, C, E, G) and relative contribution of variables (panels B, D, F, H). AUC: area under the curve; DIVA: day-to-day impact of vaginal aging.

The composite model was selected as the best because it had the highest accuracy and included the largest number of independent variables. Most of the subjective experience of VVA could be assessed by measures other than VHI, which is a relatively objective measure of VVA. In particular, three DIVA variables (feeling bad about yourself, desire/interest in sex and physical comfort related to sitting) explained a large part of the variation in the dependent variable. This highlights the importance and relevance of non-clinical measures in assessing VVA severity among women.
The questionnaire used to assess the variables included in the final model is shown in Appendix B. Further details of the modeling approaches used in this study are provided in Appendix A.
Discussion
VVA encompasses a cluster of symptoms including vaginal dryness, irritation, loss of elasticity, decreased lubrication, dyspareunia and urinary symptoms, which can vary according to age, duration of menopause and frequency of sexual intercourse [Citation7]. Beyond the clinical manifestations of VVA, there are broader biopsychosocial determinants that influence the level of distress associated with these symptoms and their impact on women’s wellbeing [Citation3]. VVA is a potential early marker of poor general health and has a substantial impact on women’s daily life and couple intimacy [Citation6]. Research has shown that the impact of VVA symptoms on QoL may be comparable to that of other chronic conditions such as arthritis, chronic obstructive pulmonary disease, asthma and irritable bowel syndrome [Citation8]. This is a particular concern, given that most women now live more than one-third of their lives in the postmenopausal period [Citation9,Citation10]. There is a need to raise awareness and improve the management of this condition and HCPs should be proactive in taking steps to ensure early recognition of VVA [Citation6].
Diagnostic tools for VVA are limited and often not well suited for use in routine clinical practice. Diagnosis of VVA can be confirmed objectively by pelvic examination including inspection of the vulva, vestibule, vagina and urethra, rating any signs of VVA using validated scales [Citation3,Citation11]. The VHI Score evaluates five parameters: vaginal elasticity, vaginal secretions, pH, epithelial mucous membrane and vaginal hydration, assigning a single score to each to obtain a final score that describes the degree of atrophy, with lower scores indicating greater urogenital atrophy [Citation3,Citation12]. However, a limitation of this instrument is that it does not consider the impact of VVA on the vulva or urinary tract [Citation13]. The Vulva Health Index evaluates the labia, urethra, clitoris and introitus, together with elasticity and pain during intercourse, to calculate a total score with higher scores corresponding to a greater degree of vulvar atrophy [Citation3,Citation13]. The more comprehensive genitourinary syndrome of the menopause (GSM) assessment tool consists of three categories of elasticity, lubrication and tissue integrity, a section on vulval, vaginal and urethral anatomy, and objective measures of vaginal pH and vaginal maturation [Citation14]. Each component is scored by severity, providing a semi-objective measure of VVA [Citation13,Citation14]. However, both the Vulval Health Index and the GSM tool require validation [Citation13].
Instruments that consider the patient’s perception of their condition and assess subjective outcomes include a visual analog scale, which measures VVA symptoms on a scale of 0–10 [Citation15] and various patient-reported outcome measures (PROMs) including the FSFI and the DIVA questionnaire. The FSFI assesses six domains of sexuality: desire, arousal, lubrication, orgasm, satisfaction and pain [Citation16], while the DIVA questionnaire is composed of 23 items on four scales: everyday activities, emotional well-being, sexual functioning, and self-concept and body image [Citation17]. Drawbacks of these instruments are that the information gathered is limited by the range of questions asked and they are potentially biased by the study population used for their development [Citation13].
Women with the same clinical severity of VVA may report differing perceptions of the impact this has on their QoL and relationships. To our knowledge, this is the first study to assess women’s subjective experiences of VVA, alongside an objective measure of clinical severity. The accuracy of the four models was similar, between 67% and 71%. DIVA variables, in particular those relating to desire, QoL and urinary incontinence, which are already known to be leading indicators of VVA [Citation4–6], were shown to make a major contribution to severity when assessed subjectively. The composite model was chosen as the best model as it was able to accurately classify VVA severity in 7 out of 10 women, based on 13 variables. This model was developed using logistic regression which is a statistical method that physicians are familiar with, so is easily understandable amongst the medical community.
A key strength of this research is that data used in developing the model were from a representative sample of postmenopausal women who participated in a cross-sectional, observational, multinational study. There are also several limitations. Firstly, the total score for all 19 symptoms evaluated does not necessarily equate to ‘subjective severity’; this approach was used because there was no universal and holistic measure of subjective severity in EVES. This assumes that the self-reported stress created by the symptoms is a good proxy for subjective severity, and that the effects of individual symptoms are additive. Secondly, because EVES was cross-sectional in design, the model can only describe women’s current experience of VVA, rather than predicting how likely they are to develop severe symptoms in the future.
Experts strongly recommend that HCPs should proactively initiate open discussion with their postmenopausal patients about urogenital symptoms. However, a post hoc analysis of the REal Women’s VIews of Treatment Options for Menopausal Vaginal ChangEs-Europe (REVIVE-EU) survey highlighted a lack of coherent discussion about VVA symptoms and a need to improve proactive communication between patients and HCPs [Citation18]. This model offers the potential to become a valuable tool in the clinical setting to encourage dialogue, enable a better understanding of women’s subjective experiences of VVA, and prevent progression of this chronic condition through earlier treatment.
In the Women’s EMPOWER Survey, 72% of participants had never discussed their VVA symptoms with an HCP. Of those who had, 85% reported initiating the discussion, with 41% instead preferring to fill out a questionnaire before seeing the HCP [Citation19]. There is potential to make the current instrument more usable in the clinical setting by creating a model that excludes the clinical measure (i.e. the VHI), removing the need for physical examination. Such a tool could easily be filled out prior to the HCP visit and used as an aid to initiate and guide physician-patient discussion.
The way in which this tool could be implemented clinically may vary by country and practice setting, depending on the technology available. In the post-Coronavirus disease 2019 (COVID-19) healthcare era, we continue to prioritize certain aspects of women’s health with face-to-face consultations. Therefore, there is a need for new screening tools to diagnose and manage conditions that have a significant impact on the patient but do not require urgent care and could be used as part of virtual telehealth consultations. We believe that this instrument could make a valuable addition to the growing number of ‘femtech’ tools that are using technology to address a range of women’s health issues including menstrual health, reproductive health, sexual health, maternal health and menopause [Citation20].
There are various unmet needs in VVA that remain unaddressed, notably the taboo attached to this intimate condition and the perception that the symptoms associated with VVA are an inevitable part of aging [Citation3]. However, effective management is feasible when a conversation takes place in routine practice [Citation3]. HCPs should handle this issue in a respectful manner, taking into account women’s needs and preferences, and explaining the clinical significance of reporting VVA-associated symptoms. The long-term consequences of VVA form part of geriatric genitourinary medicine and may lead to a series of investigations and repeated treatments that could be avoided with appropriate preventive strategies. An easy tool to uncover this sensitive topic has the potential to optimize appropriateness of care, and therefore to improve the QoL of postmenopausal women.
Conclusion
Women’s subjective experiences contribute significantly to the overall impact of VVA and should be a key component of assessment and treatment decision making. This model could form the basis of a quick and simple tool for use by patients in the clinical setting as an app or questionnaire prior to consultation. This will help HCPs gain a better understanding of women’s subjective experiences of VVA and could ultimately improve the management of this condition.
Author contributions
DA: planning and reporting of current analysis; VB: conceptualizing; designing, planning, conducting and reporting of current analysis; GH: conceptualizing; designing, planning, conducting and reporting of current analysis; REN: design and conduct of EVES study; planning and reporting of current analysis; MP: design of EVES study; planning and reporting of current analysis; NP: design and conduct of EVES study; planning and reporting of current analysis; SP: design and conduct of EVES study; planning and reporting of current analysis.
Acknowledgements
Medical writing support was provided by Sarah Stowell PhD, CMPP of Ashley Medical Communications, funded by Shionogi, in accordance with Good Publication Practice 3 guidance.
Disclosure statement
Dan Atkins is an employee of Shionogi Europe. Vivek Banerji, as part of Insight Dojo, has conducted commercial patient and physician insights projects for Shionogi. Genevieve Hall, as part of Insight Dojo, has conducted commercial patient and physician insights projects for Shionogi. Rossella E. Nappi declares past financial relationships (lecturer, member of advisory boards and/or consultant) with Boehringer Ingelheim, Ely Lilly, Endoceutics, Gedeon Richter, HRA Pharma, Procter & Gamble Co, TEVA Women’s Health Inc and Zambon SpA. At present, she declares ongoing relationships with Astellas, Bayer HealthCare AG, Exceltis, Fidia, Merck Sharpe & Dohme, Novo Nordisk, Palatin Technologies, Pfizer Inc, Shionogi Limited and Theramex. Nick Panay has lectured and/or acted in an advisory capacity for Abbott, Besins, Lawley, Mithra, Mylan, Novo Nordisk, Roche Diagnostics, Se-Cur, Shionogi and Theramex. Santiago Palacios declares financial relationships (lecturer, member of advisory boards and/or consultant) with Ely Lilly, Gedeon Richter, Teva Women’s Health, Bayer HealthCare AG, Exceltis, Merck Sharpe & Dohme, Novo Nordisk, Amgen, Pfizer Inc, Shionogi Limited, Procare, Tecnofarma, Abbott, Mithra, Lacer, Procaps and Theramex. Martire Particco was an employee of Shionogi Europe at the time the study was conducted.
Additional information
Funding
References
- Naumova I, Castelo-Branco C. Current treatment options for postmenopausal vaginal atrophy. Int J Womens Health. 2018;10:1–8. doi: 10.2147/IJWH.S158913.
- Nappi RE, Biglia N, Cagnacci A, et al. Diagnosis and management of symptoms associated with vulvovaginal atrophy: expert opinion on behalf of the Italian VVA study group. Gynecol Endocrinol. 2016;32(8):602–606. doi: 10.1080/09513590.2016.1183627.
- Nappi RE, Martini E, Cucinella L, et al. Addressing vulvovaginal atrophy (VVA)/genitourinary syndrome of menopause (GSM) for healthy aging in women. Front Endocrinol. 2019;10:561. doi: 10.3389/fendo.2019.00561.
- Palacios S, Nappi RE, Bruyniks N, et al. The European Vulvovaginal Epidemiological Survey (EVES): prevalence, symptoms and impact of vulvovaginal atrophy of menopause. Climacteric. 2018;21(3):286–291. doi: 10.1080/13697137.2018.1446930.
- Particco M, Djumaeva S, Nappi RE, et al. The European Vulvovaginal Epidemiological Survey (EVES): impact on sexual function of vulvovaginal atrophy of menopause. Menopause. 2020;27(4):423–429. doi: 10.1097/GME.0000000000001496.
- Palacios S, González SP, Fernández-Abellán M, et al. Impact of vulvovaginal atrophy of menopause in Spanish women: prevalence and symptoms according to the EVES study. Sex Med. 2019;7(2):207–216. doi: 10.1016/j.esxm.2019.01.005.
- Nappi RE, Particco M, Biglia N, et al. Attitudes and perceptions towards vulvar and vaginal atrophy in Italian post-menopausal women: evidence from the European REVIVE survey. Maturitas. 2016;91:74–80. doi: 10.1016/j.maturitas.2016.06.009.
- DiBonaventura M, Luo X, Moffatt M, et al. The association between vulvovaginal atrophy symptoms and quality of life among postmenopausal women in the United States and Western Europe. J Womens Health. 2015;24(9):713–722. doi: 10.1089/jwh.2014.5177.
- Palacios S, Henderson VW, Siseles N, et al. Age of menopause and impact of climacteric symptoms by geographical region. Climacteric. 2010;13(5):419–428. doi: 10.3109/13697137.2010.507886.
- Nappi RE, Palacios S. Impact of vulvovaginal atrophy on sexual health and quality of life at postmenopause. Climacteric. 2014;17(1):3–9. doi: 10.3109/13697137.2013.871696.
- Nappi RE. New attitudes to sexuality in the menopause: clinical evaluation and diagnosis. Climacteric. 2007;10(Suppl. 2):105–108. doi: 10.1080/13697130701599876.
- Bachmann GA, Notelovitz M, Kelly SJ, et al. Long-term non-hormonal treatment of vaginal dryness. Clin Pract Sexuality. 1992;8:3–8.
- Panay N. Genitourinary syndrome of the menopause–dawn of a new era? Climacteric. 2015;18(Suppl. 1):13–17. doi: 10.3109/13697137.2015.1070564.
- Portman DJ, Gass ML, Vulvovaginal Atrophy Terminology Consensus Conference Panel. Genitourinary syndrome of menopause: new terminology for vulvovaginal atrophy from the international society for the study of women’s sexual health and the North American menopause society. Climacteric. 2014;17(5):557–563. doi: 10.3109/13697137.2014.946279.
- Mension E, Alonso I, Tortajada M, et al. Genitourinary syndrome of menopause assessment tools. J Midlife Health. 2021;12(2):99–102.
- Rosen R, Brown C, Heiman J, et al. The Female Sexual Function Index (FSFI): a multidimensional self-report instrument for the assessment of female sexual function. J Sex Marital Ther. 2000;26(2):191–208.
- Huang AJ, Gregorich SE, Kuppermann M, et al. The day-to-day impact of vaginal aging questionnaire: a multidimensional measure of the impact of vaginal symptoms on functioning and well-being in postmenopausal women. Menopause. 2015;22(2):144–154. doi: 10.1097/GME.0000000000000281.
- Domoney C, Short H, Particco M, et al. Symptoms, attitudes and treatment perceptions of vulvo-vaginal atrophy in UK postmenopausal women: results from the REVIVE-EU study. Post Reprod Health. 2020;26(2):101–109. doi: 10.1177/2053369120925193.
- Kingsberg SA, Krychman M, Graham S, et al. The women’s EMPOWER survey: identifying women’s perceptions on vulvar and vaginal atrophy and its treatment. J Sex Med. 2017;14(3):413–424. doi: 10.1016/j.jsxm.2017.01.010.
- Yoldemir T. Telemedicine and women’s health. Climacteric. 2022;25(5):425–426. doi: 10.1080/13697137.2022.2106725.
Appendix A.
Modeling approach methodology
There were four stages in developing the models for predicting a women’s subjective experience of VVA. These were:
Identification of a dependent variable.
Identification of potential independent variables that could be used to predict the dependent variable.
Creating mathematical models that demonstrate how the independent variables relate to the dependent variable, and how the former can be used to predict the latter.
A comparison of the different mathematical models to understand which is able to predict the dependent variable with the highest level of accuracy.
Identification of the dependent variable
The purpose of this exercise was to create a model to predict a woman’s subjective experience of VVA. As such, the dependent variable selected needed to capture women’s personal experience of the condition.
The dependent variable ultimately selected for the modeling was based on a composite score calculated from women’s responses across 19 questions relating to VVA symptoms. For each symptom, women were asked to select the number that best described the level of discomfort they experienced, using a four-point scale from 0 (no discomfort) to 3 (severe discomfort). A total score for all symptoms was calculated for each participant.
A total score of 0–14 was classed as mild VVA with ≥15 classed as moderate-to-severe. A score of 15 was chosen as the cutoff to create a binary dependent variable, with approximately 30% of participants having a total score above this value. Based on the analysis above, each woman was assigned a value of either ‘mild’ or ‘moderate-to-severe’. This became the dependent variable for the modeling.
This approach was chosen as it enabled subjective severity to be assessed via a comprehensive set of VVA-specific symptoms, unlike other scales such as the EQ-5D-3L and EQVAS, that assess health more generally.
Identification of the independent variables
A set of 37 potential independent variables were chosen from those included in EVES, broadly grouped into five categories: patient demographics, behaviors, medical, clinical severity and DIVA variables.
These 37 variables were used as inputs to the model development, although only a subset of the 37 ultimately proved to be significant in the final models.
Creating the mathematical models
Having identified the dependent variable and the set of potential independent variables, these could then be used as inputs to develop the mathematical models. These models demonstrate how the independent variables relate to the dependent variable, and how the former can be used to predict the latter.
Three different approaches were used to model the data: logistic regression, random forest and support vector machines. For each approach, a ‘training set’ consisting of 70% of the sample was used to develop the initial model. The initial model was then tested on the remaining 30% of the sample (known as the ‘test set’) to understand how well the model predicted the dependent variable on a ‘new’ set of data. This is an approach commonly used to ensure that a model is valid outside the set of data on which it was built. When the model is tested on the ‘test set’, an accuracy score is calculated. This is expressed as a percentage and relates to the proportion of the data set that is accurately classified by the model.
The output from the model development is a ‘contribution score’ for each of the independent variables that the model finds important in predicting the dependent variable. The contribution scores allow us to see which independent variables have a significant impact on the dependent variable, and to what extent.
The three approaches used to model the data – logistic regression, random forest and support vector machines – differ in terms of their underlying mathematical approach. These are described below.
Logistic regression
Logistic regression is a widely accepted, traditional approach that assumes a logistic curve, and predicts the probabilities of belonging to one category or the other based on the independent variables.
Logistic regression is a modeling approach used for ‘classification’ problems – that is, when you wish to predict whether something (in this case the women) belongs to a specific class (in this case, ‘moderate-to-severe’ versus ‘mild). Logistic regression is different to linear regression, which is used when you wish to predict a continuous value/score rather than membership of a specific class.
Logistic regression looks at the interaction between certain independent variables and a dependent variable, and creates a model that calculates a probability that a particular observation will fit into a category based on its values across the independent variables. In the context of this specific exercise, the logistic model would predict how likely a woman would be to have ‘moderate-severe’ VVA based on her responses across the independent variable questions.
Logistic regression uses a logistic function to model a binary dependent variable.
When the model is run, a formula like the following can be created:
where X1 to XK are the values of the important independent variable, and θ0- θK are output parameters from the modeling process (that are optimized to produce a model with the best accuracy).
For any new observation, the model calculates the probability that the observation fits into a certain category, depending on the values across that observation’s independent variables. The following function provides that probability:
where Z = θ0 + θ1 X1 + θ2 X2 + … + θK XK
If F(z) > 0.5, then the observation is said to fit into the category that has been defined.
In this specific example, an F(z) of over 0.5 would mean that a woman would be predicted as having ‘moderate-to-severe’ VVA.
In this particular study, a logistic model was run using the 37 independent variables, with the dependent variable relating to a women’s subjective experience of VVA. The logistic model was able to predict whether a woman had ‘moderate-to-severe’ VVA (versus mild VVA) with an accuracy of 70%, based on 4 of the 37 independent variables.
Random forest
Random forest is an ensemble machine-learning method that predicts the probability of belonging to a category by creating a large number of decision trees to understand the relative importance of different independent variables in predicting the dependent variable. It creates a large number of decision trees and compares the results of each to come to a consensus of what the best answer is. The idea is that combining multiple trees increases the stability and accuracy of a prediction.
Decision trees are the fundamental building blocks of random forest modeling. The notion of a decision tree is that there are certain questions that can be asked that get you closer to classifying an observation in a particular way, and that these questions vary in terms of their importance. In the context of the problem at hand, this would translate into understanding which of the independent variables are most important in accurately predicting whether a woman has ‘moderate-severe’ or ‘mild’ VVA.
There are various algorithms that are used to generate decision trees and establish the importance of different independent variables. One such approach is ‘CART’ or ‘classification and regression trees’. This approach calculates a ‘Gini index’ for each independent variable – a figure that represents the importance of the independent variable in predicting the dependent variable. The random forest model then compares the ‘Gini index’ for each independent variable across multiple decision trees to arrive at a final contribution score for that variable.
In this particular study, a random forest was run using the 37 independent variables, with the dependent variable relating to women’s subjective experience of VVA. The random forest model was able to predict whether a woman had ‘moderate-to-severe’ VVA (vs mild VVA) with an accuracy of 67%, based on 10 of the 37 independent variables.
Support vector machines
Support vector machine is a machine-learning algorithm that can be used for either ‘classification’ (where you are trying to predict the membership of a particular category) or ‘regression’ problems (where you are predicting a continuous value or score).
In the case of a ‘classification’ problem, a support vector machine model will assign a new observation to one category or another. In the case of the question in hand, this would be assigning a woman to either the ‘moderate-to-severe’ VVA or ‘mild’ VVA group.
The objective of a support vector machine algorithm is to find a hyperplane in an N-dimensional space (where n is the number of independent variables) that distinctly classifies two sets of data points – in this case, those that represent women with ‘moderate-to-severe’ VVA, and those that represent women with ‘mild’ VVA. As there are theoretically many different planes that can separate two sets of data points, the objective of support vector machine modeling is to find the plane where the distance between the data points and the plane is at its maximum. This corresponds to the optimal means of classifying the data.
Once the optimal hyperplane has been established, is it possible to make a prediction of which group a new observation belongs to, based on where it sits in comparison to the plane.
In this particular study, a support vector machine model was run using the 37 independent variables, with the dependent variable relating to women’s subjective experience of VVA. The support vector machine model was able to predict whether a woman had ‘moderate-to-severe’ VVA (versus mild VVA) with an accuracy of 70%, based on 10 of the 37 independent variables.
Following the development of the three models described above, a fourth ‘composite’ model was developed. This used a set of independent variables that were shown to be important in the three original models as the input and was based on a logistic regression modeling approach. This composite model was able to predict whether a woman had ‘moderate-to-severe’ VVA (versus mild VVA) with an accuracy of 71%, based on 13 of the 37 independent variables.
Comparing the models
Once the four models had been created, the accuracy levels of each were compared. The model with the highest accuracy was the composite model, with an accuracy of 71%. Based on this, the composite model was selected as the optimal.
Appendix B.
Questionnaire for use to assess variables included in the final model
Clinical severity of VVA
Weight
____________Kgs
Height
____________cm
Age at last menstruation
________years
Desire/interest in sex (DIVA)
During the past four weeks, have vaginal symptoms such as dryness, soreness, irritation or itching affected your desire or interest in having sexual intercourse or other types of sexual activity (including self-stimulation or masturbation)?
○ Not at all ○ A little bit ○ Moderately ○ Quite a bit ○ Extremely
Frequency of sex (DIVA)
During the past 4 weeks, have vaginal symptoms such as dryness, soreness, irritation, or itching affected how frequently you had sexual intercourse or other types of sexual activity (including self-stimulation or masturbation)?
○ Not at all ○ A little bit ○ Moderately ○ Quite a bit ○ Extremely
Depressed or down
During the past 4 weeks, how often have vaginal symptoms such as dryness, soreness, irritation, or itching caused you to feel depressed or down?
○ Never ○ Rarely ○ Sometimes ○ Fairly often ○ Very often
Embarrassed
During the past 4 weeks, how often have vaginal symptoms such as dryness, soreness, irritation, or itching caused you to feel embarrassed?
○ Never ○ Rarely ○ Sometimes ○ Fairly often ○ Very often
Bad about yourself
During the past 4 weeks, how often have vaginal symptoms such as dryness, soreness, irritation, or itching caused you to feel bad about yourself?
○ Never ○ Rarely ○ Sometimes ○ Fairly often ○ Very often
Frequency of sex
Are you currently sexually active? ○ NO Stop date: ___________
○ YES Intercourse/week: ________
Intercourse/month: ________
Intercourse/year: ________
Use the toilet
During the past 4 weeks, how much have vaginal symptoms such as dryness, soreness, irritation, or itching made it uncomfortable for you to or interfered with your ability to use the toilet or wipe yourself after using the toilet?
○ Not at all ○ A little bit ○ Moderately ○ Quite a bit ○ Extremely
Sit for more than 1 h
During the past 4 weeks, how much have vaginal symptoms such as dryness, soreness, irritation, or itching made it uncomfortable for you to or interfered with your ability to sit for more than an hour?
○ Not at all ○ A little bit ○ Moderately ○ Quite a bit ○ Extremely