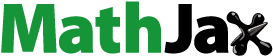
Abstract
Objective
We aimed to screen and construct a predictive model for pregnancy loss in polycystic ovary syndrome (PCOS) patients through machine learning methods.
Methods
We obtained the endometrial samples from 33 PCOS patients and 7 healthy controls at the Reproductive Center of the Second Hospital of Lanzhou University from September 2019 to September 2020. Liquid chromatography tandem mass spectrometry (LCMS/MS) was conducted to identify the differentially expressed proteins (DEPs) of the two groups. Gene Ontology (GO) as well as Kyoto Encyclopedia of Genes and Genomes (KEGG) enrichment analysis were performed to analyze the related pathways and functions of the DEPs. Then, we used machine learning methods to screen the feature proteins. Multivariate Cox regression analysis was also conducted to establish the prognostic models. The performance of the prognostic model was then evaluated by the receiver operating characteristic (ROC) curve, calibration curve, and decision curve analysis (DCA). In addition, the Bootstrap method was conducted to verify the generalization ability of the model. Finally, linear correlation analysis was performed to figure out the correlation between the feature proteins and clinical data.
Results
Four hundred and fifty DEPs in PCOS and controls were screened out, and we obtained some pathways and functions. A prognostic model for the pregnancy loss of PCOS was established, which has good discrimination and generalization ability based on two feature proteins (TIA1, COL5A1). Strong correlation between clinical data and proteins were identified to predict the reproductive outcome in PCOS.
Conclusion
The model based on the TIA1 and COL5A1 protein could effectively predict the occurrence of pregnancy loss in PCOS patients and provide a good theoretical foundation for subsequent research.
Introduction
Polycystic ovary syndrome is a complicated reproductive endocrine disorder with an overall prevalence ranging from 6% to 10% according to the different diagnostic criteria [Citation1–3]. PCOS not only causes oligo- or anovulation but also leads to adverse pregnancy [Citation4,Citation5]. It was reported that pregnancy loss is closely associated with the comorbidities of PCOS, such as obesity, metabolic syndrome, hyperinsulinemia, and hyperandrogenism [Citation6]. Pregnancy loss also brings enormous mental and economic pressure to patients. Current research on PCOS mainly focused on improving ovulatory function, whereas the mechanisms associated with adverse fertility are rarely mentioned and studied [Citation7,Citation8]. Therefore, the mechanism of pregnancy loss in PCOS is urgently in need of further exploration.
The crucial factors for reproductive success are the embryo, endometrium, and the cross-talk between them [Citation9,Citation10]. Currently, a plethora of studies have demonstrated that endometrial dysfunction may increase the risk of pregnancy complications in PCOS [Citation5,Citation10]. Abnormalities in the decidualization may induce implantation failure, miscarriage, pre-eclampsia, and premature delivery [Citation5,Citation10]. In recent years, studies have identified that intrinsic abnormalities in endometrium of women with PCOS could compromise embryo implantation by endometrial gene expression [Citation11,Citation12]. We have already established a proteome database and constructed a prognostic model focused on ferroptosis protein which can be used to predict pregnancy outcomes in PCOS [Citation13]. This inspired us to explore a prognostic model for pregnancy outcome in PCOS using machine learning further.
Machine learning research is developing rapidly and has become an important component of artificial intelligence. Meanwhile, machine learning has been a hot-topic in implementing data mining in the medical field [Citation14]. Moreover, machine learning algorithms have proved to be of great value in PCOS [Citation15,Citation16]. This study aims to use machine learning methods to remine previous proteomic data, screen the most critical feature proteins, and construct a prognostic model for predicting the pregnancy outcome, providing a theoretical foundation for the subsequent research and treatment of PCOS.
Materials and methods
Samples collection
The proteome dataset of endometrium samples collected from the Reproductive Center of the Second Hospital of Lanzhou University from September 2019 to September 2020. This dataset included 33 PCOS patients and 7 normal control subjects aged from 21 to 40 years. The patients who were recruited have to meet the Rotterdam criteria, with at least two of the three following criteria [Citation1,Citation17]: oligo- and/or anovulation [Citation2]; clinical and/or biochemical signs of hyperandrogenism [Citation3]; polycystic ovaries. The exclusion criteria were included [Citation1]: Subjects suffer from hypothyroidism, hyperprolactinemia, adrenal disease, hypertension, and diabetes [Citation2]; hormone-medication and drugs affecting glucose metabolism in the last 6 months. The control group was non-PCOS with successful pregnancy and live birth but without pregnancy loss history. They had regular menstrual cycles and normal ovarian morphology via routine ultrasound scans. The informed permission was signed from all the participants before collecting samples. The study was authorized by the Ethics Committee of Lanzhou University Second Hospital (2017 A-057).
The endometrial samples of the proliferative phase were obtained by a Pipelle endometrial aspirator and stored at −80 °C.
Clinical and prognosis data collection
Demographic characteristics collected from outpatient medical records including age and body mass index (BMI), which was calculated as weight in kilograms divided by the square of height in meters. Serum samples were collected at 2nd to 5th day of the menstrual cycle, which were used for analyzing biochemical indicators, coagulation index, and sex hormones. The biochemical indicators include serum lipids concentration, fasting plasma glucose, insulin levels, thyroid hormone, homocysteine, vitamin D3, and CA125. We mainly tested D-Dimer for coagulation index, hormones include basal testosterone (T), basal luteinizing hormone (LH), basal follicle-stimulating hormone (FSH), and the anti-mullerian hormone (AMH). The insulin resistance index (IR) is calculated by the HOMA-IR index, which was calculated as fasting plasma glucose (FPG) (mmol/l) fasting insulin (FINS) (l U/ml)/22.5, and a value of >2.6 was considered IR [Citation18]. Endometrial thickness (ET) was examined by the transvaginal ultrasound.
Reproductive outcomes and gestational duration were used as prognostic data, reproductive outcomes were presented as live birth and the gestational time of the adverse complication. Gestational time was estimated in weeks.
Protein samples preparation
Samples were first homogenized by MP FastPrep-24 homogenizer (24 × 2, 6.0 M/S, 60 s, twice), and then SDT buffer (4% SDS, 100 mM DTT, 150 mM Tris-HCl, pH 8.0) was added. The lysates were further sonicated (this step can be skipped for protein solution), and boiled for 15 min. After centrifuged at 14,000 g for 40 min, the supernatant was quantified with the BCA Protein Assay Kit (Bio-Rad, USA). The sample was stored at −80 °C. An equal aliquot from each sample in this experiment was pooled into one sample in this experiment was pooled into one sample for data dependent acquisition (DDA) library generation and quality control.
Generation of data-dependent acquisition library database
All samples were mixed for sample enzymatic hydrolysis and HPRP grading and subjected to DDA mass spectrometry data collection and library identification. A library was constructed using Spectraut pulsar X software as a database for subsequent data independent acquisition (DIA) quantification.
DIA total qualitative and quantitative data statistics
Each sample in the project is independently prepared for sample preparation and protein enzymatic hydrolysis and then subjected to DIA analysis on the computer. The obtained DIA raw file is imported into SpectrautPulsarX for analysis, and the total qualitative and quantitative quantities of all samples are obtained. We have provided a detailed experimental process (Supplementary Document 1).
Obtaination of the DEPs and the functional enrichment analysis of DEPs
The differential expression protein analysis was based on R package (limma). The screening criteria were Log fold change (LogFc) > 0.585 and adjusted p < 0.05. In order to comprehensively understand the function, localization, and biological pathways involved in proteins in organisms, Gene Ontology (GO) is used to annotate proteins. GO functional annotations are mainly divided into three categories: biological process (BP), molecular function (MF), and cellular component (CC) [Citation19]. KEGG is a database composed of researchers who have read a large amount of literature and organized numerous metabolic pathways in specific graphical languages. It includes pathway information from various aspects such as metabolism, genetic information processing, environmental information processing, cellular processes, biological systems human diseases, and drug development and is commonly used in pathway research [Citation20].
Identification of the feature proteins related to reproductive outcomes
To screen out the feature proteins, Kaplan–Meier analysis (KM) was used to identify the proteins related to fertility outcomes. We conducted a metascape analysis of these proteins. Metascape (http://metascape.org) integrates more than 40 gene function databases and supplies various visualization methods, allowing readily gene function analysis [Citation21]. Then, Identification of feature proteins by integrating multiple machine learning algorithms on the proteins selected by KM analysis. In the support vector machine (SVM), the optimal variables are identified by deleting the feature vectors generated by SVM. The ‘e1071’ package was conducted to establish the SVM model for screening out the proteins with the minimum cross-validation error. Random forests are a collection of computer-generated decision trees. The ‘randomForest’ package was applied to establish the RF model for figuring out the number of random forest trees with the minimum error. The generalized linear model (GLM) is a generalization of linear regression that allows for dependent variables to have error distributions other than a normal distribution [Citation22]. The extreme gradient boosting algorithm is a machine learning model that achieves a stronger learning effect by integrating multiple weak learners. The XGBoost model has many advantages such as strong flexibility and scalability [Citation23]. The GLM and XGB models were established in R. We hoped to select the top 2 models with minimum residual and maximum AUC for subsequent analysis by comparing four machine learning algorithms. To further identify more reliable characteristic proteins, we conducted LASSO regression algorithm. The ‘glmnet’ package was used to construct the LASSO model with penalty parameter tuning conducted by 10-fold cross-validation. Finally, the intersection proteins of the three models are used feature proteins for subsequent analysis.
Establishment and evaluation of a prognostic model for feature proteins
Multivariate Cox regression analysis was conducted to establish prognostic risk model, forward and backward method for filtering models. The risk score outcome prediction was evaluated by the feature signature (FeaSig) formula as follows: FeaSig (PCOS) = [Citation13,Citation24]. FeaSig (PCOS) represents a prognostic risk score,
represents the risk coefficient of ith prognostic feature protein.
is the expression level of the prognostic protein for the patient. The PCOS samples were divided into high risk and low risk groups. The KM method was used to estimate the reproductive outcomes of different groups.
ROC curves were performed to evaluate the FeaSig Risk Model, the decision curve analysis (DCA) was used to assess the net benefits with the risk model, and then the bootstrap method was conducted to evaluate the generalization ability [Citation25,Citation26].
Statistical analysis
The stataSE 15.0 software was conducted to calculate clinical data. We used R software to analyze the proteomic data. The results were shown as mean ± standard deviation (SD) or median (interquartile range). The G-Power was used to calculate post hoc power to evaluate if the power reaches 0.8 in our study. The Cox regression model was performed to develop a FeaSig Risk Model. All statistical tests were two-sided, and p values of < 0.05 were considered significant.
Result
Clinical and prognosis data analysis
Clinical characteristics were significantly different (p < 0.05) between PCOS patients and controls except age (p = 0.37) (). The BMI, AMH, FSH, LH, LH/FSH, T, FPG, Insulin, and Homa-IR of the PCOS group were significantly higher than those of the control group. The ET of the PCOS is thinner than control. The live birth between PCOS and the control group were significant (p < 0.05). PCOS pregnancy loss and nonpregnancy loss groups are shown in . However, so as to the small sample size, we did not see any statistically significant indicators in . But, the comparison between PCOS pregnancy loss, nonpregnancy loss, and control group had significant difference (Supplementary Table 1). BMI, AMH, LH, FPB, Insulin, and Homair-IR in pregnancy loss were significantly higher than other groups. ET was significantly thinner than other groups.
Table 1. Participant clinical characteristics of patients with PCOS and control.
Table 2. Clinical data analysis in PCOS pregnancy loss and nonpregnancy loss.
This study was a secondary analysis of proteomics, and it was necessary to calculate the sample power. We performed post hoc power calculations using observed effect sizes. The median power was 0.47 did not reach the current standard of power, 0.8. Some studies have shown that the power threshold of 0.8 is rarely achievable in some disciplines, such as surgery [Citation27,Citation28]. Due to the specificity of proteomic data, there should also be rights standards applicable to omics.
Identification and enrichment of DEPs
A total of 450 proteins were identified by hierarchical clustering analysis with significantly differential expression (Supporting Information Figure S1(A)). The R software was used to perform enrichment analysis on 450 differentially expressed proteins (DEPs), as shown in Supporting Information Figure S1(B). The biological process involved cellular process, metabolic process, and biological regulation. The molecular function analysis showed that most of proteins were involved in binding, catalytic activity, and structural molecule activity. The cellular component mainly focused on cell part, cell, organelle, and membrane. KEGG pathway analysis also revealed that up-regulated pathways were glycerophospholipid metabolism, proximal tubule bicarbonate reclamation, pantothenate and CoA biosynthesis, and histidine metabolism. On the contrary, the down-regulated pathways were circadian rhythm, influenza A, gap junction, and synthesis and degradation of ketone bodies (Supporting Information Figure S1(D)). The protein–protein interaction network of DEPs is shown in Supporting Information Figure S1(C).
Screening for feature proteins via machine learning
To further investigate the endometrial mechanism of pregnancy loss in PCOS, machine learning was used to screen feature proteins. Based on 450 differentially expressed proteins from the proteome database as variables. Thirty-nine DEPs are remarkably correlated with fertility outcomes through Kaplan–Meier analysis. The heatmap and correlation are shown in Supporting Information Figure S2(A, B). Subsequently, metascape analysis enriched pathways like viral infection pathways, HCMV infection, and Epstein–Barr virus infection (Supporting Information Figure S2(C)). This indicates that pregnancy loss in PCOS might be related to inflammation. We used R software to perform four machine learning analyses (SVM, RF, XGB, GLM) on 39 DEPs associated with pregnancy loss (Supporting Information Figure S3(A, B)). We selected the two models (SVM, RF) with the smallest residual and the largest AUC. The SVM and RF models are shown in Supporting Information Figure S3(C–E). The top five proteins with the highest importance score were used for subsequent analysis. For further analysis, The LASSO regression was performed on the aforementioned 39 proteins, resulting in the identification of nine significantly correlated proteins (Supporting Information Figure S3(F, G)). To identify the most valuable protein, we intersected the proteins obtained from three models, and two significant proteins were obtained finally (Supporting Information Figure S3(H)).
Establishment and evaluation of the risk prognostic model
Prognostic data from 33 PCOS patients and two feature proteins were used to construct the FeaSig risk prognostic model. Multivariate Cox regression analysis was performed to establish FeaSig Risk Model presented as the nomogram (). The risk score calculation formula can be obtained: FeaSig (PCOS) = –0.85991* expr (TIA1) + (–0.53855) *expr (COL5A1). We found that TIA1 and COL5A1 have a negative coefficient which indicated it could be a protective factor for live birth (). The differences of the two proteins between pregnancy loss and nonpregnancy loss groups are plotted in (). Then, the PCOS subjects were divided into high and low risk groups. Survival analysis showed that the reproductive outcomes of low risk group were consistently better than the high risk group (). With the increasing of risk score, the pregnancy outcomes deteriorate significantly. Then, the FeaSig Risk Model that incorporated the above independent predictors was developed.
Figure 1. Establishment and Evaluation of the Risk Prognostic Model: A) Nomogram to estimate the probability of pregnancy outcome of P COS use feature proteins; B) Forest plots of two feature proteins identified by multivariate Coxregression analysis; C-D) Expression of two feature proteins in pregnancy loss and NO-pregnancy loss; E) Survival analysis between high and low-risk groups; F) The ROC curve use to evaluation of the risk model. G) Decision curve analysis for the Risk model; H-I) The calibration curve of nomogram.

TimeROC package was used to test the model (). The FeaSig Risk Model’s AUC was 0.94. This proved that FeaFig model have superior prognostic performance. Consistently, the DCA for the nomogram is presented in . As the DCA indicates, the nomogram model has an obvious net benefit for almost all threshold probabilities. shows the calibration curve, which was evaluated in FeaSig Risk model. The nomogram was calibrated, and no significant difference was found between the predicted and the observed probability. To evaluate the generalization ability of the model, we conducted the bootstrapping which indicated that the results were similar to model (), and this can confirm that our model has excellent generalization ability further.
Clinical data (p ≤ 0.1 between pregnancy loss and nonpregnancy loss in PCOS) also has a good predictive performance (). Linear analysis was conducted between clinical data and feature proteins (). Most of our clinical data are negatively correlated with feature proteins, while TG and COL5A1, T4 and TIA1 are positively correlated.
Discussion
PCOS is often accompanied by metabolic comorbidities of IR, hyperinsulinemia, obesity and chronic low-grade inflammation [Citation29], and these phenotypes can affect the pregnancy outcome of PCOS [Citation6]. Studies demonstrated that the endometrium of women with PCOS have hyper-E and -A responsiveness, P-resistance and an inhospitable and inflammatory environment which can induce to poor pregnancy outcomes [Citation30]. Therefore, different pregnancy outcomes of the different phenotypes of PCOS may be due to the ‘endometrial factors’. In this study, we found that clinical data have a good predictive effect on the pregnancy outcome of PCOS, however, it is more valuable to find key factors that are decisive for the pregnancy outcome through proteomics or transcriptome for disorder prediction, and our research has also confirmed this.
We found that DEPs related to pregnancy outcomes are involved in Viral infection pathways, HCMV infection, Epstein–Barr virus infection. Interestingly, a great number of studies suggested that obesity grade and hyperandrogenism are related to severe infection of COVID-19 [Citation31,Citation32]. Patients with PCOS were more susceptible to severe COVID-19 infection. Our research is consistent with previous studies and further indicates that pregnancy loss in PCOS patients is correlated with chronic low-grade inflammation.
Poor pregnancy outcomes in PCOS are disappointing and depressing for every patient and doctor. Early diagnosis and intervention of PCOS may play an essential role in decreasing or preventing the pregnancy loss rate. However, few predictive models could accurately predict the pregnancy outcomes of PCOS. We screened two feature proteins (TIA1, COL5A1) based on machine learning methods and constructed a prognostic risk model, which may be of great value to the clinical working, assisting the PCOS and doctors to develop treatment strategies together ahead of time and reducing the pregnancy loss rate further. Interestingly, compared to our previous ferroptosis proteins model [Citation13] which used five feature proteins with an AUC of 0.884, machine learning obtained two proteins with an AUC of 0.94 which is sufficient to demonstrate the advantages of this model.
TIA1 and COL5A1 have been shown to be highly expressed in the endometrium and placenta [Citation33,Citation34]. T-cell intracellular antigen (TIA1) is an ARE-binding protein that acts as a translational silencer for proinflammatory genes including TNF-α, IL-1β, IL-6, and COX-2 [Citation35,Citation36]. TIA1 can selectively bind and down-regulate the expression of mRNA encoding immune mediators which are expressed in the endometrium [Citation33]. The decreased TIA1 expression contributes to the inflammatory environment in the peritoneum and intrauterine cavity [Citation33]. A meta-analysis also suggests that the down regulation of TIA1 could be responsible for ulcerative colitis [Citation37]. In this study, we found that the down-regulation of TIA1 in the pregnancy loss group may be a key factor in pregnancy loss in PCOS. This indicates that the down-regulation of TIA1 led to increased inflammation of the endometrium, which changed the receptive capacity of the endometrium and was not conducive to pregnancy. COL5A1 (collagen type V alpha 1 chain) is a kind of collagen which rarely studied in PCOS. Animal experiments have demonstrated that the levels of COL5A1 transcripts were elevated in the gravid horn of placentas from older mares compared to those from younger mares. Additionally, a significant increase in thickness of connective tissue within the chorionic plate was observed in the gravid horn of older mares when compared to that of younger mares [Citation34]. Regrettably, this study has a small sample size and does not explain the differences in pregnancy outcomes between older mares and younger mares. However, it suggests a significant relationship exists between COL5A1 and the placenta at least. An article studying changes in the structural integrity of fetal membranes during intrauterine inflammation found a trend toward decreased mRNA of collagen subunit COL5A1 after time-mated ewes received intra-amniotic saline lipopolysaccharide [Citation38]. We can speculate that COL5A1 may determine the pregnancy outcome of PCOS by affecting endometrium receptivity to the placenta this is still necessary to be investigated further.
The studies have shown that when age, BMI, and embryo quality are controlled, patients with PCOS-IR have lower implantation and clinical pregnancy rates than non-IR patients [Citation39]. Insulin inhibits apoptosis and promotes cell proliferation, and induces endometrial hyperplasia [Citation40]. IR decreases in nitric oxide (NO) and increases in endothelin-1 (ET-1) in arterial endothelial cells [Citation41]. This suggested that excessive insulin and high IR could be the reasons for pregnancy loss in PCOS patients. Many PCOS patients are also overweight or obese, with obesity or higher BMI exhibiting adverse pregnancy outcomes [Citation42]. PCOS patient typically suffer from metabolic syndrome, but dysregulation of fat metabolism characterized by lipid accumulation and significant increase in TG levels can induce low grade inflammation [Citation43,Citation44], which may be the cause of poor pregnancy. According to large epidemiological studies, thyroid disorders in pregnancy are associated with spontaneous abortions and preterm birth [Citation45].
The different phenotypes of PCOS have different pregnancy outcomes. We compared clinical data (IR, BMI, TG, Insulin, T4) with feature proteins. The results revealed that clinical data also have a good AUC. Then, we conducted linear analysis to investigate the relationship between various phenotypes of PCOS and feature proteins. What we identified are also concordant with other studies. For instance, a study [Citation46] has demonstrated that expression of TIA1 was reduced in both obesity and obesity accompanied by diabetes. COL5A1 was over-represented in insulin signaling [Citation47]. Our research has also got similar results, which proves the reliability of our research. Interestingly, in this study TG and COL5A1 showed a positive correlation in the pregnancy loss group and a negative correlation in the nonpregnancy loss group. This may be the key point for pregnancy loss in PCOS. However, the study and mechanism on the relationship between TG and COL5A1 are rarely studied, which is deserves to be deeply and widely explored in the future.
Our study still has some limitations that require further study. First, it was retrospective and the sample size was relatively small and the public database PCOS proteomics data were few. These findings need to be verified in the future intervention studies. Second, although we obtained only two proteins has good predictive performance, machine learning algorithms may miss some useful predictive factors which cannot be ignored. Third, our statistical data include clinical data and proteomic data. For clinical data that lacks the calculation of sample size and sample power, more clinical data should be collected to obtain more convincing results. Numerous experiments are needed to verify how these proteins and pathways affect the receptive mechanism of the endometrium in PCOS patients.
Conclusion
We conducted proteomic analysis about samples, screened DEPs and analyzed pathways related to PCOS and PCOS pregnancy loss. We further screened feature proteins based on machine learning methods and constructed a meaningful model. The model based on the TIA1 and COL5A1 protein could effectively predict the occurrence of pregnancy loss in PCOS patients and provide a good theoretical foundation for subsequent research.
Ethics statement
The studies involving human participants were reviewed and approved by the Ethics Committee of Lanzhou University Second Hospital. All methods were performed according to the Declaration of Helsinki. The patients/participants provided their written informed consent to participate in this study.
Authors’ contributions
YYW and CL supervised the whole study, designed the concept, analyzed the data, YYW wrote the manuscript. CL edited the final manuscript. YYW, FW, and JGH collected and analyzed the data. All authors read and approved the final manuscript.
Abbreviations | ||
PCOS | = | polycystic ovary syndrome |
DEPs | = | differentially expressed proteins |
GO | = | Gene Ontology |
KEGG | = | Kyoto Encyclopedia of Genes and Genomes |
ROC | = | receiver operating characteristic curve |
DCA | = | decision curve analysis |
ET | = | endometrial thickness. |
Supplemental Material
Download Zip (5.9 MB)Disclosure statement
The authors declare that they have no competing interests.
Data availability statement
The datasets presented in this study can be found in online repositories. The names of the repository/repositories and accession numbers can be found below: The mass spectrometry proteomics data have been deposited to the ProteomeXchange consortium (http://proteomecentral.proteomexchange.org) via the iProX partner repository with the dataset identifier PXD032383.
Additional information
Funding
References
- Bozdag G, Mumusoglu S, Zengin D, et al. The prevalence and phenotypic features of polycystic ovary syndrome: a systematic review and meta-analysis. Hum Reprod. 2016;31(12):1–8. doi: 10.1093/humrep/dew218.
- Palomba S, Piltonen TT, Giudice LC. Endometrial function in women with polycystic ovary syndrome: a comprehensive review. Hum Reprod Update. 2021;27(3):584–618. doi: 10.1093/humupd/dmaa051.
- Visser JA. The importance of metabolic dysfunction in polycystic ovary syndrome. Nat Rev Endocrinol. 2021;17(2):77–78. doi: 10.1038/s41574-020-00456-z.
- Palomba S. Aromatase inhibitors for ovulation induction. J Clin Endocrinol Metab. 2015;100(5):1742–1747. doi: 10.1210/jc.2014-4235.
- Palomba S, de Wilde MA, Falbo A, et al. Pregnancy complications in women with polycystic ovary syndrome. Hum Reprod Update. 2015;21(5):575–592. doi: 10.1093/humupd/dmv029.
- Dimitriadis E, Menkhorst E, Saito S, et al. Recurrent pregnancy loss. Nat Rev Dis Primers. 2020;6(1):98. doi: 10.1038/s41572-020-00228-z.
- Thessaloniki ESHRE/ASRM-Sponsored PCOS Consensus Workshop Group. Consensus on infertility treatment related to polycystic ovary syndrome. Fertil Steril. 2008;89(3):505–522.
- Teede HJ, Misso ML, Costello MF, et al. Recommendations from the international evidence-based guideline for the assessment and management of polycystic ovary syndrome. Hum Reprod. 2018;33(9):1602–1618. doi: 10.1093/humrep/dey256.
- Diedrich K, Fauser BC, Devroey P, et al. The role of the endometrium and embryo in human implantation. Hum Reprod Update. 2007;13(4):365–377. doi: 10.1093/humupd/dmm011.
- Evans J, Salamonsen LA, Winship A, et al. Fertile ground: human endometrial programming and lessons in health and disease. Nat Rev Endocrinol. 2016;12(11):654–667. doi: 10.1038/nrendo.2016.116.
- Piltonen TT, Chen J, Erikson DW, et al. Mesenchymal stem/progenitors and other endometrial cell types from women with polycystic ovary syndrome (PCOS) display inflammatory and oncogenic potential. J Clin Endocrinol Metab. 2013;98(9):3765–3775. doi: 10.1210/jc.2013-1923.
- Piltonen TT. Polycystic ovary syndrome: endometrial markers. Best Pract Res Clin Obstet Gynaecol. 2016;37:66–79. doi: 10.1016/j.bpobgyn.2016.03.008.
- Zhang J, Ding N, Xin W, et al. Quantitative proteomics reveals that a prognostic signature of the endometrium of the polycystic ovary syndrome women based on ferroptosis proteins. Front Endocrinol (Lausanne). 2022;13:871945. doi: 10.3389/fendo.2022.871945.
- Van Calster B, Wynants L. Machine learning in medicine. N Engl J Med. 2019;380(26):2588.
- Zhang X, Liang B, Zhang J, et al. Raman spectroscopy of follicular fluid and plasma with machine-learning algorithms for polycystic ovary syndrome screening. Mol Cell Endocrinol. 2021;523:111139. doi: 10.1016/j.mce.2020.111139.
- Silva IS, Ferreira CN, Costa LBX, et al. Polycystic ovary syndrome: clinical and laboratory variables related to new phenotypes using machine-learning models. J Endocrinol Invest. 2022;45(3):497–505. doi: 10.1007/s40618-021-01672-8.
- Rotterdam ESHRE/ASRM-Sponsored PCOS consensus workshop group. Revised 2003 consensus on diagnostic criteria and long-term health risks related to polycystic ovary syndrome (PCOS). Hum Reprod. 2004;19(1):41–47.
- Chen X, Yang D, Li L, et al. Abnormal glucose tolerance in Chinese women with polycystic ovary syndrome. Hum Reprod. 2006;21(8):2027–2032. doi: 10.1093/humrep/del142.
- Parker SJ, Rost H, Rosenberger G, et al. Identification of a set of conserved eukaryotic internal retention time standards for data-independent acquisition mass spectrometry. Mol Cell Proteomics. 2015;14(10):2800–2813. doi: 10.1074/mcp.O114.042267.
- Götz S, García-Gómez JM, Terol J, et al. High-throughput functional annotation and data mining with the Blast2GO suite. Nucleic Acids Res. 2008;36(10):3420–3435. doi: 10.1093/nar/gkn176.
- Zhou Y, Zhou B, Pache L, et al. Metascape provides a biologist-oriented resource for the analysis of systems-level datasets. Nat Commun. 2019;10(1):1523. doi: 10.1038/s41467-019-09234-6.
- Blankers M, van der Post LFM, Dekker JJM. Predicting hospitalization following psychiatric crisis care using machine learning. BMC Med Inform Decis Mak. 2020;20(1):332. doi: 10.1186/s12911-020-01361-1.
- Zhao Z, Yang W, Zhai Y, et al. Identify DNA-binding proteins through the extreme gradient boosting algorithm. Front Genet. 2021;12:821996. doi: 10.3389/fgene.2021.821996.
- Tian M, Wang T, Wang P. Development and clinical validation of a seven-gene prognostic signature based on multiple machine learning algorithms in kidney cancer. Cell Transplant. 2021;30:963689720969176. doi: 10.1177/0963689720969176.
- Jaki T, Su T-L, Kim M, et al. An evaluation of the bootstrap for model validation in mixture models. Commun Stat Simul Comput. 2018;47(4):1028–1038. doi: 10.1080/03610918.2017.1303726.
- Fernandez-Felix BM, García-Esquinas E, Muriel A, et al. Bootstrap internal validation command for predictive logistic regression models. Stata J. 2021;21(2):498–509. doi: 10.1177/1536867X211025836.
- Bababekov YJ, Stapleton SM, Mueller JL, et al. A proposal to mitigate the consequences of type 2 error in surgical science. Ann Surg. 2018;267(4):621–622. doi: 10.1097/SLA.0000000000002547.
- Bababekov YJ, Hung Y-C, Hsu Y-T, et al. Is the power threshold of 0.8 applicable to surgical science?—empowering the underpowered study. J Surg Res. 2019;241:235–239. doi: 10.1016/j.jss.2019.03.062.
- Dumesic DA, Oberfield SE, Stener-Victorin E, et al. Scientific statement on the diagnostic criteria, epidemiology, pathophysiology, and molecular genetics of polycystic ovary syndrome. Endocr Rev. 2015;36(5):487–525. doi: 10.1210/er.2015-1018.
- Wiwatpanit T, Murphy AR, Lu Z, et al. Scaffold-Free endometrial organoids respond to excess androgens associated with polycystic ovarian syndrome. J Clin Endocrinol Metab. 2020;105(3):769–780. doi: 10.1210/clinem/dgz100.
- Guerson-Gil A, Palaiodimos L, Assa A, et al. Sex-specific impact of severe obesity in the outcomes of hospitalized patients with COVID-19: a large retrospective study from the Bronx, New York. Eur J Clin Microbiol Infect Dis. 2021;40(9):1963–1974. doi: 10.1007/s10096-021-04260-z.
- Subramanian A, Anand A, Adderley NJ, et al. Increased COVID-19 infections in women with polycystic ovary syndrome: a population-based study. Eur J Endocrinol. 2021;184(5):637–645. doi: 10.1530/EJE-20-1163.
- Karalok HM, Aydin E, Saglam O, et al. mRNA-binding protein TIA-1 reduces cytokine expression in human endometrial stromal cells and is down-regulated in ectopic endometrium. J Clin Endocrinol Metab. 2014;99(12):E2610–9. doi: 10.1210/jc.2013-3488.
- Neto da Silva AC, Costa AL, Teixeira A, et al. Collagen and microvascularization in placentas from young and older mares. Front Vet Sci. 2021;8:772658. doi: 10.3389/fvets.2021.772658.
- Piecyk M, Wax S, Beck AR, et al. TIA-1 is a translational silencer that selectively regulates the expression of TNF-alpha. EMBO J. 2000;19(15):4154–4163. doi: 10.1093/emboj/19.15.4154.
- Dixon DA, Balch GC, Kedersha N, et al. Regulation of cyclooxygenase-2 expression by the translational silencer TIA-1. J Exp Med. 2003;198(3):475–481. doi: 10.1084/jem.20030616.
- Naz S, Khan RA, Giddaluru J, et al. Transcriptome meta-analysis identifies immune signature comprising of RNA binding proteins in ulcerative colitis patients. Cell Immunol. 2018;334:42–48. doi: 10.1016/j.cellimm.2018.09.003.
- Regan JK, Kannan PS, Kemp MW, et al. Damage-associated molecular pattern and fetal membrane vascular injury and collagen disorganization in lipopolysaccharide-induced intra-amniotic inflammation in fetal sheep. Reprod Sci. 2016;23(1):69–80. doi: 10.1177/1933719115594014.
- Chang EM, Han JE, Seok HH, et al. Insulin resistance does not affect early embryo development but lowers implantation rate in in vitro maturation-in vitro fertilization-embryo transfer cycle. Clin Endocrinol (Oxf). 2013;79(1):93–99. doi: 10.1111/cen.12099.
- Rosen MW, Tasset J, Kobernik EK, et al. Risk factors for endometrial cancer or hyperplasia in adolescents and women 25 years old or younger. J Pediatr Adolesc Gynecol. 2019;32(5):546–549. doi: 10.1016/j.jpag.2019.06.004.
- Wang J, Wu D, Guo H, et al. Hyperandrogenemia and insulin resistance: the chief culprit of polycystic ovary syndrome. Life Sci. 2019;236:116940. doi: 10.1016/j.lfs.2019.116940.
- Cena H,Chiovato L,Nappi RE. Obesity, polycystic ovary syndrome, and infertility: A new avenue for GLP-1 receptor agonists. J Clin Endocrinol Metab. 2020;105(8):e2695–e2709. doi: 10.1210/clinem/dgaa285
- Jin Y, Wu Y, Zeng Z, et al. From the cover: exposure to oral antibiotics induces gut microbiota dysbiosis associated with lipid metabolism dysfunction and low-grade inflammation in mice. Toxicol Sci. 2016;154(1):140–152. doi: 10.1093/toxsci/kfw150.
- Elhady M, Elazab A, Bahagat KA, et al. Fatty pancreas in relation to insulin resistance and metabolic syndrome in children with obesity. J Pediatr Endocrinol Metab. 2019;32(1):19–26. doi: 10.1515/jpem-2018-0315.
- Männistö T, Mendola P, Grewal J, et al. Thyroid diseases and adverse pregnancy outcomes in a contemporary US cohort. J Clin Endocrinol Metab. 2013;98(7):2725–2733. doi: 10.1210/jc.2012-4233.
- Pihlajamäki J, Boes T, Kim E-Y, et al. Thyroid hormone-related regulation of gene expression in human fatty liver. J Clin Endocrinol Metab. 2009;94(9):3521–3529. doi: 10.1210/jc.2009-0212.
- Arner P, Sahlqvist AS, Sinha I, et al. The epigenetic signature of systemic insulin resistance in obese women. Diabetologia. 2016;59(11):2393–2405. doi: 10.1007/s00125-016-4074-5.