Abstract
This paper explores the interplay between structural and dynamic complexity factors, uncovering the mechanisms that underpin it. Four in-depth inductive case studies, which comprised semi-structured interviews with senior operations and SC managers as well as the use of companies’ official documents and other secondary data, were carried out. The study offers a deep understanding and provides rich empirical descriptions of the interplay between structural and dynamic complexity factors. A general framework to represent different aspects of this interplay is also introduced, i.e. the House of Supply Chain Complexity diagram; as a practical tool for mapping the complexity factors and inferring the prevailing interplay mechanisms in specific cases. The results show four interplay mechanisms between structural and dynamic complexity factors: silo-thinking, localism, limited adaptability and increased uncertainty. Testable propositions are presented with relevant insights on the interplay between a wide range of structural and dynamic complexity factors. Research implications relate to: (i) a general framework usable to further investigate interplay mechanisms at factors level and in different SC contexts and (ii) theory building on the suggested interplay mechanisms. We contribute to enhance contingency research claiming for the importance of considering the cumulative effect of contingency factors on SC performance. The results also suggest that managers can accommodate the mechanisms of silo-thinking and increased uncertainty by information sharing and organizational means, but the interplay mechanisms of localism and limited adaptability appear to be more related to the physical structure of SC elements and thus mainly manageable by reducing complexity, i.e. reducing the factors involved in the interplay.
1. Introduction
The management of complexity in organizations and in the supply chain (SC) has been and continues to be a challenge (Ateş et al. Citation2021; Reeves et al. Citation2020). A critical aspect to consider in understanding how complexity affects organizations and how these in turn can manage these effects is that complexity factors are not independent from each other but rather interrelated (Perona and Miragliotta Citation2004; Gottfredson and Aspinall Citation2005; Mariotti Citation2007; Mocker, Weill, and Woerner Citation2014). For example, Perona and Miragliotta (Citation2004) presents the links between a number of structural complexity factors (e.g. variety of products, of customers and sales channels, of suppliers) as a key aspect underpinning the overall impact of complexity on SC performance. Furthermore, Bozarth et al. (Citation2009) and more recently Birkie and Trucco (Citation2020) have called for future research on the interplay between static and dynamic complexity, in the context of complexity and SC performance.
This paper provides some steps towards filling this gap by investigating the individual factors in the complexity types of structural and dynamic complexity. It aims to explore how these factors interact with one another within their own complexity type and between the two complexity types. Relying on extant theoretical background on Contingency Theory and conceptual reasoning, these interactions are critically analysed to form a set of generalized mechanisms underpinning the interplay between structural and dynamic complexity in supply chains.
The complexity types of structural and dynamic complexity have been studied in previous research for decades (Casti Citation1979; Park and Okudan Kremer Citation2015; Serdarasan Citation2013; Bozarth et al. Citation2009; Wu, Frizelle, and Efstathiou Citation2007; Sivadasan et al. Citation2002; Dittfeld, Scholten, and Van Donk Citation2018). Structural complexity (also known as detail complexity; Bozarth et al. Citation2009; Aitken, Bozarth and Garn Citation2016) stems from the number, variety and interconnections between system components; thus, in a supply chain context, it is driven by the diversity of elements involved (products, processes, customers, suppliers, etc.) and by the dependencies and relationships between them. Dynamic complexity stems from the ‘system’s dynamical motion’ (Casti Citation1979) and involves time and uncertainty (Serdarasan Citation2013; Bozarth et al. Citation2009). Hence, in an SC context, dynamic complexity is driven by the dynamics of SC operations (Sivadasan et al. Citation2002) and by the pace of change of SC elements or of the relationships between these (Collinson and Jay Citation2012; Maylor, Vidgen, and Carver Citation2008).
The mutual effect that each structural and dynamic complexity factor may have on the other, referred to as ‘interplay’ in this paper, has received very little attention from empirical SC studies. For example, in the business complexity literature, Mocker, Weill, and Woerner (Citation2014) argue that companies may exploit practices, such as defining digital process platforms, to address and lessen the interactions between customer and internal complexity factors. However, in the SC literature, there is very little empirical evidence of the interactions between these factors or, more importantly, between structural and dynamic complexity. Therefore, there is a lack of understanding regarding the nature of this interplay and what its contribution to the overarching relationship between complexity and SC performance may be. This is the gap that this paper aims to fill.
The paper contributes to theory building on supply chain complexity, by using inductive case studies research to explore the mechanisms that underpin the interplay between structural and dynamic complexity factors. Coherently, the following research question is set forth:
RQ: What are the mechanisms underpinning the interplay between structural and dynamic complexity in a supply chain?
It is worth mentioning that the concept of interplay mechanism investigated in this paper is somewhat similar to the ‘interactions’ presented by Dittfeld, Scholten, and Van Donk (Citation2018). In their paper, they define horizontal, vertical and diagonal interactions between complexity types, among different levels and across types and levels. However, in this paper, we search for interplay mechanisms between individual complexity factors both within and between complexity types. Therefore, we aim at investigating the phenomenon in greater detail in comparison to prior similar studies.
The interplay mechanisms underpinning the interactions between structural and dynamic complexity factors are described in the within-case analyses. It is found that the mechanisms are all driven by structural complexity and share, as a common outcome, the exacerbation of the negative impact of dynamic complexity on SC operations performance. These interplay mechanisms are labelled as: Silo-thinking, Localism, Limited adaptability and Increased uncertainty.
The rest of the paper is organized as follows. Section 2 presents the literature review for the study and delineates the research gaps in more detail. Section 3 presents the methodology of inductive case studies to address the research question. The empirical findings of four within-case and cross-case studies on the internal supply chain of manufacturing organizations are provided in Section 4. In Section 5, the discussion is presented, which leads to the introduction of testable propositions. Section 6 concludes the manuscript by underlining contributions to theory and practice, as well as limitations and opportunities for future research.
2. Literature review
In the SC literature, papers on complexity issues that examine the influence of the firm’s environment or context may be divided into two groups, depending on their approach to the subject. The first group relates to the Business Complexity literature and the second group relates to the Contingency factors literature. Each of these groups is explained in turn next.
The first group of studies is fully aligned with the Business Complexity literature (e.g. Ruiz-Hernández, Menezes, and Amrani Citation2019); hence, arguing that factors that are characteristic of the firm’s operating context, yet external to the firm, have a significant effect on the complexity borne by its SC (Ketokivi Citation2009). For instance, support for this argument can be found in the SC complexity models of Perona and Miragliotta (Citation2004) and Manuj and Sahin (Citation2011). More precisely, Perona and Miragliotta (Citation2004) propose that strategic objectives and context variables, together with the available resources, determine the SC’s ‘basic’ complexity. In a similar manner, Manuj and Sahin’s (Citation2011) grounded theory study identifies several types of complexity antecedents and, within these, underlines some contextual factors such as industry structure, regulation and environmental conditions. Therefore, these works suggest that SC complexity is, at least partially, driven by contextual factors.
The second group of works is that which describes complexity itself as a contingent factor and explores its influence on a broad range of SC management issues (Eckstein et al. Citation2015; Wong, Lai, and Bernroider Citation2015; Blome, Schoenherr, and Eckstein Citation2014; Azadegan et al. Citation2013; Grötsch, Blome, and Schleper Citation2013; Stock, Greis, and Kasarda Citation2000). In particular, Azadegan et al. (Citation2013) investigate the influence of environmental complexity (defined as the complexity of the firm’s competitive environment in terms of the number of competitors) on the relationship between lean purchasing and lean operations practices and find that the performance benefits of these practices increase in more complex environments. Similarly, Wong, Lai, and Bernroide (2015) examine the contingent effect of supply- and customer-base complexities on the effect that information integration has on business performance, and their results suggest that the performance improvements achieved through information integration benefit from lower supplier and higher customer complexities. Stock, Greis, and Kasarda (Citation2000) investigated the contingency effect of logistical complexity (i.e. network dispersion) on the relationship between enterprise logistics practices and organizational performance.
Both groups of literature presented earlier can be combined into the contingency theory approach. Contingency theory (Luthans and Stewart Citation1977; Lawrence and Lorsch Citation1967) indicates that there is not a ‘one size fits all’ approach for managing organizations; in other words, the outcomes of managerial decisions depend on the environmental and internal contingencies related to organizations (Shepard and Hougland Citation1978). Furthermore, Sousa and Voss (Citation2008) proposed that contingency theory can be used as a useful lens to understand how real-world practices, responses and performance are studied in the Operations and SC management field. In this vein, contingency variables are outside the organization or manager’s control, response variables are those that the organization uses to adapt to the contingency variables, and performance variables measure how well response aligns to contingency. As a result, the idea of best-fit or alignment between strategy and internal practices/processes should lead to higher performance.
As evidenced from both groups of literature, we propose that contingency theory can be used to increase the understanding on how the contextual environment, in which the SC organizations are embedded, can influence their different performance outcomes (e.g. Flynn, Huo, and Zhao Citation2010; Ateş and Memiş Citation2021).
In this way, this paper extends the contingency theory as originally called by Luthans and Stewart (Citation1977, 194) when they stated that
As the rate of change and the associated degree of complexity continues to accelerate, the influence of environmental variables will be increasingly significant to effective management. […] However, if the contingency approach is to realize its potential as an effective construct for maintaining and improving managerial effectiveness in a hyperdynamic environment, its development must proceed in a systematic, unified and directed manner.
Therefore, in this paper, we consider SC complexity as a contingent factor controlled through the design of three complexity dimensions: product portfolio, the internal SC and the external SC (see ).
Table 1. Adopted complexity categories and prior key classifications in the literature.
2.1. Types and definitions of SC complexity
Bozarth et al. (Citation2009) defined SC complexity as ‘the level of detail [also known as structural] complexity and dynamic complexity exhibited by the products, processes and relationships that make up a SC’ (p. 80). Detail (i.e. structural) complexity captures ‘the distinct number of components or parts that make up a system’, whereas dynamic complexity refers to ‘the unpredictability of system’s response to a given set up inputs’ (p. 79); thus, underlining the two elements of numerousness and uncertainty. A similar definition is provided by Serdarasan (Citation2013, 533), who states that structural complexity ‘describes the structure of the SC, the number and the variety of its components and strengths of interactions between these’, while dynamic complexity ‘represents the uncertainty in the SC and involves the aspects of time and randomness’. Structural complexity ‘is related to the connectivity and structure of the subsystems involved in the SC (e.g. companies, business functions and process)’; whereas dynamic complexity ‘results from the operational behavio[u]r of the system and its environment’. More recently, Bai and Sarkis (Citation2018) added the concept of trade-offs in addition to the SCM complexity characteristics of numerousness, unpredictability (uncertainty) and variety (Dittfeld, Scholten, and Van Donk Citation2018).
Perona and Miragliotta (Citation2004) as well as Aguila and ElMaraghy (Citation2018) underline the density of interactions between structural complexity factors in the SC. Serdarasan (Citation2013) notes that structural and dynamic complexity ‘are interrelated and should not be considered in isolation’ (p. 534). Along these lines, Bode and Wagner (Citation2015) argue that structural and dynamic complexity are ‘closely interrelated’. Nonetheless, to the best of the authors’ knowledge, no prior SC study has empirically investigated the influence that each of these complexity types has on another, i.e. their interplay. While some research streams have significantly contributed to the theoretical discussion of the dynamic aspects of SCs as complex systems, such as complex adaptive systems or CAS (Choi, Dooley, and Rungtusanatham Citation2001; Ashmos, Duchon, and McDaniel Citation2000), empirical SC complexity research has predominantly focussed on structural elements of complexity (Größler, Grübner, and Milling Citation2006; Inman and Blumenfeld Citation2014; Manuj and Sahin Citation2011; Birkie, Trucco, and Fernandez Campos Citation2017; Aguila and ElMaraghy Citation2018; Ruiz-Hernández, Menezes, and Amrani Citation2019).
2.2. SC complexity factors
Complexity definitions can be applied to a range of different SC elements, allowing to examine numerous aspects of complexity. This study draws from the literature (Ateş et al. Citation2021; Christopher Citation2016; Mocker, Weill, and Woerner Citation2014; Ashkenas Citation2007; Caniato and Größler Citation2015) to group complexity factors into three categories: product portfolio and design; internal SC (owned by the organization) design and external SC (customers, suppliers and outsourced activities of the SC – e.g. logistics) design (see ).
Prior literature has discussed both the relevance of structural and dynamic complexity (Ferdows Citation2018; Aitken, Bozarth and Garn Citation2016; Smart, Calinescu, and Huaccho Huatuco Citation2013; Bozarth et al. Citation2009; Choi, Dooley, and Rungtusanatham Citation2001) and the effect that each complexity type has on each other (Bode and Wagner Citation2015; Serdarasan Citation2013; Casti Citation1979), i.e. their interplay, resulting into degraded performance. Understanding the interplay mechanisms between complexity factors can help organizations tackling their efforts in managing complexity (reduction vs accommodation) and using it more strategically.
In summary, drawing from the review of the prior two groups of SC studies, i.e. the business complexity and the contingency literature, and discussing them with the lenses of contingency theory, two main streams of contributions, with associated limitations, emerge: the first, in line with the business complexity literature, suggests that complexity is influenced and can be driven by contextual factors (Manuj and Sahin Citation2011; Perona and Miragliotta Citation2004). However, limited knowledge is offered on the specific contextual factors and the aspects of complexity that might be influenced by them. The second suggests that the adoption and use of managerial practices and responses to complexity might be contingent on contextual factors (i.e. that different firms might choose different responses to a certain type of complexity driven by the characteristics of its external environment) (Vachon and Klassen Citation2002). However, limited knowledge is offered on matching complexity accommodation and reduction practices with the prevailing complexity factors and their interplay (e.g. Fernández Campos, Trucco, and Huaccho Huatuco Citation2019).
3. Methodology
3.1. Research approach
A case study ‘investigates a contemporary phenomenon within its real-life context, especially when the boundaries between phenomenon and context are not clearly evident’ (Yin Citation2003, 13). In this paper, an inductive case study methodology (Barratt, Choi, and Li Citation2011) is leveraged to address the research question to investigate the interplay mechanisms between structural and dynamic complexity. Here, the emphasis is not only on underlining what the given mechanisms that take part in the interplay are, but rather in describing and explaining how these take place. Therefore, this paper uses a qualitative methodology that can address both ‘what’ and ‘how’ questions and that is adequate to examine mechanisms as the rules or processes that underlie and shape a relationship (Pratt Citation2009; Eisenhardt Citation1989). Case studies are adequate for exploratory research, in which the phenomena are not well understood yet, and lends itself to addressing gaps in extant theory (Benbasat, Goldstein, and Mead Citation1987).
The unit of analysis of the case studies is the company’s internal SC, defined as the sub-set within the organization that directly takes part in SC activities – i.e. planning, sourcing, making and delivering (Hoole Citation2005; Gunasekaran, Patel, and McGaughey Citation2004) - which are owned by the organization. By encompassing all the main SC activities, this unit of analysis provides with a more comprehensive view on SC complexity than earlier works that have focussed solely on, for instance, manufacturing or logistics (e.g. Bozarth et al. Citation2009; Busogi et al. Citation2017; Stock, Greis, and Kasarda Citation2000). External SC refers to those SC activities that are not owned by the organization, e.g. suppliers, customers and outsourced parts of the SC, e.g. warehouse and transport.
3.2. Sampling strategy
Different suggestions can be found in the literature on the adequate sample size for inductive case studies. On one hand, a deep investigation of each case is necessary to gain a nuanced understanding of the various relationships between the constructs. On the other hand, it is central to rely on multiple cases to achieve an adequate coverage of the factors involved and to ensure that the unveiled interplay mechanisms are not industry dependent. Eisenhardt (Citation1989) recommended to strive for saturation when carrying out case-based research, with the suggestion that between four and ten case studies could suffice. In this paper, we use four in-depth case studies because they have shown saturation in the data analysis on the types of interplay between SC complexity factors, i.e. in populating the House of SC Complexity ().
Figure 1. Case A’s interplay between complexity factors and management practices in the House of SC Complexity. X→Y: X aggravates Y (oriented according to prevailing direction of influence). Coded practices reported in .
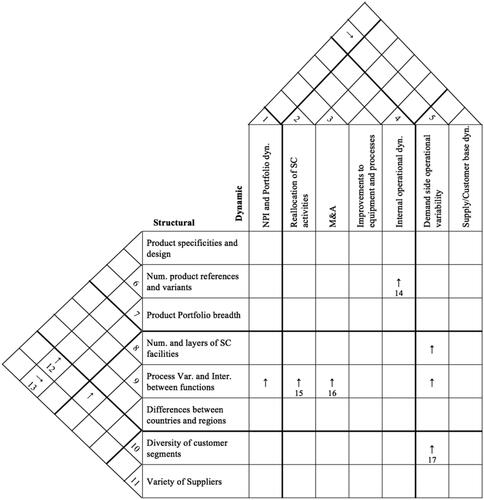
A set of potential companies were targeted, the selection criteria were as follows: manufacturing sector, large and being complexity conscious. A first examination of each potential case was made relying on available secondary documentation. This preliminary assessment served to ensure an adequate distribution of the sample along multiple relevant parameters (e.g. position within the SC, industry, areas of complexity factors) and was subsequently supported by preliminary interviews with the organization before commencing the data collection process for each case. After this, among some possible alternatives, the level of accessibility of the candidate companies was used as an element of preference. The key characteristics of the case companies in the final sample are presented in .
Table 2. Case companies’ characteristics.
3.3. Data collection
Structured data collection and analysis processes were adopted to enhance the reliability of the study (Yin Citation2003). Data were collected from multiple sources, including semi-structured interviews, company documents and archival sources, informant’s notes during and prior to the interviews, and secondary data collected for the preliminary assessment of the case. Data were triangulated to strengthen its reliability and the study’s internal validity (Voss, Tsikriktsis, and Frohlich Citation2002; Benbasat, Goldstein, and Mead Citation1987). Nonetheless, semi-structured interviews to key informants constituted the primary source of data in the study. A total of nineteen interviews were carried out. The duration of these was not less than 60 min and approximately 75 min on average. Follow-up interviews were conducted where appropriate, e.g. to gather further information on an emergent issue related to the role of an already interviewed respondent. Multiple respondents in different areas and levels of responsibility within the internal SC were selected (e.g. purchasing director, SC chief of staff, plant manager). See Online Appendix 1 for the interview protocol used.
3.4. Data analysis
The content analysis process was designed based on the recommendations and methods in Saldaña (Citation2015). Multiple content analysis methods were used to capture the complex characteristics of the phenomena in the data and enhance the depth of the findings (Coffey and Atkinson Citation1996). Using the qualitative data obtained during the semi-structured interviews, a first step employed holistic coding (Dey Citation1993) to develop a sense of the overall contents of the data. This step informed the data collection process on emerging relevant issues or areas lacking information in the case. Detailed descriptive coding was then used to develop and refine codes that summarized and categorized the data’s content (Miles and Huberman Citation1994). Finally, structural coding (MacQueen and Guest Citation2008) was used to link the developing themes, codes and insights in the data to the research questions (see ).
Table 3. Examples of data coding.
The analysis was carried out following a within-case and cross-case sequence, and tables and visual displays were employed to summarize and illustrate the empirical evidence (Miles and Huberman Citation1994; Voss, Tsikriktsis, and Frohlich Citation2002). A customized diagram was employed to graphically represent different aspects of the interplay between structural and dynamic complexity factors. Due to its graphic resemblance to the House of Quality diagram, employed to translate customers’ desires into product functional requirements (Hauser and Clausing Citation1988), this diagram is referred to as House of SC Complexity (HoSCC) diagram in this paper, which is described next.
In , structural and dynamic complexity factors are placed to the left and top of the central body, respectively. These are the factors that have emerged from the analysis in the specific case study and which will be presented in the Findings section. Likewise, the relationships within structural and dynamic complexity groups of factors are mapped in the left and top triangles, respectively; and those between these two complexity types are represented inside the diagram’s main central body. Arrows point towards the factor that is increased or aggravated by another factor. In other words, if an arrow points from factor X to factor Y, this represents that factor X either increases or aggravates Y, thus in either case increasing the adverse effects of factor Y on SC cost performance. It is important to note that these relations are not argued to be causal, as the inductive research approach allows for the building of theory, but not to claim causality. In this vein, the arrows in the diagram simply represent the influences that emerged from analysing the collected empirical data, and their direction indicates the prevailing direction of influence reported in it. In addition, the figure shows how management practices are used to lessen the negative outcomes of complexity. To this end, the cells in that correspond to an individual factor or to an interaction whose effect on performance is found to be lessened by management practices have been numbered (see ).
Table 4. Key of complexity management practices reported in : complexity accommodation; R: complexity reduction).
The specific management practices associated to the cells are subsequently reported in , which indicates whether they are predominantly employed to accommodate (A) or reduce (R) complexity. For a more detailed discussion of the logic underlining the selection of SC complexity management practices against different combinations of prevailing complexity factors, the reader is referred to Fernández Campos, Trucco, and Huaccho Huatuco (Citation2019).
4. Findings
The results are presented first in terms of the individual within-case findings followed by the cross-case findings.
4.1. Within-case findings
Key findings from the analysis of interplay mechanisms of complexity factors within each of the four individual case studies (Cases A, B, C and D) are presented next. Each case description is provided in Online Appendix 2.
4.1.1. Case A
Two main areas of interplay between structural and dynamic complexity factors emerge from the analysis: the first regards the structural complexity of the internal SC design and how it hinders the management of a series of dynamic complexity factors. The second revolves around the variety of product references in the company’s product portfolio and how this large variety can aggravate the negative influence of internal operational dynamics on SC performance
The first, structural internal SC complexity is linked to three interrelated areas that together compromise the internal SC’s ability to manage dynamic complexity. These areas are primarily: silo-thinking (i.e. lack of a holistic understanding of the internal SC), insufficient coordination between functions and poor information sharing.
4.1.1.1. Silo-thinking
The lack of holistic understanding of the internal SC leads to managers making decisions without accounting for the implications these may have for other SC areas (i.e. silo-thinking), making dynamic complexity more difficult to manage and increasing its toll on SC performance. This effect is especially relevant for the SC in the interactions between planning, manufacturing and sales. For instance, a plant manager notes how this contributes to aggravate the operational dynamics from front-end functions:
There is a continuous change. [Sales] tend to say that they are sharing the information with us, but from our side it means that each time we must go back to our monthly plans. So, we are forced to readapting them continuously, which increases the [dynamic] complexity. But this happens precisely because the knowledge of the next piece [of the SC] is missing. [Case A, Plant Manager]
4.1.1.2. Insufficient coordination
Another dynamic complexity factor, where its management is compromised by the structural complexity of the SC design in a similar manner, is Mergers and Acquisitions (M&A). The large number of functions that are involved in the integration activities and the insufficient coordination between them increase the cost and length that these activities impose on the SC. For instance, an SC master data manager recalled a past example in which the firm was not able to reallocate the acquired company’s stock within Case A’s network because finance (one of the four different teams involved in the task) failed to input the inter-company costs of products in the ERP. Overall, the examples illustrate that the successful management of this strategic dynamic complexity factor requires all involved functions to be fully aware of their dependencies with other functions. Moreover, the structural complexity of the SC’s organizational design entails that information is dispersed across functions. Managers lack a holistic view of the internal SC, and of understanding of the dependencies within SC areas, can prevent relevant information from being timely shared. Thus, this leads to a lack of coordination that also hinders the management of operational dynamic complexity. For instance, the plant manager explains that sometimes sales and procurement may have information about upcoming anomalies in the supply and demand sides that, although not critical by themselves, may together require immediate action from the planning team.
4.1.1.3. Poor information sharing
The complexity of the SC design can make managers fail to recognize the full range of potential outcomes of those events for the entire SC, hence resulting in managers’ poor information sharing. As a manager summarizes:
The problem of information that is not circulated is not one of a lack of intention but a lack of culture that one’s data can influence others. […] It’s the business culture of understanding processes. [Case A, Supply Chain master data manager]
4.1.1.4. IT systems as double-edge sword
In addition, SC managers argue that IT systems that may effectively communicate and coordinate functions during regular SC operations often fail to help when facing these more unexpected events. Several reasons appear to underpin this. The bespoke interfaces each area uses are tailored (and narrowed down) to avoid wasting managers’ focus and time. Also, the access each employee has to different areas in the software is limited for security reasons. Lastly, because of the vast volume of data these systems can hold, the parameters managers view in the system are not updated in real time to avoid overwhelming managers: ‘a human mind cannot, despite the systems’ help, go over all the parameters at once’ [Case A, Supply Chain master data manager]. For these reasons, such detailed information is often hard to gather or even unavailable for managers in other areas. Hence, these IT systems play an important role in accommodating the uncertainty that stems from complexity but can also accentuate the separation between functions and their tendency to think in silos:
[One must] understand that the ERP is not a closed box: each area can’t go on thinking and working in silos, you must know what happens before and after, because pieces of processes that may work like that [(in silos)], may not do so in a general context. [Case A, Supply Chain master data manager]
4.1.1.5. Increased uncertainty
The second, and somewhat less critical, area of interplay between structural and dynamic complexity factors regards the large variety of product references that conforms the portfolio requires SC managers to split their time (and focus) among the various SKUs, increasing managers’ probability of making mistakes and decreasing that of detecting them. Therefore, reducing the SKU count can not only improve SC performance because it concentrates volumes and enhances efficiency (i.e. reduced structural complexity) but it can also help reduce the costs associated to the operational dynamism yielded by managers’ errors or inaccuracies (i.e. operational dynamic complexity). In this manner, the structural complexity of the product portfolio is associated to an increase of uncertainty for SC operations. As summarized by a manager:
Of course, reducing [the number of] products is positive. Also, at a planning level, the planner works on a single reference, instead of two, so you have 50% the chances that an error will be drawn into the data. The more you grow [the number of products], the easier it is that a mistake will be overlooked, even though you have the system to help you match and calculate data. The larger the number of references you have to watch, the more the probability of making a mistake grows. [Case A, Supply Chain master data manager]
For the House of SC Complexity for this case, please refer to .
4.1.1.6. Case A’s key findings
The structural complexity of the internal SC results in silo-thinking, insufficient coordination among functions and poor information sharing, which compromise decision-making and the management of dynamic complexity factors, such as operational dynamics of M&A. In this respect, the variety of teams and functions involved in SC activities binds the effectiveness of project management practices to manage new product introductions (NPIs) and leads to negative impact on SC performance. Similarly, the SC struggles to integrate acquisitions, underpinned by the lack of formalized process and the interactions between functions. IT systems that are effective in coordinating teams during regular operations can also accentuate the separation between functions and thus contribute to the prior effects (i.e. silo-thinking). The diversity of product references (i.e. variants) requires managers to split their focus and leads to increased errors and inaccuracies, increasing the uncertainty of SC operations.
4.1.2. Case B
The interplay between structural and dynamic complexity factors spans three different areas: increased uncertainty, silo-thinking and divergence of response.
4.1.2.1. Increased uncertainty
The first regards the structural complexity of the internal SC’s network of facilities which increases the uncertainty of customer-related operational dynamics. More precisely, the variety of facilities and dependencies within them increase demand volatility in each facility, the number of inventory locations to plan and the number of dependencies to account for when planning; thus, fostering the exacerbation of the bull-whip effect. In line with this, rationalizing the SC network was considered as a necessary preceding step to the implementation of premium and standard delivery models, to partially reduce the accentuated dynamic complexity of SC operations. In addition, the Project Management Office has been presented as a key resource in the management of the dynamic complexity that stems from strategic changes to the internal SC design. Indeed, Project Management Office managers design, structure and implement these changes to reduce their impact on SC performance. However, a number of internal SC structural complexity factors hinder the performance of the Project Management Office, increasing the negative impact of these changes on SC performance and compromising the ‘evolution’ of the SC. As exemplified by a Project Management Office manager:
In the front-end system, we have two systems: one for the direct business and one for the channel partners. Then we have our own logistic system and we also have interfaces with the logistics partner system. In fact, the logistics partner system has two systems. So, you can imagine because the system is not fully integrated, every time we want to make an evolution in the SC we need to be able to work with different systems and to try take a helicopter view, in order to see if we want to change something in one part of the process what does it mean for the other elements. [Case B, Supply Chain program and project manager]
4.1.2.2. Silo-thinking
The second focuses on silo-thinking, which is fostered by some structural complexity factors, and compromises the management of dynamic complexity (e.g. product design trends, and life cycle events, SC flow reconfigurations). In this vein, a series of internal SC structural complexity factors (e.g. variety of partners, differences between territories, fragmented processes) are detrimental to the performance of the Project Management Office and thus hinder the management of dynamic complexity factors.
Thus, structural complexity accentuates the tendency of different functions to think in silos, contributing to the difficulty of developing a holistic view of the SC that is experienced by Project Management Office managers. Furthermore, silo-thinking is argued to hinder the management of several dynamic complexity factors. As summarized by a manager:
Most of the things that we do to improve processes or to transform, you can’t do those kinds of things in a silo by yourself, you need more and more involvement from business units outside the SC and really more integrated SC collaboration. [Case B, Planning and planning platform manager]
In fact, several of the complexity management practices which aim to establish links between functions and overcome silo-thinking are reported to enhance the management of dynamic complexity factors. For instance, the use of global forums, cross-functional KPIs and joint product reparability strategies aid in the management of product life cycle events, operational customer dynamics and cost-incurring product design trends. Moreover, silo-thinking appears to be fostered by the workforce job rotation and turnover rates in the job market, collective vs. individual incentives for managers and demanding cost-reduction performance goals. The implementation phase of projects in the SC is hindered by complexity in a somewhat different manner.
4.1.2.3. Divergence of responses – localism
Lastly, structural complexity gives place to more specific dynamics that force Case B’s managerial responses to dynamic complexity factors to diverge across the internal SC.
In particular, the variety of partners and of interfacing systems, the large number of functions involved in SC activities, fragmented processes and differences between countries impact both the design of solutions and their implementation, making Project Management Office tasks less effective and longer. A critical point regarding the design phase, and one that is also illustrated in the previous excerpt, is that these structural complexity factors prevent Project Management Office managers from developing the holistic view or comprehensive understanding of the internal SC (i.e. the complex system). However, this is necessary to let managers foreseeing the implications of specific solutions to individual teams, i.e. divergence of responses:
It is really the point I am struggling with, with this project of transformation. I have a lot a lot of meetings with many people in different functions, and from the starting point I need to recreate this end-to-end knowledge before being able to say ‘ok, now I have a good understanding of the current processes and also of the interfaces, the interconnections between the different elements’, now I can switch to a design phase where I will try to propose some things and I will be able to propose a solution. [Case B, Supply Chain program and project manager]
In this respect, structural complexity factors (e.g. differences between countries) give place to dynamics that are specific to reduced ‘local contexts’ within the SC, forcing the Project Management Office’s unique design solutions to diverge in their implementation. Hence, this phenomenon does not only increase the costs of managing these changes to the network, but also further accentuates extant differences between territories (i.e. further increasing structural complexity). For instance, during the implementation of a new design of SC flows that intended to serve several countries from the central Distribution Centre (DC), some of the extant specificities of countries made the implementation of the homogeneous solution unfeasible and forced managers implement specific delivery solutions in these areas, therefore adding new particularities to these challenging territories.
For the House of SC complexity for this case, please refer to Figure 4 in Online Appendix 2.
4.1.2.4. Case B’s key findings
The number of network facilities and layers increases uncertainty and impact of front-end operational dynamics. Complexity fosters silo-thinking, which is detrimental to the management of product lifecycle (LC) events and operational dynamism (e.g. hinders project management practices). The differences between countries results in ‘local context’ specific dynamics that force the responses to dynamic complexity factors to diverge.
4.1.3. Case C
Structural complexity factors aggravate dynamic complexity factors’ impact on SC performance along the following areas. First, a local plant specificities force responses to dynamic complexity to diverge and reduce the effectiveness of the employed managerial practices. Second, the large number of plant peculiarities and the size of the product portfolio increase the uncertainty of SC operations. Third, product portfolio structural complexity limits the adaptability of the internal SC to dynamic phenomenon. Finally, structural complexity increases the need for coordination efforts in the SC to manage the introduction of new products and continuous improvement.
The differences between SC facilities in different countries compromise Case C’s ability to manage dynamic complexity factors in the product portfolio (e.g. stylish wheel line introduction) and internal SC design (e.g. continuous improvement). In this vein, the particularities of plants hinder the knowledge-sharing benefits of benchmarking practices to manage dynamic complexity as, first, they demand the use of plant-specific KPIs to monitor and report performance, which, together with the disparate organizational designs and distinctive plant characteristics, can yield ‘misleading analyses’ [Case C, Purchasing manager].
4.1.3.1. Diverge of responses – localism
In addition, these plant specificities reduce the number of facilities that may benefit from the devised responses to dynamic complexity. Hence, developed solutions often have to be adapted to the peculiarities of plants, increasing dynamic complexity’s overall impact on SC operations cost, speed and quality. For instance, in using benchmarking to share with other plants the improvements made to packaging handling processes in a facility, a manager explains that although ‘other plants have also started to introduce the same measures, these will not be spread in the same way in each plant’ [Case C, Logistics manager]. Therefore, plant specificities make the originally implemented measures diverge, further accentuating the differences between them and increase the resources necessary to implement improvements throughout the network.
4.1.3.2. Increased uncertainty
In addition, the large number of unique features of SC facilities contributes to the uncertainty of operations, as central managers that are involved in the management of projects and changes to the production lines struggle to foresee the implications of implementing a specific solution in a given facility. For instance, the firm had to carry out bespoke extensive testing in each of its facilities to assess the feasibility of introducing the stylish wheels line of the portfolio. In a similar manner, but in relation to the firm’s portfolio, the number of products and product variants dilutes stocks and leads to increased raw material variability, thus aggravating the impact of dynamic complexity on SC performance.
4.1.3.3. Limited adaptability
An alternative effect that arises from the diversity of wheel models is that the need to ensure the compatibility of manufacturing processes and equipment with all pre-existent models limits the SC’s ability to adapt to dynamic factors. As anticipated, a similar effect involving customer requirements and the ability to reconfigure the SC is observed, as the rigid and diverse security-driven requirements render the reallocation of SC activities unfeasible. As illustrated by a manager,
Each time we are obliged to take into account the full range of products we produce before we implement something new, because we need to study any risks to degrade performance or that one [model] becomes unfeasible to make. Each time we study the full range of what we have or will have in the future, and each modification normally covers the full range of products. [Case C, Plant general manager deputy]
4.1.3.4. Increased coordination efforts
Lastly, the structural complexity of the organization and SC network is also a barrier in the management of NPIs and continuous improvement. In this respect, the management of these dynamic complexity factors demands effective interactions and communications between SC facilities (e.g. HQ and manufacturing facilities) and between teams in different functions (e.g. purchasing and quality) as they involve a multitude of areas in the SC and the organization. Also the structural complexity of the internal SC design and of the organization clearly increases this need. Indeed, some of the aforementioned practices are posed to help managers to this regard (e.g. the communication-oriented customer offer development software tool). However, most of the reviewed systems that facilitate these interactions are only implemented at local plant level and hence fall short in connecting different facilities (e.g. ERP systems).
For the House of SC complexity for this case, please refer to Figure 5 in Online Appendix 2.
4.1.3.5. Case C’s key findings
The differences between SC facilities in different countries make the originally adopted responses to manage dynamic complexity diverge, increasing the number of resources to implement improvements in the SC. The large number of unique features of SC facilities increases uncertainty of operations, limiting the SC’s ability to foresee the implications of implementing a specific solution in each facility and aggravating the impact of dynamic complexity on SC performance. The need to ensure the compatibility of manufacturing processes and equipment with the variety of products limits SC’s ability to adapt to dynamic factors. The structural complexity of the organization and SC network increases the need for coordination efforts between teams and facilities and is a barrier to the management of NPIs and continuous improvement.
4.1.4. Case D
The interaction between structural and dynamic complexity factors and management practices spans four areas. First, structural product design and portfolio complexity is found to aggravate operational dynamic complexity due to the increased uncertainty the former bring to SC operations. Second, efficiency-pursuing measures (e.g. reusable packaging) can accentuate dependencies between elements in the SC, complicating the management of dynamic complexity. Third, the variety in the product portfolio and customer base lead to ‘spread dynamics’ that demand of a diversified range of solutions and reduce the SC’s ability to manage product and customer dynamics. Lastly, IT systems are presented as a ‘double-edge sword’ that can aid the internal SC accommodate complexity but can also hinder comprehensive decision-making.
4.1.4.1. Increased uncertainty
A first area of interplay regards the effect that product design and portfolio structural complexity can have in increasing uncertainty and aggravating the dynamics of operations in the internal SC. Specifically, product portfolio size and breadth aggravates the impact of the dynamic complexity of operations on SC performance, as
When you got a large [portfolio] breadth and size you have high variability of process and uncertainty; and special types of test equipment. And more variability will bring more room for error and for cost. [Case D, Head of Supply Chain and manufacturing]
In a similar vein and related to the tight regulation in Case D’s industry, the large variety of different exporting licences required by legal authorities increases the frequency of errors from commercial functions, which can disrupt the normal flow of operations.
4.1.4.2. Accentuation of dependencies
Additionally, a series of measures taken in the pursue of efficiency (i.e. cost-related) performance objectives create additional dependencies and constraints for managers and aggravate the negative influence of product portfolio and external SC dynamic complexity on the SC. An example of this is the use of reusable packaging, which allows Case D to maximize the utilization of packaging resources. However, it also adds dependencies on customers, who must ship back the empty containers so that Case D does not eventually run out of these. Because customers are often not ready to use the equipment when they first receive it, they will tend to keep the equipment inside the reusable packaging for long periods of time, therefore hindering the SC’s ability to adapt to the dynamics of the internal SC operations. Likewise, efficiency-driving measures such as the employment of a lean workforce, sharing test equipment across projects and products and minimizing the use of prototyping during the development of new products compromise the management of dynamic complexity factors such as NPIs and unexpected customer demand. This interaction is illustrated by the head of manufacturing and SC as,
The complexity is in dealing with the dynamic movement of manufacturing, and the different priorities and calls on resources to ensure that it continues to flow without one [product line] impacting the other. [Case D, Head of Supply Chain and manufacturing]
4.1.4.3. Divergence of responses – localism
A third aspect of the interaction between structural and dynamic complexity factors is that the variety in the product portfolio and the customer base make the internal SC cope with a wider range of solutions. This is described by the head of manufacturing and SC as ‘the dynamics [being] spread’ and implies that managers are forced to employ a diversified range of solutions to manage the dynamics of products and customers due the relevance of their individual specificities. Therefore, on one hand, these ‘spread dynamics’ require of more resources and data, and they further complicate the allocation of resources. On the other, because each of these solutions can be applied to a smaller area within the internal SC, these may become un-profitable, hence limiting the extent to which the SC can manage product and customer dynamics. As exemplified by the head of SC and manufacturing in discussing continuous improvement practices,
The complexity [of continuous improvement] is further magnified by the fact that the unitary production cost would be for a range of products. The radar hall may do ten different radars. It is done for every different product. And we may see similar issues, in which case the solution to them may be a sheer solution; but they all may be individual issues, in which case you then need to implement many solutions. [Case D, Head of Supply Chain and manufacturing]
4.1.4.4. IT systems as a double-edge sword
The reliance on IT systems appears to play a dual role in Case D, as these are powerful tools to accommodate complexity but at the same time may prevent employees from developing a broader understanding of the internal SC and the organization. For instance, according to the head of SC and manufacturing, the systems’ design and the tendency to ‘think inside the box’ leads users to choose between the various available options in the ERP based on familiarity rather than on the ultimate outcome of these. This behaviour is further fostered by the scarce feedback employees receive on these outcomes and can be somewhat minimized with extensive training during the roll-out of the systems.
For the House of SC complexity for this case, please refer to Figure 6 in Online Appendix 2.
4.1.4.5. Case D’s key findings
Product design and portfolio structural complexity are found to increase the uncertainty and variability of SC operations. Maximizing resource utilization (e.g. lean workforce, reusable packaging, shared testing equipment) results in the addition of dependencies and reduction of resource flexibility and aggravates the effect of dynamic complexity on SC performance. Product portfolio and customer-base structural complexity result in more specific ‘spread dynamics’, increasing the resources needed to cope with product and customer dynamics. IT systems are presented as ‘a double-edge sword’ that can aid managers accommodate complexity but also hinder the development of a holistic understanding of the SC and dependencies within functions by employees.
Before moving on to the cross-case analysis, summarizes the key complexity areas for each within-case analysis.
Table 5. Within-case analysis: complexity areas per case.
4.2. Cross-case findings
The interplay between structural and dynamic complexity factors observed in all the four case studies are represented in the House of SC Complexity diagram in . The diagram reports different types of interplay having the negative influence on cost performance as a common effect. Relationships within structural and dynamic complexity factor categories are mapped in the left and top triangles, respectively; and those between these two complexity types are represented inside the diagram’s main central body. Arrows point towards the factor that is increased or aggravated by another factor. In other words, if an arrow points from factor X to factor Y, this represents that factor X either increases or aggravates Y, thus in either case increasing the negative effects of factor Y on SC cost performance. The figure serves to illustrate how the interplay between factors overall contribute to aggravating the influence of complexity factors on SC performance in the four case studies.
Figure 2. Interplay between structural and dynamic complexity factors showing a negative effect on cost performance. 1: Case A, 2: Case B, 3: Case C, 4: Case D. X→Y means X aggravates Y.
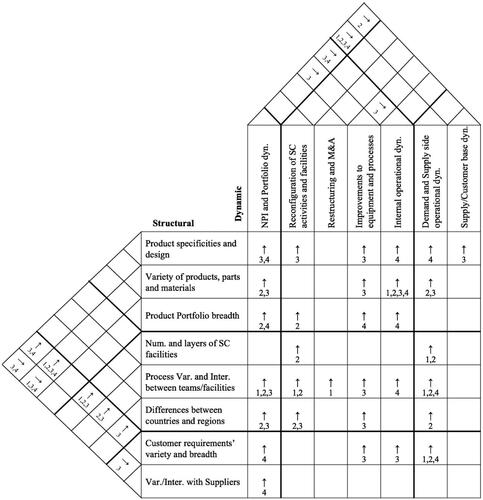
Because of the heterogeneity in the sample, some differences are observed regarding the interplay between structural and dynamic complexity factors in the four companies. For example, the variety and breadth of customer requirements with a notably smaller range of structural complexity factors in Cases B and A, which are mainly related to the variety of products and that of SC facilities, than in Cases C or D, where these requirements influence product specificities, the variety of internal processes and enhance the differences between different countries and regions in the SC. The analysis suggests that these differences derive from the different levels of involvement of customers through the various stages of the product lifecycle. Case B and Case A sell to end-consumers (B2C) and other customer types that do not demand close involvement in the design and manufacturing phases. In contrast, Cases C and D’s customers are predominantly businesses (B2B), which are more involved in the design of products, and demand higher levels of visibility and, in some cases, intervention during manufacturing. The companies’ operations model can influence the interplay between complexity factors. The interplay between product, customer and supplier complexity factors is found to be more relevant for Case D, which implements an Engineered to Order (ETO) model, than for the remaining Make to Stock (MTS) companies. By fixing the decoupling point in a more upstream position, customer may gain opportunities to be directly involved in design and production aspects. Consequently, the close relationship that exists between these parties during the engineering and production of customer solutions in Case D can facilitate the establishment of interplay between the variety and interactions with suppliers and the dynamics concerning the product portfolio of the company.
This paper shows that while structural and dynamic complexity predominantly hinder SC operational performance, some structural complexity factors may, however, enhance single performance objectives. For example, embracing the differences between internal SC facilities in different countries and regions allows Case C to reduce its lead-time to customers and Case A’s number of SC facilities and layers improves certain flexibility aspects. Then, these (and the remaining) structural complexity factors lead to a greater negative impact of dynamic complexity on SC cost serves to underline their criticality.
4.2.1. Interplay mechanisms
An in-depth examination of the relationships between structural and dynamic complexity factors allows identifying four interplay mechanisms. These four mechanisms underpin the aggravating effects of structural factors on dynamic factors that are represented in the central body of , as described next. The mechanisms are all driven by structural complexity and share as a common outcome the exacerbation of the negative impact of dynamic complexity on SC operations performance:
The four mechanisms identified in this paper are: silo-thinking, increased uncertainty, localism and limited adaptability. Each of these are defined next as well as their impact on performance.
Silo-thinking: It involves looking at the effects of complexity on the particular function, e.g. production, without considering the knock-on effects on other functions in the business, e.g. sales, marketing. Performance suffers because of low-level optimization gains in one function which may lead to high- (holistic) level losses in the overall business.
Increased uncertainty: The diversification of the product portfolio and increase of the number of SC facilities yield higher demand variations per SKU and location and accentuates customer-driven operational dynamics. This lack of predictability and uncertainty aggravates the impact of dynamic complexity on SC performance.
Localism: Managing the phenomena locally and on a case-by-case basis and to rely on different approaches to the same dynamic factor. The specificity of these dynamics increases the toll that the continuous exercise takes on SC operations performance.
Limited adaptability: The variety of products or product channels can become a barrier for the evolution of the internal SC, as extant design and processes must remain compatible and adequate for a variety of pre-existent products/channels. Hence, ensuring the performance of the internal SC for a more diversified range of products and requirements hinders the SC’s ability to adapt and underpins the negative effects of dynamic complexity factors on SC performance.
Evidence of these mechanisms from the case studies is presented next.
4.2.1.1. Silo-thinking
Structural complexity increases the amount of knowledge and information required in the management of SC activities and prevents managers from developing an end-to-end view of the internal SC and its processes. Managers tend to resort to silo-thinking and do not develop a sufficient understanding of the processes and needs of other SC areas. This is especially driven by the structural complexity of the organizational design and network of facilities of the internal SC, as these factors entail knowledge to be disseminated through the various teams, functions and facilities involved in SC activities. While a narrow focus on their domain can allow managers to achieve higher performance in the absence of dynamics, having a comprehensive understanding of the SC emerges to be critical in managing most dynamic complexity factors, especially of those that are not operational (e.g. reconfiguration of SC activities or organizational restructuring). Thus, silo-thinking aggravates the impact of dynamic complexity on SC performance. Cases A’s and B’s managers give examples of issues encountered in the management of the introduction of new products, the integration of acquired companies or the reallocation of SC activities underline that all involved functions must be knowledgeable of their dependencies with other functions to minimize the negative effect of these activities on SC performance. The lack of such a holistic view prevents managers from identifying and sharing timely information with other teams; thus, hindering coordination efforts.
4.2.1.2. Localism
Variety and diversity induce more local and specific dynamics that differ across territories, customer segments and product lines giving place to what Case D’s head of SC referred to as ‘spread dynamics’. This forces the SC to manage these phenomena locally and on a case-by-case basis and to rely on different approaches to the same dynamic factor (hence the term ‘localism’, which is employed here without any reference to its geographical meaning). Consequently, structural complexity makes SC dynamic and management solutions to dynamic complexity diverge and reduces the extent to which the SC can leverage scale in managing dynamic complexity. For instance, the NPI process followed by Case C is not compatible with one of the product lines in its portfolio. Managers cannot accommodate the particularities of this product line forces the process to diverge into two. Also, the process the firm has in place to manage continuous improvements in its production facilities differs between the various facilities due to the large differences between them. In the same vein, the implementation of a new unique SC design solution in Case B (part of an SC reconfiguration activity), diverged into a range of solutions because of the specificities of different areas or regions. This phenomenon does not only increase the number of resources and data needed to manage dynamic complexity factors throughout the internal SC, but also tends to further accentuate extant specificities, hence driving structural complexity and setting a self-reinforcing detrimental cycle (i.e. positive feedback).
4.2.1.3. Limited adaptability
The effect of extant structural complexity to reduce the extent to which the SC can adapt to dynamics. The variety of products can become a barrier for the evolution of the internal SC, as extant design and processes have to remain compatible and adequate for a variety of pre-existent products. One of the first steps that managers in Case C take to verify whether the design of a potential new product can or not be manufactured in a given plant is ensuring that the modifications to the production line required by the new product do not affect the production of all the wheel models that are already manufactured in the facility. Thus, ‘[…] each modification normally covers the full range of products’ (General plant manager deputy in Case C). Similarly, the breadth of Case B’s portfolio posed relevant constraints on (and in fact prevented) the implementation of SC reconfiguration activities. Hence, ensuring the internal SC performance for a more diversified range of products and requirements hinders the SC’s ability to adapt and underpins the negative effects of dynamic complexity factors on SC performance.
4.2.1.4. Increased uncertainty
Structural complexity increases the uncertainty associated with dynamic complexity factors in different ways. For instance, the diversification of the product portfolio and increase of the number of SC facilities yield higher demand variations per SKU and location and accentuates customer-driven operational dynamics. The high number of product variants and SKUs (e.g. in Cases A and B) increase management’s probability of making mistakes, as, for example, ‘when you got large portfolio breadth and size you have high variability of process, and uncertainty and special types of test equipment […], which will bring more room for error and cost’ (Case D’s Head of Supply Chain and manufacturing). Variety and dependencies in the internal SC reduce managers’ ability to foresee the outcomes and implications to their actions. Therefore, structural complexity accentuates the need to rely on testing and iteration during the development and introduction of products, the implementation of new SC designs or when switching between partners. For example, the difficulty to understand the interactions between product and production line specificities made Case C’s managers unable to understand and solve product quality issues during the introduction of a new product. Although the issue was finally solved by acting simultaneously on three manufacturing operations, the adopted solution was solely a result of testing and iteration, not one whose effects on the product could be foreseen by managers. This lack of predictability and uncertainty aggravates the impact of dynamic complexity on SC performance.
The interplay between complexity factors in the four case studies can be coded according to the mechanism that is predominant for each given pair. By doing this for each individual case study first, and subsequently aggregating the results across cases, the four interplay mechanisms are mapped in this figure. It provides with a visual representation of the areas of influence in which each interplay mechanism is prevalent as observed in the four case studies reported in this paper. The dotted lines in this figure represent the blurry boundaries of the influence area that were observed.
The synthesis offered in and shows how structural and dynamic complexity interact and jointly hinder SC cost performance in all case studies. It can be noted that the role that different interplay mechanisms varies in importance across companies. For example, while in Case A structural complexity aggravates the cost of product portfolio-related dynamic complexity, due to mainly increased silo-thinking, in Case B this effect is also due to localism and increased uncertainty, because of the higher structural complexity of Case B’s product portfolio.
Figure 3. Predominant areas of influence of interplay mechanisms as observed in the four case studies.
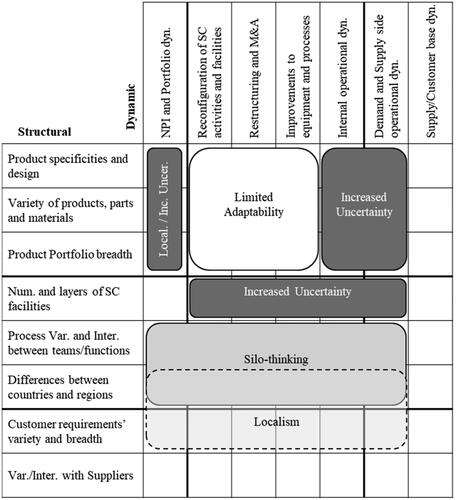
While a single predominant mechanism can be identified in most of the interactions between factors, there are some areas in which several mechanisms appear to be equally relevant. Consequently, some overlap between the areas can be observed in , as is the case of silo-thinking and localism in ruling the interplay between the ‘Differences between countries and regions’ and dynamic complexity. Similarly, increased uncertainty and localism both affect the interplay between product structural and dynamic complexity. In addition, there are a few interactions in which no prevailing mechanism arises from the analysis, mainly due to the limited data gathered on these particular interactions (specifically ‘Variety and interactions with suppliers’ vs ‘NPIs and portfolio dynamics’; ‘Product specificities and design’ vs ‘Supply and customer base dynamics’).
5. Discussion
This paper set out to investigate the research question: What are the mechanisms underpinning the interplay between structural and dynamic complexity in supply chains? We found four mechanisms: silo-thinking, localism, limited adaptability and increased uncertainty.
Despite several authors highlight its theoretical and practical relevance (Serdarasan Citation2013; Bozarth et al. Citation2009; Casti Citation1979), to the best of authors’ knowledge, there is no prior empirical research in the SC domain that has examined in detail the interplay mechanisms between individual factors of structural and dynamic complexity among themselves. Results are also relevant from a theoretical perspective, since there is very limited research grounded on Contingency Theory that delves into the interactions between contingency factors and their cumulative effect on performance.
Our results are in a similar vein with Dittfeld, Scholten, and Van Donk’s (Citation2018) paper, which studied horizontal, vertical and diagonal interactions in the food SC. However, this paper provides a step forward that can be applied to more than one sector, via a novel framework, namely the House of SC Complexity, to visualize the effects of individual complexity factors on each other. This could save time and energy for practitioners who would like to achieve the desired results in complexity management.
Nonetheless, some of the core concepts underlying the encountered mechanisms are largely theoretically discussed in the complexity literature (e.g. Menezes, Ruiz-Hernández, and Chen Citation2021), supporting the relevance of these issues and the alignment and coherence of the findings with extant literature, i.e. their external validity. The study contributes to this body of work, discussed next, by uncovering the role of these constructs in the interplay between structural and dynamic complexity. For instance, in discussing SC complexity Christopher (Citation2016) argues that the structural complexity of the organizational design can drive functions to become silos over time. This is in line with this study’s findings, in which silo-thinking is found to be the predominant interplay mechanism between functions and facilities and dynamic complexity factors. In the same manner, these findings resonate with the work of Lepore, Montgomery, and Siepe (Citation2016) which states that traditional organizational models result in silo-thinking that is not adequate for SCs or firms operating in today’s complex reality.
Adaptability is another relevant theme in the SC complexity literature (Eckstein et al. Citation2015; Manuj and Sahin Citation2011; Choi, Dooley, and Rungtusanatham Citation2001; Giannoccaro Citation2015; Masson et al. Citation2007; Hoole Citation2005). There are contrasting arguments in the literature regarding the relationship between adaptability and complexity. On one hand, some researchers argue that the complex structure of SCs can in fact underpin adaptability (Ashmos, Duchon, and McDaniel Citation2000; Stacey Citation1995). On the other hand, the literature also argues that complexity can in turn reduce SC adaptability (Masson et al. Citation2007; Hoole Citation2005; Davis, Eisenhardt, and Bingham Citation2009). Our findings suggest that excessive structural complexity (especially product portfolio-related) results in limited adaptability that aggravates the effect of dynamic complexity on SC performance, therefore supporting the latter view.
With regards to increased uncertainty, prior works discussing the links between complexity and uncertainty are quite abundant in the literature. Indeed, dynamic complexity is related to the uncertainty of issues that unfold with time (Sivadasan et al. Citation2002; Bozarth et al. Citation2009; Frizelle and Woodcock Citation1995), but prior research has also underlined the role of complexity in increasing the uncertainty in the SC (Manuj and Sahin Citation2011; Bode and Wagner Citation2015; Christopher Citation2016). Manuj and Sahin (Citation2011) propose a positive relationship between SC complexity and higher uncertainty in the form of unexpected and undesirable outcomes. This proposition is coherent with one of the findings of the present study: the structural complexity of the internal network of facilities increases the uncertainty associated to various dynamic complexity factors, including unexpected customer demand and the reallocation of SC activities in the network (most notably in Cases A and B).
Therefore, the following proposition is put forth:
Proposition 1.1: Silo-thinking, localism, limited adaptability, and increased uncertainty drive the effect of structural complexity on the relationship between dynamic complexity and SC cost performance.
Furthermore, through the four identified mechanisms of interplay, the structural complexity of the product portfolio and design, internal SC design and external SC aggravates the dynamic complexity factors associated to the internal SC function leading to a worse cost performance. In other words, the findings suggest that, due to the multifaceted interplay between complexity factors, the joint impact of structural and dynamic complexity on SC performance is greater than what the combination of their individual separate effects would otherwise be (i.e. synergistic impact). We must note, however, that because the effects of dynamic complexity on structural complexity lie outside the scope of the study, we cannot posit this synergistic joint effect to be symmetric with respect to the two complexity types, but only that structural complexity leads to such synergistic effect. Hence, the following proposition is put forth:
Proposition 1.2: Structural complexity exacerbates the negative effect of dynamic complexity on SC cost; thus, resulting in a synergistic combined negative effect of structural and dynamic complexity on SC cost.
The study’s findings on the interplay between structural and dynamic complexity highlight the multifaceted nature of the relationship between these two types of complexity and the coexistence of various interplay mechanisms. Therefore, complexity can be extremely challenging to manage and simplistic reductionist managerial approaches to the subject are not likely to be successful. Rather, the complicatedness of the relationship between structural and dynamic complexity suggests that a holistic approach is called for. A first step in this direction is to consider whether complexity reduction or accommodation strategies may be more suitable to address the four mechanisms of interplay unveiled in the study. The findings suggest that the mechanisms of silo-thinking and increased uncertainty can be accommodated by information sharing and organizational means, but the interplay mechanisms of localism and limited adaptability appear to be more related to the physical structure of SC elements and thus mainly manageable by reducing complexity, i.e. reducing the factors involved in the interplay.
6. Conclusions
6.1. Contributions to theory
Relying on the findings from four in-depth case studies, in this paper we argue that structural complexity exacerbates the negative effect of dynamic complexity on the efficiency of the SC function through four mechanisms of interplay: silo-thinking, localism, limited adaptability and increased uncertainty. This results in a synergistic joint negative effect of the two complexity types on SC operations performance. These mechanisms are industry independent and vary in strength between different groups of complexity factors, as represented in . The study also provides with a rich empirical description of the interplay and enhances the usability of these findings for further theory building and testing.
The propositions stated in this paper could be further developed and used later for a possible deductive approach. Furthermore, the contingency insights in this paper are in line with the findings by Ateş et al. (Citation2021) that complexity can lead to trade-offs in some performance dimensions, such as cost (as studied in this paper), but it could lead to improvement in other performance dimensions such as innovation (outside the scope of this paper).
The present study also contributes to expanding and strengthening contingency research in Supply Chain Management (SCM). Results from four cases each operating in different strategic manufacturing contexts (Sousa and Voss Citation2008) show that, to fully understand the role of contingency factors and manage their cumulative effect on SC performance, it is necessary to investigate possible interplay mechanisms by adopting a holistic view. Indeed, despite Contingency Theory is quite common in quantitative studies in the Organization Design and SCM domains (e.g. Parker and van Witteloostuijn Citation2010; Flynn, Huo, and Zhao Citation2010; Tangpong, Hung, and Ro Citation2010; Grötsch, Blome, and Schleper Citation2013; Wong, Lai, and Cheng Citation2011; Wong, Lai, and Bernroider Citation2015), so far research has largely overlooked investigating the cumulative effect of multiple contingency factors, which are considered in this paper. Furthermore, there are very few examples (e.g. Alazzabi, Mustafa, and Issa Citation2021) of qualitative inductive research addressing the phenomenon. In the present study, we offer a conceptual framework and a methodology, to be used in explorative research, for investigating the interplay mechanisms between contingency factors and their cumulative effect on SC operational performance, with a particular focus on cost.
6.2. Managerial implications
Complexity may be leveraged in other areas of organizations or may serve to accomplish tactical or strategic goals. Yet, complexity hinders SC operations quality, speed and, especially, cost performance dimensions. Consequently, managers must fully acknowledge the negative effects of complexity for the SC performance and ensure that these are truly compensated for at the level of the firm.
Our findings show that the relationship between structural and dynamic complexity is not a straightforward one, as different mechanisms coexist between complexity factors. This calls for the adoption of holistic complexity management approaches that can target groups of complexity factors and the interplay between them. This is especially critical for SCs operating in dynamic environments under significant change and/or turbulence (Christopher and Holweg Citation2011), or that adopt strategies that demand of intense dynamism (e.g. extensive product innovation, inorganic growth via acquisitions) since the extent to which the SC function may be able to operate under the resulting dynamic complexity on a fixed amount of resources (i.e. cost) can severely be affected by its structural complexity.
Managers can leverage on the description of the interplay and its underlying mechanisms to recognize and address this phenomenon in their organizations. Specifically, the use of the House of SC Complexity diagram to represent different aspects of the interplay between structural and dynamic factors offers managers with a tool to assess which complexity factors in their organization might be related to others (), and which interplay mechanism(s) might be predominant (). These insights can play a critical role in informing SC management decisions and, especially, complexity management decisions. Furthermore, if complemented with the guiding logics for selecting reducing or accommodating complexity management practices, as discussed in Fernández Campos, Trucco, and Huaccho Huatuco (Citation2019), managers can make better decisions to effectively govern interplay mechanisms and mitigate their negative impact on SC performance.
6.3. Limitations and future research
The use of case studies is regarded as an appropriate first step in the theory-building process (Meredith Citation1998), but one that demands from further research in order to contrast and enhance the robustness and generalisability of the findings. Findings are limited with regard to complexity’s influence on flexibility. A possible reason for this, and one that fits the empirical observations, is that flexibility is generally seen as a multi-dimensional construct (Sethi and Sethi Citation1990; Browne et al. Citation1984), hence allowing for complexity to benefit and hinder different dimensions or types of flexibility. Previous research has examined the relationship between complexity and flexibility (e.g. Chryssolouris et al. Citation2013), but adopting a multi-dimensional conceptualization of flexibility could serve to further clarify this relationship.
Another limitation is that in this research the outputs of the specific companies cannot be extended for a generalization to their SCs. This is a limitation of the case study methodology, which could be counteracted by using complementary methodologies, e.g. surveys, computer simulations or meta-analyses.
Similarly, the small sample size implies that not all the mechanisms involved in ruling the interplay between structural and dynamic complexity in other industries may have been captured. A relevant research opportunity to this respect is to investigate whether the unveiled interplay mechanisms remain valid, i.e. rule the interplay between structural and dynamic complexity. A further suggestion for future research is to explore whether the area of influence of the identified mechanisms holds for larger or smaller units of analyses.
TPPC_2114959_Supplementary_Material
Download PDF (955.3 KB)Acknowledgements
The authors would like to thank the managers at the four manufacturing organizations that have participated in this study, who have generously provided their time and data.
Disclosure statement
No potential conflict of interest was reported by the author(s).
Additional information
Notes on contributors
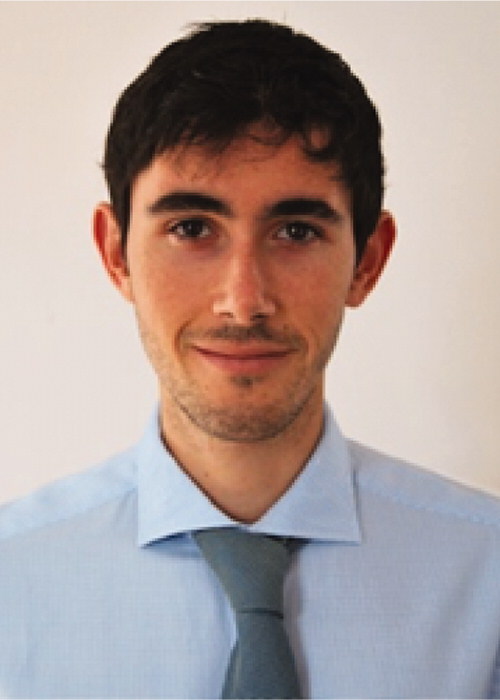
Pablo Fernández Campos
Pablo Fernández Campos is a Business Consultant and Designer. He earned his PhD in Management, Economics and Industrial Engineering at the School of Management in Politecnico di Milano. He holds an MSc in Industrial Management and in Mechanical Engineering. His research interest is in the area of operations and supply chain management, with an especial interest in complexity management and in risk and resilience. He has recently authored several journal articles that are published or under review in various operations management journals.
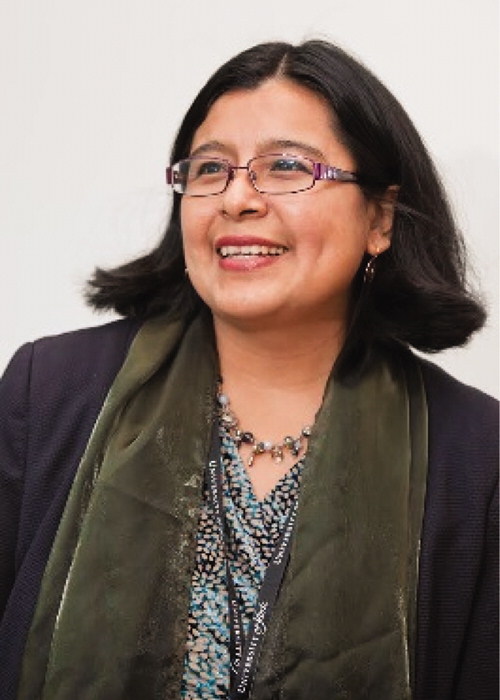
Luisa Huaccho Huatuco
Luisa Huaccho Huatuco is a Reader in Operations Management at the University of York. Her current research interests at firm and supply chain levels within the manufacturing context include: sustainable supply chains, management of disruptions to distribution/logistics, high value manufacturing and complexity in the supply chain. She has disseminated the results of her research using various routes including: internationally ranked academic journals and several international conferences. She has also been fostering links with researchers in Latin America and Europe.
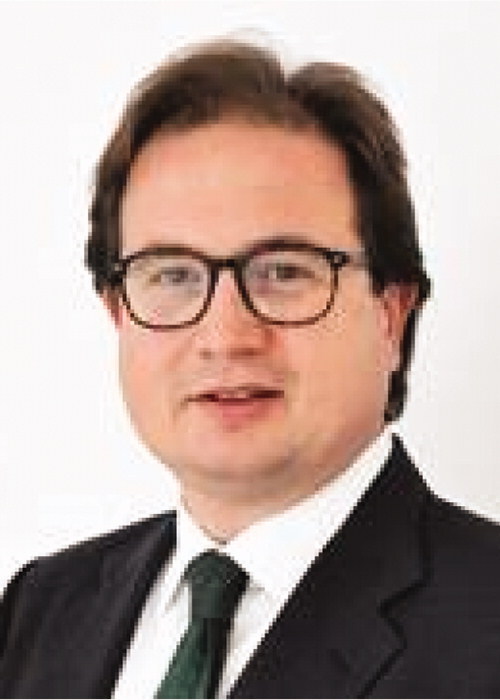
Paolo Trucco
Paolo Trucco is Full Professor of Industrial Risk Management at the School of Management, Politecnico di Milano, where he is also Director of the PhD Programme in Management Engineering. His research interests concentrate in operational risk management and resilience of complex socio-technical systems and global supply chains, with expertise in the manufacturing, oil & gas, energy, transportation and healthcare sectors. He is scientific advisor of DG Home Affairs (European Commission) and of the Lombardy Region Government (Italy) on Programmes for Critical Infrastructure Protection and Key Resource Supply Chain Resilience.
References
- Aguila, J. O., and W. ElMaraghy. 2018. “Structural Complexity and Robustness of Supply Chain Networks Based on Product Architecture.” International Journal of Production Research 56 (20): 6701–6718. doi:10.1080/00207543.2018.1489158.
- Aitken, J., C. Bozarth, and W. Garn. 2016. “To Eliminate or Absorb Supply Chain Complexity: A Conceptual Model and Case Study.” Supply Chain Management: An International Journal 21 (6): 759–774. doi:10.1108/SCM-02-2016-0044.
- Alazzabi, W. Y. E., H. Mustafa, and M. Issa. 2021. “Conceptualising the Interaction among Organisational Factors towards Internal Control Quality.” Journal of Financial Crime 28 (4): 1093–1105. doi:10.1108/JFC-07-2020-0137.
- Ashkenas, R. 2007. “Simplicity-minded Management.” Harvard Business Review 85 (12): 101–109, 146.
- Ashmos, D. P., D. Duchon, and R. R. McDaniel Jr. 2000. “Organizational Responses to Complexity: The Effect on Organizational Performance.” Journal of Organizational Change Management 13 (6): 577–595. doi:10.1108/09534810010378597.
- Ateş, M. A., R. Suurmond, D. Luzzini, and D. Krause. 2021. “Order from Chaos: A Meta-analysis of Supply Chain Complexity and Firm Performance.” Journal of Supply Chain Management 58 (1): 3–30. doi:10.1111/jscm.12264.
- Ateş, Melek Akın, and Huriye Memiş. 2021. “Embracing Supply Base Complexity: The Contingency Role of Strategic Purchasing.” International Journal of Operations & Production Management 41 (6): 830–859. doi:10.1108/IJOPM-09-2020-0662.
- Azadegan, A., P. C. Patel, A. Zangoueinezhad, and K. Linderman. 2013. “The Effect of Environmental Complexity and Environmental Dynamism on Lean Practices.” Journal of Operations Management 31 (4): 193–212.
- Blome, C., T. Schoenherr, and D. Eckstein. 2014. “The Impact of Knowledge Transfer and Complexity on Supply Chain Flexibility: A Knowledge-based View.” International Journal of Production Economics 147: 307–316.
- Bai, C., and J. Sarkis. 2018. “Honoring Complexity in Sustainable Supply Chain Research: A Rough Set Theoretic Approach.” Production Planning & Control 29 (16): 1367–1384. doi:10.1080/09537287.2018.1535133.
- Barratt, M., T. Y. Choi, and M. Li. 2011. “Qualitative Case Studies in Operations Management: Trends, Research Outcomes, and Future Research Implications.” Journal of Operations Management 29 (4): 329–342. doi:10.1016/j.jom.2010.06.002.
- Benbasat, I., D. K. Goldstein, and M. Mead. 1987. “The Case Research Strategy in Studies of Information Systems.” MIS Quarterly 11 (3): 369–386. doi:10.2307/248684.
- Birkie, S. E., and P. Trucco. 2020. “Do Not Expect Others Do What You Should! Supply Chain Complexity and Mitigation of the Ripple Effect of Disruptions.” International Journal of Logistics Management 31 (1): 123–144.
- Birkie, S. E., P. Trucco, and P. F. Campos. 2017. “Effectiveness of Resilience Capabilities in Mitigating Disruptions: Leveraging on Supply Chain Structural Complexity.” Supply Chain Management: An International Journal 22 (6): 506–521. doi:10.1108/SCM-01-2017-0009.
- Bode, C., and S. M. Wagner. 2015. “Structural Drivers of Upstream Supply Chain Complexity and the Frequency of Supply Chain Disruptions.” Journal of Operations Management 36 (1): 215–228. doi:10.1016/j.jom.2014.12.004.
- Bozarth, C., D. P. Warsing, B. Flynn, and E. J. Flynn. 2009. “The Impact of Supply Chain Complexity on Manufacturing Plant Performance.” Journal of Operations Management 27 (1): 78–93. doi:10.1016/j.jom.2008.07.003.
- Browne, J., D. Dubois, K. Rathmill, S. P. Sethi, and K. E. Stecke. 1984. “Classification of Flexible Manufacturing Systems.” The FMS Magazine 2 (2): 114–117.
- Busogi, M., K. Ransikarbum, Y. G. Oh, and M. Kim. 2017. “Computational Modelling of Manufacturing Choice Complexity in a Mixed-model Assembly Line.” International Journal of Production Research 55 (20): 5976–5990. doi:10.1080/00207543.2017.1319088.
- Caniato, Federico, and Andreas Größler. 2015. “The Moderating Effect of Product Complexity on New Product Development and Supply Chain Management Integration.” Production Planning & Control 26 (16): 1306–1317. doi:10.1080/09537287.2015.1027318.
- Casti, J. L. 1979. “Connectivity, Complexity and Catastrophe in Large-scale Systems.” International Series on Applied Systems Analysis 7. Chichester: John Wiley & Sons.
- Choi, T. Y., K. J. Dooley, and M. Rungtusanatham. 2001. “Supply Networks and Complex Adaptive Systems: Control versus Emergence.” Journal of Operations Management 19 (3): 351–366. doi:10.1016/S0272-6963(00)00068-1.
- Christopher, M. 2016. Logistics & Supply Chain Management. Harlow, England: FT Publishing International.
- Christopher, M., and M. Holweg. 2011. “‘Supply Chain 2.0’: Managing Supply Chains in the Era of Turbulence.” International Journal of Physical Distribution & Logistics Management 41 (1): 63–82. doi:10.1108/09600031111101439.
- Chryssolouris, G., K. Efthymiou, K. N. Papakostas, D. Mourtzis, and A. Pagoropoulos. 2013. “Flexibility and Complexity: Is It a Trade-off?” International Journal of Production Research 51 (23-24): 6788–6802. doi:10.1080/00207543.2012.761362.
- Coffey, A., and P. Atkinson. 1996. Making Sense of Qualitative Data: Complementary Research Strategies. Thousand Oaks: SAGE Publications.
- Collinson, S., and M. Jay. 2012. From Complexity to Simplicity: Unleash Your Organisation’s Potential. Basingstoke, UK: Palgrave Macmillan.
- Davis, J. P., K. M. Eisenhardt, and C. B. Bingham. 2009. “Optimal Structure, Market Dynamism, and the Strategy of Simple Rules.” Administrative Science Quarterly 54 (3): 413–452. doi:10.2189/asqu.2009.54.3.413.
- Dey, I. 1993. Qualitative Data Analysis: A User-friendly Guide for Social Scientists. London: Routledge.
- Dittfeld, H., K. Scholten, and D. P. Van Donk. 2018. “Burden or Blessing in Disguise: interactions in Supply Chain Complexity.” International Journal of Operations & Production Management 38 (2): 314–332. doi:10.1108/IJOPM-03-2017-0158.
- Eckstein, D., M. Goellner, C. Blome, and M. Henke. 2015. “The Performance Impact of Supply Chain Agility and Supply Chain Adaptability: The Moderating Effect of Product Complexity.” International Journal of Production Research 53 (10): 3028–3046. doi:10.1080/00207543.2014.970707.
- Eisenhardt, K. M. 1989. “Building Theories from Case Study Research.” The Academy of Management Review 14 (4): 532–550. doi:10.2307/258557.
- Ferdows, K. 2018. “Keeping up with Growing Complexity of Managing Global Operations.” International Journal of Operations & Production Management 38 (2): 390–402. doi:10.1108/IJOPM-01-2017-0019.
- Fernández Campos, P., P. Trucco, and L. Huaccho Huatuco. 2019. “Managing Structural and Dynamic Complexity in Supply Chains: Insights from Four Case Studies.” Production Planning & Control 30 (8): 611–623. doi:10.1080/09537287.2018.1545952.
- Flynn, B. B., B. Huo, and X. Zhao. 2010. “The Impact of Supply Chain Integration on Performance: A Contingency and Configuration Approach.” Journal of Operations Management 28 (1): 58–71. doi:10.1016/j.jom.2009.06.001.
- Frizelle, G., and E. Woodcock. 1995. “Measuring Complexity as an Aid to Developing Operational Strategy.” International Journal of Operations & Production Management 15 (5): 26–39. doi:10.1108/01443579510083640.
- Giannoccaro, I. 2015. “Adaptive Supply Chains in Industrial Districts: A Complexity Science Approach Focused on Learning.” International Journal of Production Economics 170: 576–589. doi:10.1016/j.ijpe.2015.01.004.
- Gottfredson, M., and K. Aspinall. 2005. “Innovation Versus Complexity.” Harvard Business Review 83 (11): 62–71.
- Größler, A., A. Grübner, and P. Milling. 2006. “Organisational Adaptation Processes to External Complexity.” International Journal of Operations & Production Management 26 (3): 254–281. doi:10.1108/01443570610646193.
- Grötsch, V. M., C. Blome, and M. C. Schleper. 2013. “Antecedents of Proactive Supply Chain Risk Management – A Contingency Theory Perspective.” International Journal of Production Research 51 (10): 2842–2867. doi:10.1080/00207543.2012.746796.
- Gunasekaran, A., C. Patel, and R. E. McGaughey. 2004. “A Framework for Supply Chain Performance Measurement.” International Journal of Production Economics 87 (3): 333–347. doi:10.1016/j.ijpe.2003.08.003.
- Hauser, J. R., and D. Clausing. 1988. “The House of Quality.” Harvard Business Review 66 (5): 63–73.
- Hoole, R. 2005. “Five Ways to Simplify Your Supply Chain.” Supply Chain Management: An International Journal 10 (1): 3–6. doi:10.1108/13598540510578306.
- Inman, R. R., and D. E. Blumenfeld. 2014. “Product Complexity and Supply Chain Design.” International Journal of Production Research 52 (7): 1956–1969. doi:10.1080/00207543.2013.787495.
- Ketokivi, M. 2009. “Elaborating the Contingency Theory of Organisations: The Case of Manufacturing Flexibility Strategies.” Production and Operations Management 15 (2): 215–228. doi:10.1111/j.1937-5956.2006.tb00241.x.
- Lawrence, P. R., and J. W. Lorsch. 1967. “Differentiation and Integration in Complex Organizations.” Administrative Science Quarterly 12 (1): 1–47. doi:10.2307/2391211.
- Lepore, D., A. Montgomery, and G. Siepe. 2016. “Managing Complexity in Organizations through a Systemic Network of Projects.” In Applications of Systems Thinking and Soft Operations Research in Managing Complexity, edited by A. J. Masys, 35–69. Switzerland: Springer International Publishing.
- Luthans, F., and T. I. Stewart. 1977. “A General Contingency Theory of Management.” The Academy of Management Review 2 (2): 181–195. doi:10.5465/amr.1977.4409038.
- MacQueen, K. M., and G. Guest. 2008. “An Introduction to Team-based Qualitative Research.” In Handbook for Team-based Qualitative Research edited by Guest and MacQueen. Lanham, MD: Alta Mira Press.
- Manuj, I., and F. Sahin. 2011. “A Model of Supply Chain and Supply Chain Decision-making Complexity.” International Journal of Physical Distribution & Logistics Management 41 (5): 511–549. doi:10.1108/09600031111138844.
- Masson, R., L. Iosif, G. MacKerron, and J. Fernie. 2007. “Managing Complexity in Agile Global Fashion Industry Supply Chains.” The International Journal of Logistics Management 18 (2): 238–254. doi:10.1108/09574090710816959.
- Mariotti, J. L. 2007. The Complexity Crisis: Why Too Many Products, Markets, and Customers are Crippling Your Company-and What to do About it. Simon and Schuster.
- Maylor, H., R. Vidgen, and S. Carver. 2008. “Managerial Complexity in Project-Based Operations: A Grounded Model and Its Implications for Practice.” Project Management Journal 39 (1_suppl): S15–S26. doi:10.1002/pmj.20057.
- Menezes, M. B. C., D. Ruiz-Hernández, and Y.-C. Chen. 2021. “On the Validity and Practical Relevance of a Measure for Structural Complexity.” International Journal of Production Economics 240: 108243. doi:10.1016/j.ijpe.2021.108243.
- Meredith, J. 1998. “Building Operations Management Theory through Case and Field Research.” Journal of Operations Management 16 (4): 441–454. doi:10.1016/S0272-6963(98)00023-0.
- Miles, M. B., and A. M. Huberman. 1994. Qualitative Data Analysis: An Expanded Sourcebook. Thousand Oaks, CA: SAGE Publications.
- Mocker, M., P. Weill, and S. L. Woerner. 2014. “Revisiting Complexity in the Digital Age.” MIT Sloan Management Review 55 (4): 72–81.
- Park, K., and G. E. Okudan Kremer. 2015. “Assessment of Static Complexity in Design and Manufacturing of a Product Family and Its Impact on Manufacturing Performance.” International Journal of Production Economics 169: 215–232. doi:10.1016/j.ijpe.2015.07.036.
- Parker, S. C., and A. van Witteloostuijn. 2010. “A General Framework for Estimating Multidimensional Contingency Fit.” Organization Science 21 (2): 540–553. doi:10.1287/orsc.1090.0464.
- Perona, M, and G. Miragliotta. 2004. “Complexity Management and Supply Chain Performance Assessment. A Field Study and a Conceptual Framework.” International Journal of Production Economics 90 (1): 103–115. doi:10.1016/S0925-5273(02)00482-6.
- Pratt, M. G. 2009. “From the Editors: For the Lack of a Boilerplate: Tips on Writing up (and Reviewing) Qualitative Research.” Academy of Management Journal 52 (5): 856–862. doi:10.5465/amj.2009.44632557.
- Reeves, M., S. Levin, T. Fink, and A. Levina. 2020. “Taming Complexity: Make Sure the Benefits of Any Addition to an Organization’s Systems Outweighs Its Costs.” Harvard Business Review 2020 (January–February): 113–121.
- Ruiz-Hernández, D., M. B. C. Menezes, and A. Amrani. 2019. “An Information-content Based Measure of Proliferation as a Proxi for Structural Complexity.” International Journal of Production Economics 212: 78–91. doi:10.1016/j.ijpe.2019.02.008.
- Saldaña, J. 2015. The Coding Manual for Qualitative Researchers. London: SAGE Publications.
- Serdarasan, S. 2013. “A Review of Supply Chain Complexity Drivers.” Computers & Industrial Engineering 66 (3): 533–540. doi:10.1016/j.cie.2012.12.008.
- Sethi, A. K., and S. P. Sethi. 1990. “Flexibility in Manufacturing: A Survey.” International Journal of Flexible Manufacturing Systems 2 (4): 289–328. doi:10.1007/BF00186471.
- Shepard, M., and J. G. Hougland. 1978. “Contingency Theory: “Complex Man” or “Complex Organization”?” The Academy of Management Review 3 (3): 413–427.
- Sivadasan, S., J. Efstathiou, G. Frizelle, R. Shirazi, and A. Calinescu. 2002. “An Information-Theoretic Methodology for Measuring the Operational Complexity of Supplier-customer Systems.” International Journal of Operations & Production Management 22 (1): 80–102. doi:10.1108/01443570210412088.
- Smart, J., A. Calinescu, and L. Huaccho Huatuco. 2013. “Extending the Information-theoretic Measures of the Dynamic Complexity of Manufacturing Systems.” International Journal of Production Research 51 (2): 362–379. doi:10.1080/00207543.2011.638677.
- Sousa, R., and C. A. Voss. 2008. “Contingency Research in Operations Management Practices.” Journal of Operations Management 26 (6): 697–713. doi:10.1016/j.jom.2008.06.001.
- Stacey, R. D. 1995. “The Science of Complexity: An Alternative Perspective for Strategic Change Processes.” Strategic Management Journal 16 (6): 477–495. doi:10.1002/smj.4250160606.
- Stock, G. N., N. P. Greis, and J. D. Kasarda. 2000. “Enterprise Logistics and Supply Chain Structure: The Role of Fit.” Journal of Operations Management 18 (5): 531–547. doi:10.1016/S0272-6963(00)00035-8.
- Tangpong, C., K.-T. Hung, and Y. K. Ro. 2010. “The Interaction Effect of Relational Norms and Agent Cooperativeness on Opportunism in Buyer–Supplier Relationships.” Journal of Operations Management 28 (5): 398–414. doi:10.1016/j.jom.2009.12.001.
- Vachon, S., and R. D. Klassen. 2002. “An Exploratory Investigation of the Effects of Supply Chain Complexity on Delivery Performance.” IEEE Transactions on Engineering Management 49 (3): 218–230.
- Voss, C., N. Tsikriktsis, and M. Frohlich. 2002. “Case Research in Operations Management.” International Journal of Operations & Production Management 22 (2): 195–219. doi:10.1108/01443570210414329.
- Wong, C. W. Y., K.-H. Lai, and E. W. N. Bernroider. 2015. “The Performance of Contingencies of Supply Chain Information Integration: The Roles of Product and Market Complexity.” International Journal of Production Economics 165: 1–11. doi:10.1016/j.ijpe.2015.03.005.
- Wong, C. W. Y., K.-H. Lai, and T. C. E. Cheng. 2011. “Value of Information Integration to Supply Chain Management: Roles of Internal and External Contingencies.” Journal of Management Information Systems 28 (3): 161–200. doi:10.2753/MIS0742-1222280305.
- Wu, Y., G. Frizelle, and J. Efstathiou. 2007. “A Study on the Cost of Operational Complexity in Customer–Supplier Systems.” International Journal of Production Economics 106 (1): 217–229. doi:10.1016/j.ijpe.2006.06.004.
- Yin, R. K. 2003. Case Study Research: Design and Methods. Applied Social Research Methods Series. Thousand Oaks, CA: SAGE Publications.