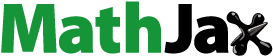
Abstract
Circular Manufacturing (CM) adoption is highly reinforced by data exploitation, gathered, used and shared thanks to specific technologies. Manufacturers still need to be supported in using data to make more informed decisions in CM; to do that, they first need to be conscious of their current state. To achieve this goal, this study aims to develop a maturity model (MM) to evaluate manufacturers exploiting and valuing data in CM to support their decision-making processes. The MM, based on five levels and four analysis dimensions, operates the assessment through a questionnaire composed of normative answers. The model was conceived by relying on the scientific literature. It was verified and improved through focus groups and interviews and validated through a pilot application in two different manufacturing companies. Finally, the MM was applied in two other manufacturing companies to assess the empirical evidence of the obtainable benefits.
1. Introduction
Beginning in the twentieth century, strategic decision plans in manufacturing companies started to be influenced by a diffused awareness to move towards sustainable and circular-oriented solutions. Today, more than ever, this consciousness needs to be practically applied and further monitored (Kravchenko, McAloone, and Pigosso Citation2020). Among the promising solutions for sustainable manufacturing, circular manufacturing (CM) plays a significant role. CM is the application of circular economy (CE) values in manufacturing companies, aimed at extending product lifecycle, reducing resource use, and creating closed-loop of resources (Bocken, Miller, and Evans Citation2016) through the concurrent adoption of CM strategies. These strategies, proposed by Acerbi and Taisch (Citation2020b), include recycling, remanufacturing, industrial symbiosis, etc. Adopting the CM paradigm requires radical cultural change in manufacturing companies (Ritzén and Sandström Citation2017). These changes can successfully modify the original make-use-dispose business models through the introduction of new processes and the involvement of local communities and external stakeholders (Mulrow et al. Citation2017) along with the consolidation of the circular supply chain concept (Lahane, Kant, and Shankar Citation2020; Taddei et al. Citation2022). Necessary is an internal re-design of the strategic plans and the establishment of external collaborations (Garcia-Muiña et al. Citation2018) requiring a strong cohesiveness, alignment and involvement of the manufacturing company’s functions supported by the establishment of structured information flows (Zeiss Citation2019; Acerbi and Taisch Citation2020a) also externally to the company considering open loop supply chains as the industrial symbiosis scenario (Fraccascia and Murat Yazan Citation2018) but also in closed-loop supply chains (Gupta et al. Citation2019). Without a proper information flow for resources, products, and processes, the transition towards CM is severely limited (Bianchini, Rossi, and Pellegrini Citation2019), hindering resource loops (Gligoric et al. Citation2019). Digitalizaion is a great driver for backing the implementation of CM (Okorie et al. Citation2018), promoting a predictive view of manufacturing companies’ practices and supporting an informed decision-making process in CE (Kristoffersen et al. Citation2020). Therefore, manufacturing companies can take advantage of the introduction of different technologies in collecting appropriate data about the product, processes, and the managerial procedures established to engage relationships with the actors along the value chain and the actors external to it (Bianchini, Rossi, and Pellegrini Citation2019). A systematisation of the involved recourses and waste flows is required to effectively achieve circularity together with the related data and information (D’adamo and Sassanelli Citation2022). Indeed, mapping and structuring data and information for CM adoption represent one of the fundamental steps to enable the circular transition of manufacturing companies (Acerbi, Sassanelli, and Taisch Citation2022). Nevertheless, data models do not support a detailed and customised assessment of companies’ strengths and weaknesses. Instead, they can be made by relying on maturity models (MM) (Pöppelbuß and Röglinger Citation2011) that might represent the cornerstone to support companies in a circular transformation (Uhrenholt et al. Citation2022).
The extant literature presents different quantitative models using data and information to concretely calculate a specific performance, such as on effective material usage within industrial plants (e.g. Braglia et al. (Citation2018)), and on energy optimisation to evaluate emission reduction and cost savings (e.g. (Prashar Citation2020)). Nevertheless, the CE assessment field of research is still quite fragmented, and most of the assessment results are based on continuous variables; therefore, a holistic and quantitative assessment model is still missing (Vinante et al. Citation2021; Sassanelli, Rosa, et al. Citation2019). Moreover, as previously anticipated, MMs represent the starting point in this direction, enabling quantification within a maturity scale, a qualitative analysis performed on the current maturity state of a company in a comprehensive way (Macchi and Fumagalli Citation2013), allowing an introspective analysis (Wagire et al. Citation2020) and supporting informed decisions (De Bruin et al. Citation2005). In the CE domain, some MMs have been developed, such as the one by Bressanelli, Perona, and Saccani (Citation2021) that attempts to assess the readiness of companies to embrace CE. However, it does not employ a prescriptive approach or stimulate an improvement path based on data-driven decisions.
Despite the MM already present in the scientific literature, a lack of an MM able to guide organisations through a systemic approach towards fully embracing CM, taking advantage of data use, is registered. Therefore, the observed gap, described in Section 3.1 of this contribution, is that the existing models lack an overall approach and vision to support manufacturers to value data properly to embrace CM and widely generate this awareness internally for the company. To cover this gap, the research objective of this study is to understand, through the development of an MM, how to assess manufacturers’ data use and information to support the decision-making process in CM, starting with an analysis of their current state. This newly developed MM can contribute to both theory and practice. Regarding the theory, the research field on data-driven CM and assessment methods has been extended, creating a modular, adaptable MM for data-driven CM and a new methodology to develop MMs. The practice has been developed, enabling an MM to support manufacturing companies in performing an introspective analysis of their current state to make informed decisions on pursuing an advanced level of CM based on data.
To address this research objective, the following research question (RQ) was posed:
RQ: How are manufacturers’ data and information use assessed to support their decision-making process in CM?
2. Research design
To address the research objective of understanding, through the development of an MM, how to assess manufacturers’ use of data and information to support the decision-making process in CM, starting from the analysis of their current state (anticipated in the Introduction and further clarified and validated in Section 3.1.), the research design is depicted in . It is inspired by the design science research (DSR) methodology (Peffers et al. Citation2006) for the identification of the problem and the clarification of the objectives for the solution, together with the Design Research Methodology (DRM) (Blessing and Chakrabarti Citation2009) for the design and validation of the artefact addressing the problem identified. This research methodology has been accompanied by the steps specifically required to develop an MM (i.e. scope, design, populate, test, deploy and maintain) proposed by De Bruin et al. (Citation2005).
Four main steps were applied. First, thanks to the extant literature, the primary problem and the initial concept of the artefact required to address the problem were clarified. Specifically, the inputs for the model conceptualisation arose from two antecedent research contributions (i.e. Acerbi, Sassanelli, et al. Citation2021; Acerbi, Sassanelli, and Taisch Citation2022) which were extended with a deeper review of the already existing CM – MM. Once the concept was defined, the model was developed (through the modelling methodology), verified, and validated (through focus groups, interviews, and pilot applications). Finally, the model prototype, once revised based on the collected feedback, was applied to two other industrial cases (in the automotive and in the whitegoods sectors). This model aimed to contribute to practice and theory by embracing an approach similar to the one for action research by combining scientific literature and academic experts with practitioners, experts and industrial cases (Sassanelli, Pezzotta, et al. Citation2019).
2.1. Problem identification and model conceptualisation
The model conceptualisation was triggered by a necessity that emerged among practitioners (the need to value data for CM) and scholars (the lack of a holistic quantitative assessment model to analyse data and information management in CM, as also declared by Acerbi, Sassanelli, and Taisch (Citation2022), clarifying the ‘scope’ step (i.e. MM in the manufacturing domain with a CM data-driven perspective). Therefore, to ground the conceptualisation of the model in the extant literature, two key research contributions linking data and information with CM were taken as inputs, as previously anticipated. In addition, a state-of-the-art analysis of MMs in CM was conducted, reinforcing the potential of using the methodology proposed by De Bruin et al. (Citation2005). Specifically, Scopus was used as the primary scientific database, querying it with the following string: TITLE-ABS-KEY (‘maturity model*’ AND (‘circular economy’ OR ‘circular manufacturing’)).
Considering the limited number of contributions found based on that string (only 9 of 20 were consistent with the scope of this research), it has been chosen to also search for TITLE-ABS-KEY (‘maturity model*’ AND ‘manufacturing’) without including the terms CE and CM in the string. This choice extended the view of traditional and well-structured MMs for manufacturing companies. Moreover, since the proposed MM aims to assess manufacturers’ use of data and information to address CM, the data and information to be considered to adopt CM strategies were inspired by the systematic literature review conducted by Acerbi, Sassanelli, et al. (Citation2021). The dimensions and subdimensions of the model were inspired by Acerbi, Sassanelli, and Taisch (Citation2022), who developed a conceptual data model structuring the data and information for CM adoption. These two research contributions were used as further inputs for MM conceptualisation and development, considering them complementary to the literature review about MMs in this study.
2.2. Model development
Establishing the elements detected in the conceptualisation phase represents the input for the model development, allowingboth the design and the populate phases to be triggered. Regarding the design phase, it was necessary to ensure that the model addresses the audience’s needs in response to the questions about why the stakeholders are willing to implement the model, how the model is applied, who needs to be involved in the application, and what can be accomplished thanks to the application of the model:
Why: manufacturers must be supported in evaluating their current data use and information for CM to define possible areas of improvement based on informed decisions.
How: on-site, face-to-face interviews relied on a questionnaire based on normative answers.
Who: the interviewee profiles were defined as those managers with a broad perspective on their companies in terms of internal strategies devoted to sustainability and circularity and the general data exploitation for their business activities. Therefore, each company can select more than one manager for the interview according to their current organisational structure.
What: is creating awareness about the company’s status stimulating an improvement in exploiting data for CM?
Regarding the populate phase, it is necessary to clarify what is measured and how the measurement is performed (De Bruin et al. Citation2005), as follows:
What is measured is based on the scientific literature and is especially inspired by Acerbi, Sassanelli, et al. (Citation2021), who clarified, through the scientific literature and expert interviews, the classes of data to be considered to embrace CM driven by data. Additional clarification about the dimensions and subdimensions to be inserted in the MM was taken from Acerbi, Sassanelli, and Taisch (Citation2022).
How the assessment is conducted is reflected in the deployment of the questionnaire, allowing investigation of the current maturity of companies in using data for CM purposes through face-to-face interviews with managers of manufacturing companies. Therefore, based on the extant literature (see Sub-section 3.1), the questions and the related normative answers have been developed, enhancing the assessment’s objectivity. To conduct the assessment, the normative answers were not shared with the interviewees. Still, the interviewer used them to objectively classify the answers received during the interviews and to compute the maturity indexes in a standard way. Further details are reported in Section 4.
2.3. Model verification and validation
Model verification and validation are reflected in the ‘test’ phase (De Bruin et al. Citation2005). The first step was to verify the completeness and reliability of the model in evaluating how manufacturing companies use data for CM adoption. Focus group and expert interviews were conducted (Bryman and Bell Citation2007). An initial focus group was assembled, including eight practitioners from Portuguese, German, Italian, and Greek technology consultancy and software companies. In particular, group members were selected because they were experienced in data management and already knowledgeable about CM, thanks to previous consultancies and research projects on this topic. These people are part of the consortium of a European-funded research project that works in the CE domain. Its impact led to substantial heterogeneous results in industry and academia and to a second-phase European-funded project to extend the previous study results. This focus group was conducted to verify the defined maturity levels and the model’s content to enhance the results obtained from the scientific literature. Moreover, the focus group helped evaluate whether the model would be comprehensive, understandable, valuable, and applicable in the industrial domain (Saunders, Lewis, and Thornhill Citation2009). This choice also enabled verification to address audience needs.
Next, separate interviews were conducted with three scientific experts from Italian universities to verify the completeness of the questions and the coherence between the maturity levels and the normative answers; the goal was to check the reliability, completeness, and applicability of the model. The three experts selected have extensive expertise and are currently involved in projects dealing with a pairwise combination of CE, digital transformation and assessment models (specifically MMs). These experts were selected based on the goal of each single interview (that, hence, were separated). Thus, their expertise was the fundamental element in the selection process, and it needed to be complementary to the expertise and knowledge of the experts from the convenient sample of the European-funded research project.
The number of practitioners and scientific experts involved in the verification phase was unbalanced. However, this is justified by the willingness of the authors to ensure the embracement of companies’ needs in this specific phase, since in the existing models, the verification was mainly based on the scientific literature. From a practitioner’s perspective, the willingness was to ensure, and in case align, the model content and structure with the companies’ needs. Indeed, the involvement of practitioners would have covered this intention, and several viewpoints would have fostered the achievement of this goal. In addition, a final verification with academic experts was conducted to guarantee its value from a scientific perspective. However, as anticipated, the model was conceptualised and developed based on previous consolidated scientific knowledge.
In the second step, a pilot assessment was conducted with two manufacturing companies in northern Italy to validate the model. Operating in different industries, they ensured heterogeneity in the sample and represented the range of possible companies that could be assessed through the model. These companies are different not only in terms of the industry in which they operate, but also in size and interest in sustainable and circular-oriented projects. Thus, they constitute a representative sample of manufacturing companies that could apply this model. The first company (Campany A) was a supplier of metal components for the aviation, automotive, electronics and other sectors at the international level. It is a medium-sized company with about 80 employees. The second company (Company B), with approximately 100 employees, is a supplier in the cosmetics industry at the international level.
Both company A and B were constrained by rigid regulations determined by the industries and their customers. Both were interested in improving their sustainable performance by introducing CM strategies. The two companies were selected to ensure a heterogeneous sample and companies for which the model would be beneficial. In Company A, the assessment was conducted with the process analyst managing internal company data and having a broad overview of the entire company. In Company B, the company was less advanced in data use. Therefore, the managers of quality, research and development, marketing, and operations gathered all the information required for the assessment.
The normative answers to the questionnaire were not shared during the interview to allow the interviewees to reply extensively. After the interviews, the answers assigned according to their replies were shared with the interviewees. This review was required to check the consistency between their actual status assessed through the model, the answers given and their links with the questionnaire answers. This pilot assessment enabled the final refinement of the model to prepare it for actual deployment. Further details are presented in Sections 4.1–4.3. The overall process is summarised in .
Table 1. Verification and validation process.
2.4. Model application
Finally, the model application covers the deployment and maintenance phases proposed by De Bruin et al. (Citation2005). The model was applied in two additional manufacturing companies (i.e. Company C and Company D) to embrace CM operations in different industries and empower the results obtained from the MM adoption, especially regarding its generalisability (as described in Section 4.4). Indeed, as mentioned for the validation stage, in this case, the two selected companies differ in terms of industry, size, role in the supply chain, interest in sustainable and circular-oriented projects, and awareness to ensure that they cover a heterogeneous sample of companies. Company C operates in the metal sector, has about 400 employees, and produces metal components for tractors. Company D is a multinational company producing white goods, changing its strategy to move towards sustainability. In both cases, the interviews took place on site to cross-check the responses during the assessment with specific documents and visible actions on the shop floor. Additionally, interviews were conducted with managers of different functions and departments to ensure objective answers and a comprehensive overview of the entire company. Considering the fast-moving trend of both data usage and CM, the model will be maintained and improved throughout its deployment over the years.
3. Results
This section presents the results of the literature review related to MMs to conceptualise the data-driven CM - MM developed in this study (Sub-section 3.1) and the model development and current structure (Sub-section 3.2).
3.1. Model conceptualisation: a literature review of maturity models for circular manufacturing
The scientific literature widely expressed the potential of MMs to support organisations in evaluating (typically based on scores) how their business activities are performed, providing them with normative descriptions of good and best practices (Macchi and Fumagalli Citation2013). The MM comes from the capability maturity model (CMM) developed for the software industry in the early 1990s and promoted by the Software Engineering Institute (Paulk et al. Citation1993). Most of the MMs developed were inspired by the methodology proposed by De Bruin et al. (Citation2005) based on standard, structured and complete phases. These models are usually based on five maturity levels (Wagire et al. Citation2020). They can be descriptive (to assess the current state of a company and to perform a diagnosis of possible problems), prescriptive (to assess the current state of a company and to provide guidelines to improve and achieve a desired level of maturity), or comparative (to benchmark the maturity of a company against others with similar characteristics) (Pöppelbuß and Röglinger Citation2011).
MMs are also proposed as assessment models to improve sustainable or circular performance, creating awareness about the current state of an organisation. For example, from an organisational perspective, examining the entire value chain, but from a strategic point of view, Sacco et al. (Citation2021) proposed an assessment model integrating a set of CE indicators within a maturity scale. At the same time, Bertassini et al. (Citation2022) proposed a model assessing the readiness of the company culture before embracing CM, while Sehnem et al. (Citation2019) proposed a model assessing the maturity of circular business models in Brazil regarding resource recirculation. Pigosso, Rozenfeld, and McAloone (Citation2013) proposed a five-level MM for product eco-design. Acerbi, Järnefelt, et al. (Citation2021) proposed a five-level maturity scale for CM developed through interaction with industrial cases.
Moreover, different MMs have been proposed that focus on specific sectors. Gorecki (Citation2019) proposed an MM focused only on the construction industry, enabling the assessment of resource management maturity. Starting from the introduction of eco-design procedures, it is then possible to reach the optimum level of maturity as the one in which the processes allowing the resource restoration are inserted systemically. In addition, Dell’Ambrogio et al. (Citation2022) developed an MM focused on the automotive sector. As the MM descriptive, it does not provide guidelines on exploiting data to support the decision-making process in products along the value chain; it only supports mapping the company’s current activities by covering all the product life cycles. For agriculture, Uztürk and Büyüközkan (Citation2022) developed an MM that does not support a holistic view of data-driven CM; it only enables a description of the current state.
Other MMs have been developed with a focus on one of the TBL pillars, like that developed by Korne et al. (Citation2022), which aims to assess environmental sustainability without considering CE principles or how to use data to pursue a circular path. Moreover, an MM has been proposed to define the maturity levels to design an appropriate waste management strategy supported by I4.0 technologies in waste collection, analysis, and treatment without reviewing the entire resource lifecycle (Fatimah et al. Citation2020). Other MMs were developed to support implementing a specific CM strategy like the one for remanufacturing proposed by (Golinska‐Dawson, Werner‐Lewandowska, and Kosacka‐Olejnik Citation2021). Instead, Romero and Molina (Citation2014) proposed new industrial ecosystems based on re-designed sustainable processes and proposed an MM to assess companies’ strategies only from a socio-technical perspective and with wider sustainability lenses than CE.
Moreover, Sehnem et al. (Citation2019) developed a model based on assessing the CE business models adopted by companies but without reviewing the data needed to apply them. Martinsen et al. (Citation2021) focus on product characteristics to address circular requirements and necessary data exchange while neglecting the entire organisation’s perspective. An attempt to link digital transformation and CE adherence has been proposed by Zhang, Zhang, and Gu (Citation2023), who developed a model focused on digital transformation where CE represents only one of several dimensions to be considered and not which data needs to be used. Finally, with a synergic perspective on data and CE, Kristoffersen et al. (Citation2020) developed an MM for data exploitation for sustainable goals in which the maturity assessed was reflected in the ability of the company to manage data in general without looking at the type of data required.
Despite the MM already present in the scientific literature, there is a lack of an MM able to guide organisations through a systemic approach towards fully embracing CM, taking advantage of registered data use. Intending to cover this gap, this study aimed to understand, through the development of an MM, how to assess manufacturers’ data use and information to support the decision-making process in CM, starting from an analysis of their current state. The main dimensions of circularity should be discussed within a model to exploit data for CM; benchmarks for the optimum should be proposed to facilitate the transition, with clear objectives in companies’ strategic plans. Specifically, the transition towards CE, when driven by data, is characterised by the dimensions identified, for instance, by Zeiss (Citation2019) that considered the physical artefacts (e.g. products, components and materials), the actors (e.g. location, behaviour, etc.) and the activities (e.g. reuse, reduce, etc.) the key elements on which require structured decisions.
In addition, Gupta et al. (Citation2019) employed an organisational perspective emphasising the role of stakeholders within the supply chain in this context and the possibility of relying on information sharing to support the decision-making process. Therefore, these dimensions were further elaborated by Acerbi, Sassanelli, et al. (Citation2021) (i.e. product, process, management, and technologies) from a manufacturing company perspective. These data-driven dimensions were also discussed in detail by Acerbi, Sassanelli, and Taisch (Citation2022), who opened the perspective to several subdimensions characterising data-driven CM according to the extant literature and a panel of experts in the field. These dimensions and related subdimensions are summarised in and constitute the dimensions characterising the MM to be developed.
Figure 2. CM dimensions and related subdimensions inspired by the theoretical framework developed by Acerbi, Sassanelli, et al. (Citation2021) and Acerbi, Sassanelli, and Taisch (Citation2022).

In conclusion, the outputs of the MM conceptualisation phase developed in this study are as follows: the dimensions and the levels of maturity. First, the common base of previously-developed MMs inspired by De Bruin et al. (Citation2005) may guide the novel data-driven CM MM. Moreover, the analytical dimensions necessary to be defined in an MM will be based on the four primary dimensions of data and information to embrace the CM strategies proposed by Acerbi, Sassanelli, and Taisch (Citation2022). These dimensions are based on expert interviews and an elaboration of the results obtained from the review of the extant literature (e.g. regarding the product (Zeiss Citation2019) and (Kristoffersen et al. Citation2020), process (Bianchini, Rossi, and Pellegrini Citation2019), stakeholders considering those participating in an industrial symbiosis network (Fraccascia and Murat Yazan Citation2018) and also those operating within a circular supply chain (Lahane, Kant, and Shankar Citation2020; Gupta et al. Citation2019), and technology including both traditional information systems (Thoben and Wortmann Citation2013) and also Industry 4.0 related technologies (Rosa et al. Citation2020). The dimensions were chosen as the core elements when starting to collect, analyse, and share data to support the decision-making process of managers and operators in applying the proper CM strategies according to the company’s current state.
Second, five maturity levels will characterise this MM as diffused in the extant literature about MMs for CM and sustainability (e.g. Kristoffersen et al. Citation2020; Sehnem et al. Citation2019; Acerbi, Järnefelt, et al. Citation2021), and in accordance with the recently-published standards about CE (BS EN 8001 Citation2017). This choice was also improved and verified by experts’ feedback during model verification (see Sub-section 4.1). More precisely, the five maturity levels facilitate a structured and fluent description of the steps required to fully embrace CM based on data by ensuring a clear distinction among them.
3.2. Model development
3.2.1. Design: model structure
The model design is based on the model conceptualisation outputs. The MM aims to evaluate manufacturing companies’ maturity using data for the CM adoption covering the four main dimensions (product, process, management, and tools) (Acerbi, Sassanelli, et al. Citation2021) and based on five maturity levels. The definition of the maturity levels is grounded on the results obtained by performing a review of the extant literature about MM (e.g. De Bruin et al., Citation2005; Sehnem et al. Citation2019; Martinsen et al. Citation2021; Acerbi, Järnefelt, et al. Citation2021). Moreover, they were re-elaborated, starting from previous research and putting the basis to conceptualise such levels (Acerbi, Järnefelt, et al. Citation2021) through the feedback collected during both the focus group and the interviews during the model verification. Also, the analysis dimensions (described in ) come from the extant scientific literature, taking inspiration especially from Acerbi, Sassanelli, et al. (Citation2021). Each dimension is characterised by related subdimensions (see ) inspired by Acerbi, Sassanelli, and Taisch (Citation2022). This detailed classification allows the evaluation of the specific criticalities of the company, defining a roadmap to pursue a desired TO-BE scenario in using data for CM.
The higher the capability of a company to gather and use the data covering the four main dimensions, the higher the maturity level, since it would represent the capacity of the company to govern data over the most critical dimensions for CM. There are five maturity levels. Starting at Level 1, data are used only to encounter mandatory sustainable and circular regulations, and Level 2 emphasises the exploratory attitude of data use towards circularity. Level 3 suggests the piloting introduction of some CM strategies supported by data use, and Level 4 starts to position the company in relation to others to exchange data for CM. Finally, Level 5 represents the significant level of data used and shared systematically with external stakeholders. These five maturity levels, summarised in , allow manufacturers to be aware of the current state of their companies, evaluating a tailored improvement path based on the clarification of the optimum level achievable to fully embrace CM.
Table 2. Maturity level definitions.
The maturity of the organisation is summarised in an integrated and comprehensive way through an overall synthetic index (OSI) covering the four CM data-driven analysis dimensions whose description is below reported:
TECHNOLOGIES AND TOOLS: the backbone of the transition since it enables data collection, analysis, use and sharing to fully benefit from the CM adoption.
EXTERNAL STAKEHOLDER MANAGEMENT: the management of specific external stakeholders is seen as the opportunity to establish external relationships with them to fully benefit from CM adoption facilitating resources loops.
PROCESS: all the processes enabling the treatment of resources sustainably and circularly necessary to fully benefit from CM adoption. Not all processes need to be established internally in the company. However, based on correct data use and sharing, the company must ensure the correct treatment of the resources outside its boundaries within external companies.
PRODUCT: the core element on which to start thinking about CM adoption. It must be designed and treated to embrace circular values by changing the company’s core business if necessary. It might cover the product produced, its packaging, the waste generated, the product turned back to be treated, and the by-products.
Moreover, for each MM dimension, a specific index is defined, whose evaluation is based on the investigation of the related specific subdimensions shown in . Below, their definitions are provided, and their computation is reported in Sub-section 3.2.2.
Technology and Tools Maturity Index (TMI): allows the assessment of the company’s maturity in using technologies for gathering and using data in CM. This index is broken down into specific indicators regarding i) the type of tool (e.g. software, technology) adopted and ii) their purposes in data use in addressing specific decisions within the CM context.
External Stakeholders’ Management Index (ESMI): allows the assessment of the company’s maturity in collecting data from external sources to manage the organisation’s relationships with specific external actors. Specifically, three subdimensions (see ) and related indicators are based on the most relevant factors to be considered when making decisions for CM strategy adoption (i.e. customer [both B2B and B2C], supplier, industrial symbiosis partner). This ensures the detailed analysis of the possible relationships to be established and the required data to be shared, supporting the decisions for CM adoption.
ProCess Maturity Index (PCMI): allows the assessment of an organisation’s maturity in collecting data for a CM purpose about processes relevant to CM. Thus, nine subdimensions (see ) have been developed. The design, production, and delivery correspond to traditional manufacturing processes, while the others, like recycling, refer to the more oriented circular processes allowing the resource’s lifecycle to be extended at their end of life. This distinction provides the manufacturer with a clear idea about the advancements performed by the company regarding the traditional and well-established manufacturing processes, as well as to verify its maturity regarding the usage of data to support the decisions related to the introduction of CM processes (these can be based on data exchanged both internally and externally to the company to create contacts with external actors in charge of specific circular processes like recycling).
ProDuct Maturity Index (PDMI): allows the maturity level of the company to be assessed concerning data use to support decisions about the product produced, its packaging, the product turned back once sold, the waste and the by-products generated. Therefore, five subdimensions (see ) are present to compute this index by evaluating for each subdimension what data the company collects and uses and the purpose of collecting and using data.
These indexes are grouped in a unique dashboard based on histograms, as depicted in Section 4 (). This provides the manufacturing company under analysis with a graphical and comprehensive view of its maturity level.
3.2.2. Populate: model questionnaire
To perform the assessment, the MM must be deployed to manufacturing companies. This was performed through a structured questionnaire comprising 38 questions, with five normative answers each. The questionnaire structure enables a deep and objective investigation of the firm’s current state under analysis by evaluating many aspects. The questionnaire allows us to evaluate i) whether data are collected and used to support the decision-making process in CM, ii) the types of data collected and used, and iii) the current purpose of data collection and use. An example of a question with related normative answers is reported in .
The normative answers are only guidelines for structuring the interviews and classifying the score corresponding to each question, facilitating the definition of a maturity level covering each relevant dimension. The interviews were also supported by the analysis of the concrete actions done by the company to justify the answer given. For instance, looking at the subdimension ‘new product’ related question and normative answers (see ), if the company replies that they do not have projects or pilot initiatives based on CM, but they are collecting data about consumer preferences for green products to perform analyses and make decisions on new market opportunities and suppliers’ opportunities for new materials, it is possible to assign Level 2 to this question.
In the questionnaire, it is possible to compute in an objective and standard way the specific maturity indexes (i.e. TMI, ESMI, PCMI, PDMI) and the OSI evaluating the company in using data for CM decision-making process support (to facilitate the deployment, an Excel file is adopted). The evaluation was performed as follows:
SPECIFIC INDEXES EVALUATION (1): each question (‘QSDj’ in the formula, where j represents the single question and ‘m’ the number of questions related to a specific subdimension i) has a weight equal to 1. All the questions concurrently operate to estimate the specific score per subdimension (‘i’ in the formula), which is finally required to generate the score associated with the single dimension (‘Dim Index’ in the formula), where the k dimensions are 4. They are the following: 1) k = tools (i.e. Dim Indextool = TMI), 2) k = external stakeholder management (i.e. Dim Indexext.stakeholders = ESMI), 3) k = process (i.e. Dim Indexprocess = PCMI), 4) k = product (i.e. Dim Indexproduct = PDMI).
To further explain the quantification of the ‘Specific Index Evaluation’, it is worth mentioning that each normative answer is linked to a certain maturity level (from 1 to 5). Therefore, according to the choice made by the respondents, it is possible to assign a score to each question, which will be determined using the formula mentioned above (1), the maturity level of each dimension of this MM.
OVERALL SYNTHETIC EVALUATION (OSI) (2): once the scores of the four indexes corresponding to the four dimensions are estimated, the generic index is computed in the following formula:
The MM developed aims to be modular, giving both a synthetic and detailed overview of the company by tailoring the analysis to the company’s characteristics. For example, if a company operates only in B2C, the B2B question can be discarded without compromising the overall evaluation. Moreover, to ensure objectivity in the assessment without being biased by the author’s interpretation of the extant literature, it has been decided not to include weights to the single dimensions and subdimensions, posing all the weights equal to 1. Nevertheless, thanks to the modular structure of this MM, it is possible to choose for each company a different weight for the several dimensions and subdimensions to align their strategic objectives with the model outcomes. includes the primary characteristics of the MM (i.e. the artefact) developed:
Table 3. Artefact structure.
4. Discussion and model verification, validation, and application
It is essential to highlight how the progression in the MM could work for companies by how data management and decision-making are likely to change in nature as we progress through the model (either in each of its four dimensions or in total, depending on the lens of analysis the company wants to use) within the five levels. This can provide practical evidence of the results obtained in this research. Therefore, it is possible to define the characteristics of companies assessed across the model’s five levels using the MM. In addition, by using the MM and looking at its results, companies can raise their awareness about CM practices and be flanked in climbing the different levels towards full data-driven CM maturity. To unveil the differences in pursuing the 5-level path of data-driven CM maturity, the descriptions of the first, third, and fifth levels are given only for the product dimension. This highlights the progression in data management and decision-making as representative of the entire model. Due to space limitations, descriptions of the process and external stakeholders’ management dimensions are not included in this section. Instead, as technology is a cross-functional dimension of the model, a glimpse of the required technologies is provided.
Regarding the product dimension, at Level 1, data collected and analysed are those needed to monitor a linear business model (e.g. product value, functions, dimensions, geometry, innovation, perishability), neglecting the option of turned-back products (and therefore related data). In this case, no data are collected to monitor and analyse the industrial waste produced, by-products and packaging. At Level 3, data (e.g. perishability, modularity, repairability, material composition and biodegradability) are exploited to explore the circular benefits generated by the development at the level of a product family with embedded characteristics facilitating circular management. Maintaining the single product family perspective, the reverse flow might be established, requiring data gathering as the product damage level and product remaining useful life. Regarding product-related industrial waste, data (e.g. waste type, waste quantity, treatment method) are managed per product family to monitor the scraps generated during production to evaluate possible exchanges or internal reuse. The same is true for by-products and packaging, even though other data are needed to be collected, such as usability of the by-product and recyclability of the packaging.
At Level 5, data collection and analysis are performed to monitor the circular benefits generated by the production of all the products produced by the company. Data sharing is systematically established with the local community, consumers, and suppliers to improve product circular characteristics. Indeed, a reverse flow is established for all the products the company delivers, requiring systematic data management (e.g. amount of the return, distances among industrial partners) and the engagement of different external actors. Concerning industrial waste and by-products, data are systematically managed on all the product families to monitor the scraps generated during the production processes and to evaluate possible exchanges or internal reuse of these scraps and by-products. Finally, the packaging (both primary and secondary) data of all the product families are managed to ensure the correct adoption of circularity and sustainability for the whole company.
Concerning the technology dimension, going from Level 1 to 5 of the MM, the four main categories (i.e. authoring tools, technologies for sustainability, Information systems and Industry 4.0) can be either not adopted or adopted at different levels in the company’s daily operations. For example, Level 1 is described. Technologies in some cases are not implemented, in other cases they implemented to be adopted to sustain the linear dynamic of flows occurring in the company. For instance, an authoring tool could be adopted only to collect data required for the traditional activities of the linear business model (to improve the designers’ productivity, design quality and communication). Technologies for sustainability (such as smart bins, and sorting technologies or disassembly technologies) are not present in plants. The same is applied to the adoption of information systems such as Product Data Management (PDM), Product Lifecycle Management (PLM), Eco-database for products and Lifecycle Inventory Database, Customer Relationship Management (CRM), Manufacturing Execution System (MES), Enterprise Resource Planning (ERP), Material Requirement Planning (MRP), Environmental Management System, and Industry 4.0 technologies (i.e. decision support tools based on data analytics, cyber-physical systems, IoT, tracking technologies, Big Data analytics, smart products, cloud manufacturing, additive manufacturing, artificial intelligence and simulation tools).
4.1. Verification step 1: focus group with practitioners
During the focus groups, some valuable comments arose (summarised in ). According to six participants, data collection represented the core aspect of CM according to their past projects; the questions characterising the questionnaire were understandable and valuable for the goal of the model. All participants confirmed the potential of developing a modular model to make it more reliable. Moreover, concerning the model deployment, five participants suggested conducting an interview without sharing the normative answers, stressing the need to have an intermediary during the assessment, thus limiting the subjectivity of the analysis.
Table 4. Focus group for model improvement and first verification.
4.2. Verification step 2: scientific expert’s interviews
The experts interviewed were asked to review all questions and related normative answers. provides the specific interview goals and related model improvements derived from the interviews.
Table 5. Academic expert interviews.
4.3. Validation: pilot industrial applications
Two pilot assessments were conducted for the final model validation.
4.3.1. Company A
The assessment of Company A reported an OSI equal to 3.4, highlighting a good level of data use for CM adoption, positioning the company at the third level of maturity: ‘DATA FOR PILOTING CIRCULARITY’. From a technological viewpoint, the maturity level assessed was relatively low (TMI = 2.75) because they addressed a digital transformation. At the same time, for the other dimensions, the results were excellent (ESMI = 4, PDMI = 3.75, PCMI = 3.11). Looking at PDMI, even though it is still driven mainly by economic purposes, it already controls the quantity and type of industrial scraps generated to sell the waste to external suppliers, which can treat scraps to create secondary materials and give them back as new inputs.
Therefore, as reflected in the results obtained in the PCMI and ESMI indexes, they monitor the waste flow for specific types of products and have already established partnerships with external actors (thus, they decide which entity provides a specific type of waste, considering its intrinsic characteristics). For certain types of products, they monitor data enabling the recycling process performed by a third party, even though they are not fully aware of the positive environmental outcomes from this type of monitoring. They are still defining the data they need to gather this information (e.g. transportation means to evaluate the environmental impacts, the emissions during the recycling process, the secondary material recovered from the recycling process, etc.). Moreover, in the case of defective products, they remanufacture them to enable selling after having collected and analysed data about the state of the product and the possibility of remanufacturing it.
Generally, even though this was a pilot assessment, the company was able to identify several opportunities for improvements, including the establishment of a structured process for waste management by keeping under control all the data concerning the social and environmental impacts to make beneficial and not detrimental decisions. A simulation model was implemented to evaluate the best path for each type of waste generated based on the data already collected. This tool would facilitate decision-makers in deciding how to treat every type of waste. Moreover, regarding B2B relationships, further data were identified as necessary to be shared with these entities to ensure the extension of the product lifecycle. Finally, considering the still limited attention on circular performances from social and environmental perspectives, they were suggested to start tracking the emissions from the assets and the transport between them and their suppliers, to start selecting the suppliers not only according to their capability of selling secondary materials but also by looking at the environmental-friendly certifications and distance.
An outcome of this pilot assessment was that the assessed indexes were constistent with the interviewee’s expectations, and the normative answers were shown to check this alignment. Some suggestions to improve the model were given. It was hinted to add, in the ‘product’ dimension, the ‘packaging’ as a new subdimension, since it was a relevant dimension to make adherent decisions for CM but not assessed in the initial version of the questionnaire. Indeed, for this company, packaging represents the first element for which they started reasoning on how to become circular. In addition, the establishment of recycling and remanufacturing processes, in this case, is mainly focused on retaining the value of resources. At the same time, the product is produced, and the questionnaire’s initial structure did not consider at which stage of the product lifecycle these processes are established. Indeed, the normative answers were revised to include this aspect in the assessment.
4.3.2. Company B
The assessment of Company B yielded an OSI of 2, positioning the company at the second level of maturity: ‘DATA FOR EXPLORING CIRCULARITY’. Therefore, the company has an exploratory approach focusing its attention especially on a specific product family. Digging into further details, the results obtained for the specific indexes are as follows: TMI = 1.7; ESMI = 1.3; PCMI = 2; PDMI = 2.75. Due to space constraints, only primary outcomes are reported.
The results obtained were aligned with the company expectations, and the analysis performed was considered by the interviewees as a comprehensive radiography of their current data use, enabling the proper internal awareness about data exploitation for CM, paving the way for further improvements. Several options to exploit their data were identified, such as the possibility of performing the Life Cycle Assessment (LCA) on different product families to control environmental impacts. Moreover, the most critical element to manage was the ‘waste’, now disposed of by paying vast amounts of money and doing nothing to exploit its value. As a result, they envisaged analysing the existing platforms to exchange resources to select the most coherent partner consistent with their sector and the company’s needs.
A key suggestion for model improvement was to consider the importance of customer management in CM. They underlined the importance of adding to the questionnaire a question about using the CRM as an additional information system to be explored from a CM perspective.
4.4. Model application: managerial implications
The model has been deployed to two other industrial cases: COMPANY C and COMPANY D. Due to space constraints, only a summary of the analysis is included in this paper. For the sake of transparency, it is worth mentioning that both assessments were conducted with the managers of different functions and departments: design and engineering, operations, production, quality, supply chain and logistics. The responsible person or manager (when present) of sustainable-oriented projects was always present.
4.4.1. Company C
The quantitative results obtained from the interview are shown in , delineating an OSI of 2.2 and positioning the company at an exploratory level.
Beginning with the TMI (a), the low result corresponds to limited company digitalisation. It is now investing in sustainability technologies, such as solar panels and sensors to monitor emissions, and energy consumption in all its industrial plants. In addition, they are investing in technologies for data tracking and analytics, especially on their industrial assets, to monitor resource consumption and optimise their production activities based on objective data supporting decisions. ICT-related investments are still few (only some efforts to integrate ERP and MES to monitor industrial asset data). There are also limited investments in technologies to restore damaged products (e.g. recycling and remanufacturing technologies). Regarding the ESMI (b), there is not yet a commitment to finding specific suppliers covering sustainable and circular aspects or identifying possible industrial players with whom to exchange resources.
Nevertheless, regarding the product dimension, the PDMI index (c), an initial interest in investigating the by-products generated to find new opportunities to exchange them with external entities, is perceived. At the same time, for a specific product family, the waste generated (in terms of type and quantity), the resource consumption, and emissions generation, especially at the beginning of the product lifecycle, were analysed to evaluate whether some design-related improvements could be made. In addition, no efforts have yet been made to enable the turnback of products through a reverse logistic network. This absence is also visible in the average value of the PCMI index (d) caused by the absence of processes beneficial for the regeneration of resources (i.e. recycling and reuse) and the still limited interest in designing the products regarding their lifecycle extension. The value of the index is compensated for by the maintenance activities that are performed once the products are sold and by the possibility of remanufacturing and disassembling the products quite quickly during the production processes in case of defectiveness.
To move towards a more circular-oriented approach, within an operative roadmap towards CM, it was suggested that the company start collecting data on product design about all the product families with a long-term view to support the decision-makers in exploring opportunities for a new design. In this way, it would be possible to start inserting in the product design the potential for recirculating products (e.g. ensuring the disassembling of product components) without limiting the attention only to waste management at the end of the product life cycle and maintenance activities. In addition, as a result of the assessment, an analysis of possible partners to recycle materials, at least during production activities, will be performed. This would enable the company to establish strong relationships with external entities to recycle part of the industrial waste by collecting and sharing data about materials and specific resources, establishing industrial symbiosis networks.
4.4.2. Company D
The quantitative results obtained from the Company D interviews are shown in , delineating an OSI of 2.80, positioning the company towards a piloting level.
Company D is already operating to enhance its circular and sustainable performance, relying on data collection and usage. Starting from the tools adopted to gather and use data, the TMI index shows that (a) the company’s current position is quite advanced, since it has invested a lot in this direction. For example, sensors monitor resource consumption, emissions generation and waste creation in several plants.
Looking at ESMI (b), structured partnerships have been established with an external actor to ensure waste recycling and reuse of the secondary material generated in their products. They are now exploring potential partners who sell their waste and potential by-products when not required in their production activities by collecting data about waste and by-product characteristics. Based on this, they select the proper data to exchange and collect.
For the PDMI (c), the Company D are starting to design products to enhance the service level (e.g. by collecting data about consumers’ behaviours to define which services are needed for consumers) and to optimise the assembling (e.g. define the assembly procedure to be undertaken to limit the efforts). They are also exploring opportunities to optimise disassembling to facilitate the product lifecycle extension. Nevertheless, until now, there has not yet been a reverse logistic network, but they systematically manage the turnback of products for maintenance activities, usually based on the substitution of parts. There is no intention to regenerate resources at the end of their lifecycle but only during their production activities (both resource recycling and product remanufacturing are performed). Limited attention is devoted to enhancing the circularity of packaging, even though some exploring initiatives have been performed to meet the European requirements.
Finally, the PCMI (d) is relatively high since, consistent with the PDMI results. They are already entirely oriented to introducing circular-oriented processes by ensuring maintenance activities and trying to remanufacture and recycle those resources that might be discarded during production activities.
Based on these observations of the weaknesses and strengths derived of this assessment, some suggestions for improvement have been provided. Among them, data collected via smart products should be enlarged to empower the design function in improving the design and facilitating the product lifecycle extension through repairments or regenerative processes. In addition, collecting data during product usage would enable us to anticipate problems that may rise in the middle of life in the next product generation. Moreover, it has been suggested that the company evaluate possible initiatives to increase the reverse flow of resources, which is currently limited to maintenance, often leading simply to the substitution of the component without considering its regeneration.
5. Conclusions and limitations
The novelty of the present contribution is the development of an MM to support manufacturers in using data to make more informed decisions regarding CM adoption by addressing the following RQ:
How are the manufacturers’ data and information use assessed to support their decision-making process in CM?’
The model structure is primarily based on the scientific literature on MM and data-driven CM. Although the diffusion in the literature of MM is vast, limited attention has been given to those regarding CM and less to data exploitation in CM. Therefore, considering the importance of data for a structured transition and the need to create this awareness in manufacturing companies, the proposed model is novel with respect to previous models and covers both scientific and practitioner needs. This was emphasised during the focus group, interviews and application cases conducted to verify, improve, validate and deploy the developed MM. Moreover, the spectrum of the four companies involved in the pilot application and the actual deployment enhanced the model’s generalisability.
5.1. Contributions to theory
From a theoretical point of view, this study has defined how to assess data use by manufacturing companies in CM adoption by developing and validating an MM based on the current literature on the envisioned gap. Despite the existing MM proposed in the literature focused on a specific CM strategy or a single sustainable pillar, the proposed MM offers a systemic approach to guide organisations towards embracing CM exploiting data. The MM research domain has been extended towards the data management field for CM. This study’s contributions to the MM field are twofold concerning the methodology for MM development and the content. First, the methodology for developing the new MM was proposed by De Bruin et al. (Citation2005). Nevertheless, this study extended the MM development methodology to integrate the data management research field. With a focus on data exploitation for CM adoption, the MM was developed starting from a CM-related conceptual data model, making the data classes required to support decision-makers in adopting CM strategies visible. Second, the developed model, especially concerning the existing MMs for CM, is modular and adaptable to the company’s characteristics and focuses on the core CM dimensions characterising a manufacturing company from an operating perspective.
This expands the more strategic and organisational perspectives usually employed and makes visible the opportunities in using data to support decision-makers in this context. The developed MM offers manufacturers the knowledge and awareness about the current state of their company in valuing data to support the decision-making process in adopting CM strategies and thus to evaluate the main criticalities and possible rooms for improvement based on structured and levelled operative steps.
The questionnaire developed for this MM has normative answers, which provide a set of guidelines to be followed to implement CM driven by data. The normative answers represent the knowledge to define the practices to be adopted and the related decisions to be made by a manufacturing company moving towards CM. Additionally, the indexes developed as one of the model’s main outputs provide a quantitative measurement evaluating the manufacturers’ current state. In the long run, once several applications are performed in the industrial domain, the model will empower the knowledge about the specific companies based on their characteristics (e.g. size and industries). Based on the outputs of this research, Sub-section 5.3 discusses future research opportunities articulated across different research streams (e.g. CE and sustainability relationship, sustainable indicators across circular maturity levels, etc.).
5.2. Contributions to practice and managerial implications
From a practical point of view, the proposed model allows classification and guidance of organisations along five maturity levels covering the four analysis dimensions (product, process, management and tools). Beginning from the lowest level in which data are collected only to apply the mandatory regulations for sustainability (e.g. mainly economic-driven strategies) in the organisation, it is possible to reach the highest level according to which the company can systematically exploit internal and external data to make proper decisions to create circular products, invest with a circular aim in technologies, establish internal processes and external relationships with other actors, allowing creation of a flexible and structured network to fully benefit from CM adoption. For example, the application of this MM enabled Company D to consider how to collect data on consumer behaviours to improve maintenance and repair services. The collected data enable them to extend their product lifecycle without cannibalising their core business, revise the design of some products, and establish a logistic network, enabling the reverse flow of resources.
These projects have started to be implemented by exploiting already available but dispersed data. Therefore, thanks to this study, companies can start thinking about what data they are already collecting and how to use the data available for CM-oriented purposes. These projects would enable the company to use data systematically towards the full embracement of CM, which would require additional collaborations and interactions with external stakeholders in the future. Moreover, with respect to the existing MMs, the proposed model is modular and adaptable to the company’s characteristics and focused on the core dimensions characterising a manufacturing company with an operating perspective, expanding the more strategic and organisational ones usually employed. Therefore, the proposed model offers manufacturers knowledge and awareness about the current state of their company in valuing data to support their decision-making process in adopting CM strategies and thus to evaluate the primary criticalities and possible room for improvement based on structured and levelled operative steps.
A primary managerial implication of the model is to clarify the company’s current state to define a customised and structured improvement path to use data in a transition to CM. Manufacturers relying on this model can benchmark, based on the indexes, their company’s current position with respect to the optimum desired TO-BE scenario for an informed strategic plan towards data-driven CM. In the long run, the empirical application of the MM is expected to be extended to a broader sample of manufacturing companies, supporting the managers in directing investments in a tailored way according to the primary critical points with room for improvement. Therefore, once several companies with similar characteristics have adopted the model, the benchmark among them could become feasible and valuable to strengthen the improvement path consistent with comparable companies’ current positioning, extending the model with a comparative purpose.Moreover, this MM could pave the way to the correct employment of Digital Product Passport (DPP). DPP is starting to become mandatory for certain types of products (e.g. vehicles batteries) and it is expanding towards other industries such as the textile and apparel ones.
5.3. Limitations and future research opportunities
The limited application of the proposed MM might hinder the model’s generalisation. Indeed, in the future, the model should be extensively applied to tailor the guidelines for improvement according to companies’ characteristics and to iteratively improve and update the model. In this regard, in future research, following the deployment of the MM to several industries, it will be possible to conduct a longitudinal analysis enabling the observation of the key improvements and achievements obtained by the projects put in practice after the assessment.
Another limitation of the MM might be the type of assessment conducted, which is based primarily on qualitative interviews. The quantitative scores only reflect the maturity level; no other key performance indicator (KPI) was calculated. Therefore, in future research, the model could be extended through integration with standard and well-diffused quantitative indexes measuring resource consumption to directly monitor the benefits derived from improvement actions in a unique model. In this regard, the sustainability achievements, covering the three pillars of sustainability (i.e. economic, environmental and social), could be easily mapped across the maturity levels, reinforcing the quantitative results obtainable from the assessment. Today, some standard assessments, such as the life cycle assessment ISO14000, are already available to companies and might be easily integrated with the proposed MM to assess and compare the AS-IS and TO-BE scenarios. In addition, other quantitative indicators have been proposed in the literature. Thus, this MM could become the structured collector of quantitative existing and new indicators, providing a quantitative overview to the managers and facilitating their understanding of the benefits of their circular-oriented choices.
Additionally, few considerations about information management theory are provided. Indeed, the theory proposed in this manuscript can contribute to this research domain, and future research can refer directly to these results. Applying the proposed MM can potentially compel companies to adopt practices for enhancing data management dynamics useful for gathering, analysing and exploiting data effectively. Based on these data, new circular-related KPIs can be defined and employed to support the pursuit of CM roadmaps. Therefore, based on the proposed MM, relevant KPIs, not yet available in the extant literature but needed by the practice to make proper circular-oriented choices and consider environmentally sustainable outcomes and economic and social benefits, can be developed. These new KPIs can be integrated into a broader framework to ease companies’ pursuit of CM roadmaps, in which the MM can be embedded as a critical component.
Finally, because the Italian and European governments in particular are establishing the conditions to allow a circular transition of society, the proposed model might represent an opportunity to have a broad view of nations to make more informed decisions from a firm-level and national perspective to direct future policies and incentives, being aware of the current maturity of companies and their needs. Moreover, in future research, it may be necessary to extend the model to help manufacturing companies address the needed country-based regulations, starting from the companies’ current state, by examining the human resources and assessing the critical competencies needed. In this regards, further elaboration about how to integrate DPP requests into the model could be perfomed to keep the model always updated in accordance with the norms.
Disclosure statement
No potential conflict of interest was reported by the author(s).
Additional information
Funding
Notes on contributors

Federica Acerbi
Federica Acerbi (female) is an Assistant Professor in the Manufacturing Group of Politecnico di Milano (Italy) at the Department of Management, Economics and Industrial Engineering. She developed her PhD research at Politecnico di Milano in both academic and industrial contexts with attention towards sustainable operations management in manufacturing. Her research focuses on data and information valorisation enabling the embracement of circular economy strategies and principles by manufacturing companies. She is involved in European and national research projects. She is also involved in industrial consultancy projects facilitating the digital transformation of manufacturing companies. She is lecturer and tutor in two master courses at Politecnico di Milano: Sustainable Manufacturing and Operations Management. Moreover, she is lecturer in a Bachelor course “Impianti Industriali e Gestione della Produzione” at Politecnico di Milano. She is member of AIDI and IFIP W.G. 5.7 scientific communities. Within the IFIP W.G. 5.7. community, she is co-leader of the Special Interest Group on Eco-efficient and Circular Industrial Systems.

Claudio Sassanelli
Claudio Sassanelli (male) is tenure-track Professor at Politecnico di Bari, Department of Mechanics, Mathematics and Management and Senior Research Fellow at École des Ponts Business School of École des Ponts ParisTech. He received his two master degrees in Management and Civil Engineering from Politecnico di Bari, respectively in 2010 and 2013, and his PhD in Management, Economics and Industrial Engineering from Politecnico di Milano in 2017, also holding visiting researcher positions at Tokyo Metropolitan University (TMU) and Universidade de São Paulo (USP). His main research interest is Product-Service System (PSS) design, specifically addressing to Product Lifecycle Management (PLM), Design for X (DfX) approaches, and Circular Economy and Industry 4.0 paradigms. To advance these research domains, he manages special issues in international journals as guest editor, and disseminates his research being co-author of 95 publications in international journals, international conferences proceedings and book chapters in the field. He is member of the IFIP WG 5.1 and of the editorial board of the journals Sustainability MDPI, Frontiers in Sustainability, Frontiers in Environmental Science, and Academia Engineering. He has a 10-year experience in research, industrial consultancy, and European research and innovation action projects. He carries out teaching activities in courses on production systems (environmental management, design and management, industrial technologies), and quality design and management, at Politecnico di Bari, Politecnico di Milano, SUPSI and LIUC Università Cattaneo.

Marco Taisch
Marco Taisch (male) is Full Professor at Politecnico di Milano, where he teaches Sustainable and Digital Manufacturing, and Operations Management. He is one of the coordinators of the Manufacturing Group of the School of Management of Politecnico di Milano. During his academic career, he has been the Rector’s delegate for Placement and Alumni, Director of the MBA and Executive MBA of MIP, the Business School of Politecnico di Milano. Since 2002, he has been particularly dedicated to the study of technological trends, carrying out some technological roadmaps and Technology Foresight studies on manufacturing as a consultant for the European Commission. He is President of MADE – Competence Centre Industria 4.0, the largest among the 8 Italian Competence Centres created by the Industry 4.0 National Plan. He is President of MIICS – Made in Italy Circolare e Sostenibile, the Extended Partnership – PE 11 created in the Italian Recovery and Resilience National Plan. He is co-founder and Scientific Chairman of the World Manufacturing Foundation, which yearly organises the World Manufacturing Forum, the global event that defines the Manufacturing agenda and which is endorsed by the European Commission. He is Vice-president of EFFRA (European Factories of the Future Research Association), member of the Board of Cluster Italiano Fabbrica Intelligente and of member of the Cluster Lombardo Fabbrica Intelligente. He is currently a member of the Unicredit Territorial Advisory Board for the Lombardy Region. He currently works as a consultant for different companies such as Leonardo or Thales Alenia Space; for these companies he has developed, together with a team, projects aimed to improve digitalisation and production processes.
References
- Acerbi, Federica, Vafa Järnefelt, Jorge Tiago Martins, Leila Saari, Katri Valkokari, Marco Taisch, Edited, Dolgui, A, et al. 2021. “Developing a Qualitative Maturity Scale for Circularity in Manufacturing.” Edited by A. Dolgui, A. Bernard, D. Lemoine, G. von Cieminski, and D. Romero. Milan, Italy: Springer Science and Business Media Deutschland GmbH. https://doi.org/10.1007/978-3-030-85906-0_42
- Acerbi, Federica, Claudio Sassanelli, and Marco Taisch. 2022. “A Conceptual Data Model Promoting Data-Driven Circular Manufacturing.” Operations Management Research 15(3–4): 838–857. https://doi.org/10.1007/s12063-022-00271-x
- Acerbi, Federica, Claudio Sassanelli, Sergio Terzi, and Marco Taisch. 2021. “A Systematic Literature Review on Data and Information Required for Circular Manufacturing Strategies Adoption.” Sustainability 13 (4): 2047. https://doi.org/10.3390/su13042047
- Acerbi, Federica, and Marco Taisch. 2020a. “Information Flows Supporting Circular Economy Adoption in the Manufacturing Sector.” In IFIP International Conference on Advances in Production Management Systems 2020, edited by D. Romero, B. Lalic, V. Majstorovic, U. Marjanovic, and G. von Cieminski, 703–710. Cham: Springer. https://doi.org/10.1007/978-3-030-57997-5_81
- Acerbi, Federica, and Marco Taisch. 2020b. “A Literature Review on Circular Economy Adoption in the Manufacturing Sector.” Journal of Cleaner Production 273 : 123086. https://doi.org/10.1016/j.jclepro.2020.123086
- Bertassini, Ana Carolina, Lucas Daniel Del Rosso Calache, Luiz César Ribeiro Carpinetti, Aldo Roberto Ometto, and Mateus Cecilio Gerolamo. 2022. “CE-Oriented Culture Readiness: An Assessment Approach Based on Maturity Models and Fuzzy Set Theories.” Sustainable Production and Consumption 31 (May): 615–629. https://doi.org/10.1016/j.spc.2022.03.018
- Bianchini, Augusto, Jessica Rossi, and Marco Pellegrini. 2019. “Overcoming the Main Barriers of Circular Economy Implementation Through a New Visualization Tool for Circular Business Models.” Sustainability 11 (23): 6614. https://doi.org/10.3390/su11236614
- Blessing, Lucienne, and Amaresh Chakrabarti. 2009. DRM, a Design Research Methodology. London: Springer. https://doi.org/10.1007/978-1-84882-587-1
- Bocken, Nancy, Karen Miller, and Steve Evans. 2016. “Assessing the Environmental Impact of New Circular Business Models.” “New Business Models” - Exploring a Changing View on Organizing Value Creation – Toulouse, France, 16–17June 2016.
- Braglia, M., D. Castellano, M. Frosolini, and M. Gallo. 2018. “Overall Material Usage Effectiveness (OME): a Structured Indicator to Measure the Effective Material Usage Within Manufacturing Processes.” Production Planning & Control 29 (2): 143–157. https://doi.org/10.1080/09537287.2017.1395920
- Bressanelli, G., M. Perona, and N. Saccani. 2021. “Assessing the Readiness of Manufacturing Companies for the Circular Economy: An Analysis and an Initial Proposal.” In Proceedings of the Summer School Francesco Turco, AIDI—Italian Association of Industrial Operations Professors, Virtual, 8–10 September 2021; Volume 7, 343–354.
- Bryman, Alan, and Emma Bell. 2007. Business Research Methods. Social Research.
- BS EN 8001. 2017. Framework for Implementing the Principles of the Circular Economy in Organizations – Guide. United Kingdom: BSI Standards Publication.
- D’adamo, Idiano, and Claudio Sassanelli. 2022. “Biomethane Community: A Research Agenda towards Sustainability.” Sustainability14 (8): 4735. https://doi.org/10.3390/su14084735
- De Bruin, Tonia, Ron Freeze, Uday Kulkarni, and Michael Rosemann. 2005. “Understanding the Main Phases of Developing a Maturity Assessment Model.” ACIS 2005 Proceedings, December.
- Dell’Ambrogio, Siro, Luca Canetta, Silvia Menato, Marzio Sorlini, and Jennifer Nika. 2022. “Design of Circular Economy Enhancing Journeys for Automotive Manufacturing Industry.” IEEE 28th International Conference on Engineering, Technology and Innovation (ICE/ITMC) & 31st International Association for Management of Technology (IAMOT) Joint Conference, 19–23 June 2022, Nancy, France.
- Fatimah, Yun Arifatul, Kannan Govindan, Rochiyati Murniningsih, and Agus Setiawan. 2020. “Industry 4.0 Based Sustainable Circular Economy Approach for Smart Waste Management System to Achieve Sustainable Development Goals: A Case Study of Indonesia.” Journal of Cleaner Production 269 (October): 122263. https://doi.org/10.1016/j.jclepro.2020.122263
- Fraccascia, Luca, and Devrim Murat Yazan. 2018. “The Role of Online Information-Sharing Platforms on the Performance of Industrial Symbiosis Networks.” Resources, Conservation and Recycling 136 (December 2017): 473–485. https://doi.org/10.1016/j.resconrec.2018.03.009
- Garcia-Muiña, Fernando, Rocío González-Sánchez, Anna Ferrari, and Davide Settembre-Blundo. 2018. “The Paradigms of Industry 4.0 and Circular Economy as Enabling Drivers for the Competitiveness of Businesses and Territories: The Case of an Italian Ceramic Tiles Manufacturing Company.” Social Sciences 7 (12): 255. Journalhttps://doi.org/10.3390/socsci7120255
- Gligoric, Nenad, Srdjan Krco, Liisa Hakola, Kaisa Vehmas, Suparna De, Klaus Moessner, Kristoffer Jansson, Ingmar Polenz, and Rob Van Kranenburg. 2019. “Smarttags: IoT Product Passport for Circular Economy Based on Printed Sensors and Unique Item-Level Identifiers.” Sensors (Basel, Switzerland) 19 (3): 586. https://doi.org/10.3390/s19030586
- Golinska‐dawson, Paulina, Karolina Werner‐lewandowska, and Monika Kosacka‐olejnik. 2021. “Responsible Resource Management in Remanufactur-Ing—Framework for Qualitative Assessment in Small and Medium‐Sized Enterprises.” Resources 10 (2): 19. https://doi.org/10.3390/resources10020019
- Gorecki, Jaroslaw. 2019. “Circular Economy Maturity in Construction Companies.” IOP Conference Series: Materials Science and Engineering 471: 112090. https://doi.org/10.1088/1757-899X/471/11/112090
- Gupta, Shivam, Haozhe Chen, Benjamin T. Hazen, Sarabjot Kaur, and Ernesto D. R. Santibañez Gonzalez. 2019. “Circular Economy and Big Data Analytics: A Stakeholder Perspective.” Technological Forecasting and Social Change 144 (July): 466–474. https://doi.org/10.1016/j.techfore.2018.06.030
- Korne, Thomas, Christian Köhler, Philipp Ewald, and Doreen Freyler. 2022. “Maturity Models for Environmental Sustainability Assessment of Applicability to Manufacturing SME.” Zeitschrift für wirtschaftlichen Fabrikbetrieb 117 (9): 537–542. https://doi.org/10.1515/zwf-2022-1105
- Kravchenko, Mariia, Tim C. McAloone, and Daniela C. A. Pigosso. 2020. “To What Extent Do Circular Economy Indicators Capture Sustainability?” Procedia CIRP 90: 31–36. https://doi.org/10.1016/j.procir.2020.02.118
- Kristoffersen, Eivind, Fenna Blomsma, Patrick Mikalef, and Jingyue Li. 2020. “The Smart Circular Economy: A Digital-Enabled Circular Strategies Framework for Manufacturing Companies.” Journal of Business Research 120 (November): 241–261. https://doi.org/10.1016/j.jbusres.2020.07.044
- Lahane, Swapnil, Ravi Kant, and Ravi Shankar. 2020. “Circular Supply Chain Management: A State-of-Art Review and Future Opportunities.” Journal of Cleaner Production 258: 120859. https://doi.org/10.1016/j.jclepro.2020.120859
- Macchi, Marco, and Luca Fumagalli. 2013. “A Maintenance Maturity Assessment Method for the Manufacturing Industry.” Journal of Quality in Maintenance Engineering 19 (3): 295–315. https://doi.org/10.1108/JQME-05-2013-0027
- Martinsen, Kristian, Carla Susana A. Assuad, Tomomi Kito, Matsumoto, Venkata Reddy, and Sverre Guldbrandsen-Dahl. 2021. Mitsutaka “Closed Loop Tolerance Engineering Modelling and Maturity Assessment in a Circular Economy Perspective.” In Sustainable Production, Life Cycle Engineering and Management, 297–308. Singapore: Springer. https://doi.org/10.1007/978-981-15-6779-7_21
- Mulrow, John S., Sybil Derrible, Weslynne S. Ashton, and Shauhrat S. Chopra. 2017. “Industrial Symbiosis at the Facility Scale.” Journal of Industrial Ecology 21 (3): 559–571. https://doi.org/10.1111/jiec.12592
- Okorie, Okechukwu, Konstantinos Salonitis, Fiona Charnley, Mariale Moreno, Christopher Turner, and Ashutosh Tiwari. 2018. “Digitisation and the Circular Economy: A Review of Current Research and Future Trends.” Energies 11 (11): 3009. https://doi.org/10.3390/en11113009
- Paulk, M. C., B. Curtis, M. B. Chrissis, and C. V. Weber. 1993. “Capability Maturity Model.” IEEE Software 10 (4): 18–27. https://doi.org/10.1109/52.219617
- Peffers, Ken., Tuunanen, Tuure Gengler, Charles E. Rossi, Matti Hui, Wendy Virtanen, and Ville; Bragge, Johanna 2006. “The Design Science Research Process : A Model for Producing and Presenting Information Systems Research.” In 1st International Conference, DESRIST 2006 Proceedings, 83–106. Claremont: Claremont Graduate University.
- Pigosso, Daniela C. A., Henrique Rozenfeld, and Tim C. McAloone. 2013. “Ecodesign Maturity Model: A Management Framework to Support Ecodesign Implementation into Manufacturing Companies.” Journal of Cleaner Production 59 (November): 160–173. https://doi.org/10.1016/j.jclepro.2013.06.040
- Pöppelbuß, Jens, and Maximilian Röglinger. 2011. “What Makes a Useful Maturity Model? A Framework of General Design Principles for Maturity Models and Its Demonstration in Business Process Management.” 19th European Conference on Information Systems, ECIS 2011, Helsinki, Finland, June.
- Prashar, Anupama. 2020. “Eco-Efficient Production for Industrial Small and Medium-Sized Enterprises through Energy Optimisation: Framework and Evaluation.” Production Planning & Control 32 (3): 198–212. https://doi.org/10.1080/09537287.2020.1719714
- Ritzén, Sofia, and Gunilla Ölundh Sandström. 2017. “Barriers to the Circular Economy - Integration of Perspectives and Domains.” Procedia CIRP 64: 7–12. https://doi.org/10.1016/j.procir.2017.03.005
- Romero, David, and Arturo Molina. 2014. “Towards a Sustainable Development Maturity Model for Green Virtual Enterprise Breeding Environments.” In IFAC Proceedings Volumes 47 (3): 4272–4279. https://doi.org/10.3182/20140824-6-ZA-1003.00430
- Rosa, Paolo, Claudio Sassanelli, Andrea Urbinati, Davide Chiaroni, and Sergio Terzi. 2020. “Assessing Relations between Circular Economy and Industry 4.0: A Systematic Literature Review.” International Journal of Production Research 58 (6): 1662–1687. https://doi.org/10.1080/00207543.2019.1680896
- Sacco, Pasqualina, Christian Vinante, Yuri Borgianni, and Guido Orzes. 2021. “Circular Economy at the Firm Level: A New Tool for Assessing Maturity and Circularity.” Sustainability 13 (9): 5288. https://doi.org/10.3390/su13095288
- Sassanelli, Claudio, Giuditta Pezzotta, Fabiana Pirola, Monica Rossi, and Sergio Terzi. 2019. “The PSS Design GuRu Methodology: Guidelines and Rules Generation to Enhance Product Service Systems (PSS) Detailed Design.” J. of Design Research 17 (2/3/4): 125–162. https://doi.org/10.1504/JDR.2019.10026968
- Sassanelli, Claudio, Paolo Rosa, Roberto Rocca, and Sergio Terzi. 2019. “Circular Economy Performance Assessment Methods: A Systematic Literature Review.” Journal of Cleaner Production 229 (August): 440–453. https://doi.org/10.1016/j.jclepro.2019.05.019
- Saunders, Mark, Philip Lewis, and Adrian Thornhill. 2009. Research Methods for Business Students. United Kingdom: Pearson Education.
- Sehnem, Simone, Lucila M. S. Campos, Dulcimar José Julkovski, and Carla Fabiana Cazella. 2019. “Circular Business Models: Level of Maturity.” Management Decision 57 (4): 1043–1066. https://doi.org/10.1108/MD-07-2018-0844
- Taddei, Emilia, Claudio Sassanelli, Paolo Rosa, and Sergio Terzi. 2022. “Circular Supply Chains in the Era of Industry 4.0: A Systematic Literature Review.” Computers & Industrial Engineering 170 (August): 108268. https://doi.org/10.1016/j.cie.2022.108268
- Thoben, K.-D, and J. C. Wortmann. 2013. The Role of IT for Extended Products’ Evolution into Product Service Ecosystems. Berlin, Heidelberg: Springer. https://doi.org/10.1007/978-3-642-40361-3_51
- Uhrenholt, Jonas Nygaard, Jesper Hemdrup Kristensen, Maria Camila Rincón, Sofie Adamsen, Steffen Foldager Jensen, and Brian Vejrum Waehrens. 2022. “Maturity Model as a Driver for Circular Economy Transformation.” Sustainability 14 (12): 7483. MDPI https://doi.org/10.3390/su14127483
- Uztürk, Deniz, and Gülçin Büyüközkan. 2022. “2-Tuple Linguistic Model-Based Circular Maturity Assessment Methodology: A Case for Agriculture.” IFAC-PapersOnLine 55 (10): 2036–2041. https://doi.org/10.1016/j.ifacol.2022.10.007
- Vinante, Christian, Pasqualina Sacco, Guido Orzes, and Yuri Borgianni. 2021. “Circular Economy Metrics: Literature Review and Company-Level Classification Framework.” Journal of Cleaner Production 288: 125090. https://doi.org/10.1016/j.jclepro.2020.125090
- Wagire, Aniruddha Anil, Rohit Joshi, Ajay Pal Singh Rathore, and Rakesh Jain. 2020. “Development of Maturity Model for Assessing the Implementation of Industry 4.0: Learning from Theory and Practice.” Production Planning & Control 32 (8): 603–622. https://doi.org/10.1080/09537287.2020.1744763
- Zeiss, Roman. 2019. “Information Flows in Circular Economy Practices.” 27th European Conference on Information Systems - Information Systems for a Sharing Society, ECIS 2019. Sweden: Stockholm & Uppsala.
- Zhang, Zhiwei, Ning Zhang, and Jiayi Gu. 2023. “A Systematic Literature Review of Digital Transformation of Manufacturing Enterprises: Bibliometric Analysis and Knowledge Framework.” Lecture Notes in Business Information Processing 481:144–155. Cham: Springer. https://doi.org/10.1007/978-3-031-32302-7_13