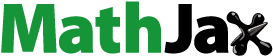
Abstract
Worldwide transport projects repeatedly exceed their budgets. This problem has received widespread attention over several decades, with little progress made to improve project cost performance. Consequently, this has led to the emergence of the following recurring question: How can public agencies responsible for delivering transport projects improve the estimation accuracy of their actual construction costs? We use the metaphor of the Sisyphean task to assist us in suggesting that the conflation of risk and uncertainty results in an inability to estimate actual construction costs accurately. Ambiguity aversion, an illusion of certainty, and the repeated occurrence of cost deviations, as demonstrated by our illustrative example and analysis of the elemental cost models of 39 transport projects, collectively create a Sisyphean loop that is impossible to break. Thus, we question the prevailing conceptualisation and treatment of risk and uncertainty used to estimate the actual construction costs of projects. We offer a new theoretical lens framed around ecologically rational heuristics and provide suggestions to reconceptualize and recalibrate the treatment and assessment of uncertainty in cost estimates. We hope this paper provides a much-needed circuit breaker to public agencies’ prevailing treatment of risk and uncertainty and stimulates new lines of inquiry to improve the cost performance of transport projects.
1. Introduction
Worldwide transport projects regularly exceed their budgets and are delivered late – a phenomenon yet to be adequately explained, though suggestions have been put forward over several decades (Merewitz Citation1973; Odeck Citation2004; Eliasson and Fosgerau Citation2013; Edwards and Kaeding Citation2015; Terrill and Danks Citation2016; Catalão, Cruz, and Sarmento Citation2019; Cavalieri, Cristaudo, and Guccio Citation2019; Love, Pinto, and Ika Citation2022; Welde and Klakegg Citation2024). A debate has unfolded on why transport projects experience cost overruns and benefit shortfalls, yet a consensus about how to address the problem remains a point of contention (Terrill and Danks Citation2016; Ika Citation2018; Flyvbjerg et al. Citation2018; Love, Ika, and Ahiaga-Dagbui Citation2019a; Kreiner Citation2020; Ika, Love, and Pinto Citation2022; Pinto Citation2023). However, with the increasing postponement or axing of projects due to labour shortages and the surging cost of materials, many governments have embarked on a period of self-reflection to determine and prioritise their infrastructure needs.
Examples include the delay of the Metro West in Sydney, Australia, which is impacting the construction of high-density apartments that are planned around most stations (Ludlow Citation2023; Tillet Citation2023) and the cancellation of High Speed 2 (HS2) in the United Kingdom (UK) (Hakimian Citation2023). At the centre of this existential introspection are governments’ concerns that their projects will exceed their cost and time forecasts and fail to deliver expected benefits. In addition, there is a fear of political fallout, the destabilisation of their term in office, and the disenfranchisement of the electorate, if not their distrust.
When projects exceed their budgets and planned completion dates, governments tend to question the nature of their procurement practices and toil by initiating inquiries and reviews to determine why this is the case. A case in point is the Ottawa Light Rail Transit project, which experienced cost increases, delays, and safety issues, resulting in an independent commission to investigate what went wrong and to identify ways to avoid similar problems in the future (Hourigan Citation2022).
Emerging from such inquiries and reviews are changes to policy and practice, which have tended to focus on the appraisal and the design and use of monitoring and evaluation before, during, and after the implementation of projects (Meunier and Welde Citation2017; Caffieri et al. Citation2018). The new or revised policies (e.g., the UK’s HM Treasury’s ‘The Greenbook 2022’) enacted under prevailing legislative and institutional frameworks (e.g., the requirement to achieve net zero carbon emissions), for example, have done little to mitigate the incidence of cost and time overruns. Project misperformance remains an ever-present reality despite the efforts to de-risk estimates using methods such as Estimating Using Risk Analysis, Real Options, Monte Carlo Simulation, and Reference Class ForecastingFootnote1 (RCF) to improve the accuracy of a transport project’s actual construction costs (Mak, Wong, and Picken Citation1998; Flyvbjerg and COWI 2004; Tseng, Zhao, and Fu Citation2009; Batselier and Vanhoucke Citation2016; Traynor and Mahmoodian Citation2019; HM Treasury Citation2022).
Burdened by the need to be accountable to taxpayers, most public sector agencies charged with procuring infrastructure assets would no doubt, at some point during the project planning and design process, have questioned their ability to establish an accurate estimate of their actual construction costs unconsciously knowing it is—what the absurdist philosopher Albert Camus (Citation1942) refers to in his writings—a Sisyphean Task; that is, an accurate assessment of actual construction costs cannot be achieved considering current practice and its tendency to conflate risk and uncertainty.
Against this backdrop, this paper addresses a question that perennially confronts the public sector: How can public agencies responsible for delivering transport projects improve the estimation accuracy of their actual construction costs? Indeed, this question has stumped many researchers and practitioners over several decades as they have sought to improve the cost performance of their projects with little avail (Love, Pinto, and Ika Citation2022). We by no means aim to provide a definitive answer to this question. Instead, we seek to identify a critical gap in knowledge contributing to and stymieing progress to procure cost-effective projects.
The conflation of risk and uncertainty – whereby uncertainty is reduced to risk – is a practice, we argue, that has contributed to inaccurate estimates of construction costs. We define risk as having a full range of potential outcomes that can be known and assigned known probabilities, whereas uncertainty can take varying forms. Uncertainty can be categorised by type (i.e. aleatory and epistemic), take various guises (e.g., ‘factual’, ‘probabilistic’, ‘meta-doxastic’, ‘value’ and ‘linguistic’) and be described using several dimensions (e.g., resolvable to radical) (Meder, Le Lec, and Osman Citation2013; Hansson Citation2022; Vis Citation2024). However, for simplicity’s sake, we adopt a ‘Knightian’ lens, whereby some of the outcomes and probabilities are unknown (Knight Citation1921).
In contrast to most previous studies examining the risk and uncertainty of cost overruns (e.g., Flyvbjerg Citation2008; Flyvbjerg Citation2012; Kim and Kwak Citation2018; Fu and Gil Citation2024), we consider these concepts as mutually exclusive concepts and, in doing so, we suggest that more accurate assessments of actual construction costs may be achieved. While our paper is not the first to distinguish between the concepts of risk and uncertainty (e.g., Ika, Love, and Pinto Citation2022: Love, Ika, and Pinto Citation2024), research has yet to couple this conceptual conflation with the depressive cycle where many projects tend to overrun their costsand the remedies are not on par with expectations, which is akin to a Sisyphean task from a metaphorical standpoint. We are cognisant that accurately determining actual construction costs is akin to an unrealistic goal. Still, strides can be made to mitigate the extent to which actual costs exceed planned budgets by applying subtle but distinct changes to estimating a project’s cost contingency and formulating its risk and uncertainty components (Love et al. Citation2021a,Citation2021b).
The central argument in our paper is that public agencies are trapped in a Sisyphean loop, repeatedly de-risking estimates using probabilistic approaches (e.g., overly relying on reporting ‘P10, ‘P50’ and ‘P90’). Treating uncertainty with the same optic for decision-making will not, as prevailing evidence for transport projects has manifestly demonstrated, result in improved assessments of actual construction costs. Thus, different rules for dealing with cost estimation under uncertainty in transport projects are required (Love, Ika, and Pinto Citation2023a).
Typically, the decisions to formulate cost estimates are based on a ‘risk characterization of an uncertain world’ (Mousavi and Gigerenzer Citation2014; Gigerenzer, Citation2014). However, structural differences between risk and uncertainty exist. Yet public agencies do not acknowledge or appear to understand these differences, as evidenced by the absence of strategies to deal with uncertainty in their published estimation guidelinesFootnote2. So, by merely reducing uncertainty to risk, we argue that public agencies unfittingly assume they have control of project costs. Consequently, they unintentionally underestimate the uncertainty that pervades the construction process, falling naïvely prey to optimism bias (Sharot Citation2011), which they, however, take to be the casus belli of cost underperformance, according to dominant theory and practice (Flyvbjerg et al. Citation2018; Love, Ika, and Pinto Citation2023a).
We commence our paper by using The Myth of Sisyphus as a framing to describe the public sector’s impossible task of accurately determining a transport project’s actual construction costs before briefly reviewing the cost performance literature (Section 2). Next, to illustrate the recurring mis-performance problem, the cost profile of transport projects delivered in New Zealand is analysed to reinforce and provide further context to assist us with addressing our research question (Section 3). In conjunction with the literature and the empirical analysis, we suggest a new theoretical lens for treating cost uncertainty and offer changes to practice to break the Sisyphus loop (Section 4). We conclude our paper by identifying future avenues for research and its contributions (Section 5).
2. The public sector’s Sisyphean task
We now draw on previous literature to examine how the public sector produces cost estimates for transport projects and identify key areas where it falls short in determining actual construction costs. Simultaneously, we refer to Camus’s (Citation1942) philosophical essay, The Myth of Sisyphus, as a metaphorical backdrop to provide deeper theoretical insights and a nuanced understanding of why inaccurate cost estimates of actual construction costs recurrently occur. Camus (Citation1942) tells the story of the mortal Sisyphus, condemned by the gods to roll a stone up a mountain for all eternity. Every time Sisyphus reaches the summit of a mountain, the stone rolls back down, forcing him to begin the task again, reinforcing the cycle that his existence is meaningless.
Producing an accurate estimate of expected actual construction costs, years or even decades in advance, for a transport project is a hopeless task for the public sector as the environment within which it is delivered is fraught with volatility, complexity, uncertainty, ambiguity (VUCA) (Flyvbjerg et al. Citation2018; Love, Pinto, and Ika Citation2022). Yet incumbent governments overwhelmingly exude confidence in their business case and decision-making processes, presenting a commitment to taxpayers that a project’s costs and delivery dates are absolute (Caffieri et al., Citation2018).
Beneath the façade of self-confidence– stemming from political aspirations of the government – the public sector agency responsible for delivering a project and its design team are confronted with a seemingly endless cycle of producing a scope and design, accommodating evolving stakeholder needs and demands, undertaking countless engineering iterations, and revising cost estimates (Caffieri et al., Citation2018; Fu and Gil Citation2024). Unquestionably, a public sector agency can relate to Sisyphus’s plight.
2.1. Naïveté: emergence of ambiguity aversion
Completing each stage of a transport project’s ‘gateway process’ provides a brief reprieve for a public agency and its design team until the proverbial stone rolls back down and work needs to begin on the next stage with more design-related information requiring estimates to be revised.
As more information becomes available, risks become known, and the pressure and stress to produce an accurate estimate build up (Love, Ika, and Ahiaga-Dagbui Citation2019a; Love et al. Citation2019b). Generating another estimate can seem as meaningless and frustrating as Sisyphus pushing a stone up the mountain. Cost delimiters are invariably identified, and the estimate is fine-tuned – sceptics may argue it is fudged – so as not to deviate too much from that affirmed from the previous stage (Flyvbjerg Citation2012). But, uncertainties can be downplayed due to ‘ignorance in knowledge’, which can take the following forms (Kutsch and Hall Citation2010; Nottelman Citation2015; Gigerenzer and Garcia-Retamero Citation2017): (1) factual ignorance (absence of knowledge of some fact); (2) object ignorance (unacquaintance with some object), (3) technical ignorance (absence of knowledge of how to do something); and (4) deliberate ignorance (a wilful decision not to know). Indeed, it is counterintuitive and irrational to forego any curiosity about the future and anticipate what could happen under varying conditions.
Without empirical research examining how ignorance influences decision-making during the formulation of cost estimates – bar Flyvbjerg (Citation2016) and Ika (Citation2018), who question whether ignorance is good or bad for projects – we take a charitable position and thus assume the technical know-how to assess uncertainties is hard to come by. In this instance, we view public agencies as consciously possessing an ‘ambiguity aversion’,Footnote3 unable to reconcile risk and uncertainty, instead focusing on the known risks impacting a project’s actual construction costs (Gigerenzer and Garcia-Retamero, Citation2017, 124).
It has been well-documented, however, that a transport project’s scope can change significantly from the date its business case is approved until it is handed over for operation (Merewitz Citation1973; Morris Citation1990; Hinze, Selstead, and Mahoney Citation1992; Love et al. Citation2017a,Citation2017b;Citation2017c; Love and Ika Citation2021; Welde and Odeck Citation2017; Welde and Dahl Citation2021; Welde and Volden Citation2022). The reasons for scope changes naturally vary, but the most common are overlooking or shifting stakeholder requirements and needs, price inflation, and resource constraints.
In 2015, the business case for the 1,700km Melbourne to Brisbane Inland Freight Rail project, for example, was estimated to cost AU$9.9 billion, and in 2021, AU$14.5 billion, with design changes being the main reason attributable for this sizeable increase (Terrill and Emslie Citation2021). Dwarfing this case is the California High-Speed Rail project, which was approved in 2008 and to be completed by 2022 at a cost of US$33 billion, but in 2022, this figure was revised to US$113 billion, with it likely to balloon to US$128 billion (Vartabedian Citation2022; Yost and Schelpp Citation2024). Design issues (e.g., tunnelling) and scope changes, inflation, delays in acquiring environmental approvals, and shortfalls in funding have contributed to increased costs and delays (Pettitt, Boyo, and Evers Citation2023).
Terrill, Emslie, and Moran (Citation2020) study of the cost performance of transport megaprojects revealed that 10% of those sampled final construction costs exceeded their approved business case estimates by more than 49%. This is a common situation, which is to be expected as the time between the approval of the business case and the completion of a project can be years or even decades; scope changes are bound to occur as the design is not finalised at a business case approval and costs will increase with inflation (Love et al. Citation2015a,Citation2015b).
Governments are certainly cognisant of the dangers of scope changes and creep, unplanned events, and the likely need for change orders, and accordingly, as we have mentioned, they have developed robust processes and procedures and utilised various tools and techniques to mitigate their impact. Calling for greater rigour in the estimation process, Terrill and Emslie (Citation2021) have called for the public sector to embrace RCF (i.e. adding an uplift to a project’s cost estimate and contingency) so that unknown risks and optimism bias can be avoided.
Regardless of the application of RCF, the risk of cost and time overruns is a chronic problem for public agencies. While they are invariably optimistic that they can control, manage and mitigate such risk, a forlorn hope prevails as there is an awareness that all their hard work to revise their cost and time estimates will likely be undone by unexpected events. A case in point occurred during the formulation of HS2’s original budget, which was determined using simple calculations that did not consider the project’s complexities and uncertainties (e.g., ground conditionsFootnote4, backfilling and stabilising mines, gas storage caverns, disposal of hazardous waste and high-cost elements)Footnote5.
Indeed, Camus (Citation1942) saw the fate of a workforce doing the same repetitive tasks daily as no less absurd than that of Sisyphus. We can draw parallels here with an epigram from Wilczek (Citation2015) – recipient of the 2004 Nobel Prize in physics – who cogently remarks, ‘naïveté is doing the same thing over and over, and always expecting the same result’. So, if public agencies continue to moderate the impact that uncertainties can have on an estimate’s formulation, transport projects will continue to mis-perform (Love, Ika, and Ahiaga-Dagbui Citation2019a).
2.2. Acceptance: an ‘illusion of certainty’
For the industrious Greeks, Sisyphus’s punishment was the ultimate torment—a futile task with no hope of completion; we equate this ineffectuality to the unlikelihood of transport projects being delivered within cost and time targets. As part of their procurement strategy, we often see public agencies offloading unidentified risks and uncertainties to the private sector and expecting them to absorb these associated costs (Love et al. Citation2021a). Traditionally, the practice of risk aversion – low-risk tolerance – has been the public sector’s typical default when modifying/extending existing and constructing new transport infrastructure assets. As such, it has provided a breeding ground for adversarial and opportunistic behaviours to manifest, resulting in contractual claims, delays, and, ultimately, a project’s mis-performance (Love, Pinto, and Ika Citation2022).
Proponents of relational (i.e. collaborative) procurement where risks are typically shared, such as Walker and Lloyd-Walker (Citation2015), Australian Constructors Association (Citation2017, Citation2021), Love et al. (Citation2021a), Walker, Vaz-Serra, and Love (Citation2022), and Haaskjold, Andersen, and Langlo (Citation2023) have been critical of public agencies’ low-risk tolerance approaches. Instead, such proponents have suggested that the public sector make greater use of relational procurement approaches such as alliancing – where collaboration, trust, and best-for-project outcomes thrive – to deliver large-scale transport projects as target costFootnote6 and time figures can be reliably estimated. The collaborative team of an alliance comprising the public agency, the design/engineering team, and a constructor jointly formulate a project’s target outturn cost and target adjustment events, which equally consider the allocation of risks and uncertainties (Walker, Love, and Matthews Citation2023).
Camus (Citation1942), like Kafka (Citation1916), Ionesco (Citation1954), and Pinter (Citation1959), to name a few, shares a similar absurdist and pessimistic vision of humanity where people vainly struggle to find a sense of purpose and control their fate—feelings of hopelessness, bewilderment, and anxiousness take centre stage. Camus (Citation1942) believed that people seek meaning in existence, but the universe is inherently meaningless, indifferent to this need. In Camus’ (Citation1942) view, Sisyphus was the archetypal absurdist hero—burdened by this meaningless task and facing an uncaring universe. Sisyphus acknowledges and accepts the absurdity of his fate. However, this is in stark contrast to the public sector, as it is unable to admit it has a mutual responsibility with the private sector to divide risks and uncertainties into two moieties based on who is best to manage them and deliver shared value (Love et al. Citation2021a).
For Camus (Citation1942), acceptance is crucial and equally so for the public sector as it must acknowledge that risks have to be shared to make headway towards ameliorating the performance of its transport projects. It is only natural for taxpayers to be vexed when a transport project’s performance goes astray from its planned cost and time targets and for incumbent governments to feel resentful when the media seeks to apportion blame on them for what was always going to be an inevitable outcome. Flyvbjerg (Citation2011) describes this inevitability with an ‘Iron Law’ where transport projects will be ‘over budget, over time, over and over again’ (321). An analogy can be drawn here with Sisyphus’ lived experience whereby he surrenders to the fate bestowed upon him.
When Sisyphus makes the long walk down the slope to retrieve the stone, he is not sad; quite the contrary. Sisyphus is at peace. Left by fate with no other options, Sisyphus revolts in the only way he can—by accepting the absurd situation, joyfully shouldering the burden, and making an ascent once again. Here, the accomplishment of the task provides contentment for Sisyphus, much like a public agency that goes through the motions to produce estimates, giving no meaningful consideration to the uncertainties that will derail a transport project’s performance. This point may be considered unfair, considering the due diligence afforded to preparing an estimate and implementing gates to improve project outcomes and mitigate risks. However, public agencies are cognisant that if a realistic assessment of uncertainties—even though they tend to implement any formal approaches to do so—were incorporated into an estimate, then a project’s cost-benefit analysis could be adversely impacted, rendering its viability questionable (Love et al. Citation2021b).
An inquiry by the New South Wales (NSW) Auditor General into Sydney’s Light Transit Rail (LRT) project, Australia, for example, revealed that the original capital budget of AU$1.6 billion established in 2013 increased by AU$549 million mainly due to mispricing and omissions in the business case (NSW Auditor-General 2016). Due to ensuing scope changes, the cost-benefit ratio decreased from 2.4 to 1.4. The project might not have proceeded if a cost-benefit ratio of 1.4 had been originally put forward in the business case.
According to Flyvbjerg and COWI (2004), estimates are often prone to optimism bias and strategic misrepresentationFootnote7. What is more, estimates are often purposefully manipulated or fudged to enable a project to appear as a viable proposition. As a matter of fact, Flyvbjerg et al. (Citation2018) make a bold claim that ‘the root cause of cost overrun [for all transport projects] is behavioral bias’ (174). To cater to such biases and strategic behaviours, RCF is drawn upon to de-risk estimates and provide an additional up-lift to a contingency to ensure actual construction costs do not exceed the project’s planned budget (Flyvbjerg Citation2008).
In essence, RCF acts as a ‘contingency on a contingency’ or ‘artificial inflator device’ and thus does not provide a rationale for accommodating cost uncertainties that can manifest during construction and derail a project’s cost and time performance (Love, Pinto, and Ika Citation2022, 3). Before proceeding any further with our paper, we must address the elephant in the room, which the UK public sector, for example, ignores when justifying uplifts derived from RCF for de-risking estimates. Optimism bias and strategic behaviour have not been shown to manifest empirically in the cost estimation process (Mak and Raftery Citation1992; Love, Pinto, and Ika Citation2022; Love, Ika, and Pinto Citation2024). Optimism bias has been only assumed to exist and while strategic behaviours may have occurred, they are difficult to detect and prove (Pinto Citation2023). Examples of strategic misbehaviour are few and far between but prominent cases receiving media attention are:
Brisbane’s Clem 7 – a 4.8-kilometre motorway tunnel, where a consultant provided misleading and deceptive estimates for a project’s toll revenue as it forecasted based 100,000 vehicles per day when the actual traffic volumes were approximately 22,000. Unable to collect enough toll revenue to pay interest on an AU$1.3 billion debt, the operator went into receivership (Tam Citation2015); and
HS2, where the intentional cost underestimationFootnote8 occurred and their subsequent escalation, was deliberately covered up (The Committee of Public Accounts, 2020; Calvert and Artbuthnott Citation2023). The project has been cancelled due to spiralling costs and mismanagement (Oakervee Citation2020; Norris and Tetlow Citation2023; Public Accounts Committee Citation2023).
The assumption that optimism bias and strategic behaviour are the root causes of cost overruns and, therefore, all estimates produced for transport projects need to be de-risked for their possible presence is devoid of ‘objective meaning’ (Love, Ika, and Ahiaga-Dagbui Citation2019a; Ika, Love, and Pinto Citation2022; Pinto Citation2023). Bolstering this position, Jørgenson et al. (2023) suggest we should not ‘reward’ misrepresentation and bias by accommodating them in a cost estimate as a risk when they are an unknown entity (3481). Nonetheless, statistical modelling can only accommodate what is known, not what is unknown. Frankly, assigning objective probabilities with future consequences, where a decision-maker ‘hasn’t got a clue’, is ‘highly questionable if not invalid in principle’ (Davidson Citation1991, 130). Moreover, ‘Bayesian decision models are not necessarily valid in the natural environment and appear to be primarily driven by mathematical convenience—and less so by Brunswik’s argument (Citation2001) that cognition and environment are indivisible like a married couple who have come to terms with each other through mutual adaptation’ (Hertwig et al. Citation2022, 468). Yet, methods relying upon statistical analysis such as RCF, for example, conflate risk and uncertainty, treating them to be ‘blindly synonymous’ and providing an ‘illusion of certainty’ for determining the actual construction costs for transport projects (Love, Pinto, and Ika Citation2022, 3; Pinto Citation2023).
We will now briefly look at previous transport cost performance studies where the recurring problem experienced by the public sector is identified before presenting what is repeatedly known using a new dataset. The absurdity here is that the cost overruns are normalised, becoming a putative feature of transport infrastructure procurement. This tragic situation resembles Sisyphus’s hopeless struggle, where he understands his fate but has no hope for reprieve. However, the lucidity he achieves with this understanding places him above his fate. This is a position the public sector seemingly takes after audits, reviews, and inquiries have been undertaken to determine why project costs exceeded their budget. Indeed, a degree of learning may occur, and changes to procurement practices may be implemented. Despite this, the public sector continues to misunderstand ‘how projects work’ in VUCA environments. Flyvbjerg (Citation2011) uses his ‘Iron Law’ to describe this state of affairs that the public sector confronts when procuring transport projects.
2.3. The recurring problem: over budget, over and over again
In , we present examples of studies that have sought to quantify the cost performance of transport projects in a range of countries. A cursory glance at reveals that the reported cost deviations vary significantly due to the delimiters that quantify their impact on a project’s performance (i.e. reference point, X Final Construction Cost, FCC). For example, the calculated cost deviation is larger in the studies where the delimiters are ‘the decision to build’ and the ‘initial estimate’ to FCC. Studies using different delimiters to determine a project’s performance make it impossible to compare findings (Love, Ika, and Ahiaga-Dagbui Citation2019a; Love et al. Citation2019b).
Table 1. Examples of transport cost performance studies (in chronological order).
If the decision to build, for example, is used as a reference point, then a more significant cost deviation is expected as the project’s level of definitionFootnote9 would typically be incomplete. As the project’s scope is defined and its design and engineering are finalised, estimated costs will tend to increase (Cavalieri, Cristaudo, and Guccio Citation2019).
However, as shown by Love et al. (Citation2019b) in , this is not always the case as estimated costs may decrease from a project’s ‘Approved Budget (AB) Pre-Tender Estimate (PTE)’ (). Bids are then called for by potential contractors (or consortiums) and negotiations about a project’s price will ensue. Additions or deletions to the project’s scope can occur during these negotiations (). Once a price for the works is agreed, the contract is awarded. The contract award provides the public agency price certainty for the agreed scope of work specified in the contract documentation; hence, it is used as a delimiter for determining cost deviations. Any changes sanctioned by the public agency (or their representative) after the contract award and/or unexpected events occur, which the estimated contingency cannot accommodate, will result in additional costs to a project.
In , scope and change orders from a contract’s award are the main contributors to cost deviations writ large (e.g., Hinze, Selstad, and Mahoney Citation1991; Vidailis and Najafi Citation2002; Makovšek, Tominc, and Logožar Citation2012; Love et al. Citation2017c; Love et al. Citation2019b). However, it has been vehemently argued by Flyvbjerg, Holm, and Buhl (Citation2002), Cantarelli et al. (Citation2012), and Flyvbjerg et al. (Citation2018) that optimism bias and strategic misrepresentation are the root causes of cost overruns in transport projects despite the lack of causal evidence to substantiate their assertions.
Explanations of why cost deviations manifest and result in transport projects repeatedly mis-performing abound in the literature. Three prominent theories have been relied upon to explain a project’s cost deviation, these being the: (1) Hiding Hand (Hirschman Citation1967); (2) Planning Fallacy (Malevolent Hand) (Flyvbjerg Citation2016); and Fifth Hand (Ika, Love, and Pinto Citation2022). It is outside the scope of this paper to examine these theoretical perspectives, as reviews of them can be found in the works of Kriener (2020) and Ika, Love, and Pinto (Citation2022). We hasten to note that no consensus exists on which theory provides the ‘best’ explanation for the causes of deviations in project costs. While Love, Pinto, and Ika (Citation2022) and Ika et al. (Citation2023) acknowledge the explanations of project behaviour suggested by the Hiding Hand and Planning Fallacy, they argue the Fifth Hand provides a balanced and nuanced account. However, empirical research is needed to test the validity of the Fifth Hand in real-life project settings.
Within a cost estimation context, we see the Hiding Hand acknowledging the role of ignorance and underestimating task complexity in projects, providing a setting for considering uncertainty but without clear-cut strategies that can enable rather than limit decision-making. In stark contrast, the Planning Fallacy does not consider the presence of ignorance but instead assumes a wilfulness to underestimate the complexity and overlook risks due to innate behavioural biases or deliberate strategic misbehaviours. As a result, uncertainty is ignored and emphasis is placed on risk settings, which gives rise to an illusion of certainty.
While the Hiding Hand and Planning Fallacy adopt monistic views to deal with uncertainty and risk or conflate these notions under their respective positions, the Fifth Hand treats them as distinct entities, giving equal credence to how they will impact a project’s performance (Ika, Love, and Pinto Citation2022). Nevertheless, the conflation of risk and uncertainty by much of the literature and public agencies results in them having to shoulder the burden of trying to produce an accurate assessment of actual construction costs.
The consequence of conflating risk and uncertainty is examined using an illustrative example and abductive reasoning. The dataset we present in the next section of this paper reinforces the studies identified in , adding to the body of work that examines project cost performance. In addition, we show that a transport authority conflates risk and uncertainty, like many others worldwide, thereby reducing uncertainty to risk.
3. Illustrating a leitmotiv of practice
Determining a project’s cost deviation appears to be a straightforward task and, as mentioned above, it is typically expressed as a ratio of the cost estimate at the decision to build or contract award, for example, over FCC (Makovšek, Tominc, and Logožar Citation2012). However, a fundamental difficulty is obtaining primary data due to political and commercial sensitivities and in a format that can be effectively used for analysis (Makovšek, Tominc, and Logožar Citation2012).
Surprisingly and without solicitation, a consultancy organisation involved with providing pre-and-post-contract cost management services to a public agency voluntarily provided us with primary cost data for a sample of 39 transport projects constructed in New Zealand as they could see parallels with the findings reported in Love et al.'s (Citation2019b) Hong Kong study (). Due to confidentiality issues, the names of the projects, the contractors, consultants, and the like have been withheld. We use procedures associated with formulating the estimates and the analysis of the project’s actual costs to provide an empirical backdrop to help us address our proposed research question.
3.1. Dataset
Under the public agency’s cost estimation guidelines, the consultancy organisation was required to complete an ‘elemental cost questionnaire’ after a project’s final account had been completed so that data could be incorporated into their ‘Elemental Cost Database’ and used to support the production of future estimates of actual costs. The questionnaire requests a considerable amount of detail about a project, such as its type, the project’s terrain, length, the nature of earthworks, details about structures and pavements, traffic management, procurement method duration, delays, and an elemental breakdown of the implementation estimate (i.e. contract award) compared with actual costs. Examples of the elemental cost data provided to the public agency at a project’s completion can be seen in and .
The cost estimates prepared before the contract award were unavailable – they were confidential. However, it is the point at which a degree of cost certainty can be provided to the public sector as they sign a contract with a construction organisation (or consortium) to deliver an asset for an agreed price. Specific details of the cost estimation processes for transport projects can be found in the Waka Kotahi New Zealand (NZ) Transport Agency (2023) ‘Cost Estimation Manual’. Within this cost estimation manual, risk and uncertainty coalesce under the auspices of an estimate’s ‘contingency’ and ‘funding risk contingency’ components, with the explicit instruction to calculate their values using statistical models. Thus, uncertainty is characterised and determined with a risk-focused lens, as noted in Section 9 of the NZ Transport Agency’s ‘Cost Estimation Manual’ (2023, 35). The data used for analysis and derived from the 39-transport project’s elemental cost models are available as a supplementary file, and all monetary values presented in our study are in NZ dollars. The analysis demonstrates that even though the price for delivering a project’s scope of work is agreed upon, cost still increases over and above the contingency allowance.
We were not permitted access to the cost contingency allowance as it was confidential for each project. However, we were advised by the consultancy organisation that an average of 5% would be a reasonable assumption to make across the sample as a significant proportion of projects were re-measurement contracts. The cost contingency assumption aligns with Clark and Lorenzoni's (Citation1985) recommendation of 3% to 5% and the empirical observations of Love et al. (Citation2015a). In the case of traditional lump sum contracts, for example, the cost contingency would be lower as the design is supposed to be complete, with the likelihood of changes being minimal. Similarly, this situation would apply to the other procurement methods adopted (e.g., D&C and ECI).
3.2. Analysis
presents a series of histograms to represent our sample project characteristics visually. We can see that 74% (n = 29) of the projects were < NZ$30 million, and only three were > NZ$100 million. provides a breakdown of the costs by project size. The sample’s costs per kilometre (km) were x̅ = NZ$9,252,306, = NZ$11,896,312.
Table 2. Breakdown of projects by size.
Several procurement methods were used to deliver projects, with traditional lump sum and re-measurementFootnote10 forms accounting for 69% (n = 27). Traditional re-measurement was required for projects where the nature of the critical work could be detailed at the time of tender. Still, quantities would have been unknown for several elements, such as earthworks and remedial works. Markedly, re-measurement methods were utilised across the spectrum of project types sampled, except for motorways (new) and carriage improvement (safety). However, most project types were upgrades and additions to existing infrastructure, 82% (n = 32), with new motorways accounting for 18% (n = 7).
The descriptive statistics for the sample’s cost and time deviations are summarised in and , with providing a breakdown by procurement method and project type. Here, cost deviations are x̅ = 18.28% and = 24.73% of the contract value, and time deviations from the expected completion, x̅ = 1.74 months and
= 5.53 months. As noted in , the mean cost contingency at contract award of 5% was insufficient, falling short, on average, by 13.28%.
We also observe a project’s contract period (i.e. duration) and reveal the cost is not a predictor of deviations (). A breakdown of cost and deviations by project type, procurement method, and size are presented in . The total cost for the 39 projects analysed is NZ$1,308,321,641, with a x̅ = NZ$33,546,708, = NZ$58,793, 985,
(min) = NZ$2,623,250, and
(max) =NZ $342,089,198.
Figure 7. Scatter plots. (a) Contract period and cost deviations. (b) Project cost and time deviations

Table 3. Project type: Cost and time characteristics.
Table 4. Procurement method: cost and time characteristics.
Table 5. Project size: cost and time deviations.
To measure the strength of the linear relationship between cost deviations and project characteristics, we performed a correlation analysis with the results presented in . The results show a significant but weak correlation between procurement method and project type, which can be explained by the public agency predominately drawing on the use of traditional methods as they sought to provide cost and time certainty as the design is complete at the time of contract award. Also, a significant but weak correlation exists between project cost and the cost per km, with a strong correlation with contract duration. Thus, larger projects will tend to have longer contract durations, and therefore, a contractor’s preliminaries will rise, and labour and materials prices are prone to escalation, for example, increasing the cost per km.
Table 6. Correlation matrix.
We performed a one-way Analysis of Variance (ANOVA) test to determine if there were any statistical differences between the means for various project types and procurement methods for time and cost deviations. In doing so, we can ascertain the characteristics of projects impacting their performance. It can be seen in that the one-way ANOVA reveals that there was not a statistically significant difference in cost deviations between project types F (23,15) = 0.968, p > 0.05, and procurement methods F (23,15) = 1.304, p > 0.05. Similarly, in , the ANOVA shows that there was not a statistically significant difference in time deviations between project types F (20,18) = 3.777, p > 0.05, and procurement methods F (18, 38) = 0.909, p > 0.05.
As we categorised project size as a nominal variable comprising five classifications, we used a Kruskal-Wallis Test to determine if there were differences in mean cost and time deviations between them. However, no significant differences exist for cost H (4) = 2.43, p = 0.65 and time deviations H (4) = 4.84, p = 0.30. These findings accord with previous studies, and, as a result, the notion that projects of the same reference class display similar statistical cost and time profiles is an inaccurate assumption to make, which renders the method of RCF as an estimating method highly questionable (Love et al. Citation2014; Love et al. Citation2019b).
presents extracts from a sample of a project’s elemental cost model’s explanation for cost and time deviations, with scope and design changes identified as the main contributors. We also note that escalation and inclement weather had an adverse impact on the cost and time of several projects. In the case of the rural alignment project that experienced a 137% and 133% cost and time deviation, respectively, a considerable amount of redesign occurred due to the uncertainty surrounding geotechnical conditions and the difficulty with acquiring Resource ConsentsFootnote11. Due to the re-design, the project’s completion date had to be extended beyond what was initially planned, and preliminary costs increased.
To recap, the data we have presented demonstrates the recurring problem of projects exceeding their expected costs and that estimating guidelines treat the concepts of risk and uncertainty as the same, ignoring their distinct differences. Despite the project cost data being rich in content ( and ), the sample is small. The differences between procurement methods, project types, and cost deviations thus should be treated with caution as the number of projects in each grouping was insufficient for definitive conclusions to be made. Additionally, we did not have access to the initial estimates and the cost contingencies at contract award. Governments and consultants rarely provide access to the actual cost data from inception to completion, so we have had to do with what was provided. In doing so, we have had to rely on abductive reasoning to make inferences based on our knowledge of the cost estimation process and the literature, contributing to answering our research question.
4. Improving the estimation of construction costs
Despite the wealth of research and the tireless efforts of practitioners, the public sector still unequivocally struggles to deliver their projects on budget and time, metaphorically resembling Sisyphus’ journey of pushing the rock up the hill. So, how can public agencies improve the cost estimation accuracy of their transport projects? We answer this question by drawing on the broader literature and the context behind the production and analysis of elemental cost models. In doing so, we hope to help break the Sisyphean loop by suggesting reconceptualizing and recalibrating the treatment and determination of a project’s cost uncertainty. Thus, changes to practice need to be engendered and enacted if strides are to be made in producing more realistic assessments of a project’s actual construction costs.
We now focus on how public agencies can engender meaning into the estimation process and counter the absurdity of accepting the fate that their transport projects will naturally succumb to the ‘Iron Law’. In doing so, we aim to provoke debate and contribute to an alternative way of thinking about decision-making uncertainty over and above the classic view of (unbounded) rationality that pervades cost estimation practiceFootnote12.
4.1. Implications for theory
We suggest that public agencies embrace the theoretical lens of ecological rationality, which extends Simon’s (Citation1955, Citation1956, Citation1990) concept of bounded rationality, in their decision-making process under uncertainty when it comes to estimating a transport project’s actual construction costs. Equally, this applies to the procurement of all types of infrastructure projects. In stark contrast to unbounded rationality, ecological rationality considers human reasoning and behaviour to be ecologically rational when adapted to the environment in which they act (Todd and Brighton Citation2016). Accordingly, it aims to identify the environmental properties that permit the simplification of a decision strategy and determine the properties that enable its ease of use and accuracy when information is limited (Hertwig et al. Citation2022). The decision strategy we refer to here is smart heuristics (Gigerenzer and Brighton Citation2009; Gigerenzer and Gaissmaier Citation2011; Gigerenzer, Citation2014).
Smart heuristics are simple, task-specific decision strategies that form part of a decision maker’s repertoire of cognitive strategies for solving judgement and decision tasks. Such heuristics, relying on limited information, can be applied consciously or unconsciously to make fast and frugal judgments under conditions of uncertainty and limited learning opportunities with high accuracy (Todd and Gigerenzer Citation2000; Gigerenzer and Gaissmaier Citation2011; Luan et al., Citation2019). Even if managers have unbounded cognitive capacities, it has been shown that smart heuristics can make more accurate, efficient, and effective decisions in the real world (Marewski and Gigerenzer Citation2012; Luan et al., Citation2019). The accuracy that smart heuristics can provide renders them the decision-making strategy of choice for determining the cost uncertainty of a project’s estimate (Gigerenzer and Goldstein Citation1996; Gigerenzer et al. Citation1999; Love et al. Citation2021b).
As heuristics enable quick decision-making, they have become associated with making errors and an accuracy-speed trade-off is deemed to prevail (Kahneman Citation2011). Be this as it may, when heuristics are used to inform strategic decision-making, for example, organisations have been shown to experience improvements in profitability and growth (Baum and Wally Citation2003). Moreover, it has been shown that when managers rely on heuristics for analytical decision-making in project environments, accurate outcomes ensue (West, Acar, and Caruana Citation2020). In theory, environments subject to VUCA, such as the procurement of transport projects, provide prime conditions to utilise smart heuristics for decision-making under uncertainty, with probability theory only appropriate in risk settings (Gigerenzer, Citation2014; Gigerenzer, Reb, and Luan Citation2022).
As shown in , we suggest that the risk component of an estimate should be dealt with separately using probability theory. Thus, it follows that smart heuristics would require public agencies to separate their treatment of risk and uncertainty formally and, in doing so, improve an estimate’s transparency. Both the traditional and RCF methods denoted in fall short in estimating a project’s actual construction costs – empirical evidence manifestly and repeatedly demonstrates this to be the case, as we have put forward in this paper. Our ‘balanced approach’ of cost estimate production identified in , which we suggest public agencies should consider, embraces the optics of unbounded and ecological rationality.
Our proposed approach is theoretically sound and, while practically intractable at this point, it offers a way for public agencies to escape the Sisyphean loop of repeatedly underestimating project costs, conflating uncertainty and risk. Indeed, we have limited understanding and knowledge about the environments where a given heuristic will succeed and in which it will fail (Ika, Love, and Pinto Citation2022). Knowing what decision strategies are the most effective within varying contexts and situations will facilitate the determination of uncertainty and re-evaluation of risk (Love et al. Citation2023a, Citation2023b, Citation2023c).
A post-completion database and piggybacking on the vicarious experiences of contractors can provide public agencies with knowledge about how smart heuristics can be coupled with an environmental structure to make decisions to help contribute to producing a realistic and accurate assessment of a project’s actual construction costs. As knowledge about ‘what goes right and wrong’ in transport projects accumulates, suitable smart heuristics can be unearthed.
To ensure success and move our understanding of how smart heuristics can be tailored to different epistemic and pragmatic tasks that confront practice in construction, an adaptive toolbox will be required to ensure their generalisability in varying contexts. An adaptive toolbox is the collection of heuristics and building blocks an individual and team have at their disposal for constructing heuristics, together with the core mental capacities that building blocks exploit (Gigerenzer et al. Citation1999; Gigerenzer, Reb, and Luan Citation2022).
Within a given adaptive toolbox there would typically be several classes of smart heuristics that enable individuals and teams to make judgments, which include (Gigerenzer and Gaissmair Citation2011): (1) recognition-based decision-making used to evaluate options based on their identification (e.g., recognition and fluency heuristics); (2) one-reason (sequential) decision-making (e.g., one-clever-cue heuristics, and take-the-best); (3) trade-off (equal weighting) decision-making where simple 0/1 or equal-eights are forgone to reduce estimating error (e.g., tallying, and 1/N rule); and (4) satisficing, where the first option is chosen to meet an aspiration level (i.e. information consideration does not follow an ordering sequence).
As mentioned, uncertainty ranges from ‘resolvable’ to ‘radical’, whereby the former can be removed with more or better information, but the latter cannot. Multiple unknowns, obscurity, ambiguity, and vagueness with unknown and unknowable outcomes characterise radical uncertainty (Vis Citation2024). So, as smart heuristics are identified and developed from actions and situations arising in projects and applied to accommodate uncertainty, we can equally see information being accumulated, enabling some uncertainties to be resolved and unknown outcomes to become knowable. Resolvable uncertainty can, therefore, be mitigated as information and knowledge become available, allowing the elicitation of subjective (or frequencies) rather than discrete probabilities for phenomena to be garnered from experts (Blass, Lach, and Manski Citation2010). Undoubtedly, practitioners will hold some subjective beliefs about cost estimation. Still, with incomplete information, there is a proclivity for them to make things up and thus overlook the reality of practice (Fischoff et al. 1999).
4.2. Implications for practice
Fields such as business, finance, medicine, law, and psychology have benefitted from utilising smart heuristics when making decisions under uncertainty (Gigerenzer Citation2008; Mousavi and Gigerenzer Citation2014; Neth, Meder, and Gigerenzer Citation2014; Luan et al., Citation2019). However, heuristics have received limited attention within the context of procuring transport projects and, more broadly, infrastructure asset delivery, but their potential use in practice is boundless (Love, Ika, and Pinto Citation2023a;Citation2023b).
Transport agencies often outsource the production of cost estimates (including the contingency) for their projects to an external consultant. A team of quantity surveyors or cost engineers with the expertise and knowledge of estimating practice will prepare a cost estimate based on the design and specification provided by a transport agency. There is an explicit expectation that the consultancy organisation adheres to its procedures and guidelines for producing, reviewing, and submitting cost estimates. Moreover, cost estimates are subjected to independent peer review (e.g., Waka Kotahi NZ Transport Agency. Citation2023) making improbable the likelihood of optimism bias manifesting in a cost estimate. In the 39 projects we analysed, the cost estimates were prepared per the transport agency’s cost estimation manual; however, uncertainty was reduced to risk, with the contingency being insufficient to accommodate subsequent changes that materialised in the projects. Apart from assigning arbitrary probabilities to possible events, no attention was given to formally assessing uncertainty.
A cogitative and inquisitive mindfulness is typically required to assess uncertainty to ‘anticipate what might go wrong’ during construction and cause cost increases and delays. Determining the unknown requires understanding a project’s context and attention to past situations where cost and time deviations from those of a similar reference class occurred (Love et al. Citation2023c). Likewise, an understanding of ‘what went right’ and the best practices used to deliver projects successfully is de rigueur. Thus, practitioners’ experience and knowledge from previous projects are needed to garner such an understanding and identify best practices. If strides are to be made to adopt smart heuristics, then the public sector needs to: (1) better learn from failure and success to manage uncertainty so that an adaptive toolbox of heuristics can be established; and (2) embrace relational contracting so that risks and uncertainties can be shared, better identified, and given greater consideration.
4.2.1. Learning from failure and success to manage uncertainty
Learning from failure and success provides a basis to unearth the intricacies, fragilities, and nuances of practice that may be taken for granted when making decisions though they can significantly impact project outcomes (Ika and Pinto Citation2022). A case in point is the repeated conflation of risk and uncertainty, a leitmotiv of practice that is theoretically questionable in our view. A forensic examination of a project’s cost data will invariably reveal that its contingency is underestimated (Baccarini and Love Citation2014) not because of optimism biasFootnote13 but rather due to its uncertainty being ignored or merely reduced to risk (Love, Ika, and Ahiaga-Dagbui Citation2019a).
Cynically, it can be argued that a project’s viability will be questioned when its uncertainty is realistically assessed and added to an estimate. However, this is a myopic view, as the actual cost for work will not be known until a contractor submits a price based on the information provided in the tender documentation and it is agreed upon. Requesting changes after a contract has been agreed upon is costly for the public sector and increases costs; it is not unusual for a contractor to submit a low bid and over-charge for scope and change orders to increase their profit margin. Conventional procurement methods, such as traditional and D&C forms, provide no incentive for project participants to minimise the cost impacts of subsequent changes. Instead, ‘they allow the incumbent participants to charge ‘monopoly’ prices for the additional work’ (Hayford Citation2018, 3).
Feedback from completed project costs, such as the elemental cost models produced by cost consultants and used in this research, provides a point of reference for public agencies to learn and formulate new estimates and simultaneously improve their ability to assess the risks that can influence actual construction costs. The creation of a post-completion database, as suggested previously, accessible by all transport agencies, containing details about scope and change orders and their justification, agreed actual start and finish dates, actual costs, and progress against performance indicators would, for example, also help improve the accuracy of estimates, though, at the moment, it does not exist (Terrill and Danks Citation2016; Liu et al. Citation2021; Love et al. Citation2023c). Should a database be created, collaboration with the private sector (e.g., contractors and consortiums) is needed to populate and maintain its currency. Still, the absence of incentives (e.g., tax relief) and mechanisms (e.g., regulatory reform) to facilitate information sharing between the public and private sectors hinders progress towards its development (Love et al. Citation2021a).
4.2.2. Embrace relational contracting
Changing tack, we now suggest public agencies actively pursue relational contracting principles to improve the cost performance of their transport projects. This suggestion has been repeatedly made for decades as a result of an array of independent reviews and calls from industry bodies worldwide. Still, relational procurement has yet to form the mainstay of the public sector’s procurement strategy for transport projects (Love, Pinto, and Ika Citation2022). Conventional procurement methods are preferred for the cost certainty, simplicity, and risk transfer they provide to public agencies (Hayford Citation2018). Yet, as reiterated in our results, we know cost certainty is simply a fallacy, yet these continue to be the procurement methods of choice by many public agencies worldwide.
As we mentioned above (Section 2.2), the reliability of a project’s target outturn cost can generally be better guaranteed under an alliancing arrangement than other procurement forms (Walker, Harley, and Mills Citation2013; Love, Pinto, and Ika Citation2022). But why and how? We must reiterate that our study found no statistical difference between procurement methods and mean cost and time deviations, though the sample is small ( and ). Thus, we are not concerned with the procurement method per se but the process of generating a robust, transparent, and reliable target outturn cost within an alliance, as it significantly differs from the conventional approach.
Like an alliance, ECI allows for collaborative input into the design from a contractor, enabling them to provide suggestions to de-risk a project’s scope, but their influence on determining actual construction cost estimates is limited. In the four projects analysed, scope and design changes (e.g., specifications, such as the example in ) were required, increasing costs and causing delays.
In short, an alliance is underpinned by a Project Alliance Agreement (PAA) and a governance system that enables collaborative behaviours (e.g., open communication, no blame, respecting diversity, and ensuring a ‘best-for-project’Footnote14 mindset) to be maintained and managed. Moreover, including a financial reward mechanism to support ‘best-for-project’ decision-making provides an incentive for both the public and private sectors to work in unison to share knowledge and produce realistic assessments of target outturn cost and target adjustment events (i.e. risks and uncertainties) (Walker, Vaz-Serra, and Love Citation2022). The collective learning environment of an alliance creates an environment where the project team focuses on sharing their knowledge and experiences to reduce uncertainty. The collaborative environment inter alia facilitates dialogue and trust and confidence in applying smart heuristics. The process of generating a target outturn cost and the willingness to treat uncertainty as a distinct entity can help an alliance better plan and manage a project.
Despite embracing a process of anticipation, it was surprising to uncover that the two alliance projects in our sample experienced 25% and 65% cost deviations, significantly larger than the samples x̅ = 18.28% (). They were, however, delivered early and on time. Regardless, there will always be exceptions to the rule, even though the prevailing evidence indicates that alliances offer superior cost performance outcomes than other procurement methods (Weigle and Garber Citation2010; Walker, Harley, and Mills Citation2013; Walker and Lloyd-Walker Citation2015; Almaaz, Zakaria, and Odimegwumas Citation2020; Walker and McCann Citation2020; Walker, Vaz-Serra, and Love Citation2022).
If we take, at face value, the cost deviations for the alliance projects, for example, it would be rational to assume that they had mis-performed. However, alliances are typically used for complex projects with an ill-defined scope (Department of Infrastructure & Regional Development Citation2015a); thus, vicissitudes are expected to arise. In both projects, significant changes to the scope were required due to complex ground conditions. One project required increased motorway length and additional in-situ road bridges (including bored piles), and the other required a level crossing and road bridges. Comprehending the context behind the number is important before a conclusion can be drawn.
Accordingly, we need to take heed and seek to understand and learn from the environment, situations, and set of actions that cause projects to mis-perform (Ika and Pinto Citation2022; Love, Ika, and Pinto Citation2023a)—and in doing so, building resilience to the unexpected. However, there is a mismatch between the unbounded view of rationality utilised in alliances and, more generally, by public sector agencies for decision-making in the VUCA environment within which they are made. The acceptance of unbounded rationality by public agencies, like Sisyphus recognising his fate, has a certain formality, as the eternal struggle against the fate of the ‘Iron Law’ encourages the adoption of statistical methods to deal with unknowns when they are seemingly inevitable.
Unlike Sisyphus, who acquiesced his fate and remained content with never reaching his goal, we believe the public sector cannot continue to accept the absurdity of normalising transport project mis-performance. Treating uncertainty in cost estimates with a probabilistic lens has contributed to this situation. As noted earlier, an alternative view to unbounded rationality is required to deal with uncertainty, which we hope public agencies will consider in their guidelines for cost estimation.
5. Conclusion
Despite the sheer volume of research that has sought to explain why and how transport projects experience cost deviations, they remain an ever-present reality for the public sector and taxpayers. Tools such as RCF – based upon applying probabilistic-based uplifts to a cost estimate and contingency – have been developed and adopted by some public agencies to de-risk projects as there is a belief that optimism bias and strategic misrepresentation are the root causes of cost overruns. However, optimism bias has never been empirically shown to exist, and only a few instances of strategic misrepresentation have been anecdotally identified. Thus, the justification for adding an uplift to a project cost estimate and contingency and thus reducing uncertainty to risk is flawed.
Reconceptualizing the treatment of risk and uncertainty is needed if strides are to be made to better estimate a project’s actual construction costs. In doing so, we have taken an absurdist view of transport cost performance in this paper using the Sisyphean loop as a metaphor to describe the public sector’s tireless effort to deliver their projects within budget while knowing that this may be a meaningless task and accepting the inevitability of a cost overrun. Repeatedly conflating risk and uncertainty and using probabilistic approaches to estimate a project’s construction costs contribute to trapping public agencies into hoping they can deliver their projects on budget.
We have suggested that the combination of ambiguity aversion, an illusion of certainty, and the repeated occurrence of cost deviations, as noted in and supported with empirical data from a small sample of 39 projects procured by a transport agency, reinforces the Sisyphean loop, rendering it impossible to break it, considering the prevailing conceptualisation and treatment of risk and uncertainty. Changes in design and scope were the leading causes of cost deviations, not optimism bias and strategic misbehaviour. In fact, the cost estimates and final elemental cost models for the examined transport projects were produced by external consultants and vetted by independent reviewers. However, the allocated cost contingency was insufficient at the contract award by an average of 13.28%. Thus, we have suggested that changes to practice are required as circuit breakers to enable public agencies to: (1) harvest knowledge from developing a post-completion database, (2) acquire vicarious experiences of contractors through collaboration and relational procurement; and (3) reconceptualize and recalibrate their treatment of uncertainty through ecological rationality lens, where smart heuristics become the apex strategy for decision-making.
We acknowledge that our practice recommendations are speculative and that research is required to develop a post-completion database’s format, structure, and content and determine how it can be utilised effectively during the cost estimation process. Similarly, knowledge about the type and nature of smart heuristics that can inform decision-making within varying environmental structures is lacking. As a result, future work is required to fill this void.
Using the Sisyphean task as a metaphor, we have put forward a different and untapped line of inquiry that may explain why projects experience cost overruns. To this end, the main contributions of our paper are twofold: (1) the analysis demonstrates that the cost profiles of projects do not vary and reconfirms that vicissitudes contribute to cost deviations, not optimism bias and strategic behaviour (2) a new conceptual framing by taking a balanced approach to examine risk and uncertainty in a cost estimate by combining unbounded and ecological rationality is presented.
Data availability statement
All data supporting this study’s findings are available in the attached supplementary file and from the corresponding author upon request.
Disclosure statement
The authors report that there are no competing interests to declare.
Additional information
Notes on contributors

Peter E. D. Love
Peter E. D. Love received a Ph.D. in operations management from Monash University, Melbourne, Australia, in 2002. He is a John Curtin Distinguished Professor with the School of Civil and Mechanical Engineering, Curtin University. His research interests include operations and production management, resilience engineering, infrastructure development and digitisation in construction. His research has been published in leading scholarly journals such as the European Journal of Operations Research, Journal of Management Information Systems, Journal of Management Studies, IEEE Transactions on Engineering Management, International Journal of Operations and Production Management, Production Planning and Control, and Transportation Research A: Policy and Practice. Professor Love holds a Higher Doctor of Science (Curtin 2012) for his contributions to the field of civil and construction engineering.

Jane Matthews
Jane Matthews received a Ph.D. in architecture from the University of East London, London, U.K., in 2001. She is a Professor of Digital Construction at the School of Architecture and Built Environment, Deakin University, Victoria, Australia. She has ten years of industry experience as a Software Design and Development Manager for the Royal Institute of British Architects. Professor Matthews has published extensively in leading scholarly journals, which include Automation in Construction, ASCE Journal of Construction Engineering and Management, Production Planning and Control, Transportation Research A: Policy and Practice, and IEEE Transactions on Engineering Management and Reliability Engineering and System Safety. Her research interests include the management and visualisation of information in construction.

Lavagnon A. Ika
Lavagnon A. Ika received a Ph.D. in project management from the Université du Quebec, QC, Canada, in 2011. He is a professor of project management and the program director for the M.Sc. in management at the Telfer School of Management, University of Ottawa, Ottawa, ON, USA. He is affiliated with the School of International Development and Global Studies, University of Ottawa. He has a keen interest in international development projects. His work has appeared in leading journals such as World Development, the IEEE Transactions on Engineering Management, Production Planning and Control, Transportation Research Part A: Policy and Practice, the International Journal of Project Management, the Project Management Journal, the European Management Review, and the Journal of African Business. His research interests include: what makes projects complex; what makes projects successful; why do projects fail and what can be done about it; why projects experience cost overruns and benefit shortfalls; how do projects really “behave” or work; and what is the role of strategy, supervision and management in project success/failure.
Notes
1 Reference class forecasting is a method of predicting the future by looking at similar past situations and their outcomes (Flyvbjerg Citation2008).
2 For example, refer to the UK’s HM Treasury’s (2022) “Greenbook” and Waka Kotahi NZ Transport Agency’s (2023) “Cost Estimation Manual (SM014)”, to name a few.
3 Ambiguity aversion is the tendency to favor the known over the unknown, including known risks over unknown risks. So, in this case, public sector agencies will be uncomfortable with situations in which there is incomplete or vague information about the probabilities associated with events impacting a project’s actual construction costs.
4 For example, drift deposits and the disintegrated brecciated [sedimentary rock type] bedrock.
5 Refer to “HS2: The £100BN Railway Dividing a Nation” Available at: https://www.youtube.com/watch?v=FSD5ps9bLQ0.
6 At target outturn cost is “a specific sum estimated as being the pre-estimate of Reimbursable Costs, Risk & Contingency Provisions, Corporate Overhead and Profit to achieve the minimum conditions of satisfaction, perform the Works and bring the Works to final completion (i.e., following completion of the defects liability period)” (Department of Infrastructure & Regional Development Citation2015a: p.12).
7 Strategic misrepresentation “is the planned, systematic distortion or misstatement of fact-lying-in response to incentives in the budget process” (Jones and Euske Citation1991: p.174).
8 For further information refer to “Secret recordings expose multibillion-pound HS2 cover-up”, Times Radio, 24th October 2023, available at “https://www.youtube.com/watch?v=O77wvGRSdSM”.
9 Several cost estimate classifications exist such as produced by the: (1) American Society of Professional Estimators (Citation2021) Level 1 – Order (Range) of Magnitude, Level 2 – Schematic/Conceptual Design, Level 3- Design Development, Level 4 – Construction Document, and Level 5 – Bid; and (2) Association for the Advancement of Cost Engineering (Citation2020) which uses the following definitions to express the level of project’s definition– Class 5 (0-2%), Class 4 (1 to 15%), Class 3 (10 to 40%), Class 2 (30-70%) and Class 1 (50-100%).
10 A contract where the amount to be paid for the work carried out is based on the remeasurement of work after it has been carried out, usually against a schedule of rates contained in the contract.
11 In New Zealand a Resource Consent is permission from the local council for an activity that might affect the environment.
12 The classic view of rationality views reasoning and behaviour as rational when they conform to the norms of logic, statistics, and probability theory (Marewski and Gigerenzer Citation2012).
13 We do not discount the presence of optimism bias, quite the contrary. However, there is a lack of empirical evidence of its presence within the formulation of cost estimates developed by a group of people and checked by independent organizations.
14 Best-for-project refers to the “determination, decision, outcome, solution, or resolution which is consistent with the aim of ensuring that the works are fit for their intended purpose and constitute value for money to the State, consistent with the stated objectives of an alliance rather than the achievement of self-serving goals (such as maximising a Non-Owner Participants’ (NOP) profit)” (www.lawinsider.com/dictionary/best-for-project) (Department of Infrastructure& Regional Development, 2015b).
References
- Almaaz, R., I. Zakaria, and T. C. Odimegwumas. 2020. “Comparative Analysis of Alliancing and Public-Private Partnership Cost Performance in Australasia Infrastructure Projects”. Proceedings of the.” IOP Conference Series: Earth and Environmental Science 498 (1): 012102. https://doi.org/10.1088/1755-1315/498/1/0122102.
- American Society of Professional Estimators. 2021. Principles of Estimating: General Principles of Estimating. Volume 1, Standard Estimating Practice. 11th ed. Vista, CA: BNi Publications Inc.
- Andrić, J. M., A.-M. Mahamadu, J. Wang, P. X. W. Zou, and R. Zhong. 2019. “The Cost Performance and Causes of Overruns in Infrastructure Development Projects in Asia.” Journal of Civil Engineering and Management 25 (3): 203–214. https://doi.org/10.3846/jcem.2019.8646.
- Association for the Advancement of Cost Engineering. 2020. “Cost Estimate Classification System.” AACE International Recommended Practice No.17R-97, TCM Framework; 7.3 – Cost Estimation and Budgeting, August 7. https://web.aacei.org/
- Australian Constructors Association. 2017. “Changing the Game: How Australia can Achieve Success in the New World of Megaprojects.” Accessed April 5. https://www.constructors.com.au/wp-content/uploads/2015/11/Changing-the-Game-Mega-Projects-Final1.pdf
- Australian Constructors Association. 2021. “Inquiry in Procurement Practices for Government-Funded Infrastructure House of Representatives Standing Committee on Infrastructure, Australian Constructors Association Submission 16th July.” https://www.constructors.com.au/wp-content/uploads/2021/07/ACA-Procurement-Inquiry-Submission-16-July-2021-1.pdf
- Baccarini, D., and P. E. D. Love. 2014. “Statistical Characteristics of Cost Contingency in Water Infrastructure Projects.” Journal of Construction Engineering and Management 140 (3). https://doi.org/10.1061/(ASCE)CO.1943-7862.0000820open_in_new.
- Batselier, J., and M. Vanhoucke. 2016. “Practical Application and Empirical Evaluation of Reference Class Forecasting for Project Management.” Project Management Journal 47 (5): 36–51. https://doi.org/10.1177/875697281604700504.
- Baum, R. J., and S. Wally. 2003. “Strategic Decision Speed and Firm Performance.” Strategic Management Journal 24 (11): 1107–1129. https://doi.org/10.1002/smj.343.
- Blass, A. A., S. Lach, and C. F. Manski. 2010. “Using Elicited Choice Probabilities to Estimate Random Utility Models: Preferences for Electricity Reliability.” International Economic Review 51 (2): 421–440. https://doi.org/10.1111/1477-9552.12398.
- Bordat, C., B. G. McCullouch, S. Labi, and K. C. Sinha. 2004. “An Analysis of Cost Overruns and Time Delays of INDOT Projects” Transportation Research. Joint Transportation Research Program Purdue University. https://doi.org/10.5703/1288284313134.
- Brunswik, E. 2001. “Scope and Aspects of the Cognitive Problem.” In The Essential Brunswik: Beginnings, Explications, Applications, edited by K. R. Hammond and T. R. Stewart, 300–312. New York, NY: Oxford University Press.
- Caffieri, J. J., P. E. D. Love, A. Whyte, and D. A. Ahiaga-Dagbui. 2018. “Planning for Production in Construction: Controlling Costs in Major Capital Projects.” Production Planning & Control 29 (1): 41–50. https://doi.org/10.1080/09537287.2017.1376258.
- Calvert, J., and G. Arbuthnott. 2023. “The secret files that expose a multi-billion pound cover-up at HS2”. Sunday Times, 21st October. Accessed October 21, 2023. https://www.thetimes.co.uk/article/hs2-billion-pound-cover, p-cost-files-investigation-skzv2nxwj. Also available on YouTube, “Secret recordings expose multibillion-pound HS2 cover-up, Times Radio, October 24, 2023.
- Camus, A. 1942. Le Mythe De Sisyphe (the Myth of Sisyphus). France: Gallimard.
- Cantarelli, C. C., B. Flyvbjerg, and S. L. Buhl. 2012. “Geographical Variation in Project Cost Performance: The Netherlands Versus Worldwide.” Journal of Transport Geography 24: 324–331. https://doi.org/10.1016/j.jtrangeo.2012.03.014.
- Catalão, F. P., C. O. Cruz, and J. M. Sarmento. 2019. “The Determinants of Cost Deviations and Overruns in Transport Projects, an Endogenous Model’s Approach.” Transport Policy 74: 224–238. https://doi.org/10.1016/j.tranpol.2018.12.008.
- Cavalieri, M., R. Cristaudo, and C. Guccio. 2019. “On the Magnitude of Cost Overruns throughout the Project Life Cycle: An Assessment for the Italian Transport Infrastructure Projects.” Transport Policy 74: 21–36. https://doi.org/10.1016/j.tranpol.2019.04.001.
- Chong, U., and O. Hopkins. 2016. “An International Experience on the Evolution of Road Costs during the Project Life Cycle.” Transport Policy 48: 60–66. https://doi.org/10.1016/j.tranpol.2016.02.010.
- Clark, F. D., and A. B. Lorenzoni. 1985. Applied Cost Engineering. New York: Dekker.
- Davidson, P. 1991. “Is Probability Theory Relevant for Uncertainty? A Post-Keynesian Perspective.” Journal of Economic Perspectives 5 (1): 129–143. https://doi.org/10.1257/jep.5.1.129.
- Department of Infrastructure & Regional Development. 2015a. National Alliance Contracting Guidelines: Guidance Note 3 Key Risk Areas and Trade-Offs, September, Canberra, ACT, Australia. Accessed November 5, 2023. https://www.infrastructure.gov.au/sites/default/files/migrated/infrastructure/ngpd/files/NACG_GN3.pdf
- Department of Infrastructure and Regional Development. 2015b. National Alliance Contracting Guidelines Template 1 Project Alliance Agreement - Template 1 Project Alliance Agreement. Department of Infrastructure and Regional Development A. C. G. Canberra, Commonwealth of Australia, Australia. Accessed November 7, 2023. https://www.infrastructure.gov.au/sites/default/files/migrated/infrastructure/ngpd/files/Template_1_PAA.pdf
- Edwards, C., and N. Kaeding. 2015. Federal Government Cost Overruns. Cato Institute Tax and Budget Bulletin, 72. Accessed October 14, 2023. www.cato.org/sites/cato.org/files/pubs/pdf/tbb-727.pdf
- Eliasson, J., and M. Fogerau. 2013. “Cost Overruns and Demand Shortfalls – Deception or Selection.” Transportation Research B: Methodological 57: 105–113. https://doi.org/10.1016/j.trb.2013.09.005.
- Fischhoff, B., N. Welch, and S. Frederick. 1999. “Construal Processes in Preference Assessment.” Journal of Risk and Uncertainty 19 (1/3): 139–164. https://doi.org/10.1023/A:1007823326511.
- Flyvbjerg, B. 2008. “Curbing Optimism Bias and Strategic Misrepresentation in Planning: Reference Class Forecasting in Practice.” European Planning Studies 16 (1): 3–21. https://doi.org/10.1080/09654310701747936.
- Flyvbjerg, B. 2011. “Over Budget, Over Time, Over and Over Again: Managing Major Projects.” In The Oxford Handbook of Project Management, edited by P. G. Morris, J. K. Pinto, and J. Söderlund, 321–344. Oxford, UK: Oxford University Press. https://doi.org/10.1093/oxfordhb/9780199563142.003.0014.
- Flyvbjerg, B. 2012. “Quality Control and Due Diligence in Project Management: Getting Decision Right by Taking the outside View.” International Journal of Project Management 31 (5): 760–774. https://doi.org/10.1016/j.ijproman.2012.10.007.
- Flyvbjerg, B. 2016. “The Fallacy of Beneficial Ignorance: A Test of Hirschman’s Hiding Hand.” World Development 84: 176–189. https://doi.org/10.1016/j.worlddev.2016.03.012.
- Flyvbjerg, B., A. Ansar, A. Budzier, S. Buhl, C. Cantarelli, M. Garbuio, C. Glenting, et al. 2018. “Five Things You Should Know about Cost Overruns.” Transportation Research Part A: Policy and Practice 118: 174–190. https://doi.org/10.1016/j.tra.2018.07.013.
- Flyvbjerg, B., M. S. Holm, and S. Buhl. 2002. “Underestimating Costs in Public Works Projects: error or Lie?” Journal of the American Planning Association 68 (3): 279–295. https://doi.org/10.1080/01944360208976273.
- Flyvbjerg, B, COWI. 2004. Procedures for Dealing with Optimism Bias in Transport Planning. Guidance Document, The British Department of Transport, Report No. 58924, Issue 1, 10th June. Accessed February 27, 2021. https://assets.publishing.service.gov.uk/government/uploads/system/uploads/attachment_data/file/191523/Procedures_for_dealing_with_optimism_bias_in_transport_planning.pdf
- Fu, Y., and N. Gil. 2024. “Financial Buffers in Megaprojects: A Contingent Model of Joint Value Production and Policy Implications.” IEEE Transactions on Engineering Management 1–17. https://doi.org/10.1109/TEM.2023.3308163.
- Gigerenzer, G. 2008. “Why Heuristics?” Perspectives on Psychological Science 3 (1): 20–29. https://doi.org/10.1111/j.1745-6916.2008.00058.x.
- Gigerenzer, G., and H. Brighton. 2009. “Homo Heuristicus: Why Biased Minds Make Better Inferences.” Topics in Cognitive Science 1 (1): 107–143. https://doi.org/10.1111/j.1756-8765.2008.01006x.
- Gigerenzer, G., and W. Gaissmaier. 2011. “Heuristic Decision Making.” Annual Review of Psychology 62 (1): 451–482. https://doi.org/10.1146/annurev-psych-120709-145346.
- Gigerenzer, G., and R. Garcia-Retamero. 2017. “Cassandra’s Regret: The Psychology of Not Wanting to Know.” Psychological Review 124 (2): 179–196. https://doi.org/10.1037/rev0000055.
- Gigerenzer, G., and D. G. Goldstein. 1996. “Reasoning the Fast and Frugal Way: Models of Bounded Rationality.” Psychological Review 103 (4): 650–669. https://doi.org/10.1037/0033-295X.104.4.650.
- Gigerenzer, G., J. Reb, and S. Luan. 2022. “Smart Heuristics for Individuals, Teams, and Organizations.” Annual Review of Organizational Psychology and Organizational Behavior 9 (1): 171–198. https://doi.org/10.1146/annurev-orgpsych-012420-090506.
- Gigerenzer, G., P. M. Todd, and ABC Research Group. 1999. Simple Heuristics That Make Us Smart. New York, NY: Oxford University Press.
- Gigernezer, G. 2014. Risk Savvy: How to Make Good Decisions. London, UK: Penguin Group.
- Haaskjold, H., B. Andersen, and J. A. Langlo. 2023. “Dissecting the Project Anatomy: Understanding the Cost of Managing Construction Projects.” Production Planning & Control 34 (2): 117–138. https://doi.org/10.1080/09537287.2021.1891480.
- Hakimian, R. 2023. “Reaction to Cancellation of HS2: Disappointed and Disheartened”. Accessed October 14, 2023. https://www.newcivilengineer.com/latest/reaction-to-cancellation-of-hs2-disappointed-and-disheartened-04-10-2023/
- Hansson, S. O. 2022. “Can Uncertainty Be Quantified?” Perspectives on Science 30 (2): 210–236. https://doi.org/10.1162/posc_a_00412.
- Hayford, O. 2018. Collaborative Contracting. PWC, March. Accessed November 11, 2023. https://www.pwc.com.au/legal/assets/collaborative-contracting-mar18.pdf
- Hertwig, R., C. Leuker, T. Pachur, L. Spiliopoulos, and T. J. Pleskac. 2022. “Studies in Ecological Rationality.” Topics in Cognitive Science 14 (3): 467–491. https://doi.org/10.1111/tops.12567.
- Hinze, J., G. Selstead, and J. P. Mahoney. 1992. “Cost Overruns on State of Washington Construction Contracts.” Transportation Research Record 1351: 87–93.
- Hinze, J., G. Selstad, and J. P. Mahoney. 1991. Analysis of WSDOT Construction Cost Overruns. RP GC 8719, Task 12, WA-RD 218.1, prepared for Washington State Transportation Committee, Department of Transportation and the US Transportation Federal Highway Administration. http://www.wsdot.wa.gov/research/reports/fullreports/218.1.pdf
- Hirschman, A. O. 1967. “The Principle of the Hiding Hand”. The Public Interest, Winter, 10–23.
- HM Treasury. 2022. The Green Book. Accessed October 20, 2023. https://www.gov.uk/government/publications/the-green-book-appraisal-and-evaluation-in-central-governent/the-green-book-2020
- Hourigan, W. 2022. Report of the Ottawa Light Rail Transit Public Inquiry. Ottawa Rail Transit Commission, 30th November, Toronto, Ottawa, Canada. Accessed December 8, 2023. https://www.archives.gov.on.ca/en/e_records/OLRTPI/files/documents/Report-of-the-Ottawa-Light-Rail-Transit-Public-Inquiry.pdf
- Ika, L. A. 2018. “Beneficial or Detrimental Ignorance: The Strawman Fallacy of Flyvbjerg’s Test of Hirschman’s Hiding Hand.” World Development 103: 369–382. https://doi.org/10.1016/j.worlddev.2017.10.016.
- Ika, L. A., P. E. D. Love, and J. K. Pinto. 2022. “Moving beyond the Planning Fallacy: The Emergence of a New Principle of Project Behavior.” IEEE Transactions on Engineering Management 69 (6): 3310–3325. https://doi.org/10.1109/TEM.2020.3040526.
- Ika, L. A., and J. K. Pinto. 2022. “The Re-Meaning of Project Success: Updating and Re-Calibrating for Modern Project Management.” International Journal of Project Management 40 (7): 835–848. https://doi.org/10.1016/j.ijproman.2022.08.001.
- Ika, L., J. K. Pinto, P. E. D. Love, and G. Pache. 2023. “Bias versus Error: Why Projects Fall Short.” Journal of Business Strategy 44 (2): 67–75. https://doi.org/10.1108/JBS-11-2021-0190.
- Ionesco, E. 1954. The Bold Soprano (La Cantatrice Chauve Suivi de La Leçon). Paris, France: Gallimard.
- Joint Legislative Audit and Review Commission (JLARC). 2001. Review of Construction Costs and Time Schedules for Virginia Highway Projects: A Report in a Series on Transportation Issues in Virginia, House Document N. 31, a Report in a Series on Transportation Issues in Virginia. Accessed November 2, 2023. http://jlarc.virginia.gov/pdfs/reports/Rpt258.pdf
- Jones, L. R., and K. J. Euske. 1991. “Strategic Misrepresentation in Budgeting.” Journal of Public Administration Research and Theory 1 (4): 437–460. https://doi.org/10.1093/oxfordjournals.jpart.a037102.
- Jørgensen, M., M. Welde, and T. Halkjelsvik. 2023. “Evaluation of Probabilistic Project Cost Estimates.” IEEE Transactions on Engineering Management 70 (10): 3481–3496. https://doi.org/10.1109/TEM.2021.3067050.
- Kafka, F. 1916. The Metamorphosis. Leipzig, Germany: Kurt Wolff Verlag.
- Kahneman, D. 2011. Thinking, Fast and Slow. New York: Farrar, Straus & Giroux.
- Kim, B. C., and Y. H. Kwak. 2018. “Improving the Accuracy and Operational Predictability of Project Cost Forecasts: An Adaptive Combination Approach.” Production Planning & Control 29 (9): 743–760. https://doi.org/10.1080/09537287.2018.1467511.
- Knight, F. 1921. Risk, Uncertainty, and Profit. Mineola, NY: Dover Publications.
- Kostka, G., and N. Anzinger. 2016. “Large Infrastructure Projects in Germany: A Cross-Sectoral Analysis.” In Large Infrastructure Projects in Germany, edited by G. Kostka, and J. Fiedler. Cham: Palgrave Macmillan. https://doi.org/10.1007/978-3-319-29233-5_2.
- Kreiner, K. 2020. “Conflicting Notions of a Project: The Battle between Albert O. Hirschman and Bent Flyvbjerg.” Project Management Journal 51 (4): 400–410. https://doi.org/10.1177/8756972820930535.
- Kutsch, E., and M. Hall. 2010. “Deliberate Ignorance in Project Risk Management.” International Journal of Project Management 28 (3): 245–255. https://doi.org/10.1016/j.ijproman.2009.05.003.
- Lee, J.-K. 2008. “Cost Overrun and Cause in Korean Social Overhead Capital Projects: Roads, Rails, Airports, and Ports.” Journal of Urban Planning and Development 134 (2): 59–62. https://doi.org/10.1061/(ASCE)0733-9488(2008)134:2(59).
- Liu, H. J., P. E. D. Love, J. Zhao, C. Lemckert, and K. Muldoon-Smith. 2021. “Transport Infrastructure Asset Resilience: Managing Government Capabilities.” Transportation Research Part D: Transport and Environment 100: 103072. https://doi.org/10.1016/j.trd.2021.103072.
- Love, P. E. D., and L. A. Ika. 2021. “The Context of Transport Project Cost Performance: Insights from Contract Award to Final Construction Costs.” Research in Transportation Economics 90: 101062. https://doi.org/10.1016/j.retrec.2021.101062.
- Love, P. E. D., C.-P. Sing, X. Wang, Z. Irani, and D, W. Thwala. 2014. “Overruns in Transportation Infrastructure Projects.” Structure and Infrastructure Engineering 10 (2): 141–159. https://doi.org/10.1080/15732479.2012.715173.
- Love, P. E. D., C.-P. Sing, B. Carey, and J.-T. Kim. 2015a. “Estimating Construction Contingency: Accommodating the Potential for Cost Overruns in Road Construction Projects.” Journal of Infrastructure Systems 21 (2): 04014035. https://doi.org/10.1061/(ASCE)IS.1943-555X.0000221.
- Love, P. E. D., J. Smith, I. Simpson, M. Regan, and O. Olatunji. 2015b. “Understanding the Landscape of Overruns in Transport Infrastructure Projects.” Environment and Planning B: Planning and Design 42 (3): 490–509. https://doi.org/10.1068/b130102p.
- Love, P. E. D., J. Zhou, D. J. Edwards, Z. Irani, and M. C. P. Sing. 2017a. “Off the Rails: The Cost Performance of Infrastructure Rail Projects.” Transportation Research Part A: Policy and Practice 99: 14–29. https://doi.org/10.1016/j.tra.2017.02.008.
- Love, P. E. D., D. Ahiaga-Dagbui, M. Welde, and J. Odeck. 2017b. “Light Rail Transit Cost Performance: Opportunities for Future-Proofing.” Transportation Research Part A: Policy & Practice 100: 27–39. https://doi.org/10.1016/j.tra.2017.04.002.
- Love, P. E. D., Z. Irani, J. Smith, M. Regan, and J. Liu. 2017c. “Cost Performance of Public Infrastructure Projects: The Nemesis and Nirvana of Change-Orders.” Production Planning & Control 28 (13): 1081–1092. https://doi.org/10.1080/09537287.2017.1333647.
- Love, P. E. D., L. A. Ika, and D. D. Ahiaga-Dagbui. 2019a. “On de-Bunking’ Fake News’ in a Post-Truth Era: Why Does the Planning Fallacy Explanation for Cost Overruns and Benefit Shortfalls Fall Short?” Transportation Research Part A: Policy & Practice 126: 397–408. https://doi.org/10.1016/j.tra.2019.06.013.
- Love, P. E. D., M. C. P. Sing, L. A. Ika, and S. Newton, 2019b. “The Cost Performance of Transportation Projects: The Fallacy of the Planning Fallacy account.” Transportation Research Part A: Policy & Practice 122: 1–20. https://doi.org/10.1016/j.tra.2019.02.004.
- Love, P. E. D. L. A. Ika, J. Matthews, and W. Fang, 2021a. “Shared Leadership, Value, and Risks in Large Scale Transport Projects: Re-Calibrating Procurement Policy for post-COVID-19.” Research in Transportation Economics 90: 100999. https://doi.org/10.1016/j.retrec.2020.100999.
- Love, P. E. D., L. A. Ika, J. Matthews, and W. Fang. 2021b. “Risk and Uncertainty in the Cost Contingency of Transport Projects: Accommodating Bias or Heuristics, or Both?” IEEE Transactions on Engineering Management. https://doi.org/10.1109/TEM.2021.3119064.
- Love, P. E. D., J. K. Pinto, and L. A. Ika. 2022. “Hundreds of Years of Pain, with Minimal Gain: Capital Project Cost Overruns, the Past, Present, and Optimistic Future.” IEEE Engineering Management Review 50 (4): 56–70. https://doi.org/10.1109/EMR.2022.3219362.
- Love, P. E. D., L. A. Ika, and JK. Pinto. 2023a. “Smart Heuristics for Decision-Making in the 'Wild’: Navigating Cost Uncertainty in the Construction of Large-Scale Transport Projects.” Production Planning & Control 1–18. https://doi.org/10.1080/09537287.2023.2248942.
- Love, P. E. D., L. A. Ika, and J. K. Pinto. 2023b. “Fast-and-Frugal Heuristics for Decision-Making in Uncertain and Complex Settings in Construction.” Developments in the Built Environment 14: 100129. https://doi.org/10.1016/j.dibe.2023.100129.
- Love, P. E. D., L. A. Ika, J. Matthews, and W. Fang. 2023c. “Large-Scale Transport Infrastructure Project Performance: Generating a Narrative of Context and Meaning.” IEEE Transactions on Engineering Management 70 (10): 3637–3652. https://doi.org/10.1109/TEM.2021.3094511.
- Love, P. E. D., L. A. Ika, and J. K. Pinto. 2024. “Homo Heuristicus: From Risk Management to Managing Uncertainty in Large-Scale Infrastructure Projects.” IEEE Transactions on Engineering Management 71: 1940–1949. https://doi.org/10.1109/TEM.2022.3170474.
- Luan, S., J. Reb, and G. Gigerenzer. 2019. “Ecological Rationality: Fast-and-Frugal Heuristics for Managerial Decision Making under Uncertainty.” Academy of Management Journal 62 (6): 1735–1759. https://doi.org/10.5465/amj.2018.0172.
- Ludlow, M. 2023. Fears energy projects could push road and rail out of $894b pipeline. Financial Review, 31st July. Accessed October 14, 2023. https://www.afr.com/companies/energy/fears-energy-projects-could-push-roads-rail-out-of-894b-pipeline-20230727-p5drt6
- Makovšek, D., P. Tominc, and K. Logožar. 2012. “A Cost Performance Analysis of Transport Infrastructure Construction in Slovenia.” Transportation 39 (1): 197–214. https://doi.org/10.1007/s11116-011-9319-z.
- Mak, S., and J. Rafterty. 1992. “Risk Attitude and Systemic Bias in Estimating and Forecasting.” Construction Management & Economics 10 (4): 303–320. https://doi.org/10.1080/01446199200000028.
- Mak, S., J. Wong, and P. Picken. 1998. “The Effect on Contingency Allowances of Using Risk Analysis in Capital Cost Estimating: A Hong Kong Case Study.” Construction Management and Economics 16 (6): 615–619. https://doi.org/10.1080/014461998371917.
- Marewski, J. N., and G. Gigerenzer. 2012. “Heuristic Decision Making in Construction.” Dialogues in Clinical Neuroscience 14 (1): 77–89. https://doi.org/10.31887/DCNS.2012.14.1/jmarewski.
- Meder, B., F. Le Lec, and M. Osman. 2013. “Decision-Making in Uncertain Times: What Can Cognitive and Decision Sciences Say about or Learn from Economic Crises?” Trends in Cognitive Science,17 (6): 257–260. https://doi.org/10.1016/j.tics.2013.04.008.
- Merewitz, L. 1973. Cost Overruns in Public Works. Reprint No. 114 Institute of Urban and Regional Development. Berkeley, CA: The University of California.
- Meunier, D., and M. Welde. 2017. “Ex-Post Evaluation in Norway and France.” Transportation Research Procedia 26: 144–155. https://doi.org/10.1016/j.trpro.2017.07.015.
- Molinari, L., E. Haezendonck, and V. Mabillard. 2023. “Cost Overruns of Belgian Transport Infrastructure Projects: Analyzing Variations over Three Land Transport Modes and Two Project Phases.” Transport Policy 134: 167–179. https://doi.org/10.1016/j.tranpol.2023.02.017.
- Morris, S. 1990. “Cost and Time Overruns in Public Sector Projects.” Economic and Political Weekly 25 (47): M154–M168.
- Mousavi, S., and G. Gigerenzer. 2014. “Risk, Uncertainty, and Heuristics.” Journal of Business Research 67 (8): 1671–1678. https://doi.org/10.1016/j.busres.2014.02.2013.
- National Audit Office. 2007. Estimating and Monitoring the Costs of Building Roads in England. Report – Value for money, Department for Transport. https://www.nao.org.uk/reports/department-for-transport-estimating-and-monitoring-the-costs-of-building-roads-in-england/
- Neth, H., Meder, Kothiyal A, and Gigerenzer, G. 2014. “Homo Heuristicus in the Financial World: From Risk Management to Managing Uncertainty.” Journal of Risk Management in Financial Institutions 7 (2): 134–144.
- New South Wales Auditor-General’s Report. 2016. Performance Audit CBD and Southeast Light Rail Project Transport for NSW, Audit Office of New South Wales, Sydney, Australia. Accessed November 1, 2023. https://www.audit.nsw.gov.au/sites/default/files/pdf-downloads/2016_Nov_Report__CBD_and_South_East_Light_Rail_Project_0.pdf
- Norris, E., and G. Tetlow. 2023. HS2 is a fiasco of change and churn. Institute for Government, 5th October. Accessed November 1, 2023. https://www.instituteforgovernment.org.uk/comment/hs2-fiasco-change-churn
- Nottelmann, N. 2015. “Ignorance.” In Cambridge Dictionary of Philosophy, edited by R. Audi, 3rd ed. Cambridge, UK: Cambridge University Press.
- O’Toole, R. 2015. Rail Transit Cost Overruns. The Antiplanner, 19th January. Accessed November 1, 2023. https://ti.org/antiplanner/?p=10036
- Oakervee, D. 2020. Oakervee Review of HS2. The Department of Transport and High-Speed Two (HS2) Limited, 11th February. Accessed November 1, 2023. Available at: https://www.gov.uk/government/publications/oakervee-review-of-hs2.
- Odeck, J. 2004. “Cost Overruns in Road Construction – What Are Their Sizes and Determinants?” Transport Policy 11 (1): 43–53. https://doi.org/10.1016/S0967-070X(03)00017-9.
- Pettitt, J., S. Boyo, and A. Evers. 2023. Why is it taking California so long to complete its high-speed rail? CNBC, 17th May. Accessed April 5, 2024. https://www.cnbc.com/video/2023/05/17/why-it-is-taking-california-so-long-to-complete-its-high-speed-rail.html
- Pickrell, D. H. 1990. Urban Rail Transit Projects: Forecast versus Actual Ridership and Costs. Final Report by Transportation Systems Center, Cambridge, Massachusetts, Prepared for the US Department of Transportation. Washington, DC, USA. Accessed November 5, 2023. https://ntl.bts.gov/lib/56000/56600/56645/
- Pinter, H. 1959. The Birthday Party. London, UK: Encore Publishing.
- Pinto, J. K. 2023. “Is This How Things Get Done?” International Journal of Project Management 41 (5): 102484. https://doi.org/10.1016/j.ijproman.2023.102484.
- Public Accounts Committee. 2023. Comment: HS2. UK Parliament. Accessed October 5, 2023. https://committees.parliament.uk/committee/127/public-accounts-committee/news/197713/comment-hs2/
- Sharot, T. 2011. “The Optimism Bias.” Current Biology 21 (23): R941–R945. https://doi.org/10.1016/j.cub.2011.10.030.
- Simon, H. A. 1955. “A Behavioral Model of Rational Choice.” The Quarterly Journal of Economics 69 (1): 99–118. https://doi.org/10.2307/1884852.
- Simon, H. A. 1956. “Rational Choice and the Structure of the Environment.” Psychological Review 63 (2): 129–138. https://doi.org/10.1037/h0042769.
- Simon, H. A. 1990. “Invariants of Human Behavior.” Annual Review of Psychology 41 (1): 1–20. https://doi.org/10.1146/annurev.psych.41.1.1.
- Tam, N. 2015. Aecom Settles Australia Toll Road Lawsuit. Infrastructure Investor. October 2, 2015. Accessed October 31, 2023. https://www.infrastructureinvestor.com/aecom-settles-australia-toll-road-lawsuit/
- Terrill, M., and L. Danks. 2016. Cost Overruns in Transport Projects. Grattan Institute, Melbourne, Australia. Accessed June 6, 2022. https://grattan.edu.au/wp-content/uploads/2016/10/878-Cost-overruns-on-transport-infrastructure.pdf
- Terrill, M., and O. Emslie. 2021. "Getting Infrastructure Megaproject Cost Estimates Right". Infrastructure Magazine, 2nd June. Accessed November 1. https://infrastructuremagazine.com.au/2021/06/02/getting-infrastructure-megaproject-cost-estimates-right/
- Terrill, M., O. Emslie, and G. Moran. 2020. The Rise of Megaprojects: Counting the Costs. Grattan Institute, November. Accessed October 27, 2023. https://grattan.edu.au/wp-content/uploads/2020/11/The-Rise-of-Megaprojects-Grattan-Report.pdf.
- Tillet, A. 2023. “Road Rail Projects Could Be Axed from $120b Pipeline.” Australian Financial Review, May 1. Accessed October 12, 2023. https://www.afr.com/politics/federal/road-rail-projects-could-be-axed-from-120b-pipeline-20230430-p5d4bs
- Todd, P. M., and G. Gigerenzer. 2000. “Precis of Simple Heuristics That Make Us Smart.” Behavioral and Brain Sciences 23 (5): 727–741. https://doi.org/10.1017/S0140525X00003447.
- Todd, P. M., and H. Brighton. 2016. “Building the Theory of Ecological Rationality.” Minds and Machines 26 (1-2): 9–30. https://doi.org/10.1007/s11023-015-9371-0.
- Traynor, B. A., and M. Mahmoodian. 2019. “Time and Cost Contingency Management Using Monte Carlo Simulation.” Australian Journal of Civil Engineering 17 (1): 11–18. https://doi.org/10.1080/14488353.2019.1606499.
- Tseng, C.-L., T. Zhao, and C. C. Fu. 2009. “Contingency Estimation Using a Real Options Approach.” Construction Management and Economics 27 (11): 1073–1087. https://doi.org/10.1080/01446190903222411.
- Vartabedian, R. 2022. How California’s bullet train went off the rails. New York Times. Accessed April 5, 2024. https://www.nytimes.com/2022/10/09/us/california-high-speed-rail-politics.html
- Verweji, S., I. F. Van Meerkrek, and I. A. Korthagan. 2015. “Reasons for Contract Changes in Implementing Dutch Transportation Infrastructure Projects: An Empirical Exploration.” Transport Policy 37 (1): 195–202. https://doi.org/10.1016/j.tranpol.2014.11.004.
- Vidalis, S. M., and F. T. Najafi. 2002. “Cost and Time Overruns in Highway Construction.” In CSCE 30th Annual Conference Proceedings: 2002 Challenges Ahead - 4th Structural Specialty Conference, 4th Transportation Specialty Conference and 2nd material Specialty Conference, 2799–2808. Vol. 2002. https://pure.psu.edu/en/publications/cost-and-time-overruns-in-highway-construction
- Vis, B. 2024. “Introducing a Conceptual Map of Political Elites’ Responses to Different Types of Uncertain Phenomena.” Political Studies Review. https://doi.org/10.1177/14789299231222847.
- Walker, D. H. T., and A. McCann. 2020. “IPD and TOC Development.” In The Routledge Handbook of Integrated Project Delivery, edited by D. H. T. Walker and S. Rowlinson, 581–604. Abingdon, Oxon: Routledge.
- Walker, D. H. T., and B. M. Lloyd-Walker. 2015. Collaborative Project Procurement Arrangements. Newtown Square, PA: Project Management Institute.
- Walker, D. H. T., J. Harley, and A. Mills. 2013. “Longitudinal Study of Performance in Large-Scale Public-Sector Infrastructure Alliances 2008-2013.” Centre for Integrated Project Solutions, School of Property, Construction and Project Management. Accessed November 11, 2023. https://cms.qut.edu.au/__data/assets/pdf_file/0020/329060/alliance-case-study-report.pdf
- Walker, D. H. T., P. E. D. Love, and J. Matthews. 2023. “Generating Value in Program Alliances: The Value of Dialogue in Large-Scale Infrastructure Projects.” Production Planning & Control 1–16. https://doi.org/10.1080/09537287.2023.2202631.
- Walker, D. H. T., Vaz-Serra, P. Love, and P. E. D. 2022. “Improved Reliability in Planning Large-Scale Project Delivery through Alliancing.” International Journal of Managing Projects in Business 15 (5): 721–741. https://doi.org/10.1108/IJMPB-02-2022-0035.
- Waka Kotahi NZ Transport Agency. 2023. “Cost Estimation Manual (SM014). Transport Services, Second Edition.” Amendment 1, March 2023, New Zealand Government, Wellington, NZ, November 6, 2023. https://www.nzta.govt.nz/resources/cost-estimation-manual/
- Weigle, G., and S. Garber. 2010. “Integrated Project Delivery (IPD) and Project Target Cost Estimate (PTCE) Relative to Traditional Project Delivery and Guaranteed Maximum Price (GMP).” Facility Care 15 (2): 10–13.
- Welde, M., and G. H. Volden. 2022. “Improving Value for Money through Better Front-End Management: An Attempt to Reduce Costs and Increase User Benefits in Planning a Motorway Project.” Case Studies on Transport Policy 10 (3): 1611–1619. https://doi.org/10.1016/j.cstp.2022.05.019.
- Welde, M., and J. Odeck. 2017. “Cost Escalations in the Front-End of Projects – Empirical Evidence from Norwegian Road Projects.” Transport Reviews 37 (5): 612–630. https://doi.org/10.1080/01441647.2016.1278285.
- Welde, M., and O. J. Klakegg. 2024. “Avoiding Cost Overrun through Stochastic Cost Estimation and External Quality Assurance.” IEEE Transactions on Engineering Management 71: 1984–1997. https://doi.org/10.1109/TEM.2022.3173175.
- Welde, M., and R. E. Dahl. 2021. “Cost Escalation in Road Construction Contracts.” Transportation Research Record: Journal of the Transportation Research Board 2675 (9): 1006–1015. https://doi.org/10.1177/03611981211005462.
- West, D. C., O. A. Acar, and A. Caruana. 2020. “Choosing among Alternative New Product Development Projects: The Role of Heuristics.” Psychology & Marketing 37 (11): 1511–1524. https://doi.org/10.1002/mar.21397.
- Wilczek, F. 2015. Einstein’s parable of quantum insanity. Scientific American, 23rd September. Accessed October 21, 2023. https://www.scientificamerican.com/article/einstein-s-parable-of-quantum-insanity/.
- Yost, C., and T. Schelpp. 2024. “For California’s Bullet Train, Progress is Being Made, But Its Completion Data Remains a Mystery”. 15th February, KTKLA5. Accessed April 5, 2024. https://ktla.com/news/california/for-californias-bullet-train-progress-is-being-made-but-its-completion-date-remains-a-mystery/#:∼:text=Now%20in%202024%2C%20the%20full,Authority%20has%20budgeted%20to%20spend