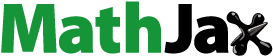
ABSTRACT
What were the effects of a 52 per cent increase in the minimum wage in the agricultural sector in South Africa in 2013? We estimate the short run effects of this policy change on the income, employment, and poverty rate of farmworkers, using individual-level panel data from the Quarterly Labour Force Surveys (QLFS). Before the implementation date, 90 per cent of farmworkers were paid below the new minimum wage level. We find that the wage gain of farmworkers is strongly quadratically related to pre-implementation wages, suggesting lower compliance as the gap between the minimum and the pre-implementation wage increases. We estimate that farmworkers received a median wage increase of 9 per cent as a result of the policy, and we find no evidence of job losses. Overall, farmworkers were 7 per cent less likely to have household income per person below the poverty line. One possible explanation for these outcomes is that endogenous compliance may mitigate against unemployment effects. While the minimum wage literature is large, our paper adds to the small subset of this literature on large increases, partial compliance, and poverty effects.
KEYWORDS:
1. Introduction
Studies of minimum wage effects in developing countries tend to find a modest impact on employment and poverty for at least two reasons. First, there is a significant amount of non-compliance in developing countries. Second, only a minority of workers are usually affected by minimum wage laws (see recent reviews by Gindling Citation2018; and Neumark and Corella Citation2021). We consider a large minimum wage increase in South Africa as a test case, where we show that there is a large shift in the sectoral distribution of wages, which thus addresses both of these concerns. Using individual-level wage variation, we study the effects of an increase in the minimum wage on wages, employment, and most importantly, on poverty, for which there are few existing estimates. Additionally, we explicitly assess the extent to which such effects vary with minimum wage compliance.
While there is a large literature on the impact of minimum wage laws in developed nations, the literature on the effect of minimum wage laws on employment in developing countries is rather small. Developing countries differ from developed countries in terms of their average income levels, the presence of larger (and thus uncovered) informal sectors, generally higher unemployment rates, and weaker institutions to enforce compliance with the law.
While the focus of this literature has been on employment and dis-employment effects, only a small number of studies have focused on the bigger question– the effects of minimum wages on poverty. There are several possible explanations for this. First, most minimum wage studies are focused on developed countries, where poverty rates are relatively low. Second, the data required to investigate the poverty effects of minimum wages are more onerous than those used to investigate the effects on employment. Poverty measures would require the ability to look at household-level dynamics, in terms of both composition and income, and then translate these into poverty measures. Third, for the law to have a meaningful impact on poverty rates, the industry (or group) that is covered by the law needs to be large enough to have a measurable impact on the aggregate earnings distribution. In addition, the increase in the minimum wage needs to be substantial in magnitude, rather than a small incremental change. These considerations pose a challenge to the empirical identification of the effects of minimum wages on poverty.
Our case study focuses on farmworkers in South Africa. In 2013, the agricultural minimum wage increased from 69 rands to 105 rands per 9-hour working day, an increase of just over 50 per cent (or 48 per cent in real terms). In addition, about 90 per cent of agricultural workers had wages below the new minimum wage level just before it was implemented. These factors suggest that we might expect to observe a substantial reduction in poverty in the period following the increase. At the same time, there are reasons to be cautious about the poverty-reducing effects of the law. First, low levels of compliance with minimum wages in South Africa have been well documented by previous researchers. Bhorat, Kanbur, and Mayet (Citation2012) estimate minimum wage non-compliance for nearly half of covered workers, and the agricultural sector had one of the lowest compliance rates. Potential explanations for the low levels of compliance include a lack of resources for enforcement and low penalties. Second, the rates of unemployment are exceedingly high, and may increase due to a large hike in the minimum wage. Some job losses may result in distributional shifts that could actually increase aggregate poverty.
plots the cross-sectional distribution of farmworker wages for the quarters surrounding the implementation of this minimum wage. In the two years prior to the increase, real hourly wages had a relatively stable distribution, with the mode being close to the previous minimum of R7.66. After implementation, there is a large shift in the entire distribution of hourly wages towards the new minimum of R11.66, which is stable in the year thereafter. Despite the large area under the density plot that is to the left of the minimum wage, which indicates substantial non-compliance in all periods, the aggregate shift is remarkable and demonstrates at least partial compliance with the policy change. At the same time, as we show in this paper, compliance is heterogeneous for low-wage compared to high-wage workers.
Figure 1. Farmworker hourly wages by year, old and new minimum wage. Source: Own calculations using the Labour Market Dynamics cross-sectional surveys 2011–2014, Statistics South Africa.
Notes: Hourly wages for agricultural workers are plotted, and defined as real monthly wage divided by weekly hours times 4. Fourth quarter incomes are plotted for each year. The previous minimum wage was R7.66 (first reference line), and was changed in 2013:q1 to ZAR11.66 per hour (second reference line). Observations are weighted by sampling weights in the cross-section.

This paper examines the extent to which this change in wages (resulting from the large increase in the agricultural minimum wage) led to reductions in the poverty rate of farmworkers. The cross-sectional poverty rates plotted in show decreases in poverty in the quarter immediately following the increase in the minimum wage, of about 4 percentage points using the poverty line from Budlender, Leibbrandt, and Woolard (Citation2015), a much larger drop of 13 percentage points using a 50 per cent higher poverty line, and a drop of about 5 percentage points using a 100 per cent higher poverty line. The changes in poverty rates depend on the line chosen, and also fluctuates in the surrounding quarters.
Figure 2. Cross sectional trends in farmworker poverty rates. Source: Own calculations using the Labour Market Dynamics surveys 2010–2014, Statistics South Africa.
Notes: The figure shows the proportion of farmworkers over time with household income per person below the poverty line. We show different multiples of the reference poverty line. The vertical line indicates implementation of the new minimum wage.

On the other hand, the aggregate statistics presented in augment the concern regarding disemployment effects. The table shows that there was a substantial reduction in agricultural employment between 2012 and 2013, but it also shows that 2012 was an outlier in terms of employment compared to previous years.Footnote1 Overall, despite the large increase in mean hourly wages and in the aggregate wage bill presented in , these potential disemployment effects, along with possible heterogeneity in compliance and effects by poverty line, leave open many empirical questions.
Table 1. Farmworker wages, employment and poverty rates by year.
In what follows we use variations in individual farmworkers’ wages prior to the law to empirically identify the short-run effects of the law. Our primary findings are that the change in the minimum wage increased average wages substantially, but that it is more difficult to attribute the decline in employment to the law. Consequently, average household income increased, and poverty declined, but with important heterogeneity for each of these outcomes.
Our paper makes two main contributions to the body of evidence on the effects of minimum wages. Firstly, while the purpose of a minimum wage policy is typically to reduce poverty, there is only a small literature directly evaluating the impact on poverty, especially in developing countries. While studies generally tend to find that poverty levels decrease, the results depend on the proportion of workers affected, the poverty line selected, and the magnitude of any disemployment effects (Gindling Citation2018; Neumark, Cunningham, and Siga Citation2006; Saget Citation2001). Aside from adding evidence to this literature, our context is of interest because this is a case with an especially large minimum wage increase, where 90 per cent of workers were below the new minimum wage level prior to its implementation. We also follow Dube (Citation2019) by examining a range of poverty lines, and show how this choice matters greatly for shaping our overall assessment of the policy.
Secondly, compliance is a recurring issue in the literature, depending on the rate of informality and the proportion of large formal firms (Bhorat, Kanbur, and Mayet Citation2012; Gindling Citation2018; Gindling and Terrell Citation2010). We find large effects despite around 40 per cent of farmworkers not having a written contract, which is a standard measure of informality. More importantly, our panel dataset enables us to track the effects on individual workers over time, which is particularly helpful in examining the extent to which compliance and disemployment effects depend on the pre-implementation wage. We find that the lowest paid workers do not receive the highest wage increases, and we also find much weaker compliance in small firms. Despite lower compliance, these groups do not appear to experience very different poverty effects. This may reflect an optimal response to weak enforcement; i.e., non-compliance where the minimum wage binds the most may mitigate unemployment effects (Basu, Chau, and Kanbur Citation2010). Badaoui and Walsh (Citation2022) model how authorities may intentionally ‘turn a blind eye’ in order to dampen unemployment effects, and this is reinforced by the fact that much of enforcement is driven through worker complaints (noting that workers would be unlikely to complain if they thought that the firm would go out of business as a result).
In the South African literature on minimum wages, previous studies have tended to find large increases in wages and little evidence of disemployment (Bhorat, Kanbur, and Mayet Citation2013; Dinkelman and Ranchhod Citation2012). The exception has been in the agricultural sector, with evidence of negative effects from a minimum wage implemented in 2003 (Bhorat, Kanbur, and Stanwix Citation2014; Van der Zee Citation2017). Piek, von Fintel, and Kirsten (Citation2020) find large disemployment effects for the 2013 minimum wage (the subject of this study), though most of this is from slower job creation and their empirical strategy for identifying these effects is not clear. No paper in the South African literature on minimum wages to date has considered the effects on poverty, even though this is particularly important in assessing the related welfare consequences, given the country’s high levels of both poverty and unemployment. Our biggest contributions relative to these papers is to quantify the effects of a minimum wage on poverty, and to explicitly consider how this varies with the extent of minimum wage compliance.
The remainder of our paper is structured as follows: Section 2 describes the context, data, and our empirical strategy. Our main results on wages, employment, household income and poverty are contained in Section 3. We discuss compliance and distributional effects in Section 4, and we conclude in Section 5.
2. Context, Data and Empirical Strategy
2.1. Context
The long-term trends in agriculture are important for understanding the relevance of the agricultural sector and the emphasis on poverty in South Africa. The agricultural sector is a low-wage industry that has been exhibiting strong negative employment trends over the past four decades: shedding jobs, relying on casual labour, and reducing the social wage (Hall et al. Citation2013). Van der Zee (Citation2017) notes that 1.2 million jobs are estimated to have been lost in the sector over the period from 1970 to 1995. Together with strong seasonal fluctuations, this makes causal analysis challenging.
In South Africa, agriculture has historically accounted for a substantial share of employment for low-skilled workers. This remains true at present, but over the past three decades, agriculture has become increasingly commercialized and mechanized, while agricultural output is increasingly being sold in highly competitive global markets. This is a trend relevant to several other African countries. An interesting and difficult policy question arises as to how a government might protect workers’ well-being in such an environment, where agricultural workers have amongst the lowest mean wages in the economy. Van der Zee (Citation2017) describes the poor living conditions of farmworkers in South Africa, including food insecurity which results in the malnourishment of children, inadequate housing and access to services, high levels of violence and alcohol abuse, and poor health and education outcomes.
Minimum wage policies present one policy tool to increase the welfare of low-paid and vulnerable workers. The increase in the minimum wage studied in this paper was implemented beginning on the 1st of March 2013. It increased the previous minimum of R69 per day by 52 per cent—to R105 per 9-hour working day (about $8 per day at a R13 exchange rate). The increase came as a large shock to farm owners, since a minimum wage review had been completed in early 2012 and the next review was scheduled for 2015 (Van der Zee Citation2017). Prior to 2013, the agricultural minimum wage from 2003 had generally been adjusted annually in response to inflation, with occasional small increases of a few percentage points above inflation. The 2013 change was a response to sudden and widespread protests that occurred in November 2012 in the Western Cape, with workers demanding a wage of R150 a day. R105 was the compromise set by South Africa’s Minister of Labour.
2.2. Data
We make use of 20 waves of the Quarterly Labour Force Surveys for our study, using all quarters or waves between 2010 and 2014. These surveys are collected by the national statistics agency, Statistics South Africa (StatsSA). Out of these 20 waves, nearly 13 precede the minimum wage increase on 1 March 2013 (two-thirds of the way through the 13th wave). These are nationally representative datasets which are collected four times per year, and are released with wage data as the Labour Market Dynamics surveys. The surveys are administered to each household member above the age of 15, and questions are asked about labour force participation, industry and occupation if employed, earnings, hours of employment, and other characteristics such as whether the person has a written contract or not. The wage data was converted to a monthly equivalent value and adjusted for inflation, using the quarterly CPI benchmarked on December 2012.
We classify a farmworker as follows: Any employed adult aged 15–64 who self-identifies as (a) having an occupation other than a manager, professional or clerk, (b) working in the crop or animal farming industries, (c) with a real income less than R5000 per month (or missing),Footnote2 (d) who are employed for the purposes of receiving remuneration and (e) who reports their race as being either African or Coloured.
The age restriction restricts our analyses to working-aged adults, while the occupational restrictions excludes relatively high earners who would probably be unaffected by the minimum wage. The R5000 income per month limit is also used to exclude a small number of high earners, and excludes about 1.5 per cent of the remaining sample. Our restriction relating to paid remuneration excludes primarily subsistence farmers or people who work on family-owned properties, as these workers are unlikely to be affected by the change in the law. Finally, the racial restriction is also due to our wanting to isolate a sample of workers who will likely be affected by the law. This rule excludes about 1 per cent of our sample, almost all of whom are white, and who typically earn well above the minimum wage. A breakdown of the sample sizes and how they are affected by some of these rules are presented in Table A1 in the appendix. Across all 20 waves, we have just over 18,000 farmworker observations that meet these criteria, with an average of approximately 900 farmworkers per cross-section.
Although this survey is focused on the labour market, we can estimate the household income per person amongst farmworkers as the total labour market income in the household plus the income from state provided social grants. Using other data sources, this accounts for over 90 per cent of household income for everyone but the top decile (Bassier et al. Citation2021). We proxy Old Age Grant (OAG) income by the number of farmworker household members aged 60 years or older multiplied by the OAG value, and we proxy income from the Child Support Grant (CSG) as the number of observed children in the household times the value of the CSG. As our definition for farmworkers excludes those earning above 5,000, the relevant means tests for grant eligibility are unlikely to matter. While there is some imprecision in this measure, household grants are a stable aspect of household income especially over the short run, which is the focus of our study.
For our regression analyses, we make use of a subset of the Quarterly Labour Force Surveys (QLFS). Over the 20 waves that we are using, the QLFS has a rotating panel component at the dwelling level, with a 25 per cent out-rotation rate. By working with StatsSA, we matched individuals who had maintained residency in the same dwelling over time, using data from their names, surnames, age, race and gender. We thus have a sub-sample of individuals who are observed in multiple consecutive waves, up to a maximum of four waves. A summary of the overall sample size for the rotating panel, by entering cohort, is displayed in Table A2 in the Appendix. We have just over 250,000 unique respondents aged 15–64 for whom we have repeated observations, and over 135,000 respondents that we observe for the maximum of 4 consecutive quarters. Over waves 2–17, nearly two-thirds of individuals are observed for all four quarters. In Figure A1 in the Appendix, we show that the panel trend is similar to the cross-sectional trend, thus alleviating concerns of differential attrition. We also perform a robustness check on our results by using attrition-adjusted weights.
To provide some sense of farmworker characteristics, we present summary statistics from our panel sample using waves of data prior to the law (Appendix Table A3). There are about half a million farm workers in each quarter, out of over 10 million employed people in the population. These farmworkers have very low hourly wages, with a median wage less than half the median wage of all employed workers. Ninety percent of farmworkers earn below the new minimum wage level, highlighting the potential impact of the increase in the minimum wage. Note, however, that only 30 per cent earned between the old and new minimum; i.e., 60 per cent were earning less than the old minimum wage, which reflects the high rates of non-compliance. Only about half of all farmworkers have a permanent contract, meaning that half are seasonally or precariously employed, and about 40 per cent do not have a written contract and are thus informally employed. Farmworkers are more likely to be male and are much more likely to reside in rural areas.
The reference poverty line that we use in this study is the one proposed by Budlender, Leibbrandt, and Woolard (Citation2015), which more closely follows international best practice. This line is calculated using the 2011 Income and Expenditure Survey, and is set at R1,042 per person per month. The authors follow a Cost of Basic Needs method, with calculations based on household expenditures, and caloric information for different foods. In brief, the authors find the price per calory of food using the average basket of food items for deciles 3–5 of the expenditure distribution, and then calculate the cost to reach a recommended minimum caloric intake benchmark of 2,100 kcal. This gives the food poverty line; the upper bound poverty line, which we use, adds on the average expenditure of households with food spending equal to the estimated food poverty line. This (upper bound) poverty line that the authors recommend is about a third higher than the (upper bound) poverty line published by StatsSA. All poverty lines involve numerous unavoidable assumptions. Thus, to reduce the dependence of our findings on the specific line chosen, we evaluate poverty effects using a range of poverty lines, indexed as multiples of the reference line. Seventy-six percent of farmworker household members are below our reference poverty line, which is higher than the national average of 69 per cent for household members who reside with all working adults.
2.3. Empirical Strategy
We use a standard treatment effects framework, which should be understood within the context of partial compliance. The agricultural minimum wage is not necessarily binding, in the sense that the monitoring of wages is low and farm employers could easily not comply, with only a small probability of being penalised (Bhorat, Kanbur, and Stanwix Citation2015). The ‘treatment’ therefore operates through a number of other possible channels: enforcement through organized workers who could report non-compliant employers, the threat of damages to public image, the threat of worker retaliation that could reduce productivity,Footnote3 or spillover mechanisms that force wages up to maintain recruitment under monopsonistic competition. For example, Badaoui and Walsh (Citation2022) model partial compliance where enforcement is driven by worker complaints.
Given this framing, several empirical approaches can be used to estimate the causal effects of the law. One of the most popular approaches is the difference-in-differences method (DD), which involves identifying a group of similar workers who would have experienced the same trends in employment and wages absent the law, but where some workers are subject to the law and some are not. This provides a natural experiment to estimate the difference in outcomes between the treated and untreated groups.
In our case, however, it is difficult to imagine the comparison group that would experience the same trends and shocks as agricultural workers, but are unaffected by the law. First, we do not have geographic variation as the law was a national law for the entire agricultural sector. Second, we might consider workers in other sectors but the shocks to agriculture, such as weather and pestilence, can be highly idiosyncratic and would be unlikely to affect workers in other industries in a similar manner. This would apply regardless of whether we considered a single other industry or a composite group of workers from multiple other industries. An alternative is to use the variation in average wage gap by district council, as done by Bhorat, Kanbur, and Stanwix (Citation2014). Out of 52 district councils over 2012 and 2013, only 28 have 100 or more rural adult observations in our data, of which farmworkers form a relatively small proportion. It is unclear how reliable the estimates would be if the identifying variation comes from so few observations. Any unobserved regional factors that are correlated with lower wage districts will also tend to confound the estimates.
Ultimately, we decided to use individual-level variation in pre-treatment wages below the new minimum wage level to measure variation in treatment intensity. We test whether this variation is systematically related to the likelihood of wage gains, job loss, and income changes amongst farmworkers, in a way that is different to any relation that may have been present in earlier waves. The ‘parallel trends assumption’ here is that outcomes in the treated year 2013 would have changed in a similar way to earlier years, absent the minimum wage intervention. To help alleviate concerns about contemporaneous shocks in 2013, we present the trends in agricultural investment and production by type of produce in Appendix Figure A2, which shows no clear breaks in the treatment year.
We restrict the estimation sample to members of the panel who were farmworkers in the first quarter of previous years (2010q1, 2011q1, 2012q1 or 2013q1). Wave 13, being the wave that contains the 1st of March 2013, is our treatment wave, while farmworkers from time periods before the law capture our pre-treatment period. We used only these pre-treatment waves for two reasons. First, this implicitly accounts for any seasonal variations in agricultural employment. Second, since no individual could be in the panel for more than four quarters, there is no chance of overlap between respondents in these different cohorts.
A key caveat about the empirical strategy is that this is purely a short-run evaluation. While our panel design is constrained to go at most three periods forward due to the 25 per cent out-rotation design, provides some justification for the short-run outlook. The trends for different percentiles of the farmworkers’ hourly wage distribution makes it immediately apparent that there is substantial non-compliance, with the 10th and 25th percentiles not reacting to the increase in the minimum wage. However, there is a sharp jump for the 50th and 75th percentiles. A key conclusion from this figure, which supports our empirical strategy’s focus on the first quarter after implementation, is that much of the adjustment in wages in this figure occurs during that first quarter.
Figure 3. Cross sectional trends in farmworker hourly wages, by percentile. Source: Own calculations using the Labour Market Dynamics surveys 2010–2014, Statistics South Africa.
Notes: The sample includes all working age adults. The vertical line indicates minimum wage implementation.

Our main specification is as follows: for t = 1, 2 or 3 and wave = 1,5,9,13 on the sample of farmworkers only,
The post-treatment dummy indicates 1 for wave 13 and 0 for earlier waves, gap = 100* (2275-monthly wage) / 2275; and X are age, age-squared, and Coloured and female dummies. We additionally include a quadratic in the gap to account for non-linearities in compliance, which is important for identification, as shown in our main results. The 10th, 50th and 90th percentile values of gap are respectively 2, 36 and 74, and the mean value of gap is 38.
Our treatment intensity variable is a percentage GAP variable, which is defined as the inflation adjusted difference between a farmworker’s wages and the 2013 minimum wage. Farmworkers who earn more than the 2013 minimum (in real terms) are assigned a value of zero for the percentage GAP variable. Recall that most workers were paid below the new minimum wage level before implementation. Our primary dependent variables are percent wage gain, job loss, percent change in household income per person, and change in poverty status.Footnote4 Poverty status requires the selection of a poverty line, and we primarily use 1.5 times the R1.042 poverty line in Budlender, Leibbrandt, and Woolard (Citation2015), but we present effects for a range of lines.Footnote5 We use absolute poverty lines in real terms, and divide household income by household size (which is recorded in the survey) to estimate a per-capita income variable.
We use a simple division by household size to calculate our poverty rates, instead of a parametrized equivalence scale, primarily for simplicity. Woolard and Leibbrandt (Citation1999, 14) evaluated the sensitivity of poverty estimates in South Africa to a broad range of parameter values using equivalence scales, and found that ‘the poverty profile changes very little even when we make large adjustments to the scale parameters. The poverty rate amongst Africans, Coloured and rural and urban dwellers remains astonishingly unchanged.’ In later work, Leibbrandt et al. (Citation2010) argue further that the choice of equivalence scale matters even less in the South African context, if the focus of the research is on changes in poverty rates, as long as the underlying samples and methods are consistent across time. Nonetheless, we recognize the importance of equivalence scaling adjustments by checking the sensitivity of our estimates to a widely used equivalence scale adjustment.
Each of the changes in our dependent variables are taken between the treatment quarter (quarter 1) and the following quarter (quarter 2). We show robustness for 2 and 3 quarters ahead, but attrition in the panel leads to greater imprecision in our estimates.
Our regression models were fitted using the conventional sampling weights from the cross-sections. We also estimated the results using attrition-adjusted inverse probability weights, which corrects for non-random attrition that is related to observable characteristics (see Wooldridge Citation2002, 587–590). The two different sets of weights do not change the results in a substantive way.
3. Results
Overall, we find large increases in wages for all farmworkers, but also find evidence of lower compliance for the lowest paid workers. There is some evidence that lower paid workers were more likely to keep their jobs, and that disemployment effects were generally small—although these results are not precisely estimated. The wage increases passed through to household income, resulting in large poverty declines in the short run for most farmworkers. There are indications of some increases in poverty for the lowest-paid farmworkers who likely lost their jobs.
3.1. Main Results
We estimate the main specification for the four outcomes, each with and without controls. The gap estimator is not straightforward to interpret, especially with a squared term, and we therefore present the full coefficient estimates in Appendix Table A4 while focusing on below which summarizes and interprets the coefficients.
Table 2. Summary of main regression results.
For ease of interpretation, panel A of shows the average 1-quarter change in each outcome for various groups, which makes up the implicit Difference-in-Differences comparison (adjusted for controls). Row (i) of column one shows that farmworkers in previous years with a gap value of 0 (i.e., paid R2275 or more) experienced a decrease in wages of about 11 per cent, which likely indicates mean reversion error for those workers who have relatively high observed wages. Row (ii) shows farmworkers in previous years too, this time at the median value of the wage gap regressor: they received a small wage increase of about 3 per cent. Rows (iii) and (iv) show the corresponding averages for farmworkers in the year of treatment; i.e., 2013: farmworkers with no gap were paid about 4 per cent less compared to 10 per cent less in row (i), and farmworkers with median gap were paid about 12 per cent more compared to 3 per cent more in row (ii).
The Difference-in-Differences estimate for the median gap is calculated by the difference in the estimates in row (iv) minus row (ii); i.e., the change for farmworkers in 2013 at the median gap netting out the counterfactual change as estimated by previous years. We show this in panel B, as the treatment effect at gap percentile 50 where the gap value is about 40. Column 1, row (vi) therefore shows that our estimated causal effect of the increase in the minimum wage level on wages one quarter later was to increase wages by 9 per cent for those at the median gap value. Since the median gap is a specific value, we show treatment effects at the 25th percentile (row v) where the gap value is 27 (showing a slightly higher wage increase of 9.5 per cent) and the 75th percentile (row vii) where the gap value is 55 (showing a lower wage increase of 6 per cent). We also show the treatment effect at the mean gap value which is similar to the median at 40, similarly with a predicted treatment effect of about 9 per cent, and the mean treatment effect (the average of all farmworker predicted effects), with a treatment effect of 6 per cent. Note that the last statistic, the mean treatment effect, includes farmworkers with 0 gap value and is therefore expected to be attenuated to 0.
Recall that a higher gap value implies lower pre-implementation wages. These results suggest that the percentage change in wages was lower for those with lower wages initially, which is the opposite of what one would expect in a high compliance context.
The results in columns 2–4 should be interpreted as above for the other outcomes. Column 2 shows employment loss– positive values imply farmworkers were more likely to lose their job. The coefficients are in fact negative (and statistically significant), implying that farmworkers were less likely to lose their job after the minimum wage; lower paid workers were the most likely to keep their jobs.
Column 3 shows the percentage change in household income per capita, with large and significant increases of between 10 per cent and 13 per cent for those below the previous minimum wage.Footnote6 Consequently, poverty fell strongly by between 5 per cent and 7 per cent. Despite their differences in wages and employment, the effects on household income and poverty are similar for previously lower paid workers compared to previously higher paid workers.
We run several robustness specifications on these results in the Appendix. Table A5 compares outcomes 2 quarters later (instead of 1 quarter later as in the primary specification). To some extent this traces a time path, but suffers from smaller sample sizes and therefore a decrease in precision. The wage effects are generally larger than for the one-quarter change (and are statistically significant), with a 12.5 per cent wage change treatment effect at the median gap. Lower paid workers have similar wage effects to the mean gap worker, and higher effects than higher paid workers. None of the employment loss results are significant, though the point estimates are positive (which would suggest employment loss). Household income per capita increased significantly for lower- and median-gap farmworkers, though the poverty changes are not statistically significant.
Given that the sample sizes are about half of those for the primary specification, and the corresponding estimates are therefore imprecise, we are cautious in interpreting these results. The results seem to confirm some level of compliance (with positive wage point estimates), but that compliance is partial and non-linear (under perfect compliance, one would expect much higher wage changes for the high gap workers, not the same as median gap workers). They also confirm that there was some durable pass-through of these wage changes into household income, especially for median gap farmworkers.
Table A6 provides estimates using alternative outcome measures for both 1 and 2 quarters ahead. There is no significant change in hours worked, and the point estimates are of different signs with a small magnitude of less than an hour change. One weakness of our specification is that it focuses on incumbent workers, rather than adjustments through hires. Columns 3 and 4 consider job gain, by simply comparing the proportion of job gain in the treatment compared to previous years (there is no gap variable for these workers). The point estimates suggest lower job gain 1 quarter later and higher job gain 2 quarters later.Footnote7 Finally, we expect household income to change differently for farmworkers who kept their jobs versus those who lost their jobs. Columns 5 and 6 consider the change in household income for job-keepers, showing that this is higher than the corresponding estimates for all workers, and is even more significant for the 2-quarter change. Columns 7 and 8 show the household income change for job-losers, which is not statistically significant. The point estimates for the job-loser households are generally positive 1 quarter later (presumably through dual-income or multiple-income households), but negative two quarters later.
Before proceeding to the poverty estimates (which are novel in the South African minimum wage literature), it is worth comparing our employment estimates to existing studies. Perhaps of most interest is the paper by Bhorat, Kanbur, and Stanwix (Citation2014) who find a negative employment effect from the 2003 minimum wage. One possible reason for the difference in findings is the different methods employed; as explained above, we use individual-level variation in wages over time whereas they rely on district-level average wages which may be more vulnerable to bias.Footnote8 However, it is also possible that the real effects of the minimum wages were different: they studied the introduction of a new minimum wage, whereas we studied an increase in the existing minimum wage. The initial law may have led to excess labour being shed, whereas in the 2013 case there may be a greater productivity cost of shedding further labour. There were also strong underlying trends at the time of agricultural liberalization and restructuring, as described above (Hall et al. Citation2013), which may have interacted with the law.
What might explain positive or non-negative employment effects of the minimum wage? This is by no means an outlier estimate, as several studies find similar effects from minimum wages in other contexts. Typically these results are explained by employers having some wage-setting power, as in the case of monopsonistic competition (Card and Krueger Citation1994). Employers with monopsony power pay workers below their contribution to production, meaning that as long as the minimum wage remains below their contribution, it is profitable for the employer not to fire the worker. As we discuss later, monopsony power may be particularly plausible in the context of South African agriculture. Other potential explanations include gift exchange, efficiency wages and fairness norms in the wage setting process, and demand-side effects from increased disposable income in the affected communities.
3.2. Implications for Poverty
The treatment effects summarized in refer to a poverty line 1.5 times greater than the reference poverty line. These estimates suggest decreases in poverty one quarter after implementation. Since this line was idiosyncratically selected, we next present poverty effects for a range of different poverty lines.
In , we present the estimated poverty rates corresponding to different poverty lines for different time periods. We present these estimates for quarter 1 and quarter 2 of the treatment year (2013), while for earlier years we present the average poverty rates (). The x-axis shows the poverty line as a multiple of the reference line: for example, 100 per cent of the reference line is just the reference line (R1,111 in 2013), and 150 per cent of the reference line equates to a poverty line of R1,667. The poverty rate corresponding to each line is the proportion of farmworkers with household income per person below that value. At the 150 per cent reference point presented in the main analysis, the light red shows that the overall poverty rate was 84 per cent in quarter 1 (i.e., before the minimum wage was increased) and 80 per cent in quarter 2 (i.e., 1 quarter after the minimum wage was increased). In contrast, the poverty rate in previous years at the same poverty line had increased by 1 percentage point between quarter 1 and quarter 2 of those years. This pattern is similar for poverty lines at 75 per cent, 125 per cent and 175 per cent of the reference line. However, the poverty rate measured at the reference line itself shows only a 0.3 percentage point decrease in the treatment year, relative to a 1.7 percentage point increase in earlier years.
Figure 4. Cross-sectional farmworker poverty rates before and after the minimum wage for different poverty lines. Source: Confidential panel data from the Labour Market Dynamics survey, Statistics South Africa.
Notes: The figure plots the average cross-sectional poverty rate for earlier years (2010–2012, blue) and the treatment year (2013, red) corresponding to before and after the new minimum wage. This is done separately for qtr 1 (lighter colour) and qtr 2 (darker colour) of each year. The reference poverty line is reported in Budlender, Leibbrandt, and Woolard (Citation2015); see main text for discussion. The sample includes only working age farmworkers in the relevant quarters.

Our primary specification compares the 1-quarter change in the poverty rate in the treatment year, to the corresponding 1-quarter change in the poverty rate in earlier years. It also identifies this effect at specific percentiles of the gap wage, which allows us to explore the effects of different levels of treatment intensity, as well as allowing for heterogeneity in these effects for different gap values. presents the coefficients and standard errors from our primary specification, where each value on each x-axis is derived from a separate regression. For example, the top left subfigure shows the treatment effects on poverty 1-quarter after the minimum wage was increased, for workers at the median gap value. The point estimates indicate reductions in poverty of between 5 and 10 percentage points across most poverty lines, with significance of the 95 per cent confidence interval for the 125 per cent, 150 per cent and 175 per cent poverty lines.
Figure 5. Minimum wage effect on poverty rate for different poverty lines, for workers at the percentiles of gap. Source: Confidential panel data from the Labour Market Dynamics survey, Statistics South Africa.
Notes: The figure plots the post coefficient for each separate regression on incremental percentages of the poverty line. The reference national per capita poverty line is reported in Budlender, Leibbrandt, and Woolard (Citation2015). The dotted lines show 95per cent confidence intervals (2 standard errors on either side). The sample includes only working age farmworkers in 2010:q1, 2011:q1, 2012:q1 and 2013:q1. Controls include age, age squared, gender and race. Regressions are weighted by sampling weights.

The estimated effects are similar for the 25th percentile of the gap wage (the top right subfigure), with a 5–10 percentage point poverty reduction which is statistically significant at the 125 per cent, 150 per cent and 175 per cent poverty lines. The 75th percentile of the gap wage (bottom left subfigure) exhibits a similar pattern, but with a concerning indication that poverty rose for lower poverty lines, i.e., those set at the 50 per cent and 75 per cent poverty lines. These increases in poverty are likely connected to job loss, which is consistent with the data in appendix table A6, which showed that the changes in household income were very different for job keepers and job losers. In the bottom right subfigure, we plot the mean treatment effect (using the actual gap values of farmworkers), which shows significant decreases in poverty of 3–5 percentage points across the 125 per cent, 150 per cent and 175 per cent poverty lines. Overall, these figures show a robust decrease in poverty across a range of higher poverty lines for most farmworkers, with some increases in poverty for the lowest paid workers at relatively low poverty lines.
4. Discussion
4.1. Compliance and Firm Size
A puzzle is why there is any ‘compliance’ in a low-enforcement environment. As argued earlier, there are likely other mechanisms involved which help ‘enforce’ the minimum wage, such as public reputation, spillovers, or organized workers reporting non-compliance or using the new minimum wage level as a fair wage benchmark.
Firm size is a key correlate of these alternative enforcement mechanisms. Large firms are more likely to have a public image to protect, to have organized workers, and even to have monopsonistic wage setting power. We consider heterogeneity by firm size by plotting the distribution of hourly wages before and after the new minimum (). The distributions of large firms (more than 50 workers) and small firms (fewer than 20 workers) are similar before the new minimum, in the sense that they are both centred around the old minimum wage, with larger firms paying slightly higher wages as expected.
Figure 6. Impact of minimum wage on wages by firm size. Source: Own calculations using the Labour Market Dynamics cross-sectional surveys 2011–2014, Statistics South Africa.
Notes: Hourly wages for agricultural workers are plotted, and defined as real monthly wage divided by weekly hours times 4. Firm size is identified at the worker-level. Fourth quarter incomes are plotted for each year. The previous minimum wage was R7.66 (first reference line), and was changed in 2013:q1 to ZAR11.66 per hour (second reference line). Observations are weighted by sampling weights in the cross-section.

However, after the new minimum is announced, there is a remarkable divergence in the distributions. Large firm wages move more decisively towards the new minimum. Small firm wages shift too, but with a smaller increase and greater variance in the wage distribution. The mean and standard deviations of hourly wages change from R7.10 (sd = 3.6) to R9.10 (sd = 13) in small firms, and from R8.30 (sd = 3.6) to R11.20 (sd = 10) in large firms. For both sets of firms, substantial non-compliance remains, but the distribution of wages is clearly more compliant in large firms.
The poverty effects presented in Appendix figure A3, are likewise larger for large firms. Poverty decreases by about 10 percentage points for the poverty lines equal to 125–175 per cent of the reference poverty line for those employed in large firms (focusing on the median gap), compared to a decrease closer to 7 percentage points for those working at small firms for those same poverty lines.
These figures provide some insight into the nature of compliance, as one partially mediated by large firms. This analysis is only suggestive, as the disaggregation by firm size results in small sample sizes. Nonetheless, larger firms are more likely to be productive/profitable, and also have greater monopsony power. They are thus more able to increase wages without incurring job losses. Smaller, less productive/profitable firms on the other hand, may be more constrained and would have to decrease employment to comply with the higher minimum wage, so these firms opt for non-compliance– with some risk of legal penalties and perhaps with tacit worker consent (Badaoui and Walsh Citation2022).
This explanation requires some wage-setting power by larger firms. Monopsony power appears to be high in South Africa generally (Bassier Citation2023), perhaps related to the high unemployment rate, limited outside options for workers, and severe job search frictions. These factors may be much worse in the agricultural sector, comprised of low-wage workers with little formal education and who are less desirable employees, and may entail higher transportation costs to other local job opportunities as well as to job opportunities in urban centres.
4.2. Distributional Effects of Minimum Wage
In the primary results above, we provided some evidence of distributional effectsat the 25th, 50th and 75th percentiles of the wage gap. We now decompose this further by considering the impact at each decile of farmworker earnings (Appendix Figure A4). However, this decomposition comes at the cost of precision.
We run a regression of the outcome on an indicator for post (equal to 1 in 2013 and 0 in 2010–2012), indicators for each decile of farmworker earnings, and the interaction between post and each decile indicator. We interpret the interacted coefficient as the difference-in-differences effect on each decile of farmworker earnings, where the first difference is the differenced outcome and the second difference is the effect on farmworker earnings deciles in earlier years. The outcomes are percent wage gain, employment loss and change in household income per capita, where all changes are across 1 quarter as above.
On the left hand side of Appendix Figure A4 we show the average 1-quarter change in outcome for 2013 (red) compared to earlier years (blue), separately for each farmworker earnings decile. On the right side we provide, by decile, the differenced estimate; i.e., the red minus the blue bars from the figures on the left. As noted above, this decomposition has low precision, and so the differenced estimates in the figures on the right have large standard errors.
The top panel shows the wage change. Note the evidence of mean reversion here, with large wage increases for lower earning farmworkers even in non-treatment years. The treatment effect magnitudes suggest large wage increases across the deciles, except for the very bottom. This is in line with the non-linear compliance observed earlier in the primary results.
Employment loss seems to show negative estimates (i.e., farm workers are more likely to retain the job) in the treatment year. As discussed before, these effects do not appear to carry over to the second quarter and so should be interpreted with caution. This may be reflecting some seasonality effects as the period two-quarters after the minimum wage was increased would have coincided with the winter months, when agricultural employment is typically at its lowest. The final panel, showing household income, again shows clear increases in point estimates across the farmworker earnings deciles, except for the bottom decile. This is consistent with the evidence discussed earlier that some low-paid farmworkers may have fallen into poverty. Overall, these decile estimates are consistent with the primary results.
5. Conclusion
This paper looks at the short-run effects of a large increase in the minimum wage for South Africa’s agricultural sector.
In the short-run we find large wage increases with no evidence of disemployment effects, and large increases in household income per person. Correspondingly, poverty decreases across a range of poverty lines. However, we find evidence of less compliance for the lowest paid workers, so some of them are shifted into poverty, although the net effect is to reduce overall poverty rates substantially.
Partial compliance is a fascinating aspect of this minimum wage study since it is characteristic of developing country settings and has received relatively little attention in the economics literature. In our study, over two-thirds of farm workers were initially recorded as being paid below the previous minimum wage, and in addition there is a low expected probability of legal penalty (Bhorat, Kanbur, and Mayet Citation2012). Perhaps the question should be why there is any compliance with minimum wage laws. We believe a shift in norms may help to ‘enforce’ the minimum wage, such as the threat of worker retaliation, organized workers reporting firms, damages to public image, or spillovers from monopsonistic wage setting. Consistent with this, we provide evidence that large firms, which are more likely to be influenced through these channels, are more compliant than small firms.
This study focused on 1-quarter changes in outcomes, with some evidence concerning 2-quarter changes, but estimating the size and direction of medium- and longer-run adjustments are left for future work. Our focus on the short-run is driven by our empirical method which uses the panel, and the 25 per cent out-rotation of the sample imposes a maximum of 4 quarters of repeated observations. Any panel method will also have to deal with the high worker turnover rate in South Africa. In addition, the QLFS updated its master sample for the survey at the beginning of 2015, which seems to have affected agricultural workers substantially and would have to be disentangled from any real economic effects of the minimum wage. Finally, any longer run analysis would have to contend with confounding longer run trends.Footnote9
Since we look at one minimum wage increase in the agricultural sector of South Africa, future work will need to determine the extent to which this is generalizable to other years, sectors or countries. Indeed, as we have discussed above, the context in this study is a low-wage environment which is likely subject to severe monopsony power and a difficult legal enforcement environment. This is also a limit case due to the size of the minimum wage increase, although we view this as particularly helpful for testing the limits of minimum wage policy.
Finally, many papers in this small but important literature on poverty effects of the minimum wage caution against using the minimum wage as a poverty reduction tool (Gindling Citation2018; Neumark and Wascher Citation2002). The 5–7 percentage point reduction in poverty that we estimated in this paper is substantial. As a comparison point, Woolard and Leibbrandt (Citation2013) simulate the effect of dropping all social grants in 2008, and find that the headcount poverty rate only increases from 70 per cent to 71 per cent. A full welfare calculation is out of the scope of this paper, but our results suggest that the minimum wage increase in this case was effective in reducing poverty amongst farmworker households.
Supplemental Material
Download MS Word (609.1 KB)Acknowledgements
[I/we] would like to use this opportunity to acknowledge and thank the reviewers who reviewed this article and aided in its publication. We also thank participants in the SALDRU seminar for valuable comments.
Disclosure Statement
No potential conflict of interest was reported by the author(s).
Notes
1 This may be reflecting lagged effects of rainfall outcomes. South Africa had very good and increasing rainfall from 2009 to 2011, and then experienced a prolonged multi-year drought beginning in 2012.
2 We included workers with missing income values as farmworkers, as the vast majority of African or Coloured workers in the agricultural sector do not earn more than R5000 per month. They are thus included in farmworker summary statistics. However, observations with missing income values are excluded from the estimation samples of the regressions, as well as any related poverty estimates.
3 An anecdote with a conference participant highlights this view. As the child of a farm owner, he had grown up on a farm and periodically spent time working there. He thought that the main reason a farm owner would raise wages in response to the minimum wage law was not to do with legal enforcement, but rather that workers would expect an increase in wages, failing which the disgruntled workers would (in ways difficult to trace) damage tools and property. The wage increase need not correspond to the law, but rather to workers’ expectations. This highlights an aspect of wage-setting under non-contractable employment, where the wage-setting is dependent on workers’ expectations which in turn depend on laws and neighboring workplace norms.
4 In terms of linearity of the relationship between the squared percentage gap and the outcomes, we rely on the result that the regression coefficients should be interpreted as providing the ‘best linear approximation to the underlying conditional expectation function, regardless of its shape’, and similarly we rely on this interpretation to justify using binary outcomes of employment and poverty outcome variables (Angrist and Pischke Citation2009). We use heteroskedasticity-robust standard errors in all our specifications to account for any heteroskedasticity.
5 We adjust the reference poverty line (see above) using the CPI to get R1111 per person per month in 2013, and since we are using 1.5 their poverty threshold as our reference line, our cut-off value in 2013 is equal to a value of R1667 per person per month.
6 We confirm that this result is robust to the use of standard household equivalence scales when calculating household income per capita. Specifically, we use the original OECD equivalence scale of assigning 1 to the household head, 0.7 to each additional adult household member, and 0.5 to each child. We find that the percentage change in household income per capita (equivalence adjusted), under the same regression specification as above, gives large and significant increases of 11 to 14per cent, which is just slightly higher than the main results reported above.
7 While these coefficients are statistically significant, they require very strong assumptions in order to be unbiased estimates of the effect of the law. In addition, even if one accepts that these do represent a valid causal estimate, these results would indicate a greater chance of finding employment as a farmworker two quarters after the law had come into effect.
8 The average may hide substantial dispersion, as a district could have a large proportion under the minimum wage while on average still having a relatively high wage. This leads to a potential measurement error problem, which would attenuate the estimate.
9 Some authors have tried to measure the long-run effects of minimum wage laws by including lags of the minimum wage in their employment regressions. These coefficients are often quite small, which indicates that most of the impact of minimum wages are observed in the periods immediately after a minimum wage law is passed. This suggests that the long-run effects are similar to the short-term effects. See Neumark and Wascher (Citation1992), and Dube, Lester, and Reich (Citation2010).
References
- Angrist, J. D., and J. S. Pischke. 2009. Mostly Harmless Econometrics: An Empiricist's Companion. Princeton, NJ: Princeton University Press.
- Badaoui, E., and F. Walsh. 2022. ‘Productivity, Non-Compliance and the Minimum Wage.’ Journal of Development Economics 155: 102778. see: https://www.sciencedirect.com/science/article/pii/S0304387821001383
- Bassier, I. 2023. ‘Firms and Inequality When Unemployment is High.’ Journal of Development Economics 161: 103029.
- Bassier, I., J. Budlender, R. Zizzamia, M. Leibbrandt, and V. Ranchhod. 2021. ‘Locked Down and Locked Out: Repurposing Social Assistance as Emergency Relief to Informal Workers.’ World Development 139: 105271. See: https://www.sciencedirect.com/science/article/pii/S0305750X20303983
- Basu, A. K., N. H. Chau, and R. Kanbur. 2010. ‘Turning a Blind Eye: Costly Enforcement, Credible Commitment and Minimum Wage Laws.’ The Economic Journal 120: 244–269.
- Bhorat, H., R. Kanbur, and N. Mayet. 2012. ‘Minimum Wage Violation in South Africa.’ International Labour Review 151 (3): 277–287.
- Bhorat, H., R. Kanbur, and N. Mayet. 2013. ‘The Impact of Sectoral Minimum Wage Laws on Employment, Wages, and Hours of Work in South Africa.’ IZA Journal of Labor & Development 2 (1): 1–27.
- Bhorat, H., R. Kanbur, and B. Stanwix. 2014. ‘Estimating the Impact of Minimum Wages on Employment, Wages, and Non-Wage Benefits: The Case of Agriculture in South Africa.’ American Journal of Agricultural Economics 96 (5): 1402–1419.
- Bhorat, H., R. Kanbur, and B. Stanwix. 2015. ‘Partial Minimum Wage Compliance.’ IZA Journal of Labor & Development 4 (1): 1–20.
- Budlender, J., M. Leibbrandt, and I. Woolard. 2015. South African Poverty Lines: A Review and Two New Money-Metric Thresholds. SALDRU WP 151. Cape Town: SALDRU, University of Cape Town.
- Card, D., and A. B. Krueger. 1994. ‘Minimum Wages and Employment: A Case Study of the Fast-Food Industry in New Jersey and Pennsylvania.’ American Economic Review 84 (4): 772–793.
- Dinkelman, T., and V. Ranchhod. 2012. ‘Evidence on the Impact of Minimum Wage Laws in an Informal Sector: Domestic Workers in South Africa.’ Journal of Development Economics 99 (1): 27–45.
- Dube, A. 2019. ‘Minimum Wages and the Distribution of Family Incomes.’ American Economic Journal: Applied Economics 11 (4): 268–304.
- Dube, A., T. W. Lester, and M. Reich. 2010. ‘Minimum Wage Effects Across State Borders: Estimates Using Contiguous Counties.’ Review of Economics and Statistics 92 (4): 945–964.
- Gindling, T. H. 2018. ‘Does Increasing the Minimum Wage Reduce Poverty in Developing Countries?’ IZA World of Labor 30 (2): 1–10.
- Gindling, T. H., and K. Terrell. 2010. ‘Minimum Wages, Globalization, and Poverty in Honduras.’ World Development 38 (6): 908–918.
- Hall, R., P. Wisborg, S. Shirinda, and P. Zamchiya. 2013. ‘Farm Workers and Farm Dwellers in Limpopo Province, South Africa.’ Journal of Agrarian Change 13 (1): 47–70.
- Leibbrandt, M., A. Finn, J. Argent, and I. Woolard. 2010. ‘Changes in Income Poverty Over the Post-Apartheid Period: An Analysis Based on Data from the 1993 Project for Statistics on Living Standards and Development and the 2008 Base Wave of the National Income Dynamics Study.’ Studies in Economics and Econometrics 34 (3): 25–43.
- Neumark, D., and L. F. M. Corella. 2021. ‘Do Minimum Wages Reduce Employment in Developing Countries? A Survey and Exploration of Conflicting Evidence.’ World Development 137: 105165. See: https://www.sciencedirect.com/science/article/pii/S0305750X20302928
- Neumark, D., and W. Wascher. 1992. ‘Employment Effects of Minimum and Subminimum Wages: Panel Data on State Minimum Wage Laws.’ ILR Review 46 (1): 55–81.
- Neumark, D., and W. Wascher. 2002. ‘Do Minimum Wages Fight Poverty?’ Economic Inquiry 40 (3): 315–333.
- Neumark, D., W. Cunningham, and L. Siga. 2006. ‘The Effects of the Minimum Wage in Brazil on the Distribution of Family Incomes: 1996–2001.’ Journal of Development Economics 80 (1): 136–159.
- Piek, M., D. von Fintel, and J. Kirsten. 2020. Separating Employment Effects Into Job Destruction and Job Creation: Evidence from a Large Minimum Wage Increase in the Agricultural Sector Using Administrative Tax Data (WIDER Working Paper No. 2020/51). Helsinki: UNU-WIDER.
- Saget, C. 2001. ‘Poverty Reduction and Decent Work in Developing Countries: Do Minimum Wages Help?’ International Labour Review 140: 237–269.
- Van der Zee, K. 2017. ‘Assessing the Effects of Two Agricultural Minimum Wage Shocks in South Africa.’ Master's thesis, University of Cape Town.
- Woolard, I., and M. Leibbrandt. 1999. Measuring Poverty in South Africa. Development and Poverty Research Unit Working Paper 99/033. Cape Town: DPRU, University of Cape Town.
- Woolard, I., and M. Leibbrandt. 2013. ‘The Evolution and Impact of Unconditional Cash Transfers in South Africa.’ In Development Challenges in a Postcrisis World, edited by L. Harrison, J. Lin, and C. Sepulveda. Washington, DC: World Bank. pp. 363–384.
- Wooldridge, J. M. 2002. Econometric Analysis of Cross Section and Panel Data. Cambridge, MA: MIT Press.