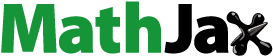
Abstract
The continuous combination of digital network technology and traditional financial services has given birth to digital financial networks, which explore massive economic data under the AI-driven models to achieve intelligent connections among financial institutions, markets, transactions, and instruments. Empirical asset pricing is a challenging task in financial analysis, which has attracted research attention. However, existing studies only focus on tackling the challenges of equity risk premium in the single stock market. Considering multiple economic linkages between the two countries, the transaction history of the US stock market as empirical knowledge is a powerful supplement to improve the prediction of equity risk premium in the China market. In this paper, we aim to fully leverage the prior information in two stock markets for empirical asset pricing models. Due to the rich financial domain knowledge, there may be various characteristic signals that partially overlap in different periods. To address these issues, we propose a framework based on long-short dual-mode knowledge distillation, termed as LSDM-KD, which incorporates US and China stock market models, and a shared characteristic signals model. The method effectively understands the relationships between assets and market behaviour, reducing reliance on expensive correlation databases and professional knowledge. Extensive experiments conducted on US and China stock market datasets demonstrate that our LSDM-KD can significantly improve the performance of empirical asset pricing.
1. Introduction
Currently, with the rapid development of network information (S. He et al., Citation2022; Liang et al., Citation2019) technology, digital finance (Ai et al., Citation2023; Ozbayoglu et al., Citation2020) plays a vital role in the economic development, such as Internet payment, online banking, and virtual currency. Driven by the continuous promotion of artificial intelligence (AI) technology, the Internet of Things (IoT) (Deng et al., Citation2019; Jing, Cui, Zhang et al., Citation2023) devices and applications significantly continue to evolve towards the trend of intelligence. Therefore, a series of intelligent applications can better satisfy the demands of various scenarios, such as intelligent transportation (Liang et al., Citation2023), energy management (Dai et al., Citation2022; Deng et al., Citation2015), recommender systems (Jing, Cui, Guan et al., Citation2023), and financial data analysis (Guo et al., Citation2022). On this basis, the continuous integration of traditional financial services and emerging AI technology (Z. Wang et al., Citation2023) has greatly propelled the emergence of financial technology (fintech) companies, which fully leverage new technologies and innovations to provide financial industry.
As an emerging paradigm of financial services, digital financial networks are driven by fintech tools to automatically interconnect various financial entities, such as financial institutions, markets, and assets. As shown in Figure , digital financial networks can be constructed through the network information technology to interactively connect various financial participants, including merchants, cardholders, banks, and the digital certificate authorities. Under the context of the digital economy, financial networks provide the considerable opportunities for digital financial markets, which can help users understand the risks and structure of financial markets. In addition, financial networks also have extremely broad application scenarios through AI technology in the financial field, such as mobile payment (Yuan et al., Citation2022), digital currency (Islam et al., Citation2022), stock market, digital bank (Islam, Citation2022), and the blockchain systems (Shi et al., Citation2021; W. Wang et al., Citation2023). On this basis, empirical asset pricing is a challenging task in the field of financial management, which has attracted great research attention. This task aims to explore the economic links between different stock markets and predict the risk premium in the China stock market, which alleviates the urgent need for expensive data support and professional financial knowledge in the application of industrial financial networks. For practical application scenarios, this modelling approach can improve the assessment accuracy of correlation modelling between financial assets and the effectiveness of investment decisions in China stock market.
Figure 1. Illustration of digital financial networks architecture, which is an emerging network pattern that effectively leverages the digital network technology as the core support to conduct various financial activities (e.g. electronic payment, securities transaction, and FinTech). The digital financial network is mainly built from multiple participants in the network transactions, including cardholder, merchant, payment gateway, acquiring bank, isssuing bank, and digital certificate authority.

Previous machine learning methods (Huang et al., Citation2022, Citation2023) have brought huge breakthroughs in various fields, such as image identification and natural language processing. In particular, its application in empirical asset pricing has attracted considerable interest from the research community (Chen et al., Citation2020). For a typical problem of empirical asset pricing: measuring equity risk premiums, machine learning predictions can bring huge benefits to investors. In some cases, these algorithms have been shown to perform better than regression-based methods in the literature. Therefore, for machine learning methods, it shows more promising performance due to its ability to search for linear and nonlinear relationships between characteristic signals with equity risk premiums (Rezaei et al., Citation2021).
However, there are increasing concerns that whether these machine learning algorithms work well depends on the size of the dataset. The empirical asset pricing methods are always based on portfolios, which means that it requires the long time periods and large-scale stock samples to support its training process. Compared with the US stock market, the development of the China stock market is relatively late and its scale is relatively limited under this condition. Many existing research efforts only focus on finding some abnormal signals that can provide effective excess returns, while they ignore how to utilise the limited data to improve model performance.
To improve predictions of China equity risk premiums, we consider global economic integration and the existence of various economic linkages between different countries and their respective stock markets, such as China and US markets. Since the US stock market has the characteristics of a long cycle and large scale, it can provide relatively abundant auxiliary information for improving the prediction of equity risk premium in the China stock market. We aim to combine the domain knowledge of US and China stock markets and employ these to develop in China stock market, which is developing relatively late and has a limited stock sample size. The current research mainly suffers from the following two key limitations. First, the selected characteristic signals are partially overlapped in China and US stock markets. Over the past few decades, the search for signals that can explain the cross-section of expected stock returns has produced hundreds of potential signals candidates. Second, considering the large amount of potential signals, the selected asset pricing models in different countries are not completely overlapped as the following reasons: (1) There are both truly useful factors and useless signals in the factor set. (2) The same signal information may appear differently in different countries. To address the inconsistent signals and misaligned time period, different from conventional distillation methods, our work attempts to simultaneously leverage both US and China stock markets to improve the performance of empirical asset pricing models via a dual-mode knowledge distillation leaning manner.
In this paper, to address the above challenges in the empirical asset pricing models, we introduce a novel framework based on long-short dual-mode knowledge distillation, termed as LSDM-KD. As shown in Figure , the LSDM-KD consists of the following modules: US and China stock market models as the dual-mode teacher network, and a shared characteristic signals model as the student network. Since the inputs of prediction model is based on selected characteristic signals from US and China stock markets, the shared characteristic signals model as a student network can effectively avoid the impact of missing input information on model performance. Specifically, we first train US stock market model until the same point time, and then utilise both US and China stock market models to update the shared characteristic signals model, which can compile the financial domain knowledge. Experiment results show the superiority performance of our proposed LSDM-KD compared with state-of-the-art methods.
Figure 2. Illustration of our proposed LSDM-KD framework, which incorporates US stock market model, China stock market model and a shared characteristic signals model. Under the joint guidance of a dual-mode teacher network, the shared characteristic signals model as the student network can effectively absorb the overlapped signals of China and US stock markets. For the names of common signals, we use the names of the US stock markets as the representatives.

The main contributions in this work can be summarised as follows:
We present a novel empirical asset pricing model in digital financial networks with a long-short dual-mode knowledge distillation framework, termed as LSDM-KD. To the best of our knowledge, this is the first attempt to study the predicting stock risk premiums under the joint action of stock markets in two countries.
To address the linkage between China and US stock markets and improve the prediction of China equity risk premium, the shared characteristic signals model as the student network effectively absorbs the rich knowledge under the joint guidance of a dual-mode teacher networks. This framework has a strong generalisation ability, which can be applied to the stock market of different countries under economic globalisation.
Extensive experiments on China stock market dataset demonstrate the superiority of proposed LSDM-KD method in the long-short portfolio over the classic and advanced methods, the effectiveness of each component module, and the robustness to different sizes of training period parameter.
The rest of this paper is organised as follows: Section 2 briefly reviews the related work. In Section 3, we introduce the problem formulation. Section 4 introduces our proposed LSDM-KD scheme in detail. The experimental results and analyses are presented in Section 5, and Section 6 discusses a brief conclusion of this work.
2. Related work
2.1. Empirical asset pricing via machine learning
Empirical asset pricing task is one of the core issues in financial data analysis research. Previous studies mainly model the cross-sectional returns (Fama & French, Citation2008) or time series (Welch & Goyal, Citation2008) returns of assets as a function of lagged characteristics through linear regression. Due to the limitations of linear regression and the continued advancement of big data technology, machine learning-based methods have gradually appeared in asset pricing models. Generally, the empirical asset pricing models based on machine learning aim to mine the correlations between characteristic signals and conduct model prediction evaluation. Rapach and Zhou (Citation2013) utilised the LASSO model to predict global stock market returns based on the lagged returns of all countries, while Yao et al. (Citation2000) adopted a neural network to predict derivatives prices. Butaru et al. (Citation2016) adopted the regression tree model to predict consumer credit card delinquency and default behaviour. Recently, machine learning-based methods have been used in particular for cross-sectional stock return studies. For example, Gu et al. (Citation2020) used multiple machine learning methods to predict stock returns and found that shallow neural networks can achieve the best performance. Kelly and Pruitt (Citation2013) proposed a dimensionality reduction method to estimate and validate signal pricing models.
According to relevant research, the linkages between different stock markets are critical to the stability of world financial markets. For example, the supply and demand of oil will affect the China stock market in the short term, and impact the US dollar index and US stock market in the long term. Goh et al. (Citation2013) found that using US economic variables can significantly predict the China stock market, which is based on the impact of the fluctuation patterns of the US stock market on the China stock market. Pukthuanthong and Roll (Citation2009) derive a new integration measure for international markets. Besides, Teng et al. (Citation2020) proposed a machine learning-based approach to capture useful information through using a large set of predictors for improving the predictability of stock returns. Cooper et al. (Citation2024) proved that the ability to price cross-sectional returns decreases significantly when constructing the factors considering indicators of investment in intangible assets. In summary, previous studies utilise econometric models to describe the transmission of volatility between markets, while exploring how connections between world markets change over time and relative trends increase during periods of high volatility.
2.2. Knowledge distillation
Knowledge distillation (L. Wang & Yoon, Citation2021; Zhao et al., Citation2022) is one type of model compression method based on the teacher–student network training manner, which has been widely studied and applied to various machine learning tasks. Specifically, the knowledge distillation system mainly consists of domain knowledge attribute, distillation algorithm and teacher–student structure, which explores how to transfer knowledge from teacher model to student model. To boost the generalisation performance of data-driven models, the key of knowledge distillation can effectively utilise the additional knowledge to assist in training traditional neural networks.
Aiming to solve the issues of online deployment of large integrated models, Hinton et al. (Citation2015) first proposed a knowledge distillation framework for converting information in the large models into training small models. Generally, according to whether the teacher network is updated or not, knowledge distillation can be roughly divided into three architectures: offline distillation, online distillation and self-distillation. The offline distillation process consists of two stages, including the teacher model pre-training and knowledge transfer. For example, Ma et al. (Citation2023) designed a multimodal contrastive knowledge distillation framework, which teacher network adaptively refine the knowledge to during multimodal contrastive learning. However, online distillation adopts an end-to-end training manner, which teacher model and student model can be updated at the same time. For example, Li et al. (Citation2022) introduced a novel online distillation scheme based on feature fusion and self-distillation. This approach can jointly train student networks to learn diverse information and reduce the computational complexity of model during deployment. The self-distillation framework means that both the teacher and student networks adopt the same network structure, in which the student network learns knowledge by itself. In particular, to explore the instructive knowledge unavailable to teacher networks, Zhang et al. (Citation2022) proposed a self-distillation method to adaptively generate internal and external relationships between its targets as instructive knowledge. To address the most important sample regions, Gou et al. (Citation2022) designed multilevel attention-based sample correlations for knowledge distillation for image classification and and person reidentification.
3. Problem formulation
We first define three basic stock market prediction models, including China stock market model named , US stock market model named
and a shared characteristic signals model named
. Our research mainly explores an investment task in China stock market for
periods. As for the data in China stock market, during the tth period, the monthly return rate of the set of China stock is denoted as
. Hence, the size of set is based on the number of stocks in the previous
th period, which can be denoted as
. Mathematically, the monthly return rate
can be represented as
.
Based on the empirical asset pricing tasks, we further use the integrated characteristic signals to predict stock returns. The number of selected characteristic signals in the China stock market is recorded as . As for each stock sample in the
th period, the input vector of the ith stock sample can be correspondingly represented as
. The empirical asset pricing task is a standard supervised learning and regression problem, which is to find the following functional form as follows:
(1)
(1) where
defines a function with parameter
, which is a prediction model used for empirical asset pricing in China stock market,
represents the error term of ith stock samples during the tth period.
After predicting each stock sample , we sort the stocks in descending order according to the prediction results and monthly return rate, and then establish a long-short investment portfolio, i.e. long
of the stock with the best expected monthly return rate and short
of the worst stock. Notably, both the long and short portfolios are allocated with equal weighted. Holding the portfolio for a month can earn the corresponding investment return, which is a metric used to determine the performance of different methods.
Moreover, as for the data in US stock market, the periods can be denotes as . Considering that US stock market developed earlier than China, naturally
. During US stock market in the tth period, the monthly return rate is set to
respectively input vectors (the ith characteristic signal of stock samples) are
. Similarly, the empirical asset pricing task in US stock market is defined as follows:
(2)
(2) where
is a prediction model used for empirical asset pricing in the US stock market.
In this work, the US stock market dataset starts from July 1963 and ends in December 2019, and China stock market dataset starts from January 1997 to December 2019. In addition, we define the length of each round of training data to be 12 months, which is denoted as . Ultimately, our work aims to improve the empirical asset pricing performance in China stock market by learning from both China and US stock market prediction models (
and
).
4. Proposed methodology
4.1. Motivation
Considering the trend of economic globalisation and linkage between China and US stock markets, we mainly explore China empirical asset pricing predictions via simultaneously leveraging China and US stock markets. Although it is a good idea to learn from both stock markets and generalise empirical asset pricing predictions in relatively young stock market, this still causes the following potential problems. First, from the perspective of the stock market periods, US stock market periods are longer than China stock market. Additionally, regarding the training data, there is a partial overlap in the characteristic signals selected in the China and US stock market. The final challenge is learning the model weights in a stock market that may not always exhibit the competitive prediction performance. Due to the late development of China stock market, the size of stock sample is limited, and it is unstable for training only with data from China stock market.
Generally, as for the knowledge distillation framework, suppose T represents a teacher model with the learnable parameters and S denotes a student model with the learnable parameters
. Considering that
is much smaller than
, the proposed knowledge distillation method is used to train the teacher–student network by minimising the objective function on the training samples
. The temperature setting in knowledge distillation mainly depends on the degree of attention paid to the negative labels during the student network training stage, which is directly related to the parameter size of the student model. Mathematically, the objective function of knowledge distillation is defined as follows:
(3)
(3) where
and
denote the learnable parameters of teacher and student networks, respectively.
is the Cross-Entropy loss,
and y are the predicted results and ground-truth labels, and λ is the trade-off hyperparameter.
In our work, the shared characteristic signal set is the intersection of China and US market characteristic signal sets, which can be defined as the shared characteristic signal model . It is well known that knowledge distillation (Han et al., Citation2019) can transfer the rich knowledge learned from cumbersome models to relatively simple models. Inspired by knowledge distillation, due to different time periods, we can first train the US market model until the data cross-sections of the two stock markets reach the same time point. Moreover, the basic form of knowledge distillation is based on student and teacher networks, which includes the corresponding cross-entropy loss of the true labels and the distillation loss of the predicted labels. Based on this, we need a hype parameter λ to control these two loss functions. In our framework, we utilise this hype parameter to represent the teacher's performance, which means that the weight of the distillation loss changes with the predicted performance of the teacher network and is not fixed.
We assume that the prediction of China equity risk premiums is based on the stock markets of two countries (i.e. China and US stock markets). However, the abnormal factors used in model training between China and US partially overlap, and the time period of US stock market is longer than China. Therefore, we can learn the knowledge of two stock markets by performing knowledge distillation on China and US stock market models in sequence. And under the supervision of the model and labels, the model takes into account both generalisation ability and prediction ability and performs better compared to a single stock market. Based on the above conditions, we aim to establish a framework to improve the prediction of China equity risk premia by leveraging China and US stock markets, rather than just training for the China stock market.
4.2. Proposed framework
In this section, we first introduce the cross-section of stock markets, and then detail the proposed LSDM-KD framework. As shown in Figure , our proposed framework is based on a teacher–student network, including US stock market model, China stock market model, and a shared characteristic signals model.
4.2.1. The cross-section of stock markets
In this paper, we use the data before the decision-making time to fit and determine the model parameters. To ensure the effectiveness of calculations and the feasibility of investment, the sliding window method is adopted to divide the training and testing datasets. The pre-processing of training and testing data sets is three steps. First, assuming the starting time is January 2000, our model can determine the portfolio for that month. Thus, we use the characteristic signals and monthly return rate of the past months (in 1999) as the training dataset. Second, the characteristic signal data in December 1999 can be input into the trained model to obtain the prediction of monthly stock returns in January 2000. Finally, based on the prediction results, we sort the stocks in the cross-section and construct a long-short portfolio, i.e. long the 10
stocks with the best expected monthly returns and short the 10% stocks with the worst expected monthly returns. Notably, both long and short positions are equally weighted, and the portfolio can be held for one month to obtain investment income in January 2000.
4.2.2. Long-short dual knowledge distillation
Considering that US stock market starts earlier than China stock market (i.e. ), we first train the US stock market model
until China and US stock markets can both provide the training data at the same time (i.e.
). The update of US stock market model
is based on the mean squared loss between its prediction results and the true targets. The goal of
can be defined as follows:
(4)
(4) where
is the number of
th period US cross-section of stock markets.
After pre-training US stock market model , we begin to jointly train China and US stock market models
and
. Similar to
, the update of China stock market model
can be defined as follows:
(5)
(5) where
is the number of
th period China cross-section of stock markets.
Enlightened by knowledge distillation methods, our framework treats shared characteristic signals model as a student network and learns based on the knowledge mined by China and US stock market models (
and
). The loss function of knowledge distillation in Equation (Equation3
(3)
(3) ) mainly consists of two parts. One is the cross-entropy loss based on the predicted results
of student network and its corresponding ground truth label y, and the other is the distillation loss that compares the predicted results of the student and teacher networks. In addition, λ is the trade-of parameter to balance the contributions of different loss terms.
In our LSD-KD framework, since the output vector of each stock sample is and the values of λ, we respectively define the similarity of US and China stock market models between the predicted results and the truth label based on the cosine similarity as follows:
(6)
(6) where
and
represent the prediction similarity of US and China stock market models, respectively.
And then, we combine the teacher–student network and the above-predicted similarity together to update the shared characteristic signals model . The objective of
training on US stock market with
can be defined as follows:
(7)
(7) Then, in this similar manner, the objective of
training on China stock market with
can be defined as follows:
(8)
(8) By combining the predicted results of teacher network and the truth labels, the student network (i.e.
) can learn knowledge effectively. Furthermore, our framework can reduce the influence of the teacher network from poor prediction results, and avoid low generalisation ability due to deep constraints on ground truth labels.
Ultimately, we obtain the on US stock market model
and China stock model
respectively, and set a small learning rate
compared with η to train
again on China stock market without a teacher network. The main goal of the joint shared stock market model
can be defined as follows:
(9)
(9) The training procedure of our proposed method LSDM-KD is summarised in Algorithm 1.
5. Experiments
5.1. Experimental settings
5.1.1. Dataset
As for US stock market, we selected 94 characteristics (Green et al., Citation2017), each of which can be entirely calculated from CRSP, Compustat, or I/B/E/S data. Our dataset covers 56 and a half years from July 1963 to December 2019 and contains 94 characteristics and their descriptions. We start with all companies with common stock on the NYSE, AMEX, or NASDAQ that have a month-end market capitalisation on the CRSP and for which the value of the common stock is not missing from their annual financial statements. Then we integrate the data between Compustat, I/B/E/S, and CRSP, and calculate to adjust aligned calendar time characteristics. Specifically, for each return in month t, we calculate the characteristics at the end of month . Assuming that annual accounting data for month
are available at the end of month
if the company's fiscal year ends at least six months before the end, and that quarterly data are available at the end of the month (
1) if the fiscal quarter ends at least four months before the end of the month (
1 ). I/B/E/S and CRSP data are aligned in calendar time using the I/B/E/S statistical period date and the CRSP month-end date.
For China stock market, we selected 95 characteristics (Green et al., Citation2017), among which company characteristics and stock return data are from the CSMAR database. Our dataset covers 23 years from January 1997 to December 2019. The Shanghai and Shenzhen Stock Exchanges implemented a limit on the trading price of listed stocks starting from 16 December 1996. In order to avoid the impact of this major change in trading mechanism on our research, we chose January 1997 as the starting point. Notably, our sample includes Chinese A-share companies. Due to the poor market performance of ST stocks and the risk of delisting, we exclude ST stocks to avoid additional impact on the research. Some characteristic structures of financial stocks are different from other listed companies, so this paper also excludes financial stocks. And due to the IPO underpricing effect, the stock price may fluctuate abnormally in the first year of listing, we also exclude data from the first year of listing. Similar to the treatment for the US data, for each return in month t we compute the characteristics at the end of month
. Due to the lag in financial statement disclosure, the basic principle of filling in data is to fill in data only after all required statements are complete.
Both the US and China stock market data, after the characteristic construction and data alignment, there are significant differences in the magnitude and distribution of different signals values, which may lead to biased prediction. Based on this, we normalise the two datasets. Specifically, for each month's characteristic data, we sort all values and make them uniformly distributed centred on 0, and then fill the remaining missing values with 0. According to statistics, among the 94 characteristics of US capital market and the 95 characteristics of China capital market, there are 77 common characteristics. It is worth noting that the two national markets have 17 and 18 unique characteristics, respectively.
5.1.2. Implementation details
In our experiments, we set the training period to months, the testing period to the next one month, and each training period is set to 200 epochs. The adaptive moment (adam) optimiser is adopted for our model optimisation. Specifically, from July 1963 to January 1997, we first trained US stock market model
, and set the learning rate η to 0.02. Starting from January 1997,
and
are trained for each period
months, respectively. For the optimisation of
, we use the teacher networks
and
in turn to perform model training for 200 epochs, and the learning rate is set to
. Finally, we train the joint model
on the cross-section of China stocks dataset with the adjusted learning rate
and then complete the prediction task of stock risk premium. The backbone networks of
,
, and
are ResNet (K. He et al., Citation2016) structures. Specifically, since the training phase of
is from January 1997 to December 2019 and
, the value of
is set as
. In addition, our experiments are implemented by PyTorch and conducted on a single NVIDIA GeForce RTX 3090 GPU.
5.2. Experimental results
5.2.1. Comparison with classic and advanced methods
To illustrate the effectiveness of our proposed LSDM-KD framework, we conduct a comparative analysis of portfolio performance with several classic and advanced prediction methods on China stock market dataset. To ensure the comprehensiveness of our experiments, we mainly select the following machine learning-based methods as comparison baselines, including ordinary least squares regression (OLS), partial least squares regression (PLS), ridge regression (Ridge), lasso regression (Lasso), support vector machine (SVM), elastic net regression (ElasticNet), and deep neural network (DNN) (Samek et al., Citation2016). These prediction methods have been widely used in financial academic research and achieved good prediction performance (Light et al., Citation2017). Moreover, we set the inputs of the DNN models used in China and US stock market models to and
, and the two DNN models that use 77 common characteristics and 95 China capital market characteristics are represented as DNN77 and DNN95, respectively. In our experiments, we adopt the same training and test datasets, and fine-tune all comparison methods to report the optimal results in terms of each metric.
Table shows the portfolio performance comparison between our proposed LSDM-KD and other classic and advanced methods on China stock market dataset. It includes the following evaluation metrics: portfolio Mean Returns, T-statistics, and Sharpe Ratio. From the table, we can see that our LSDM-KD in the long-short portfolio outperforms all comparison baselines on various performance metrics, which demonstrates its significant superiority for solving empirical asset pricing modelling task. Obviously, our approach achieves an optimal portfolio Mean Returns of 3.3860 and a Sharpe Ratio of 2.0484, while other portfolio Mean Returns range from 2.5219
to 3.2554
, and Sharpe Ratio ranges from 1.2467 to 1.9661. The performance in long portfolio and short portfolio obtained the unsatisfactory results, indicating that the importance of jointly considering both China and US stock markets for asset pricing models.
Table 1. Portfolio performance comparison between proposed LSDM-KD and several classic and advanced methods on China stock market dataset.
Table 2. Risk-adjusted portfolio performance comparison between our proposed LSDM-KD and several classic and advanced methods on China stock market dataset.
Table shows the risk-adjusted portfolio performance comparison between our proposed LSDM-KD and other classic and advanced methods on China stock market dataset. It includes the following evaluation metrics: Fama-French three-factor (FF3-α ()), T-value-3, Fama-French five-factor (FF5-α (
)), and T-value-5 . From the table, we can observe that our method always shows the best performance regardless of adjusting the returns through FF3 or FF5. Moreover, when the input signals increase (changing from DNN77 to DNN95), the model performance of the long portfolio can be obviously improved.
According to the results in Tables and , it is worth noting that our proposed method both exhibits significant performance differences in the Short-term portfolios. One possible reason is that US stock market data with a long history may require injecting rich teacher knowledge into the Long-term portfolios in the dual-mode distillation learning process, which is difficult to play a role in the Short-term portfolios only.
5.2.2. Ablation analysis
In this part, we mainly explore the contribution of each component of our proposed LSDM-KD method. In our experiments, we compared the performance by removing the relevant components using the following schemes: without knowledge distillation, only short teacher network, only long teacher network, conventional knowledge distillation, mutual knowledge distillation and cross-dual knowledge distillation.
Table shows the ablation study of our proposed LSDM-KD scheme on China stock market dataset. From the table, we can see that involved component show inferior classification performance compared to ours, illustrating that they generate positive effects for empirical asset pricing tasks. The only short teacher network and only long teacher network represent training only China or US stock market model, respectively. These two variants both obtain the unsatisfactory performance, demonstrating that it is difficult to effectively learn professional financial knowledge by relying only on a single teacher network. In addition, compared to different forms of knowledge distillation frameworks, without knowledge distillation shows the poor performance. It demonstrates that knowledge distillation learning is an effective framework capable of integrating characteristic signals of China and US stock markets. Our proposed cross-dual knowledge distillation outperforms conventional knowledge distillation and mutual knowledge distillation, which verifies that cross-dual knowledge distillation can fully capture the shared characteristic signals between different stock markets and effectively improve model performance.
Table 3. Ablation study of our proposed LSDM-KD scheme on China stock market dataset.
5.2.3. Parameter sensitivity analysis
In this part, we further discuss the parameter sensitivity to the scale of . In our experiments, to investigate the influence of training periods
, we consider adjusting
within the range
and compare with using deep neural network (DNN95) only on China stock market.
Figure shows the sensitivity analysis of the portfolio performance between our proposed LSDM-KD approach and DNN95 on China stock market dataset. From the figures, we can find that our proposed LSDM-KD achieved the best portfolio performance when is set to
. Nevertheless, except the Sharpe Ratio when
, all metrics of our method in the long-short portfolio perform better than the strongest benchmark DNN95 model.
Figure 3. Sensitivity analysis of the portfolio performance between our proposed LSDM-KD approach and DNN95 in terms of (a) Portfolio Mean Returns, (b) T-statistics, and (c) Sharpe Ratio on China stock market dataset.

Figure shows the sensitivity analysis of the risk-adjusted portfolio performance between our proposed LSDM-KD approach and DNN95 on China stock market dataset. From the figures, we can find that our LSDM-KD obtained the best risk-adjusted portfolio performance when is set to
. In addition, adjusting portfolio returns through FF3 and FF5 asset pricing models further demonstrate the dominance of our approach. Except the FF5-α when
, all metrics of our method in the long-short portfolio perform better than DNN95 model. When we compare the performance of portfolios in different
, the results show little change in α between the different portfolios, indicating that it manages risk more effectively. In summary, our LSDM-KD framework performs relatively robustly and outperforms the comparison baselines when we vary the scale of
.
6. Conclusion
As an emerging financial services, digital financial networks realise the organic combination of finance and technology, which has become a popular research topic of finance analysis. Currently, financial networks have extremely broad application scenarios, such as mobile payments, stock markets, digital banks, and blockchain systems. On this basis, empirical asset pricing is a challenging task in the field of financial management, which alleviates the urgent need for expensive data support and improves the accuracy of correlation modelling between financial assets.
In this paper, we present a long-short dual-mode knowledge distillation (termed as LSDM-KD) framework for empirical asset pricing models in digital financial networks. Specifically, to address the empirical asset pricing task, our LSDM-KD scheme effectively improves the prediction performance of China stock risk premiums by jointly learning additional knowledge from China and US stock markets. Additionally, our proposed framework can simultaneously handle the inconsistent characteristic signals and different time periods between the stock markets of two countries with high generalisation. Extensive experimental results on two stock market datasets (US and China stock market datasets) demonstrate that our method can significantly improve the performance of empirical asset pricing over classic and advanced methods. The ablation study proves the effectiveness of each component module. The sensitivity analysis of parameters to training periods scale is further explored, which verifies the model stability and feasibility of our framework.
In the future, we plan to improve the model prediction and interpretability of knowledge-enhanced empirical asset pricing models by automating knowledge selection, multimodal knowledge fusion, and establishing an open-source application platform.
Disclosure statement
No potential conflict of interest was reported by the author(s).
Additional information
Funding
References
- Ai, Y., Sun, G., & Kong, T. (2023). Digital finance and stock price crash risk. International Review of Economics & Finance, 88, 607–619. https://doi.org/10.1016/j.iref.2023.07.003
- Butaru, F., Chen, Q., Clark, B., Das, S., Lo, A. W., & Siddique, A. (2016). Risk and risk management in the credit card industry. Journal of Banking & Finance, 72, 218–239. https://doi.org/10.1016/j.jbankfin.2016.07.015
- Chen, C., Zhang, P., Liu, Y., & Liu, J. (2020). Financial quantitative investment using convolutional neural network and deep learning technology. Neurocomputing, 390, 384–390. https://doi.org/10.1016/j.neucom.2019.09.092
- Cooper, M., Gulen, H., & Ion, M. (2024). The use of asset growth in empirical asset pricing models. Journal of Financial Economics, 151, 103746. https://doi.org/10.1016/j.jfineco.2023.103746
- Dai, H., Xu, Y., Chen, G., Dou, W., Tian, C., Wu, X., & He, T. (2022). Rose: Robustly safe charging for wireless power transfer. IEEE Transactions on Mobile Computing, 21(6), 2180–2197. https://doi.org/10.1109/TMC.2020.3032591
- Deng, X., Jiang, Y., Yang, L. T., Lin, M., Yi, L., & Wang, M. (2019). Data fusion based coverage optimization in heterogeneous sensor networks: A survey. Information Fusion, 52, 90–105. https://doi.org/10.1016/j.inffus.2018.11.020
- Deng, X., Wang, B., Liu, W., & Yang, L. T. (2015). Sensor scheduling for multi-modal confident information coverage in sensor networks. IEEE Transactions on Parallel and Distributed Systems, 26(3), 902–913. https://doi.org/10.1109/TPDS.2014.2315193
- Fama, E. F., & French, K. R. (2008). Dissecting anomalies. The Journal of Finance, 63(4), 1653–1678. https://doi.org/10.1111/jofi.2008.63.issue-4
- Goh, J. C., Jiang, F., Tu, J., & Wang, Y. (2013). Can us economic variables predict the Chinese stock market?. Pacific-Basin Finance Journal, 22, 69–87. https://doi.org/10.1016/j.pacfin.2012.10.002
- Gou, J., Sun, L., Yu, B., Wan, S., Ou, W., & Yi, Z. (2022). Multilevel attention-based sample correlations for knowledge distillation. IEEE Transactions on Industrial Informatics, 19(5), 7099–7109. https://doi.org/10.1109/TII.2022.3209672
- Green, J., Hand, J. R., & Zhang, X. F. (2017). The characteristics that provide independent information about average us monthly stock returns. The Review of Financial Studies, 30(12), 4389–4436. https://doi.org/10.1093/rfs/hhx019
- Gu, S., Kelly, B., & Xiu, D. (2020). Empirical asset pricing via machine learning. The Review of Financial Studies, 33(5), 2223–2273. https://doi.org/10.1093/rfs/hhaa009
- Guo, L., Chen, J., Li, S., Li, Y., & Lu, J. (2022). A blockchain and IoT-based lightweight framework for enabling information transparency in supply chain finance. Digital Communications and Networks, 8(4), 576–587. https://doi.org/10.1016/j.dcan.2022.03.020
- Han, X., Song, X., Yao, Y., Xu, X.-S., & Nie, L. (2019). Neural compatibility modeling with probabilistic knowledge distillation. IEEE Transactions on Image Processing, 29, 871–882. https://doi.org/10.1109/TIP.83
- He, K., Zhang, X., Ren, S., & Sun, J. (2016). Deep residual learning for image recognition. In Proceedings of the IEEE conference on computer vision and pattern recognition (pp. 770–778).
- He, S., Shi, K., Liu, C., Guo, B., Chen, J., & Shi, Z. (2022). Collaborative sensing in internet of things: A comprehensive survey. IEEE Communications Surveys & Tutorials, 24(3), 1435–1474. https://doi.org/10.1109/COMST.2022.3187138
- Hinton, G., Vinyals, O., & Dean, J. (2015). Distilling the knowledge in a neural network. arXiv preprint arXiv:1503.02531.
- Huang, W., Ye, M., & Du, B. (2022). Learn from others and be yourself in heterogeneous federated learning. In Proceedings of the IEEE conference on computer vision and pattern recognition (pp. 10143–10153).
- Huang, W., Ye, M., Shi, Z., Li, H., & Du, B. (2023). Rethinking federated learning with domain shift: A prototype view. In Proceedings of the IEEE conference on computer vision and pattern recognition (pp. 16312–16322). IEEE.
- Islam, M. M. (2022). A privacy-preserving transparent central bank digital currency system based on consortium blockchain and unspent transaction outputs. IEEE Transactions on Services Computing, 16(4), 2372–2386. https://doi.org/10.1109/TSC.2022.3226120
- Islam, M. M., Islam, M. K., Shahjalal, M., Chowdhury, M. Z., & Jang, Y. M. (2022). A low-cost cross-border payment system based on auditable cryptocurrency with consortium blockchain: Joint digital currency. IEEE Transactions on Services Computing, 16(3), 1616–1629. https://doi.org/10.1109/TSC.2022.3207224
- Jing, P., Cui, K., Guan, W., Nie, L., & Su, Y. (2023). Category-aware multimodal attention network for fashion compatibility modeling. IEEE Transactions on Multimedia. https://doi.org/10.1109/TMM.2023.3246796
- Jing, P., Cui, K., Zhang, J., Li, Y., & Su, Y. (2023). Multimodal high-order relationship inference network for fashion compatibility modeling in internet of multimedia things. IEEE Internet of Things Journal. https://doi.org/10.1109/JIOT.2023.3285601
- Kelly, B., & Pruitt, S. (2013). Market expectations in the cross-section of present values. The Journal of Finance, 68(5), 1721–1756. https://doi.org/10.1111/jofi.2013.68.issue-5
- Li, S., Lin, M., Wang, Y., Wu, Y., Tian, Y., Shao, L., & Ji, R. (2022). Distilling a powerful student model via online knowledge distillation. IEEE Transactions on Neural Networks and Learning Systems. https://doi.org/10.1109/TNNLS.2022.3152732
- Liang, W., Li, Y., Xie, K., Zhang, D., Li, K.-C., Souri, A., & Li, K. (2023). Spatial-temporal aware inductive graph neural network for C-ITS data recovery. IEEE Transactions on Intelligent Transportation Systems, 24(8), 8431–8442. https://doi.org/10.1109/TITS.2022.3156266
- Liang, W., Tang, M., Long, J., Peng, X., Xu, J., & Li, K.-C. (2019). A secure fabric blockchain-based data transmission technique for industrial Internet-of-Things. IEEE Transactions on Industrial Informatics, 15(6), 3582–3592. https://doi.org/10.1109/TII.9424
- Light, N., Maslov, D., & Rytchkov, O. (2017). Aggregation of information about the cross section of stock returns: A latent variable approach. The Review of Financial Studies, 30(4), 1339–1381. https://doi.org/10.1093/rfs/hhw102
- Ma, W., Chen, Q., Zhou, T., Zhao, S., & Cai, Z. (2023). Using multimodal contrastive knowledge distillation for video-text retrieval. IEEE Transactions on Circuits and Systems for Video Technology. https://doi.org/10.1109/TCSVT.2023.3257193
- Ozbayoglu, A. M., Gudelek, M. U., & Sezer, O. B. (2020). Deep learning for financial applications: A survey. Applied Soft Computing, 93, 106384. https://doi.org/10.1016/j.asoc.2020.106384
- Pukthuanthong, K., & Roll, R. (2009). Global market integration: An alternative measure and its application. Journal of Financial Economics, 94(2), 214–232. https://doi.org/10.1016/j.jfineco.2008.12.004
- Rapach, D., & Zhou, G. (2013). Forecasting stock returns. In Handbook of economic forecasting (Vol. 2, pp. 328–383). Elsevier.
- Rezaei, H., Faaljou, H., & Mansourfar, G. (2021). Stock price prediction using deep learning and frequency decomposition. Expert Systems with Applications, 169, 114332. https://doi.org/10.1016/j.eswa.2020.114332
- Samek, W., Binder, A., Montavon, G., Lapuschkin, S., & Müller, K.-R. (2016). Evaluating the visualization of what a deep neural network has learned. IEEE Transactions on Neural Networks and Learning Systems, 28(11), 2660–2673. https://doi.org/10.1109/TNNLS.5962385
- Shi, N., Tan, L., Li, W., Qi, X., & Yu, K. (2021). A blockchain-empowered AAA scheme in the large-scale HetNet. Digital Communications and Networks, 7(3), 308–316. https://doi.org/10.1016/j.dcan.2020.10.002
- Teng, H. W., Li, Y.-H., & Chang, S.-W. (2020). Machine learning in empirical asset pricing models. In 2020 international conference on pervasive artificial intelligence (ICPAI) (pp. 123–129). IEEE.
- Wang, L., & Yoon, K.-J. (2021). Knowledge distillation and student-teacher learning for visual intelligence: A review and new outlooks. IEEE Transactions on Pattern Analysis and Machine Intelligence, 44(6), 3048–3068. https://doi.org/10.1109/TPAMI.2021.3055564
- Wang, W., Wang, Y., Duan, P., Liu, T., Tong, X., & Cai, Z. (2023). A triple real-time trajectory privacy protection mechanism based on edge computing and blockchain in mobile crowdsourcing. IEEE Transactions on Mobile Computing, 22(10), 5625–5642. https://doi.org/10.1109/TMC.2022.3187047
- Wang, Z., Liu, K., Hu, J., Ren, J., Guo, H., & Yuan, W. (2023). Attrleaks on the edge: Exploiting information leakage from privacy-preserving co-inference. Chinese Journal of Electronics, 32(1), 1–12. https://doi.org/10.23919/cje.2022.00.031
- Welch, I., & Goyal, A. (2008). A comprehensive look at the empirical performance of equity premium prediction. The Review of Financial Studies, 21(4), 1455–1508. https://doi.org/10.1093/rfs/hhm014
- Yao, J., Li, Y., & Tan, C. L. (2000). Option price forecasting using neural networks. Omega, 28(4), 455–466. https://doi.org/10.1016/S0305-0483(99)00066-3
- Yuan, Y.-P., Tan, G. W.-H., & Ooi, K.-B. (2022). Does COVID-19 pandemic motivate privacy self-disclosure in mobile fintech transactions? A privacy-calculus-based dual-stage SEM-ANN analysis. IEEE Transactions on Engineering Management, 71, 2986–3000. https://doi.org/10.1109/TEM.2022.3204285
- Zhang, P., Kang, Z., Yang, T., Zhang, X., Zheng, N., & Sun, J. (2022). Lgd: Label-guided self-distillation for object detection. In Proceedings of the AAAI conference on artificial intelligence (Vol. 36(3), pp. 3309–3317).
- Zhao, B., Cui, Q., Song, R., Qiu, Y., & Liang, J. (2022). Decoupled knowledge distillation. In Proceedings of the IEEE/CVF conference on computer vision and pattern recognition (pp. 11953–11962).