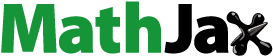
Abstract
Purpose: Radiotherapy prescription dose and dose fractionation protocols vary little between individual patients having the same tumor grade and stage. To personalize radiotherapy a predictive model is needed to simulate radiation response. Previous modeling attempts with multiple variables and parameters have been shown to yield excellent data fits at the cost of non-identifiability and clinically unrealistic results.
Materials and methods: We develop a mathematical model based on a proliferation saturation index (PSI) that is a measurement of pre-treatment tumor volume-to-carrying capacity ratio that modulates intrinsic tumor growth and radiation response rates. In an adaptive Bayesian approach, we utilize an increasing number of data points for individual patients to predict patient-specific responses to subsequent radiation doses.
Results: Model analysis shows that using PSI as the only patient-specific parameter, model simulations can fit longitudinal clinical data with high accuracy (R2=0.84). By analyzing tumor response to radiation using daily CT scans early in the treatment, response to the remaining treatment fractions can be predicted after two weeks with high accuracy (c-index = 0.89).
Conclusion: The PSI model may be suited to forecast treatment response for individual patients and offers actionable decision points for mid-treatment protocol adaptation. The presented work provides an actionable image-derived biomarker prior to and during therapy to personalize and adapt radiotherapy.
Introduction
Radiation therapy (RT) is the single most utilized therapeutic agent in oncology (Torres-Roca Citation2012). Radiation is generally delivered in many small fractions to achieve a large total dose and to provide temporal windows for normal tissue recovery (Beasley et al. Citation2005). The conventional fraction size of 1.8–2.0 Gy once a day, Monday through Friday schedule has been established for logistic convenience and clinical efficacy in multiple cancers, including non-small cell lung cancer (NSCLC). Different alternative fractionation schedules have been prospectively evaluated for efficacy and toxicity (summarized in Ahmed et al. Citation2014) including recent developments towards stereotactic ablative radiotherapy (SABR) with larger doses per fraction (Palma et al. Citation2012). In current clinical practice, the radiation protocol is prescribed prior to treatment. Efforts to adapt RT mid-treatment due to anatomy changes and radiographic response mainly address adapting the target volume to limit toxicity to adjacent organs-at-risk (Hansen et al. Citation2006; Woodford et al. Citation2007). Predicting which patient will respond with tumor regression significant for treatment adaptation and when this will occur is of high clinical value (Caudell et al. Citation2017).
Mathematical and physical modeling has a long history in radiation therapy, including the linear-quadratic dose-dependent survival probability concept (LQ-Model) (Fowler Citation1989), biologically equivalent dose calculations (Fowler Citation2010), tumor cure probability estimations (O'Rourke et al. Citation2009) and normal tissue complication probability analysis (Brodin et al. Citation2018). Inroads have been made more recently into using patient-specific data to simulate untreated tumor growth, predict how individual patients would respond to radiation, and how to evaluate clinical responses (Rockne et al. Citation2010; Corwin et al. Citation2013; Prokopiou et al. Citation2015; Poleszczuk et al. Citation2018).
In a recent modeling study, a variety of tumor growth and treatment response models with different biological resolutions and complexities were analyzed against clinical data of non-small cell lung cancer (Tariq et al. Citation2016). An adaptive Bayesian approach was introduced to derive patient-specific model parameters with increasing availability of longitudinal volumetric response data early in radiation therapy to predict volumetric regression during the remainder of the treatment. This study used the data of twenty five non-small cell lung cancer (NSCLC) patients previously reported (Woodford et al. Citation2007). The tumor volume was available for each patient at all radiation fractionation time points. With such large data set, Akaike Information Criterion (AIC) analysis selected for models with better fits to the data despite larger degrees of freedom (number of parameters) (Burnham and Anderson Citation2002). The chosen model was a two-variable, coupled differential equation model simulating total tumor volume as the sum of living and dead cancer cells. Living cells grow following specific growth laws, and dead cells are degraded at exponential rate. Radiation transitions a fraction of living cells into the dead cancer cell compartment. As only tumor volumes are reported, the parameters in this two-population model are non-identifiable. In fact, the best model fit to the data was obtained with parameters that would kill all living cancer cells after 4–6 Gy in 2–3 days (of the conventional 45 days schedule), and the modeled exponential decay of the dead cell compartment mimicked the overall tumor volume regression (Tariq et al. Citation2016). Whilst providing excellent fits to the clinical data, the model implications are clinically unrealistic and yield predictions that cannot be trusted. Here, we evaluate if a single-variable, single-term, single-parameter model can fit the data equally well, and make trustworthy predictions for radiation response. We have previously developed a single-variable radiotherapy response model that simulates patient-specific volume regression during radiation using the concept of a proliferation saturation index, PSI (Prokopiou et al. Citation2015; Poleszczuk et al. Citation2018). We propose the PSI model to simulate the discussed clinical data with less biological resolution (one-variable, one parameter equation) but higher predictive power.
Tumors are composites of proliferating and hypoxic growth arrested cells. Overall radiation response depends on their respective proportions at irradiation. In multi-compartment mathematical models that distinguish between proliferating and growth-arrested cells, proliferation and oxygenation status-dependent radiation response can be simulated on the cellular level (Enderling et al. Citation2009; Gao et al. Citation2013). Tumor growth in vivo can be approximated by Gompertzian or logistic growth dynamics (Gerlee Citation2013; Benzekry et al. Citation2014; Enderling & Chaplain Citation2014). Initial exponential growth at low cell densities when most cells have access to ample resources decelerates when cells at the core of the tumor become growth-arrested, mainly due to limited space and exhausted intratumoral nutrient supply when resources are consumed by cells closer to the tumor surface. This established the notion of a tumor carrying capacity (K) as the maximum tumor volume that can be supported by a given environment. The tumor carrying capacity is likely to be highly patient-specific as it has co-evolved with the tumor. We propose that the ratio of the initial pre-treatment tumor volume (Vpre-TX) to its carrying capacity ratio (Vpre-TX/K) describes the saturation in overall tumor cell proliferation as the tumor approaches its carrying capacity, which we call proliferation saturation index, PSI = Vpre-TX/K (Prokopiou et al. Citation2015). Tumor volumes close to their carrying capacity, i.e. a high PSI, have only a small proportion of proliferating cells that are most sensitive to radiation-induced damage and are likely to exhibit a shallower response to radiation than tumors with lower PSI.
Materials and methods
Mathematical model
We propose logistic growth of tumor volumes that decreases proportional to the intrinsic potential doubling time, day−1, scaled by the current environmental condition-dependent carrying capacity (K):
(1)
(1)
where, V(t) is the tumor volume at time t, and dV(t)/dt is the change of tumor volume over time. Although carrying capacity has evolved pre-therapy and may itself be variable with time (Hahnfeldt et al. Citation1999), to limit the number of variables and unmeasurable parameters we here assume K to be constant during RT. Following the Norton-Simon hypothesis, treatment response is proportional to tumor growth rate (Norton and Simon Citation1977). Therefore, response to each radiation fraction is modeled as instantaneous volume change with the volume after irradiation being calculated from volume before radiation minus the fraction of proliferating pre-irradiated volume that dies due to irradiation,
where
represents radiation-induced death after dose d following the LQ-model with radiosensitivity parameters α (Gy−1) and β (Gy−2). In line with Tariq’s work and references within we set α/β = 10 Gy for NSCLC (Jin et al. Citation2010; Tariq et al. Citation2016). From this it follows that β = α/10 Gy−2 and we only estimate parameter α herein. It follows from Equationeqn. (1)
(1)
(1) that larger Vpre-TX/K ratios in patients with a high PSI lead to small volume changes and low fraction of proliferating cell in radiation-resistant tumors, whereas tumors with low PSI have more proliferative cells and thus are more radiosensitive. In this model, two patients that present with similar tumor volumes could have a different PSI, which would result in different responses to the same radiotherapy protocol (Poleszczuk et. al. Citation2018). To predict patient-specific radiation response, tumor carrying capacity K and thus PSI needs to be estimated.
Patient population
Twenty-five non-small cell lung cancer patients were treated for NSCLC with standard of care radiation treatment over an 8-week period in the Hi-Art Helical Tomotherapy unit at the London Regional Cancer Program, Ontario, Canada, from 2005 to 2007 (Tariq et al. Citation2016). Volumetric tumor measurements were collected at each treatment fraction.
Data fitting and parameter estimation
We utilize a genetic algorithm to derive parameter values for which the sum of residuals between data and simulation results is minimized (Prokopiou et al. Citation2015). We first find the best parameter values () for each individual patient, i. Then we find the best tumor growth and radiation sensitivity parameters that are uniform across all patients (
) with only PSI being patient-specific, PSIi. To compare the 3-parameter and 1-parameter models, we calculate the AIC using
(2)
(2)
where, k is the number of model parameters, n is the number of data samples, and RRS is the residual sum of squares between model simulation and data.
Adaptive Bayesian prediction
The twenty-five patients were randomized into model training (13 patients) and prediction (12 patients) cohorts. Uniform tumor growth and radiation response parameters () are derived for patients in the model training cohort, that are then kept constant for patients in the prediction cohort. For adaptive treatment response prediction, we follow the approach by Tariq et al. (Citation2016). Using growth rate and radiation response parameters learned from the training cohort, patient-specific PSIi are derived from a genetic algorithm approach that minimizes the least square error between model simulation and clinical data from the first treatment week. Radiation responses are then simulated using for the remaining treatment weeks. A concordance C-index is calculated as a measure of the difference between the model-predicted and clinically observed tumor volumes for each patient to evaluate the prediction performance of the mathematical model (Harrell et al. Citation1982; Walker et al. Citation2017). A C-index = 0.5 represents poor predictive ability (random guessing), and as the C-index approaches 1.0 it represents a very strong predictive ability of the model. After each subsequent treatment week, PSIi is re-estimated using all patient data up to that time point, and treatment response prediction is re-evaluated for the remainder of radiation therapy. The distribution of C-indices over all patient simulations is reported to evaluate the prediction performance of the patient-specific PSI given the data up to each week during treatment.
Results
PSI model fits the data with individual patient-specific parameters
The PSI model with parameter sets [λi, αi, PSIi] fits the data for each individual patient i with high accuracy (overall R2=0.91, 0.0–0.98; ). Median tumor growth rate was λ = 0.01 day−1 (mean 0.07; 0.002–0.99), median radiation sensitivity was α = 0.06 Gy−1 (mean 0.08; 0.013–0.312), and median PSI was PSI = 0.88 (mean 0.7; 0.05–0.999; ). As in the Tariq study (Tariq et al. Citation2016) the best fit to individual patient’s data was achieved for patient 9 (R2=0.98, ). A patient with average mean squared error between model and data (R2=0.81) is shown in .
Figure 1. Simulated patient-specific tumor volume regression during radiotherapy. (A) Correlation of simulated volumes and measured volumes for all 25 patients (R2=0.91). (B) Distribution of model parameter values across all 25 patients. (C) Patient 9 with the best fit of the mathematical model (blue curve) to the data (red circles, R2=0.98). (D) Patient 6 with an average fit of the model to the data (R2=0.81).

PSI model fits the data with PSI as single patient-specific parameter
The standard deviation of the distribution of tumor growth rate λi and radiation sensitivity αi are significantly smaller than the deviation of the PSIi distribution (coefficient of variation CoV = 150%; ). This suggests that PSI may be contributing more to patient-specific treatment responses, and that tumor growth rate λ and radiation sensitivity α may be kept uniform across all patients. Simultaneous fit of the model to all patient data yields uniform tumor growth parameter λ = 0.012 day−1 and radiation sensitivity α = 0.029 Gy−1 with only patient-specific PSI. This one-parameter model slightly reduces quality of fit (R2=0.84; ) but yields a significantly lower median AIC (−4.5 vs. 0.45, p = .00017). As model parameters are non-orthogonal, keeping λ and α constant reduces the coefficient of variation for the distribution of PSI across all patients compared to the three-parameter model (CoV = 46% vs. CoV = 150%; ). Patient 9 with the best three-parameter model fit remains the best fit of the 1-parameter model to the data with the goodness of fit only dropping one percentage point (R2=0.97; ). Patient 6 remains an average-fit patient 6 (R2=0.77; ).
Figure 2. Simulated patient-specific tumor volume regression during radiotherapy with only PSI as patient-specific parameter with derived uniform tumor growth parameter λ = 0.012 day−1 and radiation sensitivity α = 0.029 Gy−1. (A) Correlation of simulated volumes and measured volumes for all 25 patients (R2=0.84). (B) Distribution of PSI across all 25 patients. (C) Patient 9 with the best fit of the mathematical model to the data (R2=0.97). (D) Patient 6 with an average fit of the model to the data (R2=0.77).

Adaptive Bayesian prediction of treatment responses
shows Bayesian prediction plots for sample patients with varying quality of prediction performances (C-index). shows the variation of C-indices dependent on increasing patient-specific data used for PSI calculation for predictions. With patient-specific tumor volumes of one treatment week and growth and death parameters from the model training cohort, the median C-index >0.86 (0.67–0.98) is significantly restricted indicating an accuracy in prediction of NSCLC response to radiotherapy as soon as week 2 of therapy.
Figure 3. Adaptive Bayesian prediction of radiation response. Model training derived parameters are λ = 0.001 day−1 and α = 0.069 Gy−1. Red circles: patient data. Colored curves: Model-predicted trajectories. Colored numbers: Derived PSI for the data up to marked time point. (A) Patient 14 with high prediction accuracy after 2 weeks data. (B) Patient 4 with median prediction accuracy. (C) Patient 6 with lowest prediction accuracy. Using just four weeks of data, subsequent responses can be predicted with high accuracy. (D) Distribution of C-index for prediction accuracy for all 25 patients as a function of number of weeks used to derive patient-specific PSIs.

Discussion
Mathematical modeling is becoming an emerging tool in simulating clinical oncology data. Whilst the field is still in its infancy, most work is focused on analyzing retrospective data to build mathematical models and evaluate their predictive potential for patient-specific treatment responses (Rockne et al. Citation2010; Yankeelov et al. Citation2015; Geng et al. Citation2017). The art of the trade is to balance and synergize available data, model complexity and model usefulness. From limited resolution of clinical data, reconstruction of radiobiological parameters may be an ill-posed problem (Chvetsov et al. Citation2015). With introduction of additional variables that have not and cannot be identified longitudinally in a noninvasive manner should be met with caution as it may render the model non-identifiable and generate clinically unrealistic predictions (Chvetsov et al. Citation2014; Tariq et al. Citation2016).
Here we have proposed to use a simple mathematical model of tumor growth and radiation therapy response that has one patient-specific parameter. The proliferation saturation index, PSI, a measure of the pre-treatment tumor volume-to-carrying capacity ratio in individual patients, is undoubtedly a gross oversimplification of the complex biology underlying tumor growth and response to radiation. However, despite its simplicity or maybe because of its simplicity, the PSI model may be able to derive patient-specific cancer and cancer response properties that could be predictive and prognostic. We set out to re-evaluate the radiation response of twenty-five NSCLC patients with the simple PSI model (Prokopiou et al. Citation2015) and compare its adaptive Bayesian prediction power to the more complex model introduced by Tariq et al. (Citation2016). In our simple logistic growth and radiation response model, the model parameters tumor growth rate, carrying capacity, and radiation sensitivity are not independent. Changing one of the three parameters could yield identical fits to longitudinal patient data after adaptation of the other two parameters. By analyzing model fits, we identified the tumor carrying capacity, and in particular the pre-treatment tumor volume-to-carrying capacity ratio, as the most likely driver of interpatient heterogeneity in radiotherapy responses. The error introduced by a uniform tumor growth rate and radiosensitivity for all patients is kept small as both parameters are multiplied by a factor containing the remaining lose parameter, the patient-specific carrying capacity. Despite a slight reduction in goodness of fit, fixing the growth and death parameters enables higher confidence in calculation of PSI and ultimately higher predictive power. The presented work is retrospective in nature and limited to a previously published dataset in NSCLC. Before general conclusions can be drawn about the applicability of the PSI framework for NSCLC or other tumors treated with radiation, validation in an independent cohort is of utmost importance.
The model assumed that the underlying dynamics follow logistic growth. Different growth laws may provide equally good fits of to the data but may predict grossly different responses to therapy (Poleszczuk et al. Citation2018). Therefore, as mathematical oncologists we are well advised to develop, compare, and compete multiple models with different assumptions and complexities against each other to evaluate where model predictions disagree or agree with one another. This may raise confidence in in silico predictions and have highest chances of success for clinical translation.
Disclosure statement
The authors declare no conflicts of interest.
Additional information
Funding
Notes on contributors
Enakshi D. Sunassee
Enakshi D. Sunassee, is a senior undergraduate student in Chemical Engineering, and a research intern in Dr. Enderling's research group at Moffitt Cancer Center, Tampa, FL, USA.
Dean Tan
Dean Tan, was a HIP IMO high school intern in the Integrated Mathematical Oncology department at Moffitt Cancer Center when he contributed to this study, and is currently a freshman at Northwestern University, Chicago, IL, USA.
Nathan Ji
Nathan Ji, was a HIP IMO high school intern in the Integrated Mathematical Oncology department at Moffitt Cancer Center when he contributed to this study, and is currently a freshman at Johns Hopkins University, Baltimore, MD, USA.
Renee Brady
Renee Brady, Ph.D. in applied mathematics, works as a mathematical oncologist in the Integrated Mathematical Oncology department at Moffitt Cancer Center, Tampa, FL, USA
Eduardo G. Moros
Eduardo G. Moros, Ph.D. in mechanical engineering, is chief of medical physics in the department of Radiation Oncology at Moffitt Cancer Center, Tampa, FL, USA.
Jimmy J. Caudell
Jimmy J. Caudell, M.D., Ph.D. in molecular biology, is director of research as well as the section chief of head and neck in the department of Radiation Oncology at Moffitt Cancer Center, Tampa, FL, USA.
Slav Yartsev
Slav Yartsev, Ph.D., D.Sc., works as a medical physicist in the London Regional Cancer Program at the University of Western Ontario, London, ON, CA.
Heiko Enderling
Heiko Enderling, Ph.D. in mathematical biology, directs a research group on Quantitative Personalized Oncology at the Integrated Mathematical Oncology department, and is Director for Education and Outreach at the Physical Sciences in Oncology Center at Moffitt Cancer Center, Tampa, FL, USA.
References
- Ahmed KA, Correa CR, Dilling TJ, Rao NG, Shridhar R, Trotti AM, Wilder RB, Caudell JJ. 2014. Altered fractionation schedules in radiation treatment: a review. Semin Oncol. 41:730–750.
- Beasley M, Driver D, Dobbs HJ. 2005. Complications of radiotherapy: improving the therapeutic index. Cancer Imaging. 5:78–84.
- Benzekry S, Lamont C, Beheshti A, Tracz A, Ebos JML, Hlatky L, Hahnfeldt P. 2014. Classical mathematical models for description and prediction of experimental tumor growth. F. Mac Gabhann, ed. PLoS Comput Biol. 10:e1003800.
- Brodin NP, Kabarriti R, Garg MK, Guha C, Tomé WA. 2018. Systematic review of normal tissue complication models relevant to standard fractionation radiation therapy of the head and neck region published after the QUANTEC reports. Int J Radiat Oncol Biol Phys. 100:391–407.
- Burnham KP, Anderson DR. 2002. Model selection and multimodel inference: a practical information-theoretic approach. 2nd ed., New York: Springer-Verlag.
- Caudell JJ, Torres-Roca JF, Gillies RJ, Enderling H, Kim S, Rishi A, Moros EG, Harrison LB. 2017. The future of personalised radiotherapy for head and neck cancer. Lancet Oncol. 18:e266–e273.
- Chvetsov AV, Sandison GA, Schwartz JL, Rengan R. 2015. Ill-posed problem and regularization in reconstruction of radiobiological parameters from serial tumor imaging data. Phys Med Biol. 60:8491–8503.
- Chvetsov AV, Yartsev S, Schwartz JL, Mayr N. 2014. Assessment of interpatient heterogeneity in tumor radiosensitivity for nonsmall cell lung cancer using tumor-volume variation data. Med Phys. 41:064101.
- Corwin D, Holdsworth C, Rockne RC, Trister AD, Mrugala MM, Rockhill JK, Stewart RD, Phillips M, Swanson KR. 2013. Toward patient-specific, biologically optimized radiation therapy plans for the treatment of glioblastoma. N. Cordes, ed. PloS One. 8:e79115.
- Enderling H, Chaplain MAJ. 2014. Mathematical modeling of tumor growth and treatment. Curr Pharm Des. 20:4934–4940.
- Enderling H, Park D, Hlatky L, Hahnfeldt P. 2009. The importance of spatial distribution of stemness and proliferation state in determining tumor radioresponse. Math Model Nat Phenom. 4:117–133.
- Fowler JF. 2010. 21 years of biologically effective dose. Br J Radiol. 83:554–568.
- Fowler JF. 1989. The linear-quadratic formula and progress in fractionated radiotherapy. Br J Radiol. 62:679–694.
- Gao X, McDonald JT, Hlatky L, Enderling H. 2013. Acute and fractionated irradiation differentially modulate glioma stem cell division kinetics. Cancer Res. 73:1481–1490.
- Geng C, Paganetti H, Grassberger C. 2017. Prediction of treatment response for combined chemo- and radiation therapy for non-small cell lung cancer patients using a bio-mathematical model. Sci Rep. 7:13542.
- Gerlee P. 2013. The model muddle: in search of tumor growth laws. Cancer Res. 73:2407–2411.
- Hahnfeldt P, et al. 1999. Tumor development under angiogenic signaling: a dynamical theory of tumor growth, treatment response, and postvascular dormancy. Cancer Res. 59:4770–4775.
- Hansen EK, Bucci MK, Quivey JM, Weinberg V, Xia P. 2006. Repeat CT imaging and replanning during the course of IMRT for head-and-neck cancer. Int J Radiat Oncol Biol Phys. 64:355–362.
- Harrell FE, Califf RM, Pryor DB, Lee KL, Rosati RA. 1982. Evaluating the yield of medical tests. JAMA. 247:2543–2546.
- Jin J-Y, Kong F-M, Chetty IJ, Ajlouni M, Ryu S, Ten Haken R, Movsas B. 2010. Impact of fraction size on lung radiation toxicity: hypofractionation may be beneficial in dose escalation of radiotherapy for lung cancers. Int J Radiat Oncol Biol Phys. 76:782–788.
- Norton L, Simon R. 1977. Growth curve of an experimental solid tumor following radiotherapy. J Natl Cancer Instit. 58:1735–1741.
- O'Rourke SFC, McAneney H, Hillen T. 2009. Linear quadratic and tumour control probability modelling in external beam radiotherapy. J Math Biol. 58:799–817.
- Palma DA, et al. 2012. Stereotactic ablative radiotherapy for comprehensive treatment of oligometastatic tumors (SABR-COMET): study protocol for a randomized phase II trial. BMC Cancer. 12:305.
- Poleszczuk J, Walker R, Moros EG, Latifi K, Caudell JJ, Enderling H. 2018. Predicting patient-specific radiotherapy protocols based on mathematical model choice for proliferation saturation index. Bull Math Biol. 80:1195–1206.
- Prokopiou S, et al. 2015. A proliferation saturation index to predict radiation response and personalize radiotherapy fractionation. Radiat Oncol (London, England). 10:159.
- Rockne R, Rockhill JK, Mrugala M, Spence AM, Kalet I, Hendrickson K, Lai A, Cloughesy T, Alvord EC, Swanson KR, et al. 2010. Predicting the efficacy of radiotherapy in individual glioblastoma patients in vivo: a mathematical modeling approach. Phys Med Biol. 55:3271–3285.
- Tariq I, Chen T, Kirkby NF, Jena R. 2016. Modelling and Bayesian adaptive prediction of individual patients’ tumour volume change during radiotherapy. Phys Med Biol. 61:2145–2161.
- Torres-Roca JF. 2012. A molecular assay of tumor radiosensitivity: a roadmap towards biology-based personalized radiation therapy. Person Med. 9:547–557.
- Walker R, Mejia J, Lee JK, Pimiento JM, Malafa M, Giuliano AR, Coppola D, Enderling H. 2017. Personalizing gastric cancer screening with predictive modeling of disease progression biomarkers. Appl Immunohistochem Mol Morphol. [accessed 2017 Oct 27]. DOI:10.1097/PAI.0000000000000598.
- Woodford C, Yartsev S, Dar AR, Bauman G, Van Dyk J. 2007. Adaptive radiotherapy planning on decreasing gross tumor volumes as seen on megavoltage computed tomography images. Int J Radiat Oncol Biol Phys. 69:1316–1322.
- Yankeelov TE, Quaranta V, Evans KJ, Rericha EC. 2015. Toward a science of tumor forecasting for clinical oncology. Cancer Res. 75:918–923.