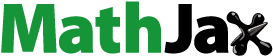
Abstract
Purpose
Benchmark dose (BMD) modeling is a method commonly used in chemical toxicology to identify the point of departure (POD) from a dose-response curve linked to a health-related outcome. Recently, its application in the analysis of transcriptional data for quantitative adverse outcome pathway (AOP) development is being explored. As AOPs are informed by diverse data types, it is important to understand the impact of study parameters such as dose selection, the number of replicates and dose range on BMD outputs for radiation-induced genes and pathways.
Materials and methods
Data were selected from the Gene Expression Omnibus (GSE52403) that featured gene expression profiles of peripheral blood samples from C57BL/6 mice 6 hours post-exposure to 137Cs gamma-radiation at 0, 1, 2, 3, 4.5, 6, 8 and 10.5 Gy. The dataset comprised a broad dose range over multiple dose points with consistent dose spacing and multiple biological replicates. This dataset was ideal for systematically transforming across three categories: (1) dose range, (2) dose-spacing and (3) number of controls/replicates. Across these categories, 29 transformed datasets were compared to the original dataset to determine the impact of each transformation on the BMD outputs.
Results
Most of the experimental changes did not impact the BMD outputs. The transformed datasets were largely consistent with the original dataset in terms of the number of reproduced genes modeled and absolute BMD values for genes and pathways. Variations in dose selection identified the importance of the absolute value of the lowest and second dose. It was determined that dose selection should include at least two doses <1 Gy and two >5 Gy to achieve meaningful BMD outputs. Changes to the number of biological replicates in the control and non-zero dose groups impacted the overall accuracy and precision of the BMD outputs as well as the ability to fit dose-response models consistent with the original dataset.
Conclusion
Successful application of transcriptomic BMD modeling for radiation datasets requires considerations of the exposure dose and the number of biological replicates. Most important is the selection of the lowest doses and dose spacing. Reflections on these parameters in experimental design will provide meaningful BMD outputs that could correlate well to apical endpoints of relevance to radiation exposure assessment.
1. Introduction
Scientific knowledge emerging over recent decades indicates that at radiation exposures relevant to public and occupational levels, the risk of adverse health outcomes is uncertain (ICRP Citation2007, Citation2012; NCRP Citation2015, Citation2018). As transcriptional changes are one of the earliest quantifiable effects of stressor exposures, transcriptomic studies offer opportunities to improve understanding of low-level exposures, particularly when integrated within a systems biology approach such as the adverse outcome pathway (AOP) framework (Ankley et al. Citation2010). In this framework, AOP components known as key events are identified to describe the path of disease progression using weighted evidence from all types of studies that support the modified Bradford-Hill criteria (OECD (Organisation for Economic Co-operation and Development) Citation2017, Citation2018). Alongside conventional endpoints, transcriptional dose-response evidence is valuable for discerning underlying mechanisms and connectivity of the key events within AOPs, thereby, improving understanding of risk from low-dose exposures (Cassman Citation2005; Brockmeier et al. Citation2017).
With the wealth of transcriptomic data in the radiation field and the recognition of these as early biological effects that could be correlated to overt phenotypic changes, their value to support risk assessment strategies needs to be explored. Indeed, efforts internationally (e.g. NASA Genelab, Space Omics Topical Team, Organisation for Economic Cooperation and Development) are recognizing the importance of transcriptomic data and initiatives are underway to produce frameworks for the harmonized reporting and use of ‘transcriptomic’ data in risk assessment (Harrill et al. Citation2019; Madrigal et al. Citation2020; Harrill et al. Citation2021). The European Space Agency has performed transcriptomic analysis of life science experiments during spaceflight to gain a global assessment of early molecular events that underlie phenotypic maladaptations from space travel (Hasin et al. Citation2017). These types of studies and those deposited in data repositories such as NASA Genelab and Gene Omnibus Expression are valuable datasets for exploring in radiation risk assessment.
Although robust radiation-responsive genes and pathways have been identified, the dose at which these responses are perturbed has not been examined particularly in relation to disease progression. Additionally, there is a lack of understanding of the consistency of these dose values across different organs/tissues/cell types and for various radiation parameters (dose and dose-rate of exposure as well as exposure type). Integration of this information within the AOP framework will allow for an understanding of early transcriptional genotoxic markers associated with key events in an AOP and their linkages to disease progression, including information on the lowest dose for activation. This knowledge may then be used to support or refine dose limits, which are currently derived using high dose data extrapolated to low doses using cancer risk models that are not accurate for use on non-cancer outcomes and early predictive endpoints linked to adversity. Definitive steps to make linkages between transcriptional responses and risk estimates have yet to be demonstrated. Recent efforts from the chemical field using novel modeling approaches could be leveraged and extended for application in the radiation field to address relevant regulatory questions related to defining dose of risk estimates for chronic low dose exposures (Chauhan et al. Citation2019b, Citation2020a, Citation2020b, Citation2021a).
In the chemical field, benchmark dose (BMD) modeling has been used for decades to model apical endpoints in conventional toxicology studies to identify the dose at which a change relative to background occurs. A BMD provides an alternative to the no-observed-adverse-effect level (NOAEL), which defines the highest dose at which no adverse effect has been detected. Although NOAELs/BMDs are not used in the radiation field, the approach could be informative for identifying dose limits for health risks for chronic low dose exposures where there is considerable uncertainty. The advantage of the BMD approach is that it simplifies data interpretation, and many areas of toxicology are adopting it over the NOAEL as the metric to define the dose at which effects begin to occur. The BMD is specifically defined as the dose value corresponding to some predefined change (e.g. +10%) in the response characterized by a dose-response relationship of an endpoint of interest. This approach is now being applied to transcriptomic datasets to derive points of departure (PODs) for genes and pathways, with emerging evidence demonstrating concordance with conventional toxicity assays (Thomas et al. Citation2013; National Toxicology Program Citation2018; Gannon et al. Citation2019; Phillips et al. Citation2019; Ramaiahgar et al. Citation2019; Gwinn et al. Citation2020; Johnson et al. Citation2020; Rowan-Carroll et al. Citation2021). For example, studies in rodents exposed to chemicals show a high degree of correlation between transcriptional BMD values and those for cancer endpoints (Thomas et al. Citation2013; Moffat et al. Citation2015; Webster et al. Citation2015; Gwinn et al. Citation2020). Furthermore, it has also been shown that BMDs for pathways associated with key events in a chemical mode of action are strong predictors of the dose associated with the adverse effect (Bhat et al. Citation2013; Chepelev et al. Citation2015; Hester et al. Citation2015; Webster et al. Citation2015).
With the use of the BMD approach well established in the chemical field, extending its application to the radiation field may provide new opportunities to understand low-dose exposures to ionizing radiation. BMD modeling could be explored using currently available transcriptional data, featuring different radiation types, doses, dose rates, tissue types and time-points. Indeed, our group has applied the BMD approach to both radiation data collected “in-house” and datasets available through the Gene Expression Omnibus (GEO) (Chauhan et al. Citation2016; Qutob et al. Citation2018; Chauhan et al. Citation2019a, Citation2021b). The results show some valuable applications of transcriptomic BMD modeling related to radiation qualities and cell/tissue/organ sensitivities. Broadly, we envision transcriptional BMDs may: (1) identify mechanistic pathways and associated low dose values for activation and correlation with sub-pathological effects in support of refining current safety limits and informing risk assessment strategies; (2) be used to compare tissue/organ/subcellular level responses, providing information on weighting factors; and (3) be used to compare radiation qualities informing their relative biological effectiveness. Past publications by our group have made initial efforts to demonstrate this using available transcriptomic data (Chauhan et al. Citation2016; Qutob et al. Citation2018; Chauhan et al. Citation2019a, Citation2021b). Furthermore, transcriptional BMDs can be used for discerning differences in BMDs for cancer and non-cancer outcomes.
Preliminary assessment of the BMD approach using radiation datasets found it to be reproducible for studies conducted in very similar experimental conditions using equivalent dose points (Chauhan et al. Citation2016); however, it was noted when comparing ionizing radiation studies that variations in dose range may be a critical driver of the modeling output and hence, the derived BMDs (Chauhan et al. Citation2021b). Thus, a critical evaluation of the impact of dose selection and dose range on BMD outputs is needed for applicability in the comparison of disparate datasets conducted under different experimental conditions for quantitative AOP development. In addition, it is important to assess the minimal biological replicates needed to ensure precision in the BMD outputs to ensure valid interpretation of results. Although the Environmental Protection Agency provides guidance (United States Environmental Protection Agency (US EPA) Citation2012) on elements of experimental design best suited for BMD analyses, this has been derived from studies generated in the chemical toxicity field on individual apical endpoints rather than the massively parallel sets of gene transcripts that would be modeled in a toxicogenomic experiment. Based on our initial use of the approach, it is of value to validate these recommendations using an ionizing radiation dataset.
In this study, we present an analysis of the impact of systematically varying replicate frequency, dose range and dose spacing on BMD values. In selecting a dataset for this purpose, considerations were based on a study that spanned a broad dose range, used a large sample size, and comprised a number of consistently spaced dose points. With these criteria, manipulation of the dataset would be facilitated, allowing for a comprehensive understanding of the impact of the removal of doses and replicates on the BMD outputs, including the effectiveness to extrapolate to the low dose range, where often there is limited data. For this purpose, a study by Lucas et al. (Citation2014) was selected that met our criteria for doses and sample type for the purpose of the analysis. The study examines global gene expression in blood samples of total body irradiated mice. Relative comparisons were made across common genes and pathways for the different transformations, with no interpolations on the meaning of the absolute BMD values in the context of radiation protection. The systematic assessment of the Lucas et al. (Citation2014) dataset allowed for an understanding of design choice related to dose selection and replicate frequency on BMD outputs
2. Materials and methods
2.1. Datasets
Data were obtained from GEO and were originally collected by Lucas et al. (Citation2014) (GEO accession number: GSE52403). The dataset contains gene expression profiles of peripheral blood samples harvested from male and female C57BL/6 mice 6 hours post-exposure to total body irradiation from a 137Cs gamma source at dose levels of 0, 1, 2, 3, 4.5, 6, 8 and 10.5 Gy. Samples at 0 Gy serve as the control measurements. The gene expression profiles were produced using an Affymetrix mouse 430 A 2.0 microarray. The dataset was ideal as it used a broad dose range with a sufficient number of biological replicates such that it could be manipulated and re-analyzed for the purposes of this study. The selected data is henceforth referred to as the nominal dataset.
2.2. Systematic changes made to the nominal dataset
The nominal dataset was manipulated across different parameters as outlined in to generate a transformed dataset upon which a BMD analysis was performed. The three parameters define experimental categories. Their definitions and rationale are as follows:
Table 1. Summary of the transformed datasets for each experimental category. Doses that are shaded denote those removed from each of the specific analyses.
Removal of dose values (DV) – For this series of datasets, one or more dose values from the original dataset were removed in a systematic manner. The justification for exploring this type of variation was to (1) identify if specific dose values are more crucial to the accuracy of BMD values than others; and (2) assess if a reduced number of doses can be used to achieve similar levels of BMD output to the nominal dataset. This category is divided into two sub-categories in , DV1 – removal of a single dose, DV2 – removal of two or more doses.
Variation in the dose range upper limit (UL) – These experiments examined the impact of the highest dose value on the transcriptomic BMDs. It is expected that the highest dose may constrain the fit of the dose-response curve to the data, and thus impact the BMD outputs at the gene and pathway level. Inclusion of a high dose may also shift the overall mean or median BMD value of a group of genes if there exist genes that preferentially only fit with the inclusion of higher doses.
Variation of replicate frequency (RF) – These experiments examined the impact of the number of control and dose replicates in an experiment on the BMD outputs. The number of replicates is expected to have a statistical impact on the data, affecting the accuracy and precision of the BMDs relative to the nominal dataset.
provides a summary of all transformed datasets for each of the three experimental categories: DV, UL and RF. In total there are 29 transformed datasets.
2.3. BMD analysis
BMD analyses were performed on the nominal and transformed datasets using the BMDExpress software v2.3 (Phillips et al. Citation2019) following the methodology discussed by Chauhan et al. (Citation2021b). Briefly, data were pre-filtered using the built-in Williams’ Trend Test feature with a p-value cutoff threshold of ≤.05 and |fold-change| of ≥2.0. Genes passing these criteria were then modeled by linear, exponential, power, polynomial and Hill functions to find the best fit curve and identify the BMD value and the corresponding 95% CI with a lower (BMDL) and upper (BMDU) bound value. A set of filters was applied to the best BMD, BMDL and BMDU values to remove outliers and erroneous results that did not model well (best-fit criteria). Here, best denotes the best fit model of the aforementioned functions. The filter criteria used to select non-erroneous values with good fit values were as follows (as verbatim in BMDExpress v2.3):
Best BMD ≤ Highest dose of the dataset
Best BMDU/BMD ≤20
Best BMD/BMDL ≤20
Best BMDU/BMDL ≤40
Best fitPValue ≥.1
Genes passing the above criteria were then assigned to gene sets in the Reactome pathway library (Jassal et al. Citation2020). Similarly, pathways were also subject to filters according to their constituent genes. The filters used were as follows (as verbatim in BMDExpress v2.3):
Genes That Passed All Filters ≥3
Percentage ≥5
The Percentage variable refers to the percentage of genes from the array that passed the gene filter criteria and were used as input to the BMD analysis. A full description for each of the above parameters as defined by BMD Express v2.3 can be found here: https://bmdexpress-2.readthedocs.io/en/feature-readthedocs/functional_classifications. For each dataset the following information was assessed: the total number of genes and pathways modeled, best-fit model, BMD median, and the 95% CI of the BMD value with respective lower (BMDL) and upper (BMDU) limits. The number and type of genes and pathways that were similar and different across each dataset were also examined.
2.4. Measures of variation for BMD outputs
We define two variables to measure variations of the BMD outputs across the transformed datasets when compared to the nominal dataset. For each gene and pathway common to both a transformed dataset and the nominal dataset, the percentage variation from its nominal BMD value, v% is defined as follows:
(1)
(1)
where
is the BMD of a specific gene or pathway (i) from the transformed dataset and xi is the corresponding BMD value from the nominal dataset.
In this work, a reproduced gene or pathway for a transformed dataset is one that passes the filter selection criteria for both the transformed dataset and the nominal dataset. We define the reproducibility, R% of genes or pathways of a transformed dataset as follows:
(2)
(2)
where NN is defined as the number of genes or pathways which passed the filter selection criteria in the nominal dataset and
is the number of genes or pathways that passed the criteria for both the nominal dataset and the transformed dataset (j). Therefore, for each transformed dataset, the ratio
/NN is always less than or equal to one.
3. Results
Following analysis of the nominal and transformed datasets with the BMD Express v2.3 software and application of quality selection criteria, outputs were compiled such that comparisons between the nominal and transformed datasets could be made to assess the following:
Reproducibility of modeled genes and pathways
Precision and accuracy of BMD values across reproducible genes and pathways
Best-fit models of reproducible genes
Alterations in BMD values for established radiation-relevant genes and pathways
Categories i–iii investigate the reproducibility of genes and pathways at three successive levels of granularity. Category iv is focused on a specific subset of genes and pathways relevant to radiation exposure.
Due to a large number of comparisons possible between datasets, only general trends across each of the three transformed categories (DV, RF and UL) and notable observations specific to certainly transformed datasets are presented here. Complete tabulations of the BMD outputs across all 29 transformed datasets appear in the appendices.
3.1. Reproducibility of modeled genes and pathways
shows the gene and pathway reproducibility of the transformed datasets. Along the x-axis of each Figure are the average BMDL and BMDU (error bars) and BMD values (points); a summary is provided in . The datasets DV2-8 and DV2-9 both had fewer than 100 reproduced genes and zero pathways that were reproduced from the nominal dataset. These datasets are excluded from further discussion. In general, most of the other transformed datasets reproduced average gene and pathway BMD values of the nominal dataset within a narrow dose range. The mean gene and pathway BMD value of the nominal dataset was 0.67 Gy and 0.48 Gy respectively. The ranges across the DV, UL and RF categories for gene BMDs were, respectively, 0.65–1.03 Gy, 0.47–0.87 Gy and 0.58–0.65 Gy. For pathways, it was found to be 0.37–0.78 Gy (DV), 0.28–0.56 Gy (UL) and 0.38–0.48 Gy (RF). Most transformed datasets showed high reproducibility (∼80% or more) at the gene and pathway level. The poorest reproducibility (∼60% or less) was observed for datasets DV1-1, DV2-6, UL3 and UL4.
Figure 1. Scatter plots of the mean gene (left) and pathway (right) BMDs for the DV (panels A and B), RF (panel C) and UL (panel D) experimental categories. Each data point represents the mean gene or pathway BMD of a dataset with an error bar that spans the range of the mean BMDL and BMDU values. For reference, the nominal data is shown in black and y-axis values correspond to the reproducibility of each dataset (as defined in EquationEquation (2)(2)
(2) ).

Table 2. Summary of the range of mean gene and pathway BMD values across the nominal and the transformed datasets of the three experimental categories DV, RF and UL.
3.2. Precision and accuracy of BMD values across reproducible genes and pathways
For reproducible genes and pathways, values of v% were calculated. As an example, the distribution of v% is shown for three datasets (RF2, RF6 and RF9) from the RF experimental category in . These three datasets span most of the range of variation for the RF experimental category and illustrate the impact of these variations on BMD values. The mean (μ) and standard deviation (σ) of these v%-distributions are measured for the accuracy (μ) and precision (σ) of each respective dataset to reproduce the BMD values of reproducible genes and pathways from the nominal dataset. summarizes the ranges of gene-by-gene and pathway-by-pathway variation among common genes and pathways across all transformed datasets within each of the three experimental categories. Values of μ for the DV and UL categories were within 20% for all transformed datasets except DV1-1, DV2-7, UL3 and UL4. In the RF category only four; RF1, RF2, RF4 and RF8 were within 20%. Values of σ spanned 24–123% across all three experimental categories. The greatest of these variations appeared among the RF transformed datasets. A complete summary of these (μ, σ)-statistics for each of the transformed datasets in the RF, DV and UL experimental categories is in Appendix B.
Figure 2. Gene BMD variation (%) for three select datasets transformed according to replicate frequency.

Table 3. Summary of the range of mean (μ) and standard deviation (σ) values for the distribution of v% (see EquationEquation (1)(1)
(1) ) across transformed datasets for each of the three experimental categories.
3.3. Best-fit models of reproducible genes
Reproducible genes are subject to a final comparison of the best-fit models that determine their BMD values. The fit of a model (e.g. polynomial or exponential) to the data points of each dataset is driven by the presence/omission of specific dose values. The transformed datasets of the DV and UL experimental categories explore the best-fit sensitivity to dose value selection. Datasets in the RF category explore the sensitivity with respect to replicate frequency. shows a complete summary of gene BMD values, reproduced nominal genes and the percentage of those modeled by the same best-fit model type between each of the transformed datasets and the nominal dataset. The average absolute variation (|v%|) of gene BMD values between each transformed dataset and the nominal dataset for genes with matching and mismatched best-fit models are also shown. Outside of DV1-1 and DV2-5 the value of |v%| across the DV and UL categories for matching best-fit models is no greater than 21%. For mismatched models, it is no smaller than 35%. Across the RF category values of |v%| range from 9 to 46% for matching best-fit models and 42–100% for mismatched models.
Table 4. Top-level summary of gene BMD values across each transformed dataset. Doses that are shaded denote those removed from each of the specific analyses.
shows the relative percentages of reproduced genes categorized based on their best-fit models across the DV and UL experimental categories. In general, across most of the datasets of the DV category, the proportion of genes modeled by exponential, Hill, linear and polynomial models are consistent with the nominal dataset. This consistency reduces slightly within the DV2 subcategory where two or more doses are removed. Notable exceptions include DV1-1 and UL2, UL3 and UL4 of the UL category. The UL datasets generally favor models with fewer parameters such as a polynomial model of degree 2 (POLY2). No significant changes are observed across the datasets of the RF. An equivalent figure for this category can be found in the Appendix.
Figure 3. The distribution of model types applied to genes across the DV and UL experimental categories. Each model type is denoted by a different color along the horizontal axis. Model types include: polynomials of quadratic (POLY2) and cubic (POLY3) types, linear, Hill and exponential (EXP2, EXP4 and EXP5). The results of the UL1 and DV1-7 datasets are shown as a single result as these datasets apply the same transformation (omission of the 10.5 Gy dose value).

3.4. Alterations in BMD values for established radiation-relevant genes and pathways
To evaluate BMD outputs for established genes and pathways to radiation injury, a specific subset of pathways were chosen that are known to be induced by radiation exposure for further study. The following five pathways relevant to DNA damage/repair and cell response/death were selected: (1) response to external stimuli/stress, (2) DNA double-strand break (DSB) repair, (3) programmed cell death, (4) cell cycle and (5) TP53 regulation. A total of 113 genes were fit to models across these five pathways in the nominal dataset. For the transformed datasets, not all 113 genes passed filtering criteria and were therefore not successfully modeled. A visual summary of these genes and their status (i.e. fit to a model or not) after applying the filter selection criteria is shown in for the DV, RF and UL experimental categories. summarizes the mean, minimum and maximum gene BMD values across all 113 genes for successfully modeled genes within the transformed datasets of the DV and UL categories. The results for the RF category can be found in the appendix.
Figure 4. Filter selection criteria status of 113 genes relevant to 5 pathways associated with DNA repair and cell death. Each column represents a single gene, with each row corresponding to a specific dataset of the DV (top panel), UL (middle panel) and RF (bottom panel) experimental categories. The rows within each panel are ordered from top to bottom in descending order by the number of successfully modeled genes.

Table 5. The mean, minimum and maximum BMD values for each successfully modeled gene from a group of 113 genes specifically relevant to pathways associated with DNA repair and cell death. Doses that are shaded denote those removed from each of the specific analyses.
We then investigated how the individual dose-response curves were modeled across the transformed datasets for a selection of these 113 genes. For example, herein we show the dose-response model fits for MRE11A, POLE4 and RPA1 genes (). Across the datasets, the best fit model types and the constituent parameters for each model varied as a result of the transformed input data. However, the dose-response modeling was not highly impacted by removing doses, with the exception of the 1 Gy dose, which caused the largest variations in modeling relative to the other dose ranges, but only for 1 of the 3 genes examined.
4. Discussion
We investigated how a series of systematic changes to an ionizing radiation exposure dataset impacted BMD outputs. The reproducibility of gene and pathway BMD values at four levels of granularity were explored: (1) overall reproducibility, (2) accuracy and precision of reproduced genes and pathways, (3) best-fit models of reproduced genes and (4) reproducibility of genes relevant to radiation exposure pathways. Despite no data <1 Gy, the broad coverage of doses used in the original study allowed for modeling extrapolation to lower doses enabling comparison of BMD values across the transformed datasets. Ideally, it would have been beneficial to validate the findings with another dataset under similar experimental conditions, but no study was identified for this purpose.
Overall, the results demonstrate that some transformations are more impactful than others. However, the average BMDL, BMDU and BMD values for genes and pathways of each transformed dataset were, for the most part, consistent and within the range of the average BMDL, BMDU and BMDU values of the nominal dataset.
It was noted that 86% of the genes in the nominal dataset had BMD values <1 Gy. Removal of the lowest dose at 1 Gy led to a significant change in BMD outputs with only 46% of the nominal genes being reproduced with low accuracy. This suggests that the inclusion of at least two doses <1 Gy (e.g. 0.1 Gy and 0.5 Gy) would improve the accuracy of BMD outputs and is aligned with the US EPA guidance that indicates it is preferable to have studied with one or more doses near the expected BMD value (United States Environmental Protection Agency (US EPA) Citation2012). Furthermore, for this dataset the average BMD value was biased upwards due to the first non-zero dose point being 2 Gy, forcing the BMD method to interpolate over a wider range to determine a turn-on of the dose-response. As demonstrated for the MRE11A gene, removal of the 1 Gy dose can transform the data into appearing quantal, wherein the dose-response for all non-zero doses is uniform. In these instances, the resulting BMD value can only occur between zero and the lowest non-zero dose, in this case, the 2 Gy. Indeed, US EPA guidance highlights that datasets in which all non-zero doses have essentially the same response level provide limited information on dose-response relationships (United States Environmental Protection Agency (US EPA) Citation2012). Given that gene expression is not quantal data, this result flags an area of particular concern that emphasizes the critical importance of appropriate dose distributions. This is important in the context of developing quantitative AOPs, wherein quantitative results from studies are synthesized to describe the magnitude of change required in upstream key events to evoke change in downstream key events (OECD (Organisation for Economic Co-operation and Development) Citation2017). Development on defining the features of quantitative AOPs is ongoing (Spinu et al. Citation2020) and it is not yet clear how the stochastic effects of ionizing radiation would be treated in this context.
With respect to dose selection in general it was determined that the removal of any single dose >1 Gy does not significantly impact the overall reproducibility of genes from the nominal dataset. However, when considering the removal of two or more doses >1 Gy, the inclusion of at least two doses in each of the subintervals 0–3 Gy (with one being 1 Gy) and >5 Gy (with one being 10.5 Gy) could reproduce ∼90% of the pathway data. Therefore, although the nominal dataset featured seven dose points, through optimized selection of lower and higher doses, this can be reduced to four or five with only a ∼10% loss in BMD output. This is in line with the work by Kuljus et al. (Citation2006) and Slob et al. (Citation2005). Kuljus et al. (Citation2006) used a mathematical treatment for optimizing the experimental design of BMD studies and found that more than four non-zero doses are required. Adding subsequent dose groups after this led to diminishing increases in the quality of the BMD outputs. Slob et al. (Citation2005) identified through simulations that a combination of lower and higher doses is necessary to produce reliable BMD outputs. The results of the UL experimental category highlight the reduced reproducibility of genes when omitting one or more higher dose values. Indeed, in the context of future studies, it is difficult to prescribe the optimal dose selection if the dose response is unknown. However, the results presented here corroborate the importance of dose selection criteria that includes multiple lower and higher dose values for ionizing radiation datasets.
It was also noted that there was a general trend where the percentage of reproduced genes with matched models to those from the nominal dataset decreases as the number of removed dose values increases. This means that in the case of transformed datasets reproducing the same percentage of genes, datasets with fewer dose values are more likely to mis-match models of the dose-response. Such issues could be treated through model averaging, which has developed since its inclusion in the 2012 EPA guidance for BMD modeling (Haber et al. Citation2018) and now features in the latest release of BMD Express (version 3): https://github.com/auerbachs/BMDExpress-3. A second observation relates to the case when at least two doses were removed from the nominal dataset. The average absolute variation of BMD values for genes with matching models was 56% when omitting the 2 Gy dose value compared to 21% for omitting the 3 Gy value. This suggests that lower doses are more important for reproducing BMD values than higher doses. It should also be noted that whilst variations of 56% are large, in the context of this specific dataset, with a nominal average gene BMD value of 0.67 Gy, such variations are equivalent to ∼0.37 Gy and may not be significant at a clinical level with respect to ionizing radiation exposure. Overall, the above effects are of particular importance when combining results from multiple datasets (e.g. for AOP development). Our findings can help determine which study designs for radiation gene set analyses should be given the greatest weight in AOP development.
Differences in the BMD results obtained when the replicate frequency was varied relate primarily to the best fitting statistical models selected for each data set. In this work, the dataset with the lowest control and replicate frequencies [3 control, 3 replicates] was still able to reproduce >90% of the nominal pathways. However, the consequences of a small dataset have a lesser effect on the absolute values of the BMD values, but rather their accuracy (μ) and precision (σ). This loss in accuracy and precision leads to a higher proportion of mismatched models for genes. Ultimately, the control and replicate frequency should be determined based on the specific objective of a given study and the resources available. Studies with low numbers of controls and replicates are not uncommon in cases where there is a natural limit to data collection; e.g. data from emergency scenarios or space missions (McDonald et al. Citation2020).
Finally, we observed that the general trends described above were consistent when only 113 genes relevant to radiation exposure were included. For each transformed dataset, the difference between the mean BMD value relative to the nominal dataset across these 113 genes was consistent with the global mean across all genes. This provides some confidence that future analyses comparing results across a more restricted, smaller set of genes relevant to ionizing radiation would provide meaningful information and yield results consistent with the global gene distribution of their constituent datasets. Such a targeted approach may be a more optimal route for future work when applying the BMD approach to ionizing radiation datasets.
5. Summary and conclusion
We undertook a systematic investigation of the parameters that influence BMD outputs from radiation-based transcriptomic datasets to work toward defining optimal practices for use in radiation research. A single nominal dataset (Lucas et al. Citation2014) was transformed multiple times based on three categories of experimental change. Through comparison of the BMD outputs from each transformed dataset with the nominal output, the impact of dose selection, specifically of lower and higher doses values, emerged as critically important to BMD derivation. In addition, the data highlight the importance of dose selection on best model fit and variability across BMDs for dose values above 1 Gy.
Overall, the robustness of the BMD values and trends presented corroborate preexisting work from other fields. Our results support that BMD analysis of transcriptional data involving ionizing radiation exposure is also applicable and could inform discussions on radiation exposure assessment strategies. In the field of chemical toxicology, the robustness of the BMD approach has previously been discussed by Slob (Citation2014a, Citation2014b), with a specific emphasis that the collation of BMD data across multiple chemicals is encouraged to better probe the true response of an endpoint to chemical stress. This complements the design philosophy of AOPs wherein multiple stressors are considered. It is hoped that this can also be successfully realized for ionizing radiation. It should therefore be noted that the observations here are applicable to a single radiation source (137Cs) and exposure scenario (total body irradiation) from which the original data were collected. Additional work is warranted to explore optimal study designs addressing different scenarios to enable more broad inference across radiation sources, exposures and model systems and also using a dataset that encompasses dose values <1Gy. The results have prompted us to further investigate experimental studies using multiple low-doses to accurately identify gene BMDs following radiation exposure. This work is currently underway and may be extended to include other applicable forms of stressors related to co-exposure scenarios, such as radon and smoking, as well as space travel for future development.
Supplemental Material
Download Zip (174 KB)Acknowledgement
The authors would like to acknowledge Sami Qutob and Lindsay Beaton for critical review. The authors also acknowledge support from the Government of Canada Genomics Research and Development Initiative.
Disclosure statement
The authors report no conflict of interest.
Additional information
Notes on contributors
Robert Stainforth
Robert Stainforth is a physical scientist at Health Canada.
Ngoc Vuong
Ngoc Vuong is a lab technician at Health Canada.
Nadine Adam
Nadine Adam is a biologist at Health Canada.
Byron Kuo
Byron Kuo is a bioinformatic/statistician at Health Canada.
Ruth C. Wilkins
Ruth C. Wilkins is a research scientist at Health Canada.
Carole Yauk
Carole Yauk is a professor at the University of Ottawa.
Afshin Beheshti
Afshin Beheshti is a bioinformatician and principal investigator at the KBR/NASA Ames Research Center.
Vinita Chauhan
Vinita Chauhan is a research scientist at Health Canada.
References
- Ankley GT, Bennett RS, Erickson RJ, Hoff DJ, Hornung MW, Johnson RD, Mount DR, Nichols JW, Russom CL, Schmieder PK, et al. 2010. Adverse outcome pathways: a conceptual framework to support ecotoxicology research and risk assessment. Environ Toxicol Chem. 29(3):730–741.
- Bhat VS, Hester SD, Nesnow S, Eastmond DA. 2013. Concordance of transcriptional and apical benchmark dose levels for conazole-induced liver effects in mice. Toxicol Sci. 136(1):205–215.
- Cassman M. 2005. Barriers to progress in systems biology. Nature. 438(7071):1079.
- Brockmeier EK, Hodges G, Hutchinson TH, Butler E, Hecker M, Tollefsen KE, Garcia-Reyero N, Kille P, Becker D, Chipman K, et al. 2017. The role of omics in the application of adverse outcome pathways for chemical risk assessment. Toxicol Sci. 158(2):252–262.
- Chauhan V, Kuo B, McNamee JP, Wilkins RC, Yauk CL. 2016. Transcriptional benchmark dose modeling: exploring how advances in chemical risk assessment may be applied to the radiation field. Environ Mol Mutagen. 57(8):589–604.
- Chauhan V, Rowan-Carroll A, Gagné R, Kuo B, Williams A, Yauk CL. 2019a. The use of in vitro transcriptional data to identify thresholds of effects in a human lens epithelial cell-line exposed to ionizing radiation. Int J Radiat Biol. 95(2):156–169.
- Chauhan V, Said Z, Daka J, Sadi B, Bijlani D, Marchetti F, Beaton D, Gaw A, Li C, Burtt J, et al. 2019b. Is there a role for the adverse outcome pathway framework to support radiation protection? Int J Radiat Biol. 95(2):225–223.
- Chauhan V, Stricklin D, Cool D. 2020a. The integration of the adverse outcome pathway framework to radiation risk assessment. Int J Radiat Biol. 97(1):60–67.
- Chauhan V, Sherman S, Said Z, Yauk CL, Stainforth R. 2020b. A case example of a radiation-relevant adverse outcome pathway to lung cancer. Int J Radiat Biol. 97(1):68–84.
- Chauhan V, Villeneuve D, Cool D. 2021a. Collaborative efforts are needed among the scientific community to advance the adverse outcome pathway concept in areas of radiation risk assessment. Int J Radiat Biol. 20:1–12.
- Chauhan V, Adam N, Kuo B, Williams A, Yauk CL, Wilkins R, Stainforth, R. 2021b. Meta-analysis of transcriptomic datasets using benchmark dose modeling shows value in supporting radiation risk assessment. Int J Rad Biol. 97(2):1–36.
- Chepelev NL, Moffat ID, Labib S, Bourdon-Lacombe J, Kuo B, Buick JK, Lemieux F, Malik AI, Halappanavar S, Williams A, et al. 2015. Integrating toxicogenomics into human health risk assessment: lessons learned from the benzo[a]pyrene case study. Crit Rev Toxicol. 45(1):44–52.
- Gannon AM, Moreau M, Farmahin R, Thomas RS, Barton-Maclaren TS, Nong A, Curran I, Yauk CL. 2019. Hexabromocyclododecane (HBCD): a case study applying tiered testing for human health risk assessment. Food Chem Toxicol. 131:110581.
- Gwinn WM, Auerbach SS, Parham F, Stout MD, Waidyanatha S, Mutlu E, Collins B, Paules RS, Merrick BA, Ferguson S, et al. 2020. Evaluation of 5-day in vivo rat liver and kidney with high-throughput transcriptomics for estimating benchmark doses of apical outcomes. Toxicol Sci. 176(2):343–354.
- Haber LT, Dourson ML, Allen BC, Hertzberg RC, Parker A, Vincent MJ, Maier A, Boobis AR. 2018. Benchmark dose (BMD) modeling: current practice, issues, and challenges. Crit Rev Toxicol. 48(5):387–415.
- Harrill J, Shah I, Setzer RW, Haggard D, Auerbach S, Judson R, Thomas RS. 2019. Considerations for strategic use of high-throughput transcriptomics chemical screening data in regulator decisions. Curr Opin Toxicol. 15:64–75.
- Harrill JA, Viant MR, Yauk CL, Sachana M, Gant TW, Auerbach SS, Beger RD, Bouhifd M, O'Brien J, Burgoon L, et al. 2021. Progress towards an OECD reporting framework for transcriptomics and metabolomics in regulatory toxicology. Regul Toxicol Pharmacol. 125:105020.
- Hasin Y, Seldin M, Lusis A. 2017. Multi-omics approaches to disease. Genome Biol. 18(1):83.
- Hester S, Eastmond DA, Bhat VS. 2015. Developing toxicogenomics as a research tool by applying benchmark dose-response modelling to inform chemical mode of action and tumorigenic potency. IJBT. 14(1):28–46.
- ICRP 2007. The 2007 recommendations of the international commission on radiological protection. ICRP Publication 103. Ann ICRP. 37(2–4):11–16. https://doi.org/10.1016/j.icrp.2007.10.003.
- ICRP 2012. ICRP statement on tissue reactions/early and late effects of radiation in normal tissues and organs – threshold doses for tissue reactions in a radiation protection context. ICRP publication 118. Ann ICRP. 41(1/2):19–23. https://doi.org/10.1016/j.icrp.2012.02.001.
- Jassal B, Matthews L, Viteri G, Gong C, Lorente P, Fabregat A, Sidiropoulos K, Cook J, Gillespie M, Haw R, et al. 2020. The reactome pathway knowledgebase. Nucleic Acids Res. 48(D1):D498–D503.
- Johnson KJ, Auerbach SS, Costa E. 2020. A rat liver transcriptomic point of departure predicts a prospective liver or non-liver apical point of departure. Toxicol Sci. 176(1):86–102.
- Kuljus K, von Rosen D, Sand S, Victorin K. 2006. Comparing experimental designs for benchmark dose calculations for continuous endpoints. Risk Anal. 26(4):1031–1043.
- Lucas J, Dressman HK, Suchindran S, Nakamura M, Chao NJ, Himburg H, Minor K, Phillips G, Ross J, Abedi M, et al. 2014. A translatable predictor of human radiation exposure. PLOS One. 9(9):e107897.
- McDonald JT, Stainforth R, Miller J, Cahill T, Silveira WAd, Rathi KS, Hardiman G, Taylor D, Costes SV, Chauhan V, et al. 2020. NASA GeneLab platform utilized for biological response to space radiation in animal models. Cancers. 12(2):381–387.
- Madrigal P, Gabel A, Villacampa A, Manzano A, Deane CS, Bezdan D, Carnero-Diaz E, Medina FJ, Hardiman G, Grosse I, et al. 2020. Revamping space-omics in Europe. Cell Syst. 11(6):555–556.
- Moffat I, Chepelev N, Labib S, Bourdon-Lacombe J, Kuo B, Buick JK, Lemieux F, Williams A, Halappanavar S, Malik A, et al. 2015. Comparison of toxicogenomics and traditional approaches to inform mode of action and points of departure in human health risk assessment of benzo[a]pyrene in drinking water. Crit Rev Toxicol. 45(1):1–43.
- National Toxicology Program 2018. NTP research report on national toxicology program approach to genomic dose-response modeling. Research Triangle Park (NC): National Toxicology Program. Natl Toxicol Program Res Rep Ser. April;(RR-05):1-42.
- NCRP 2018. Implications of recent epidemiologic studies for the linear-non threshold model and radiation protection, NCRP commentary. National Council on Radiation Protection and Measurements, 2018. 27.
- NCRP 2015. Health effects of low doses of radiation: perspectives on integrating radiation biology and epidemiology, NCRP commentary No. 24, national council on radiation protection and measurements, Bethesda, MD.
- OECD (Organisation for Economic Co-operation and Development) 2017. Revised guidance document on developing and assessing adverse outcome pathways. OECD environment, health and safety publications, series on testing and assessment, Paris, France. No. 184.
- OECD (Organisation for Economic Co-operation and Development) 2018. Users’ handbook supplement to the guidance document for developing and assessing AOPs. OECD series on adverse outcome pathways No.1.
- Phillips JR, Svoboda DL, Tandon A, Patel S, Sedykh A, Mav D, Kuo B, Yauk CL, Yang L, Thomas RS, et al. 2019. BMDExpress 2: enhanced transcriptomic dose-response analysis workflow. Bioinformatics. 35(10):1780–1782.
- Qutob SS, Chauhan V, Kuo B, Williams A, Yauk CL, McNamee JP, Gollapudi B. 2018. The application of transcriptional benchmark dose modeling for deriving thresholds of effects associated with solar-simulated ultraviolet radiation exposure. Environ Mol Mutagen. 59(6):502–515.
- Ramaiahgar SC, Auerbach SS, Saddler TO, Rice JR, Dunlap PE, Sipes NS, DeVito MJ, Shah RR, Bushel PR, Merrick BA, et al. 2019. The power of resolution: contextualized understanding of biological responses to liver injury chemical using high-throughput transcriptomics and benchmark concentration modeling. Toxicol Sci. 169(2):553–566.
- Rowan-Carroll A, Reardon A, Leingartner K, Gagné R, Williams A, Meier MJ, Kuo B, Bourdon-Lacombe J, Moffat I, Carrier R, et al. 2021. High-throughput transcriptomic analysis of human primary hepatocyte spheroids exposed to per- and polyfluoroalkyl substances as a platform for relative potency characterization. Toxicol Sci. 181(2):199–214.
- Slob W, Moerbeek M, Rauniomaa E, Piersma AH. 2005. A statistical evaluation of toxicity study designs for the estimation of the benchmark dose in continuous endpoints. Toxicol Sci. 84(1):167–185.
- Slob W. 2014a. Benchmark dose and the three Rs. Part I. Getting more information from the same number of animals. Crit Rev Toxicol. 44(7):557–567.
- Slob W. 2014b. Benchmark dose and the three Rs. Part II. Consequencies for study design and animal use. Crit Rev Toxicol. 44(7):568–580.
- Spinu N, Cronin MTD, Enoch SJ, Madden JC, Worth AP. 2020. Quantitative adverse outcome pathway (qAOP) models for toxicity prediction. Arch Toxicol. 94(5):1497–1510.
- Thomas RS, Wesselkamper SC, Wang NCY, Zhao QJ, Petersen DD, Lambert JC, Cote I, Yang L, Healy E, Black MB, et al. 2013. Temporal concordance between apical and transcriptional points of departure for chemical risk assessment. Toxicol Sci. 134(1):180–194.
- United States Environmental Protection Agency (US EPA) 2012. Benchmark Dose Technical Guidance. (EPA/100/R-12/001). Risk Assessment Forum, Washington, DC. Available online: http://www.epa.gov/raf/publications/pdfs/benchmark_dose_guidance.pdf.
- Webster AF, Zumbo P, Fostel J, Gandara J, Hester SD, Recio L, Williams A, Wood CE, Yauk CL, Mason CE, et al. 2015. Mining the archives: a cross-platform analysis of gene expression profiles in archival formalin-fixed paraffin-embedded tissues. Toxicol Sci. 148(2):460–472.